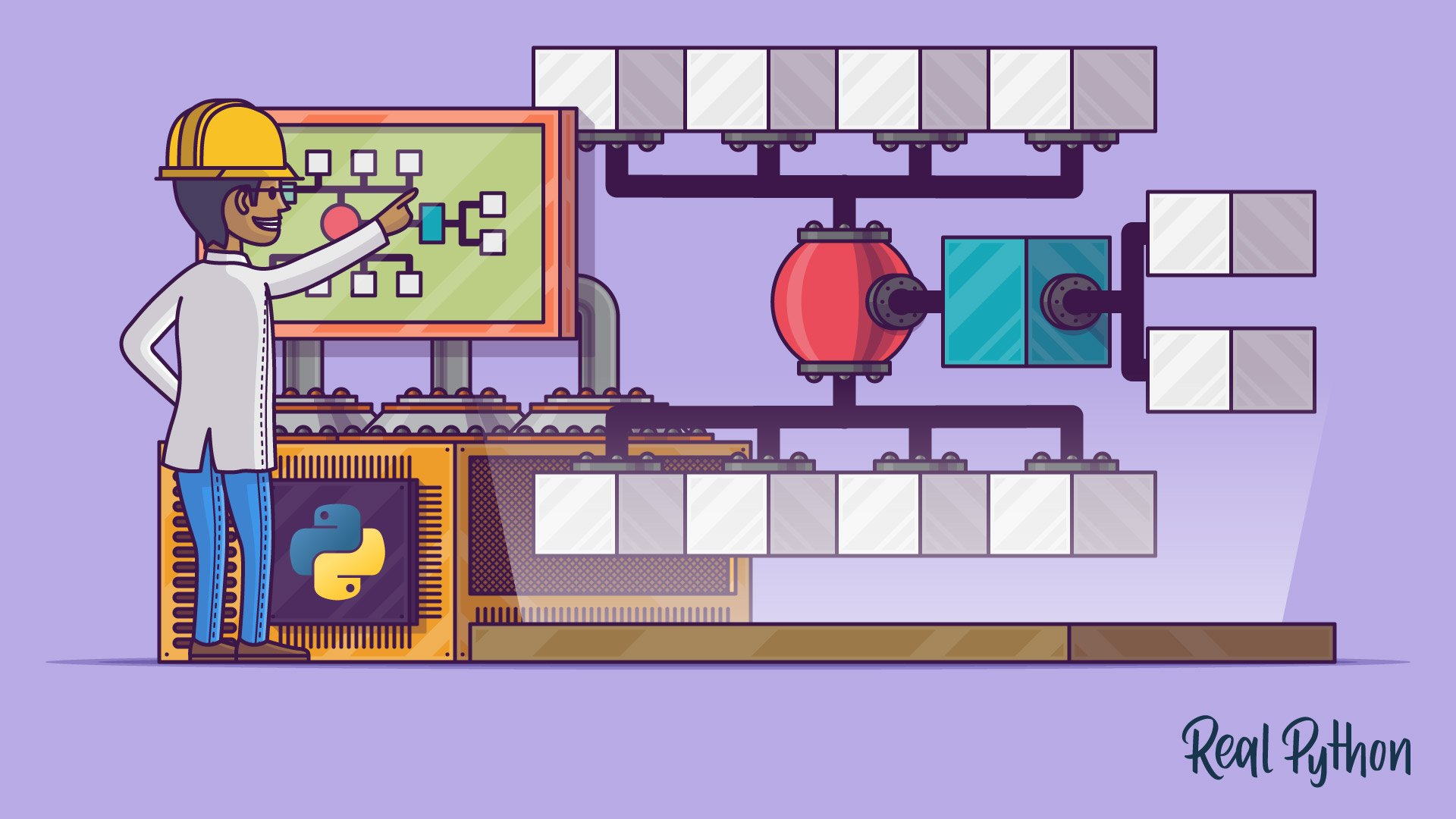

Common Python Data Structures (Guide)
Table of Contents
- dict: Your Go-to Dictionary
- collections.OrderedDict: Remember the Insertion Order of Keys
- collections.defaultdict: Return Default Values for Missing Keys
- collections.ChainMap: Search Multiple Dictionaries as a Single Mapping
- types.MappingProxyType: A Wrapper for Making Read-Only Dictionaries
Dictionaries in Python: Summary
- list: Mutable Dynamic Arrays
- tuple: Immutable Containers
- array.array: Basic Typed Arrays
- str: Immutable Arrays of Unicode Characters
- bytes: Immutable Arrays of Single Bytes
- bytearray: Mutable Arrays of Single Bytes
Arrays in Python: Summary
- dict: Simple Data Objects
- tuple: Immutable Groups of Objects
Write a Custom Class: More Work, More Control
- dataclasses.dataclass: Python 3.7+ Data Classes
- collections.namedtuple: Convenient Data Objects
- typing.NamedTuple: Improved Namedtuples
- struct.Struct: Serialized C Structs
- types.SimpleNamespace: Fancy Attribute Access
Records, Structs, and Data Objects in Python: Summary
- set: Your Go-to Set
- frozenset: Immutable Sets
- collections.Counter: Multisets
Sets and Multisets in Python: Summary
- list: Simple, Built-in Stacks
- collections.deque: Fast and Robust Stacks
- queue.LifoQueue: Locking Semantics for Parallel Computing
Stack Implementations in Python: Summary
- list: Terribly Sloooow Queues
- collections.deque: Fast and Robust Queues
- queue.Queue: Locking Semantics for Parallel Computing
- multiprocessing.Queue: Shared Job Queues
Queues in Python: Summary
- list: Manually Sorted Queues
- heapq: List-Based Binary Heaps
- queue.PriorityQueue: Beautiful Priority Queues
Priority Queues in Python: Summary
Conclusion: python data structures.
Watch Now This tutorial has a related video course created by the Real Python team. Watch it together with the written tutorial to deepen your understanding: Stacks and Queues: Selecting the Ideal Data Structure
Data structures are the fundamental constructs around which you build your programs. Each data structure provides a particular way of organizing data so it can be accessed efficiently, depending on your use case. Python ships with an extensive set of data structures in its standard library .
However, Python’s naming convention doesn’t provide the same level of clarity that you’ll find in other languages. In Java , a list isn’t just a list —it’s either a LinkedList or an ArrayList . Not so in Python. Even experienced Python developers sometimes wonder whether the built-in list type is implemented as a linked list or a dynamic array.
In this tutorial, you’ll learn:
- Which common abstract data types are built into the Python standard library
- How the most common abstract data types map to Python’s naming scheme
- How to put abstract data types to practical use in various algorithms
Note: This tutorial is adapted from the chapter “Common Data Structures in Python” in Python Tricks: The Book . If you enjoy what you read below, then be sure to check out the rest of the book .
Free Download: Get a sample chapter from Python Tricks: The Book that shows you Python’s best practices with simple examples you can apply instantly to write more beautiful + Pythonic code.
Dictionaries, Maps, and Hash Tables
In Python, dictionaries (or dicts for short) are a central data structure. Dicts store an arbitrary number of objects, each identified by a unique dictionary key .
Dictionaries are also often called maps , hashmaps , lookup tables , or associative arrays . They allow for the efficient lookup, insertion, and deletion of any object associated with a given key.
Phone books make a decent real-world analog for dictionary objects. They allow you to quickly retrieve the information (phone number) associated with a given key (a person’s name). Instead of having to read a phone book front to back to find someone’s number, you can jump more or less directly to a name and look up the associated information.
This analogy breaks down somewhat when it comes to how the information is organized to allow for fast lookups. But the fundamental performance characteristics hold. Dictionaries allow you to quickly find the information associated with a given key.
Dictionaries are one of the most important and frequently used data structures in computer science. So, how does Python handle dictionaries? Let’s take a tour of the dictionary implementations available in core Python and the Python standard library.
dict : Your Go-to Dictionary
Because dictionaries are so important, Python features a robust dictionary implementation that’s built directly into the core language: the dict data type.
Python also provides some useful syntactic sugar for working with dictionaries in your programs. For example, the curly-brace ({ }) dictionary expression syntax and dictionary comprehensions allow you to conveniently define new dictionary objects:
There are some restrictions on which objects can be used as valid keys.
Python’s dictionaries are indexed by keys that can be of any hashable type. A hashable object has a hash value that never changes during its lifetime (see __hash__ ), and it can be compared to other objects (see __eq__ ). Hashable objects that compare as equal must have the same hash value.
Immutable types like strings and numbers are hashable and work well as dictionary keys. You can also use tuple objects as dictionary keys as long as they contain only hashable types themselves.
For most use cases, Python’s built-in dictionary implementation will do everything you need. Dictionaries are highly optimized and underlie many parts of the language. For example, class attributes and variables in a stack frame are both stored internally in dictionaries.
Python dictionaries are based on a well-tested and finely tuned hash table implementation that provides the performance characteristics you’d expect: O (1) time complexity for lookup, insert, update, and delete operations in the average case.
There’s little reason not to use the standard dict implementation included with Python. However, specialized third-party dictionary implementations exist, such as skip lists or B-tree–based dictionaries.
Besides plain dict objects, Python’s standard library also includes a number of specialized dictionary implementations. These specialized dictionaries are all based on the built-in dictionary class (and share its performance characteristics) but also include some additional convenience features.
Let’s take a look at them.
collections.OrderedDict : Remember the Insertion Order of Keys
Python includes a specialized dict subclass that remembers the insertion order of keys added to it: collections.OrderedDict .
Note: OrderedDict is not a built-in part of the core language and must be imported from the collections module in the standard library.
While standard dict instances preserve the insertion order of keys in CPython 3.6 and above, this was simply a side effect of the CPython implementation and was not defined in the language spec until Python 3.7. So, if key order is important for your algorithm to work, then it’s best to communicate this clearly by explicitly using the OrderedDict class:
Until Python 3.8 , you couldn’t iterate over dictionary items in reverse order using reversed() . Only OrderedDict instances offered that functionality. Even in Python 3.8, dict and OrderedDict objects aren’t exactly the same. OrderedDict instances have a .move_to_end() method that is unavailable on plain dict instance, as well as a more customizable .popitem() method than the one plain dict instances.
collections.defaultdict : Return Default Values for Missing Keys
The defaultdict class is another dictionary subclass that accepts a callable in its constructor whose return value will be used if a requested key cannot be found.
This can save you some typing and make your intentions clearer as compared to using get() or catching a KeyError exception in regular dictionaries:
collections.ChainMap : Search Multiple Dictionaries as a Single Mapping
The collections.ChainMap data structure groups multiple dictionaries into a single mapping. Lookups search the underlying mappings one by one until a key is found. Insertions, updates, and deletions only affect the first mapping added to the chain:
types.MappingProxyType : A Wrapper for Making Read-Only Dictionaries
MappingProxyType is a wrapper around a standard dictionary that provides a read-only view into the wrapped dictionary’s data. This class was added in Python 3.3 and can be used to create immutable proxy versions of dictionaries.
MappingProxyType can be helpful if, for example, you’d like to return a dictionary carrying internal state from a class or module while discouraging write access to this object. Using MappingProxyType allows you to put these restrictions in place without first having to create a full copy of the dictionary:
All the Python dictionary implementations listed in this tutorial are valid implementations that are built into the Python standard library.
If you’re looking for a general recommendation on which mapping type to use in your programs, I’d point you to the built-in dict data type. It’s a versatile and optimized hash table implementation that’s built directly into the core language.
I would recommend that you use one of the other data types listed here only if you have special requirements that go beyond what’s provided by dict .
All the implementations are valid options, but your code will be clearer and easier to maintain if it relies on standard Python dictionaries most of the time.
Array Data Structures
An array is a fundamental data structure available in most programming languages, and it has a wide range of uses across different algorithms.
In this section, you’ll take a look at array implementations in Python that use only core language features or functionality that’s included in the Python standard library. You’ll see the strengths and weaknesses of each approach so you can decide which implementation is right for your use case.
But before we jump in, let’s cover some of the basics first. How do arrays work, and what are they used for? Arrays consist of fixed-size data records that allow each element to be efficiently located based on its index:
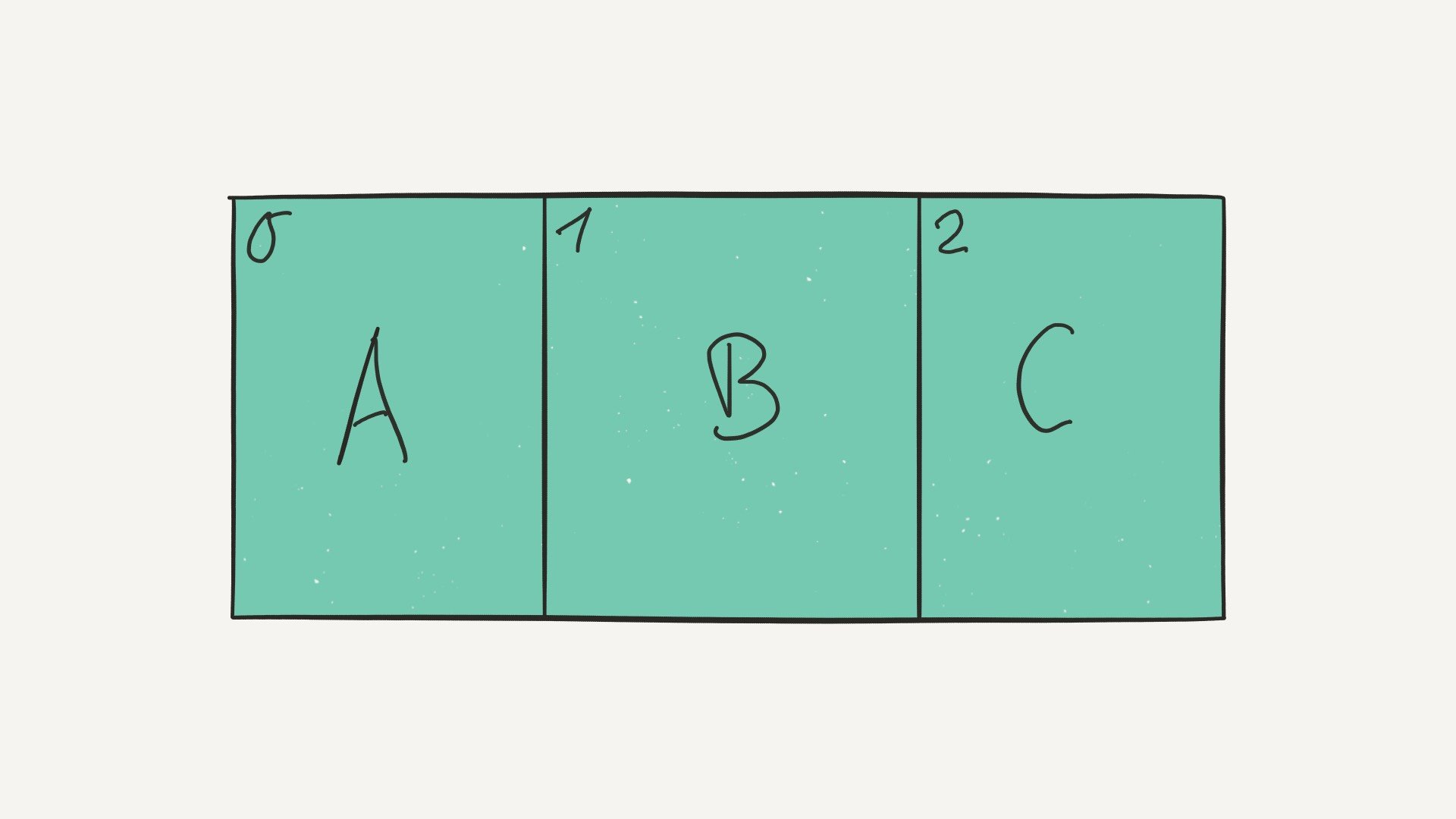
Because arrays store information in adjoining blocks of memory, they’re considered contiguous data structures (as opposed to linked data structures like linked lists, for example).
A real-world analogy for an array data structure is a parking lot. You can look at the parking lot as a whole and treat it as a single object, but inside the lot there are parking spots indexed by a unique number. Parking spots are containers for vehicles—each parking spot can either be empty or have a car, a motorbike, or some other vehicle parked on it.
But not all parking lots are the same. Some parking lots may be restricted to only one type of vehicle. For example, a motor home parking lot wouldn’t allow bikes to be parked on it. A restricted parking lot corresponds to a typed array data structure that allows only elements that have the same data type stored in them.
Performance-wise, it’s very fast to look up an element contained in an array given the element’s index. A proper array implementation guarantees a constant O (1) access time for this case.
Python includes several array-like data structures in its standard library that each have slightly different characteristics. Let’s take a look.
list : Mutable Dynamic Arrays
Lists are a part of the core Python language. Despite their name, Python’s lists are implemented as dynamic arrays behind the scenes.
This means a list allows elements to be added or removed, and the list will automatically adjust the backing store that holds these elements by allocating or releasing memory.
Python lists can hold arbitrary elements—everything is an object in Python, including functions. Therefore, you can mix and match different kinds of data types and store them all in a single list.
This can be a powerful feature, but the downside is that supporting multiple data types at the same time means that data is generally less tightly packed. As a result, the whole structure takes up more space:
tuple : Immutable Containers
Just like lists, tuples are part of the Python core language. Unlike lists, however, Python’s tuple objects are immutable. This means elements can’t be added or removed dynamically—all elements in a tuple must be defined at creation time.
Tuples are another data structure that can hold elements of arbitrary data types. Having this flexibility is powerful, but again, it also means that data is less tightly packed than it would be in a typed array:
array.array : Basic Typed Arrays
Python’s array module provides space-efficient storage of basic C-style data types like bytes, 32-bit integers, floating-point numbers, and so on.
Arrays created with the array.array class are mutable and behave similarly to lists except for one important difference: they’re typed arrays constrained to a single data type.
Because of this constraint, array.array objects with many elements are more space efficient than lists and tuples. The elements stored in them are tightly packed, and this can be useful if you need to store many elements of the same type.
Also, arrays support many of the same methods as regular lists, and you might be able to use them as a drop-in replacement without requiring other changes to your application code:
str : Immutable Arrays of Unicode Characters
Python 3.x uses str objects to store textual data as immutable sequences of Unicode characters . Practically speaking, that means a str is an immutable array of characters. Oddly enough, it’s also a recursive data structure—each character in a string is itself a str object of length 1.
String objects are space efficient because they’re tightly packed and they specialize in a single data type. If you’re storing Unicode text, then you should use a string.
Because strings are immutable in Python, modifying a string requires creating a modified copy. The closest equivalent to a mutable string is storing individual characters inside a list:
bytes : Immutable Arrays of Single Bytes
bytes objects are immutable sequences of single bytes, or integers in the range 0 ≤ x ≤ 255. Conceptually, bytes objects are similar to str objects, and you can also think of them as immutable arrays of bytes.
Like strings, bytes have their own literal syntax for creating objects and are space efficient. bytes objects are immutable, but unlike strings, there’s a dedicated mutable byte array data type called bytearray that they can be unpacked into:
bytearray : Mutable Arrays of Single Bytes
The bytearray type is a mutable sequence of integers in the range 0 ≤ x ≤ 255. The bytearray object is closely related to the bytes object, with the main difference being that a bytearray can be modified freely—you can overwrite elements, remove existing elements, or add new ones. The bytearray object will grow and shrink accordingly.
A bytearray can be converted back into immutable bytes objects, but this involves copying the stored data in full—a slow operation taking O ( n ) time:
There are a number of built-in data structures you can choose from when it comes to implementing arrays in Python. In this section, you’ve focused on core language features and data structures included in the standard library.
If you’re willing to go beyond the Python standard library, then third-party packages like NumPy and pandas offer a wide range of fast array implementations for scientific computing and data science.
If you want to restrict yourself to the array data structures included with Python, then here are a few guidelines:
If you need to store arbitrary objects, potentially with mixed data types, then use a list or a tuple , depending on whether or not you want an immutable data structure.
If you have numeric (integer or floating-point) data and tight packing and performance is important, then try out array.array .
If you have textual data represented as Unicode characters, then use Python’s built-in str . If you need a mutable string-like data structure, then use a list of characters.
If you want to store a contiguous block of bytes, then use the immutable bytes type or a bytearray if you need a mutable data structure.
In most cases, I like to start out with a simple list . I’ll only specialize later on if performance or storage space becomes an issue. Most of the time, using a general-purpose array data structure like list gives you the fastest development speed and the most programming convenience.
I’ve found that this is usually much more important in the beginning than trying to squeeze out every last drop of performance right from the start.
Records, Structs, and Data Transfer Objects
Compared to arrays, record data structures provide a fixed number of fields. Each field can have a name and may also have a different type.
In this section, you’ll see how to implement records, structs, and plain old data objects in Python using only built-in data types and classes from the standard library.
Note: I’m using the definition of a record loosely here. For example, I’m also going to discuss types like Python’s built-in tuple that may or may not be considered records in a strict sense because they don’t provide named fields.
Python offers several data types that you can use to implement records, structs, and data transfer objects. In this section, you’ll get a quick look at each implementation and its unique characteristics. At the end, you’ll find a summary and a decision-making guide that will help you make your own picks.
Note: This tutorial is adapted from the chapter “Common Data Structures in Python” in Python Tricks: The Book . If you enjoy what you’re reading, then be sure to check out the rest of the book .
Alright, let’s get started!
dict : Simple Data Objects
As mentioned previously , Python dictionaries store an arbitrary number of objects, each identified by a unique key. Dictionaries are also often called maps or associative arrays and allow for efficient lookup, insertion, and deletion of any object associated with a given key.
Using dictionaries as a record data type or data object in Python is possible. Dictionaries are easy to create in Python as they have their own syntactic sugar built into the language in the form of dictionary literals . The dictionary syntax is concise and quite convenient to type.
Data objects created using dictionaries are mutable, and there’s little protection against misspelled field names as fields can be added and removed freely at any time. Both of these properties can introduce surprising bugs, and there’s always a trade-off to be made between convenience and error resilience:
tuple : Immutable Groups of Objects
Python’s tuples are a straightforward data structure for grouping arbitrary objects. Tuples are immutable—they can’t be modified once they’ve been created.
Performance-wise, tuples take up slightly less memory than lists in CPython , and they’re also faster to construct.
As you can see in the bytecode disassembly below, constructing a tuple constant takes a single LOAD_CONST opcode, while constructing a list object with the same contents requires several more operations:
However, you shouldn’t place too much emphasis on these differences. In practice, the performance difference will often be negligible, and trying to squeeze extra performance out of a program by switching from lists to tuples will likely be the wrong approach.
A potential downside of plain tuples is that the data you store in them can only be pulled out by accessing it through integer indexes. You can’t give names to individual properties stored in a tuple. This can impact code readability.
Also, a tuple is always an ad-hoc structure: it’s difficult to ensure that two tuples have the same number of fields and the same properties stored in them.
This makes it easy to introduce slip-of-the-mind bugs, such as mixing up the field order. Therefore, I would recommend that you keep the number of fields stored in a tuple as low as possible:
Classes allow you to define reusable blueprints for data objects to ensure each object provides the same set of fields.
Using regular Python classes as record data types is feasible, but it also takes manual work to get the convenience features of other implementations. For example, adding new fields to the __init__ constructor is verbose and takes time.
Also, the default string representation for objects instantiated from custom classes isn’t very helpful. To fix that, you may have to add your own __repr__ method, which again is usually quite verbose and must be updated each time you add a new field.
Fields stored on classes are mutable, and new fields can be added freely, which you may or may not like. It’s possible to provide more access control and to create read-only fields using the @property decorator, but once again, this requires writing more glue code.
Writing a custom class is a great option whenever you’d like to add business logic and behavior to your record objects using methods. However, this means that these objects are technically no longer plain data objects:
dataclasses.dataclass : Python 3.7+ Data Classes
Data classes are available in Python 3.7 and above. They provide an excellent alternative to defining your own data storage classes from scratch.
By writing a data class instead of a plain Python class, your object instances get a few useful features out of the box that will save you some typing and manual implementation work:
- The syntax for defining instance variables is shorter, since you don’t need to implement the .__init__() method.
- Instances of your data class automatically get nice-looking string representation via an auto-generated .__repr__() method.
- Instance variables accept type annotations, making your data class self-documenting to a degree. Keep in mind that type annotations are just hints that are not enforced without a separate type-checking tool.
Data classes are typically created using the @dataclass decorator , as you’ll see in the code example below:
To learn more about Python data classes, check out the The Ultimate Guide to Data Classes in Python 3.7 .
collections.namedtuple : Convenient Data Objects
The namedtuple class available in Python 2.6+ provides an extension of the built-in tuple data type. Similar to defining a custom class, using namedtuple allows you to define reusable blueprints for your records that ensure the correct field names are used.
namedtuple objects are immutable, just like regular tuples. This means you can’t add new fields or modify existing fields after the namedtuple instance is created.
Besides that, namedtuple objects are, well . . . named tuples. Each object stored in them can be accessed through a unique identifier. This frees you from having to remember integer indexes or resort to workarounds like defining integer constants as mnemonics for your indexes.
namedtuple objects are implemented as regular Python classes internally. When it comes to memory usage, they’re also better than regular classes and just as memory efficient as regular tuples:
namedtuple objects can be an easy way to clean up your code and make it more readable by enforcing a better structure for your data.
I find that going from ad-hoc data types like dictionaries with a fixed format to namedtuple objects helps me to express the intent of my code more clearly. Often when I apply this refactoring, I magically come up with a better solution for the problem I’m facing.
Using namedtuple objects over regular (unstructured) tuples and dicts can also make your coworkers’ lives easier by making the data that’s being passed around self-documenting, at least to a degree:
typing.NamedTuple : Improved Namedtuples
Added in Python 3.6, typing.NamedTuple is the younger sibling of the namedtuple class in the collections module. It’s very similar to namedtuple , with the main difference being an updated syntax for defining new record types and added support for type hints .
Please note that type annotations are not enforced without a separate type-checking tool like mypy . But even without tool support, they can provide useful hints for other programmers (or be terribly confusing if the type hints become out of date):
struct.Struct : Serialized C Structs
The struct.Struct class converts between Python values and C structs serialized into Python bytes objects. For example, it can be used to handle binary data stored in files or coming in from network connections.
Structs are defined using a mini language based on format strings that allows you to define the arrangement of various C data types like char , int , and long as well as their unsigned variants.
Serialized structs are seldom used to represent data objects meant to be handled purely inside Python code. They’re intended primarily as a data exchange format rather than as a way of holding data in memory that’s only used by Python code.
In some cases, packing primitive data into structs may use less memory than keeping it in other data types. However, in most cases that would be quite an advanced (and probably unnecessary) optimization:
types.SimpleNamespace : Fancy Attribute Access
Here’s one more slightly obscure choice for implementing data objects in Python: types.SimpleNamespace . This class was added in Python 3.3 and provides attribute access to its namespace.
This means SimpleNamespace instances expose all of their keys as class attributes. You can use obj.key dotted attribute access instead of the obj['key'] square-bracket indexing syntax that’s used by regular dicts. All instances also include a meaningful __repr__ by default.
As its name proclaims, SimpleNamespace is simple! It’s basically a dictionary that allows attribute access and prints nicely. Attributes can be added, modified, and deleted freely:
As you’ve seen, there’s quite a number of different options for implementing records or data objects. Which type should you use for data objects in Python? Generally your decision will depend on your use case:
If you have only a few fields, then using a plain tuple object may be okay if the field order is easy to remember or field names are superfluous. For example, think of an (x, y, z) point in three-dimensional space.
If you need immutable fields, then plain tuples, collections.namedtuple , and typing.NamedTuple are all good options.
If you need to lock down field names to avoid typos, then collections.namedtuple and typing.NamedTuple are your friends.
If you want to keep things simple, then a plain dictionary object might be a good choice due to the convenient syntax that closely resembles JSON .
If you need full control over your data structure, then it’s time to write a custom class with @property setters and getters .
If you need to add behavior (methods) to the object, then you should write a custom class, either from scratch, or using the dataclass decorator, or by extending collections.namedtuple or typing.NamedTuple .
If you need to pack data tightly to serialize it to disk or to send it over the network, then it’s time to read up on struct.Struct because this is a great use case for it!
If you’re looking for a safe default choice, then my general recommendation for implementing a plain record, struct, or data object in Python would be to use collections.namedtuple in Python 2.x and its younger sibling, typing.NamedTuple in Python 3.
Sets and Multisets
In this section, you’ll see how to implement mutable and immutable set and multiset (bag) data structures in Python using built-in data types and classes from the standard library.
A set is an unordered collection of objects that doesn’t allow duplicate elements. Typically, sets are used to quickly test a value for membership in the set, to insert or delete new values from a set, and to compute the union or intersection of two sets.
In a proper set implementation, membership tests are expected to run in fast O (1) time. Union, intersection, difference, and subset operations should take O ( n ) time on average. The set implementations included in Python’s standard library follow these performance characteristics .
Just like dictionaries, sets get special treatment in Python and have some syntactic sugar that makes them easy to create. For example, the curly-brace set expression syntax and set comprehensions allow you to conveniently define new set instances:
But be careful: To create an empty set you’ll need to call the set() constructor. Using empty curly-braces ( {} ) is ambiguous and will create an empty dictionary instead.
Python and its standard library provide several set implementations. Let’s have a look at them.
set : Your Go-to Set
The set type is the built-in set implementation in Python. It’s mutable and allows for the dynamic insertion and deletion of elements.
Python’s sets are backed by the dict data type and share the same performance characteristics. Any hashable object can be stored in a set:
frozenset : Immutable Sets
The frozenset class implements an immutable version of set that can’t be changed after it’s been constructed.
frozenset objects are static and allow only query operations on their elements, not inserts or deletions. Because frozenset objects are static and hashable, they can be used as dictionary keys or as elements of another set, something that isn’t possible with regular (mutable) set objects:
collections.Counter : Multisets
The collections.Counter class in the Python standard library implements a multiset, or bag, type that allows elements in the set to have more than one occurrence.
This is useful if you need to keep track of not only if an element is part of a set, but also how many times it’s included in the set:
One caveat for the Counter class is that you’ll want to be careful when counting the number of elements in a Counter object. Calling len() returns the number of unique elements in the multiset, whereas the total number of elements can be retrieved using sum() :
Sets are another useful and commonly used data structure included with Python and its standard library. Here are a few guidelines for deciding which one to use:
- If you need a mutable set, then use the built-in set type.
- If you need hashable objects that can be used as dictionary or set keys, then use a frozenset .
- If you need a multiset, or bag, data structure, then use collections.Counter .
Stacks (LIFOs)
A stack is a collection of objects that supports fast Last-In/First-Out (LIFO) semantics for inserts and deletes. Unlike lists or arrays, stacks typically don’t allow for random access to the objects they contain. The insert and delete operations are also often called push and pop .
A useful real-world analogy for a stack data structure is a stack of plates. New plates are added to the top of the stack, and because the plates are precious and heavy, only the topmost plate can be moved. In other words, the last plate on the stack must be the first one removed (LIFO). To reach the plates that are lower down in the stack, the topmost plates must be removed one by one.
Performance-wise, a proper stack implementation is expected to take O (1) time for insert and delete operations.
Stacks have a wide range of uses in algorithms. For example, they’re used in language parsing as well as runtime memory management, which relies on a call stack . A short and beautiful algorithm using a stack is depth-first search (DFS) on a tree or graph data structure.
Python ships with several stack implementations that each have slightly different characteristics. Let’s take a look at them and compare their characteristics.
list : Simple, Built-in Stacks
Python’s built-in list type makes a decent stack data structure as it supports push and pop operations in amortized O (1) time.
Python’s lists are implemented as dynamic arrays internally, which means they occasionally need to resize the storage space for elements stored in them when elements are added or removed. The list over-allocates its backing storage so that not every push or pop requires resizing. As a result, you get an amortized O (1) time complexity for these operations.
The downside is that this makes their performance less consistent than the stable O (1) inserts and deletes provided by a linked list–based implementation (as you’ll see below with collections.deque ). On the other hand, lists do provide fast O (1) time random access to elements on the stack, and this can be an added benefit.
There’s an important performance caveat that you should be aware of when using lists as stacks: To get the amortized O (1) performance for inserts and deletes, new items must be added to the end of the list with the append() method and removed again from the end using pop() . For optimum performance, stacks based on Python lists should grow towards higher indexes and shrink towards lower ones.
Adding and removing from the front is much slower and takes O ( n ) time, as the existing elements must be shifted around to make room for the new element. This is a performance antipattern that you should avoid as much as possible:
collections.deque : Fast and Robust Stacks
The deque class implements a double-ended queue that supports adding and removing elements from either end in O (1) time (non-amortized). Because deques support adding and removing elements from either end equally well, they can serve both as queues and as stacks.
Python’s deque objects are implemented as doubly-linked lists , which gives them excellent and consistent performance for inserting and deleting elements but poor O ( n ) performance for randomly accessing elements in the middle of a stack.
Overall, collections.deque is a great choice if you’re looking for a stack data structure in Python’s standard library that has the performance characteristics of a linked-list implementation:
queue.LifoQueue : Locking Semantics for Parallel Computing
The LifoQueue stack implementation in the Python standard library is synchronized and provides locking semantics to support multiple concurrent producers and consumers.
Besides LifoQueue , the queue module contains several other classes that implement multi-producer, multi-consumer queues that are useful for parallel computing.
Depending on your use case, the locking semantics might be helpful, or they might just incur unneeded overhead. In this case, you’d be better off using a list or a deque as a general-purpose stack:
As you’ve seen, Python ships with several implementations for a stack data structure. All of them have slightly different characteristics as well as performance and usage trade-offs.
If you’re not looking for parallel processing support (or if you don’t want to handle locking and unlocking manually), then your choice comes down to the built-in list type or collections.deque . The difference lies in the data structure used behind the scenes and overall ease of use.
list is backed by a dynamic array, which makes it great for fast random access but requires occasional resizing when elements are added or removed.
The list over-allocates its backing storage so that not every push or pop requires resizing, and you get an amortized O (1) time complexity for these operations. But you do need to be careful to only insert and remove items using append() and pop() . Otherwise, performance slows down to O ( n ).
collections.deque is backed by a doubly-linked list, which optimizes appends and deletes at both ends and provides consistent O (1) performance for these operations. Not only is its performance more stable, the deque class is also easier to use because you don’t have to worry about adding or removing items from the wrong end.
In summary, collections.deque is an excellent choice for implementing a stack (LIFO queue) in Python.
Queues (FIFOs)
In this section, you’ll see how to implement a First-In/First-Out (FIFO) queue data structure using only built-in data types and classes from the Python standard library.
A queue is a collection of objects that supports fast FIFO semantics for inserts and deletes. The insert and delete operations are sometimes called enqueue and dequeue . Unlike lists or arrays, queues typically don’t allow for random access to the objects they contain.
Here’s a real-world analogy for a FIFO queue:
Imagine a line of Pythonistas waiting to pick up their conference badges on day one of PyCon registration. As new people enter the conference venue and queue up to receive their badges, they join the line (enqueue) at the back of the queue. Developers receive their badges and conference swag bags and then exit the line (dequeue) at the front of the queue.
Another way to memorize the characteristics of a queue data structure is to think of it as a pipe. You add ping-pong balls to one end, and they travel to the other end, where you remove them. While the balls are in the queue (a solid metal pipe) you can’t get at them. The only way to interact with the balls in the queue is to add new ones at the back of the pipe (enqueue) or to remove them at the front (dequeue).
Queues are similar to stacks. The difference between them lies in how items are removed. With a queue , you remove the item least recently added (FIFO) but with a stack , you remove the item most recently added (LIFO).
Performance-wise, a proper queue implementation is expected to take O (1) time for insert and delete operations. These are the two main operations performed on a queue, and in a correct implementation, they should be fast.
Queues have a wide range of applications in algorithms and often help solve scheduling and parallel programming problems. A short and beautiful algorithm using a queue is breadth-first search (BFS) on a tree or graph data structure.
Scheduling algorithms often use priority queues internally. These are specialized queues. Instead of retrieving the next element by insertion time, a priority queue retrieves the highest-priority element. The priority of individual elements is decided by the queue based on the ordering applied to their keys.
A regular queue, however, won’t reorder the items it carries. Just like in the pipe example, you get out what you put in, and in exactly that order.
Python ships with several queue implementations that each have slightly different characteristics. Let’s review them.
list : Terribly Sloooow Queues
It’s possible to use a regular list as a queue , but this is not ideal from a performance perspective. Lists are quite slow for this purpose because inserting or deleting an element at the beginning requires shifting all the other elements by one, requiring O ( n ) time.
Therefore, I would not recommend using a list as a makeshift queue in Python unless you’re dealing with only a small number of elements:
collections.deque : Fast and Robust Queues
Python’s deque objects are implemented as doubly-linked lists. This gives them excellent and consistent performance for inserting and deleting elements, but poor O ( n ) performance for randomly accessing elements in the middle of the stack.
As a result, collections.deque is a great default choice if you’re looking for a queue data structure in Python’s standard library:
queue.Queue : Locking Semantics for Parallel Computing
The queue.Queue implementation in the Python standard library is synchronized and provides locking semantics to support multiple concurrent producers and consumers.
The queue module contains several other classes implementing multi-producer, multi-consumer queues that are useful for parallel computing.
Depending on your use case, the locking semantics might be helpful or just incur unneeded overhead. In this case, you’d be better off using collections.deque as a general-purpose queue:
multiprocessing.Queue : Shared Job Queues
multiprocessing.Queue is a shared job queue implementation that allows queued items to be processed in parallel by multiple concurrent workers. Process-based parallelization is popular in CPython due to the global interpreter lock (GIL) that prevents some forms of parallel execution on a single interpreter process.
As a specialized queue implementation meant for sharing data between processes, multiprocessing.Queue makes it easy to distribute work across multiple processes in order to work around the GIL limitations. This type of queue can store and transfer any pickleable object across process boundaries:
Python includes several queue implementations as part of the core language and its standard library.
list objects can be used as queues, but this is generally not recommended due to slow performance.
If you’re not looking for parallel processing support, then the implementation offered by collections.deque is an excellent default choice for implementing a FIFO queue data structure in Python. It provides the performance characteristics you’d expect from a good queue implementation and can also be used as a stack (LIFO queue).
Priority Queues
A priority queue is a container data structure that manages a set of records with totally-ordered keys to provide quick access to the record with the smallest or largest key in the set.
You can think of a priority queue as a modified queue. Instead of retrieving the next element by insertion time, it retrieves the highest-priority element. The priority of individual elements is decided by the order applied to their keys.
Priority queues are commonly used for dealing with scheduling problems. For example, you might use them to give precedence to tasks with higher urgency.
Think about the job of an operating system task scheduler:
Ideally, higher-priority tasks on the system (such as playing a real-time game) should take precedence over lower-priority tasks (such as downloading updates in the background). By organizing pending tasks in a priority queue that uses task urgency as the key, the task scheduler can quickly select the highest-priority tasks and allow them to run first.
In this section, you’ll see a few options for how you can implement priority queues in Python using built-in data structures or data structures included in Python’s standard library. Each implementation will have its own upsides and downsides, but in my mind there’s a clear winner for most common scenarios. Let’s find out which one it is.
list : Manually Sorted Queues
You can use a sorted list to quickly identify and delete the smallest or largest element. The downside is that inserting new elements into a list is a slow O ( n ) operation.
While the insertion point can be found in O (log n ) time using bisect.insort in the standard library, this is always dominated by the slow insertion step.
Maintaining the order by appending to the list and re-sorting also takes at least O ( n log n ) time. Another downside is that you must manually take care of re-sorting the list when new elements are inserted. It’s easy to introduce bugs by missing this step, and the burden is always on you, the developer.
This means sorted lists are only suitable as priority queues when there will be few insertions:
heapq : List-Based Binary Heaps
heapq is a binary heap implementation usually backed by a plain list , and it supports insertion and extraction of the smallest element in O (log n ) time.
This module is a good choice for implementing priority queues in Python . Since heapq technically provides only a min-heap implementation, extra steps must be taken to ensure sort stability and other features typically expected from a practical priority queue:
queue.PriorityQueue : Beautiful Priority Queues
queue.PriorityQueue uses heapq internally and shares the same time and space complexities. The difference is that PriorityQueue is synchronized and provides locking semantics to support multiple concurrent producers and consumers.
Depending on your use case, this might be helpful, or it might just slow your program down slightly. In any case, you might prefer the class-based interface provided by PriorityQueue over the function-based interface provided by heapq :
Python includes several priority queue implementations ready for you to use.
queue.PriorityQueue stands out from the pack with a nice object-oriented interface and a name that clearly states its intent. It should be your preferred choice.
If you’d like to avoid the locking overhead of queue.PriorityQueue , then using the heapq module directly is also a good option.
That concludes your tour of common data structures in Python. With the knowledge you’ve gained here, you’re ready to implement efficient data structures that are just right for your specific algorithm or use case.
In this tutorial, you’ve learned:
If you enjoyed what you learned in this sample from Python Tricks , then be sure to check out the rest of the book .
If you’re interested in brushing up on your general data structures knowledge, then I highly recommend Steven S. Skiena’s The Algorithm Design Manual . It strikes a great balance between teaching you fundamental (and more advanced) data structures and showing you how to implement them in your code. Steve’s book was a great help in the writing of this tutorial.
🐍 Python Tricks 💌
Get a short & sweet Python Trick delivered to your inbox every couple of days. No spam ever. Unsubscribe any time. Curated by the Real Python team.
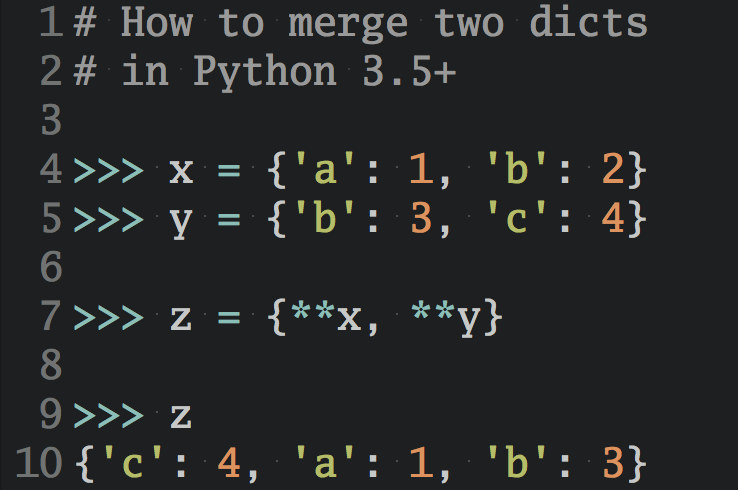
About Dan Bader
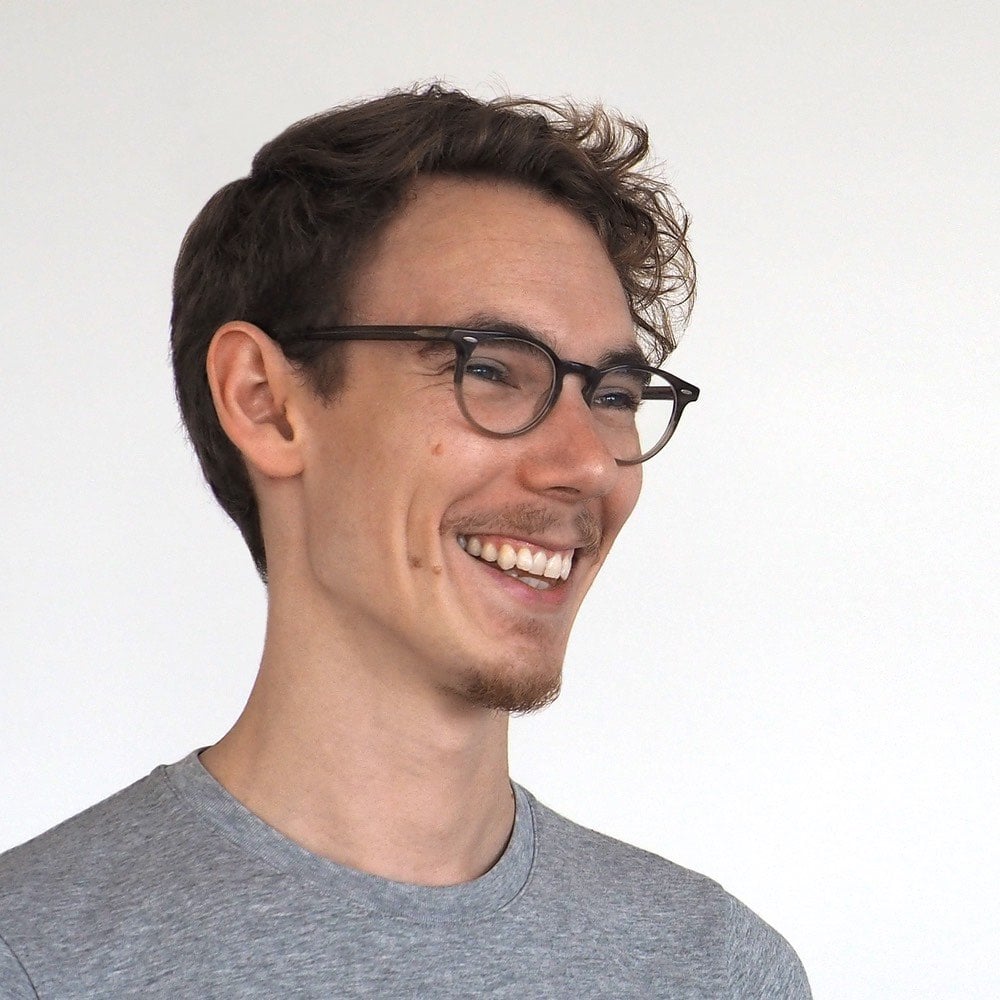
Dan Bader is the owner and editor in chief of Real Python and the main developer of the realpython.com learning platform. Dan has been writing code for more than 20 years and holds a master's degree in computer science.
Each tutorial at Real Python is created by a team of developers so that it meets our high quality standards. The team members who worked on this tutorial are:
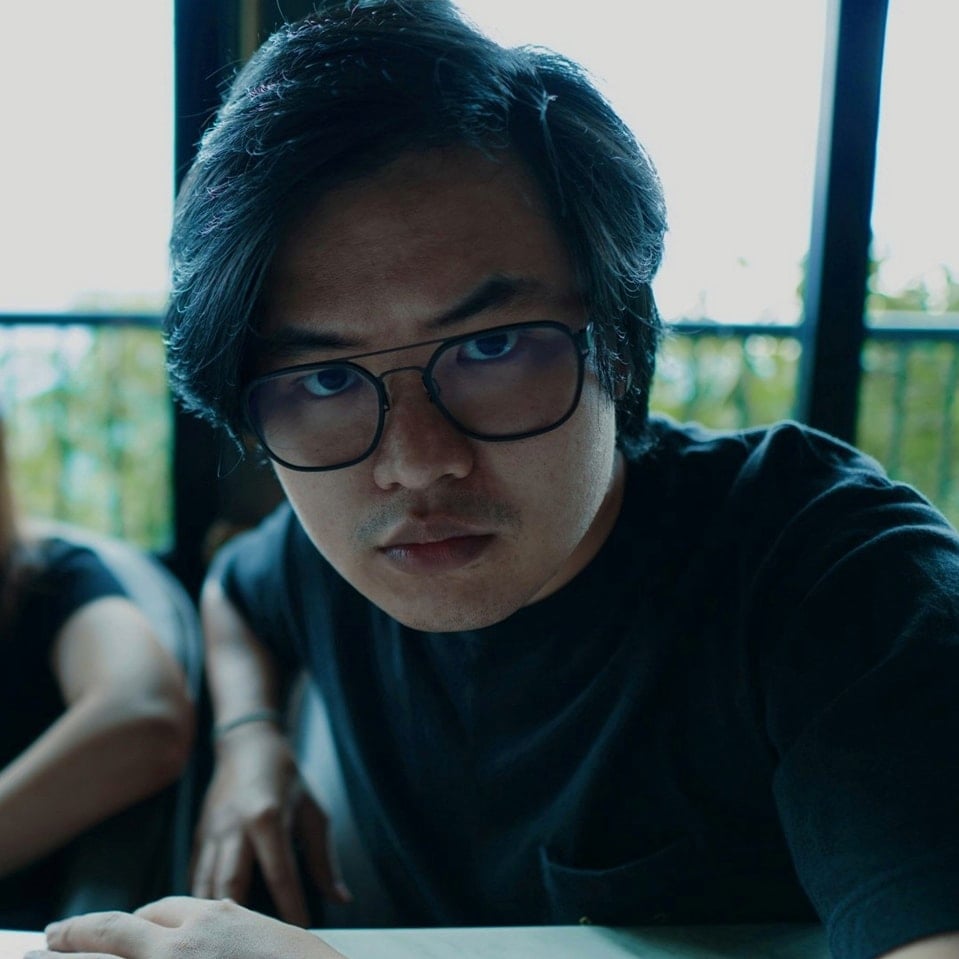
Master Real-World Python Skills With Unlimited Access to Real Python
Join us and get access to thousands of tutorials, hands-on video courses, and a community of expert Pythonistas:
Join us and get access to thousands of tutorials, hands-on video courses, and a community of expert Pythonistas:
What Do You Think?
What’s your #1 takeaway or favorite thing you learned? How are you going to put your newfound skills to use? Leave a comment below and let us know.
Commenting Tips: The most useful comments are those written with the goal of learning from or helping out other students. Get tips for asking good questions and get answers to common questions in our support portal . Looking for a real-time conversation? Visit the Real Python Community Chat or join the next “Office Hours” Live Q&A Session . Happy Pythoning!
Keep Learning
Related Topics: basics data-structures python
Recommended Video Course: Stacks and Queues: Selecting the Ideal Data Structure
Keep reading Real Python by creating a free account or signing in:
Already have an account? Sign-In
Almost there! Complete this form and click the button below to gain instant access:
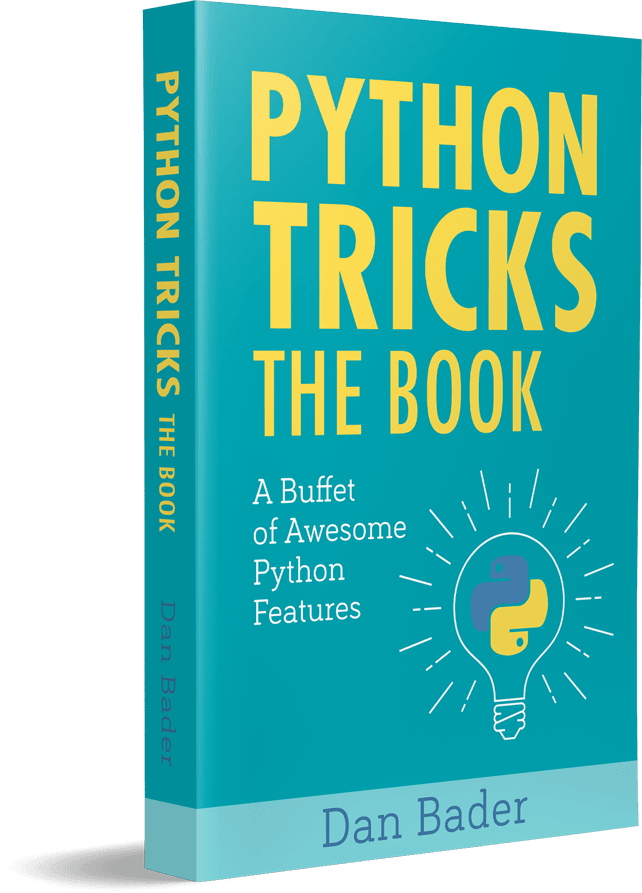
"Python Tricks: The Book" – Free Sample Chapter (PDF)
🔒 No spam. We take your privacy seriously.
- Python »
- 3.12.4 Documentation »
- The Python Tutorial »
- 5. Data Structures
- Theme Auto Light Dark |
5. Data Structures ¶
This chapter describes some things you’ve learned about already in more detail, and adds some new things as well.
5.1. More on Lists ¶
The list data type has some more methods. Here are all of the methods of list objects:
Add an item to the end of the list. Equivalent to a[len(a):] = [x] .
Extend the list by appending all the items from the iterable. Equivalent to a[len(a):] = iterable .
Insert an item at a given position. The first argument is the index of the element before which to insert, so a.insert(0, x) inserts at the front of the list, and a.insert(len(a), x) is equivalent to a.append(x) .
Remove the first item from the list whose value is equal to x . It raises a ValueError if there is no such item.
Remove the item at the given position in the list, and return it. If no index is specified, a.pop() removes and returns the last item in the list. It raises an IndexError if the list is empty or the index is outside the list range.
Remove all items from the list. Equivalent to del a[:] .
Return zero-based index in the list of the first item whose value is equal to x . Raises a ValueError if there is no such item.
The optional arguments start and end are interpreted as in the slice notation and are used to limit the search to a particular subsequence of the list. The returned index is computed relative to the beginning of the full sequence rather than the start argument.
Return the number of times x appears in the list.
Sort the items of the list in place (the arguments can be used for sort customization, see sorted() for their explanation).
Reverse the elements of the list in place.
Return a shallow copy of the list. Equivalent to a[:] .
An example that uses most of the list methods:
You might have noticed that methods like insert , remove or sort that only modify the list have no return value printed – they return the default None . [ 1 ] This is a design principle for all mutable data structures in Python.
Another thing you might notice is that not all data can be sorted or compared. For instance, [None, 'hello', 10] doesn’t sort because integers can’t be compared to strings and None can’t be compared to other types. Also, there are some types that don’t have a defined ordering relation. For example, 3+4j < 5+7j isn’t a valid comparison.
5.1.1. Using Lists as Stacks ¶
The list methods make it very easy to use a list as a stack, where the last element added is the first element retrieved (“last-in, first-out”). To add an item to the top of the stack, use append() . To retrieve an item from the top of the stack, use pop() without an explicit index. For example:
5.1.2. Using Lists as Queues ¶
It is also possible to use a list as a queue, where the first element added is the first element retrieved (“first-in, first-out”); however, lists are not efficient for this purpose. While appends and pops from the end of list are fast, doing inserts or pops from the beginning of a list is slow (because all of the other elements have to be shifted by one).
To implement a queue, use collections.deque which was designed to have fast appends and pops from both ends. For example:
5.1.3. List Comprehensions ¶
List comprehensions provide a concise way to create lists. Common applications are to make new lists where each element is the result of some operations applied to each member of another sequence or iterable, or to create a subsequence of those elements that satisfy a certain condition.
For example, assume we want to create a list of squares, like:
Note that this creates (or overwrites) a variable named x that still exists after the loop completes. We can calculate the list of squares without any side effects using:
or, equivalently:
which is more concise and readable.
A list comprehension consists of brackets containing an expression followed by a for clause, then zero or more for or if clauses. The result will be a new list resulting from evaluating the expression in the context of the for and if clauses which follow it. For example, this listcomp combines the elements of two lists if they are not equal:
and it’s equivalent to:
Note how the order of the for and if statements is the same in both these snippets.
If the expression is a tuple (e.g. the (x, y) in the previous example), it must be parenthesized.
List comprehensions can contain complex expressions and nested functions:
5.1.4. Nested List Comprehensions ¶
The initial expression in a list comprehension can be any arbitrary expression, including another list comprehension.
Consider the following example of a 3x4 matrix implemented as a list of 3 lists of length 4:
The following list comprehension will transpose rows and columns:
As we saw in the previous section, the inner list comprehension is evaluated in the context of the for that follows it, so this example is equivalent to:
which, in turn, is the same as:
In the real world, you should prefer built-in functions to complex flow statements. The zip() function would do a great job for this use case:
See Unpacking Argument Lists for details on the asterisk in this line.
5.2. The del statement ¶
There is a way to remove an item from a list given its index instead of its value: the del statement. This differs from the pop() method which returns a value. The del statement can also be used to remove slices from a list or clear the entire list (which we did earlier by assignment of an empty list to the slice). For example:
del can also be used to delete entire variables:
Referencing the name a hereafter is an error (at least until another value is assigned to it). We’ll find other uses for del later.
5.3. Tuples and Sequences ¶
We saw that lists and strings have many common properties, such as indexing and slicing operations. They are two examples of sequence data types (see Sequence Types — list, tuple, range ). Since Python is an evolving language, other sequence data types may be added. There is also another standard sequence data type: the tuple .
A tuple consists of a number of values separated by commas, for instance:
As you see, on output tuples are always enclosed in parentheses, so that nested tuples are interpreted correctly; they may be input with or without surrounding parentheses, although often parentheses are necessary anyway (if the tuple is part of a larger expression). It is not possible to assign to the individual items of a tuple, however it is possible to create tuples which contain mutable objects, such as lists.
Though tuples may seem similar to lists, they are often used in different situations and for different purposes. Tuples are immutable , and usually contain a heterogeneous sequence of elements that are accessed via unpacking (see later in this section) or indexing (or even by attribute in the case of namedtuples ). Lists are mutable , and their elements are usually homogeneous and are accessed by iterating over the list.
A special problem is the construction of tuples containing 0 or 1 items: the syntax has some extra quirks to accommodate these. Empty tuples are constructed by an empty pair of parentheses; a tuple with one item is constructed by following a value with a comma (it is not sufficient to enclose a single value in parentheses). Ugly, but effective. For example:
The statement t = 12345, 54321, 'hello!' is an example of tuple packing : the values 12345 , 54321 and 'hello!' are packed together in a tuple. The reverse operation is also possible:
This is called, appropriately enough, sequence unpacking and works for any sequence on the right-hand side. Sequence unpacking requires that there are as many variables on the left side of the equals sign as there are elements in the sequence. Note that multiple assignment is really just a combination of tuple packing and sequence unpacking.
5.4. Sets ¶
Python also includes a data type for sets . A set is an unordered collection with no duplicate elements. Basic uses include membership testing and eliminating duplicate entries. Set objects also support mathematical operations like union, intersection, difference, and symmetric difference.
Curly braces or the set() function can be used to create sets. Note: to create an empty set you have to use set() , not {} ; the latter creates an empty dictionary, a data structure that we discuss in the next section.
Here is a brief demonstration:
Similarly to list comprehensions , set comprehensions are also supported:
5.5. Dictionaries ¶
Another useful data type built into Python is the dictionary (see Mapping Types — dict ). Dictionaries are sometimes found in other languages as “associative memories” or “associative arrays”. Unlike sequences, which are indexed by a range of numbers, dictionaries are indexed by keys , which can be any immutable type; strings and numbers can always be keys. Tuples can be used as keys if they contain only strings, numbers, or tuples; if a tuple contains any mutable object either directly or indirectly, it cannot be used as a key. You can’t use lists as keys, since lists can be modified in place using index assignments, slice assignments, or methods like append() and extend() .
It is best to think of a dictionary as a set of key: value pairs, with the requirement that the keys are unique (within one dictionary). A pair of braces creates an empty dictionary: {} . Placing a comma-separated list of key:value pairs within the braces adds initial key:value pairs to the dictionary; this is also the way dictionaries are written on output.
The main operations on a dictionary are storing a value with some key and extracting the value given the key. It is also possible to delete a key:value pair with del . If you store using a key that is already in use, the old value associated with that key is forgotten. It is an error to extract a value using a non-existent key.
Performing list(d) on a dictionary returns a list of all the keys used in the dictionary, in insertion order (if you want it sorted, just use sorted(d) instead). To check whether a single key is in the dictionary, use the in keyword.
Here is a small example using a dictionary:
The dict() constructor builds dictionaries directly from sequences of key-value pairs:
In addition, dict comprehensions can be used to create dictionaries from arbitrary key and value expressions:
When the keys are simple strings, it is sometimes easier to specify pairs using keyword arguments:
5.6. Looping Techniques ¶
When looping through dictionaries, the key and corresponding value can be retrieved at the same time using the items() method.
When looping through a sequence, the position index and corresponding value can be retrieved at the same time using the enumerate() function.
To loop over two or more sequences at the same time, the entries can be paired with the zip() function.
To loop over a sequence in reverse, first specify the sequence in a forward direction and then call the reversed() function.
To loop over a sequence in sorted order, use the sorted() function which returns a new sorted list while leaving the source unaltered.
Using set() on a sequence eliminates duplicate elements. The use of sorted() in combination with set() over a sequence is an idiomatic way to loop over unique elements of the sequence in sorted order.
It is sometimes tempting to change a list while you are looping over it; however, it is often simpler and safer to create a new list instead.
5.7. More on Conditions ¶
The conditions used in while and if statements can contain any operators, not just comparisons.
The comparison operators in and not in are membership tests that determine whether a value is in (or not in) a container. The operators is and is not compare whether two objects are really the same object. All comparison operators have the same priority, which is lower than that of all numerical operators.
Comparisons can be chained. For example, a < b == c tests whether a is less than b and moreover b equals c .
Comparisons may be combined using the Boolean operators and and or , and the outcome of a comparison (or of any other Boolean expression) may be negated with not . These have lower priorities than comparison operators; between them, not has the highest priority and or the lowest, so that A and not B or C is equivalent to (A and (not B)) or C . As always, parentheses can be used to express the desired composition.
The Boolean operators and and or are so-called short-circuit operators: their arguments are evaluated from left to right, and evaluation stops as soon as the outcome is determined. For example, if A and C are true but B is false, A and B and C does not evaluate the expression C . When used as a general value and not as a Boolean, the return value of a short-circuit operator is the last evaluated argument.
It is possible to assign the result of a comparison or other Boolean expression to a variable. For example,
Note that in Python, unlike C, assignment inside expressions must be done explicitly with the walrus operator := . This avoids a common class of problems encountered in C programs: typing = in an expression when == was intended.
5.8. Comparing Sequences and Other Types ¶
Sequence objects typically may be compared to other objects with the same sequence type. The comparison uses lexicographical ordering: first the first two items are compared, and if they differ this determines the outcome of the comparison; if they are equal, the next two items are compared, and so on, until either sequence is exhausted. If two items to be compared are themselves sequences of the same type, the lexicographical comparison is carried out recursively. If all items of two sequences compare equal, the sequences are considered equal. If one sequence is an initial sub-sequence of the other, the shorter sequence is the smaller (lesser) one. Lexicographical ordering for strings uses the Unicode code point number to order individual characters. Some examples of comparisons between sequences of the same type:
Note that comparing objects of different types with < or > is legal provided that the objects have appropriate comparison methods. For example, mixed numeric types are compared according to their numeric value, so 0 equals 0.0, etc. Otherwise, rather than providing an arbitrary ordering, the interpreter will raise a TypeError exception.
Table of Contents
- 5.1.1. Using Lists as Stacks
- 5.1.2. Using Lists as Queues
- 5.1.3. List Comprehensions
- 5.1.4. Nested List Comprehensions
- 5.2. The del statement
- 5.3. Tuples and Sequences
- 5.5. Dictionaries
- 5.6. Looping Techniques
- 5.7. More on Conditions
- 5.8. Comparing Sequences and Other Types
Previous topic
4. More Control Flow Tools
- Report a Bug
- Show Source
Python Data Structures
Week 1 - assignment 6.5.
Write code using find() and string slicing to extract the number at the end of the line below. Convert the extracted value to a floating point number and print it out.
text = "X-DSPAM-Confidence: 0.8475";
text = "X-DSPAM-Confidence: 0.8475"
Colpos = text.find(':') # Colon Position
text_a_Colpos = text[Colpos+1 : ] # Text after colon position
number = text_a_Colpos.strip()
print(float(number))
ans = float(text_a_Colpos)
# Using Split and join functions
num_str = text_a_Colpos.split() # string format of number in list
d = ""
num = d.join(num_str) # converts list into string
num_f = float(num)
print(num_f)
=============================================================================================
Week 3 - Assignment 7.1
Write a program that prompts for a file name, then opens that file and reads through the file, and print the contents of the file in upper case. Use the file words.txt to produce the output below.
when you are testing below enter words.txt as the file name.
file = input('Enter the file name: ')
fhandle = open(file)
for line in fhandle:
line_strip = line.strip()
line = line_strip.upper()
print(line)
Assignment 7.2
Write a program that prompts for a file name, then opens that file and reads through the file, looking for lines of the form:
X-DSPAM-Confidence: 0.8475
Count these lines and extract the floating point values from each of the lines and compute the average of those values and produce an output as shown below. Do not use the sum() function or a variable named sum in your solution.
when you are testing below enter mbox-short.txt as the file name.
fname = input('Enter the file name: ')
fhandle = open(fname)
for line in fhandle :
if 'X-DSPAM-Confidence:' in line :
Colpos = line.find(':')
num_string = line[Colpos + 1 : ]
num = float(num_string)
count = count + 1
Total = Total + num
avg = Total / count
print('Average spam confidence:',avg)
===============================================================================================
Week 4 - Assignment 8.4
Open the file romeo.txt and read it line by line. For each line, split the line into a list of words using the split() method. The program should build a list of words. For each word on each line check to see if the word is already in the list and if not append it to the list. When the program completes, sort and print the resulting words in alphabetical order.
fhandle = open('romeo.txt')
lst = list()
words = line.split()
print(words)
for word in words:
if lst is None:
lst.append(word)
elif word in lst:
Assignment 8.5
Open the file mbox-short.txt and read it line by line. When you find a line that starts with 'From ' like the following line:
From [email protected] Sat Jan 5 09:14:16 2008
You will parse the From line using split() and print out the second word in the line (i.e. the entire address of the person who sent the message). Then print out a count at the end.
Hint: make sure not to include the lines that start with 'From:'.
if line.startswith('From') :
if line[4] is ':' :
req_line = line.split()
print(req_line[1])
print('There were',count, 'lines in the file with From as the first word')
==============================================================================================
Week 5 - Assignment 9.4
Write a program to read through the mbox-short.txt and figure out who has sent the greatest number of mail messages. The program looks for 'From ' lines and takes the second word of those lines as the person who sent the mail. The program creates a Python dictionary that maps the sender's mail address to a count of the number of times they appear in the file. After the dictionary is produced, the program reads through the dictionary using a maximum loop to find the most prolific committer.
reg_mailer = dict() # regular mailer
mail = words[1]
# reg_mailer[mail] = reg_mailer.get(mail,0) + 1
if reg_mailer is None or mail not in reg_mailer :
reg_mailer[mail] = 1
reg_mailer[mail] = reg_mailer[mail] + 1
a = max(reg_mailer.values())
for key, value in reg_mailer.items() :
if value == a :
print(key,a)
Week 6 - Assignment 10.2
Write a program to read through the mbox-short.txt and figure out the distribution by hour of the day for each of the messages.
You can pull the hour out from the 'From ' line by finding the time and then splitting the string a second time using a colon.
Once you have accumulated the counts for each hour, print out the counts, sorted by hour as shown below.
time_mail = dict()
time = words[5]
time_tup = time.split(':')
time_tuple = time_tup[0]
time_mail[time_tuple] = time_mail.get(time_tuple,0) + 1
# if reg_mailer is None or mail not in reg_mailer :
# reg_mailer[mail] = 1
# reg_mailer[mail] = reg_mailer[mail] + 1
ans = sorted(time_mail.items())
for k,v in ans:
Python Programming
Python Data Structure Exercise for Beginners
Updated on: December 8, 2021 | 116 Comments
This data structure exercise is for beginners to understand and practice basic data structure in Python. Practice Python List , Set , Dictionary , and Tuple questions.
The data structure is widely used to hold any data. To perform any programming tasks in Python, good knowledge of data structure is a must.
Solve this exercise to have a good understanding of basic data structure in Python
Also, Solve :
- Python List exercise
- Python Dictionary exercise
- Python Tuple exercise
- Python Set exercise
This Exercise includes the followings
- The exercise contains 10 questions and solution provided for each question
- Questions cover list manipulation, dictionary, set, and tuple methods.
Use Online Code Editor to solve exercise questions .
Exercise 1: Create a list by picking an odd-index items from the first list and even index items from the second
Given two lists, l1 and l2, write a program to create a third list l3 by picking an odd-index element from the list l1 and even index elements from the list l2.
Expected Output :
Use list slicing
To access a range of items in a list, use the slicing operator : . With this operator, you can specify where to start the slicing, end, and specify the step.
For example, the expression list1[ start : stop : step] returns the portion of the list from index start to index stop, at a step size step.
- for 1st list: Start from the 1st index with step value 2 so it will pick elements present at index 1, 3, 5, and so on
- for 2nd list: Start from the 0th index with step value 2 so it will pick elements present at index 0, 2, 4, and so on
Exercise 2: Remove and add item in a list
Write a program to remove the item present at index 4 and add it to the 2nd position and at the end of the list.
Use the list methods, pop() , insert() and append()
- pop(index) : Removes and returns the item at the given index from the list.
- insert(index, item) : Add the item at the specified position(index) in the list
- append(item) : Add item at the end of the list.
Exercise 3: Slice list into 3 equal chunks and reverse each chunk
Expected Outcome :
- Divide the length of a list by 3 to get the each chunk size
- Run loop three times and use the slice() function to get the chunk and reverse it
- Get the length of a list using a len() function
- Divide the length by 3 to get the chunk size
- Run loop three times
- In each iteration, get a chunk using a slice(start, end, step) function and reverse it using the reversed() function
- In each iteration, start and end value will change

Exercise 4: Count the occurrence of each element from a list
Write a program to iterate a given list and count the occurrence of each element and create a dictionary to show the count of each element.
Exercise 5: Create a Python set such that it shows the element from both lists in a pair
Use the zip() function. This function takes two or more iterables (like list, dict, string), aggregates them in a tuple , and returns it.
Exercise 6: Find the intersection (common) of two sets and remove those elements from the first set
See : Python Set
- Use the intersection() and remove() method of a set
- Get the common items using the first_set.intersection(second_set)
- Next, iterate common items using a for loop
- In each iteration, use the remove() method of on first set and pass the current item to it.
Exercise 7: Checks if one set is a subset or superset of another set. If found, delete all elements from that set
Use the below methods of a set class
- issuperset()
Exercise 8: Iterate a given list and check if a given element exists as a key’s value in a dictionary. If not, delete it from the list
Exercise 9: get all values from the dictionary and add them to a list but don’t add duplicates, exercise 10: remove duplicates from a list and create a tuple and find the minimum and maximum number.
Did you find this page helpful? Let others know about it. Sharing helps me continue to create free Python resources.
About Vishal

I’m Vishal Hule , the Founder of PYnative.com. As a Python developer, I enjoy assisting students, developers, and learners. Follow me on Twitter .
Related Tutorial Topics:
Python exercises and quizzes.
Free coding exercises and quizzes cover Python basics, data structure, data analytics, and more.
- 15+ Topic-specific Exercises and Quizzes
- Each Exercise contains 10 questions
- Each Quiz contains 12-15 MCQ
Loading comments... Please wait.
About PYnative
PYnative.com is for Python lovers. Here, You can get Tutorials, Exercises, and Quizzes to practice and improve your Python skills .
Explore Python
- Learn Python
- Python Basics
- Python Databases
- Python Exercises
- Python Quizzes
- Online Python Code Editor
- Python Tricks
To get New Python Tutorials, Exercises, and Quizzes
Legal Stuff
We use cookies to improve your experience. While using PYnative, you agree to have read and accepted our Terms Of Use , Cookie Policy , and Privacy Policy .
Copyright © 2018–2024 pynative.com
- Python Course
- Python Basics
- Interview Questions
- Python Quiz
- Popular Packages
- Python Projects
- Practice Python
- AI With Python
- Learn Python3
- Python Automation
- Python Web Dev
- DSA with Python
- Python OOPs
- Dictionaries
Learn DSA with Python | Python Data Structures and Algorithms
This tutorial is a beginner-friendly guide for learning data structures and algorithms using Python. In this article, we will discuss the in-built data structures such as lists, tuples, dictionaries, etc, and some user-defined data structures such as linked lists , trees , graphs , etc, and traversal as well as searching and sorting algorithms with the help of good and well-explained examples and practice questions.
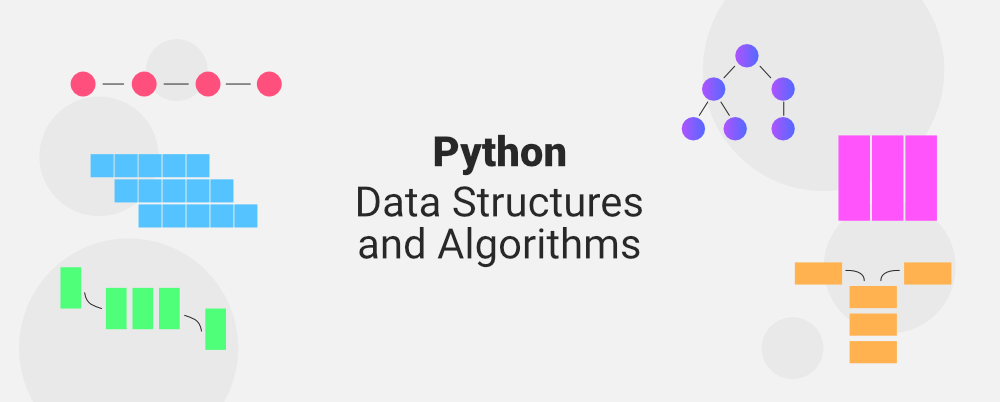
Python Lists are ordered collections of data just like arrays in other programming languages. It allows different types of elements in the list. The implementation of Python List is similar to Vectors in C++ or ArrayList in JAVA. The costly operation is inserting or deleting the element from the beginning of the List as all the elements are needed to be shifted. Insertion and deletion at the end of the list can also become costly in the case where the preallocated memory becomes full.
Example: Creating Python List
List elements can be accessed by the assigned index. In python starting index of the list, a sequence is 0 and the ending index is (if N elements are there) N-1.
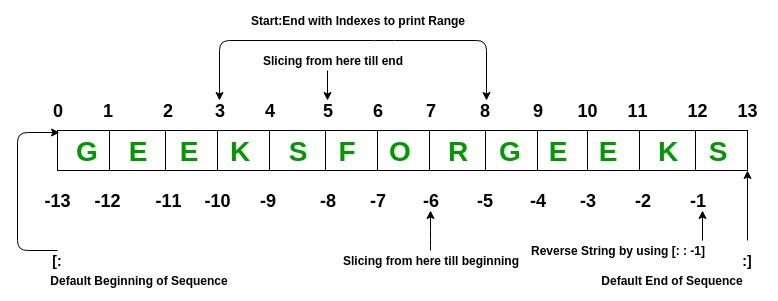
Example: Python List Operations
Python tuples are similar to lists but Tuples are immutable in nature i.e. once created it cannot be modified. Just like a List, a Tuple can also contain elements of various types.
In Python, tuples are created by placing a sequence of values separated by ‘comma’ with or without the use of parentheses for grouping of the data sequence.
Note: To create a tuple of one element there must be a trailing comma. For example, (8,) will create a tuple containing 8 as the element.
Example: Python Tuple Operations
Python set is a mutable collection of data that does not allow any duplication. Sets are basically used to include membership testing and eliminating duplicate entries. The data structure used in this is Hashing, a popular technique to perform insertion, deletion, and traversal in O(1) on average.
If Multiple values are present at the same index position, then the value is appended to that index position, to form a Linked List. In, CPython Sets are implemented using a dictionary with dummy variables, where key beings the members set with greater optimizations to the time complexity.
Set Implementation:
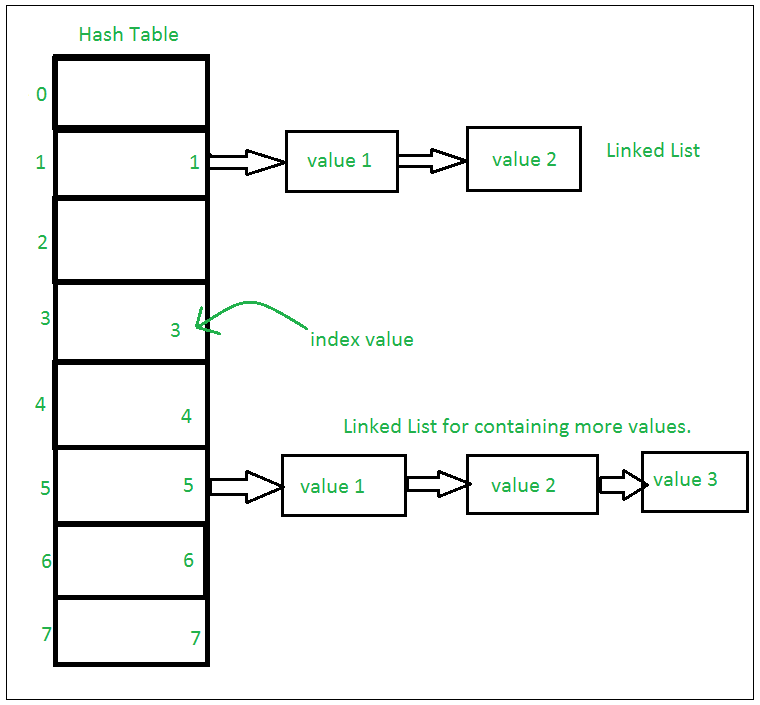
Sets with Numerous operations on a single HashTable:
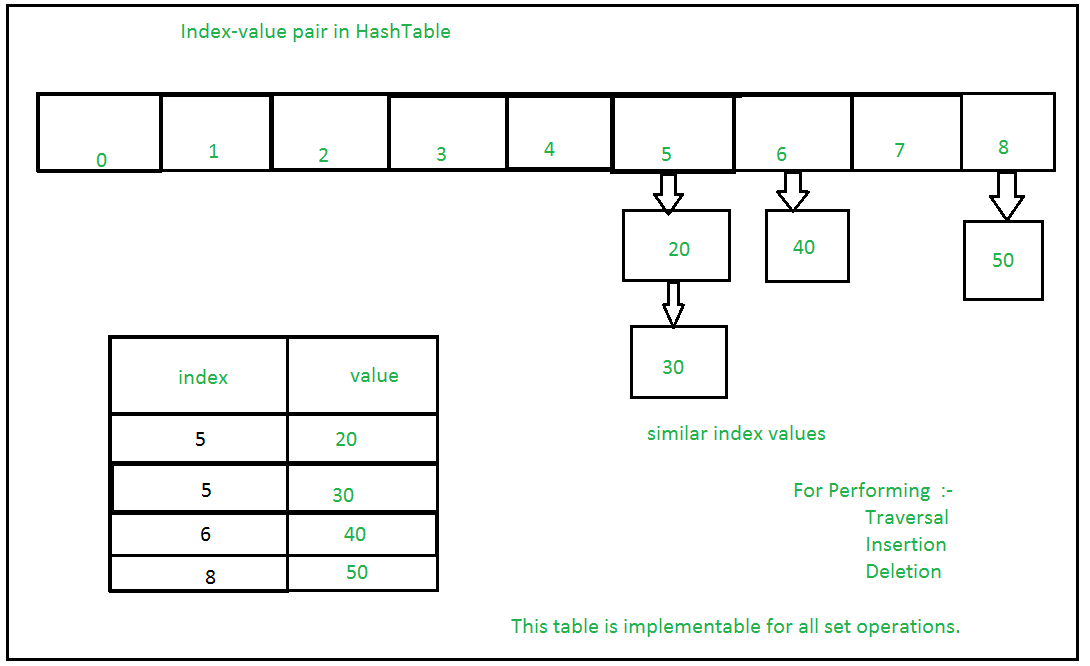
Example: Python Set Operations
Frozen sets.
Frozen sets in Python are immutable objects that only support methods and operators that produce a result without affecting the frozen set or sets to which they are applied. While elements of a set can be modified at any time, elements of the frozen set remain the same after creation.
Example: Python Frozen set
Python Strings is the immutable array of bytes representing Unicode characters. Python does not have a character data type, a single character is simply a string with a length of 1.
Note: As strings are immutable, modifying a string will result in creating a new copy.
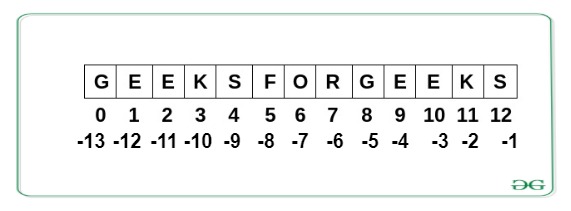
Example: Python Strings Operations
Python dictionary is an unordered collection of data that stores data in the format of key:value pair. It is like hash tables in any other language with the time complexity of O(1). Indexing of Python Dictionary is done with the help of keys. These are of any hashable type i.e. an object whose can never change like strings, numbers, tuples, etc. We can create a dictionary by using curly braces ({}) or dictionary comprehension.
Example: Python Dictionary Operations
A matrix is a 2D array where each element is of strictly the same size. To create a matrix we will be using the NumPy package .
Example: Python NumPy Matrix Operations
Python Bytearray gives a mutable sequence of integers in the range 0 <= x < 256.
Example: Python Bytearray Operations
Linked list.
A linked list is a linear data structure, in which the elements are not stored at contiguous memory locations. The elements in a linked list are linked using pointers as shown in the below image:
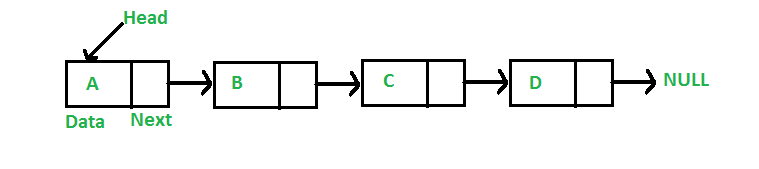
A linked list is represented by a pointer to the first node of the linked list. The first node is called the head. If the linked list is empty, then the value of the head is NULL. Each node in a list consists of at least two parts:
- Pointer (Or Reference) to the next node
Example: Defining Linked List in Python
Let us create a simple linked list with 3 nodes.
Linked List Traversal
In the previous program, we have created a simple linked list with three nodes. Let us traverse the created list and print the data of each node. For traversal, let us write a general-purpose function printList() that prints any given list.
More articles on Linked List
- Linked List Insertion
- Linked List Deletion (Deleting a given key)
- Linked List Deletion (Deleting a key at given position)
- Find Length of a Linked List (Iterative and Recursive)
- Search an element in a Linked List (Iterative and Recursive)
- Nth node from the end of a Linked List
- Reverse a linked list
>>> More
A stack is a linear data structure that stores items in a Last-In/First-Out (LIFO) or First-In/Last-Out (FILO) manner. In stack, a new element is added at one end and an element is removed from that end only. The insert and delete operations are often called push and pop.
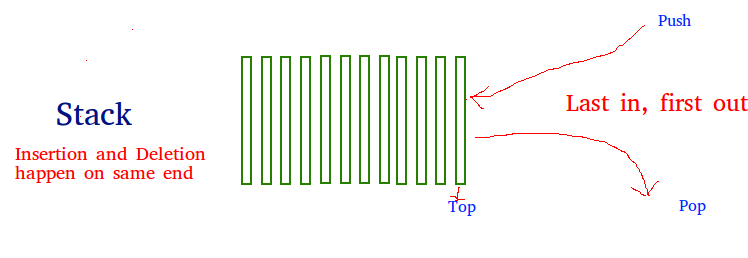
The functions associated with stack are:
- empty() – Returns whether the stack is empty – Time Complexity: O(1)
- size() – Returns the size of the stack – Time Complexity: O(1)
- top() – Returns a reference to the topmost element of the stack – Time Complexity: O(1)
- push(a) – Inserts the element ‘a’ at the top of the stack – Time Complexity: O(1)
- pop() – Deletes the topmost element of the stack – Time Complexity: O(1)
More articles on Stack
- Infix to Postfix Conversion using Stack
- Prefix to Infix Conversion
- Prefix to Postfix Conversion
- Postfix to Prefix Conversion
- Postfix to Infix
- Check for balanced parentheses in an expression
- Evaluation of Postfix Expression
As a stack, the queue is a linear data structure that stores items in a First In First Out (FIFO) manner. With a queue, the least recently added item is removed first. A good example of the queue is any queue of consumers for a resource where the consumer that came first is served first.
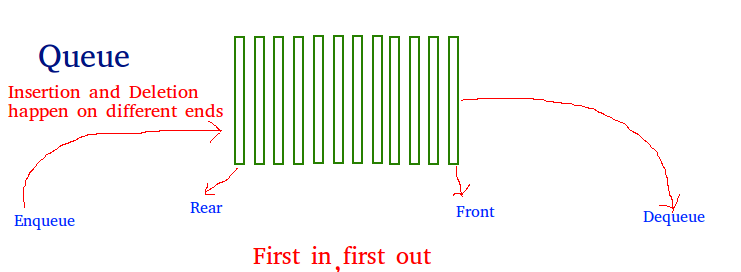
Operations associated with queue are:
- Enqueue: Adds an item to the queue. If the queue is full, then it is said to be an Overflow condition – Time Complexity: O(1)
- Dequeue: Removes an item from the queue. The items are popped in the same order in which they are pushed. If the queue is empty, then it is said to be an Underflow condition – Time Complexity: O(1)
- Front: Get the front item from queue – Time Complexity: O(1)
- Rear: Get the last item from queue – Time Complexity: O(1)
More articles on Queue
- Implement Queue using Stacks
- Implement Stack using Queues
- Implement a stack using single queue
Priority Queue
Priority Queues are abstract data structures where each data/value in the queue has a certain priority. For example, In airlines, baggage with the title “Business” or “First-class” arrives earlier than the rest. Priority Queue is an extension of the queue with the following properties.
- An element with high priority is dequeued before an element with low priority.
- If two elements have the same priority, they are served according to their order in the queue.
heapq module in Python provides the heap data structure that is mainly used to represent a priority queue. The property of this data structure is that it always gives the smallest element (min heap) whenever the element is popped. Whenever elements are pushed or popped, heap structure is maintained. The heap[0] element also returns the smallest element each time. It supports the extraction and insertion of the smallest element in the O(log n) times.
Generally, Heaps can be of two types:
- Max-Heap: In a Max-Heap the key present at the root node must be greatest among the keys present at all of it’s children. The same property must be recursively true for all sub-trees in that Binary Tree.
- Min-Heap: In a Min-Heap the key present at the root node must be minimum among the keys present at all of it’s children. The same property must be recursively true for all sub-trees in that Binary Tree.
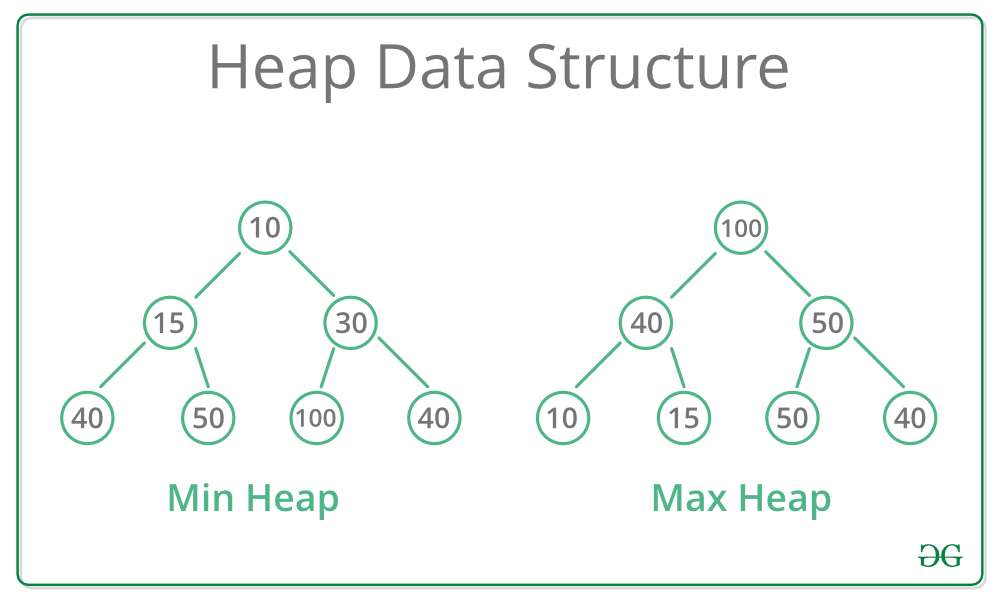
More Articles on Heap
- Binary Heap
- K’th Largest Element in an array
- K’th Smallest/Largest Element in Unsorted Array
- Sort an almost sorted array
- K-th Largest Sum Contiguous Subarray
- Minimum sum of two numbers formed from digits of an array
Binary Tree
A tree is a hierarchical data structure that looks like the below figure –
The topmost node of the tree is called the root whereas the bottommost nodes or the nodes with no children are called the leaf nodes. The nodes that are directly under a node are called its children and the nodes that are directly above something are called its parent.
A binary tree is a tree whose elements can have almost two children. Since each element in a binary tree can have only 2 children, we typically name them the left and right children. A Binary Tree node contains the following parts.
- Pointer to left child
- Pointer to the right child
Example: Defining Node Class
Now let’s create a tree with 4 nodes in Python. Let’s assume the tree structure looks like below –
Example: Adding data to the tree
Tree traversal.
Trees can be traversed in different ways. Following are the generally used ways for traversing trees. Let us consider the below tree –
Depth First Traversals:
- Inorder (Left, Root, Right) : 4 2 5 1 3
- Preorder (Root, Left, Right) : 1 2 4 5 3
- Postorder (Left, Right, Root) : 4 5 2 3 1
Algorithm Inorder(tree)
- Traverse the left subtree, i.e., call Inorder(left-subtree)
- Visit the root.
- Traverse the right subtree, i.e., call Inorder(right-subtree)
Algorithm Preorder(tree)
- Traverse the left subtree, i.e., call Preorder(left-subtree)
- Traverse the right subtree, i.e., call Preorder(right-subtree)
Algorithm Postorder(tree)
- Traverse the left subtree, i.e., call Postorder(left-subtree)
- Traverse the right subtree, i.e., call Postorder(right-subtree)
Time Complexity – O(n)
Breadth-First or Level Order Traversal
Level order traversal of a tree is breadth-first traversal for the tree. The level order traversal of the above tree is 1 2 3 4 5.
For each node, first, the node is visited and then its child nodes are put in a FIFO queue. Below is the algorithm for the same –
- Create an empty queue q
- temp_node = root /*start from root*/
- print temp_node->data.
- Enqueue temp_node’s children (first left then right children) to q
- Dequeue a node from q
Time Complexity: O(n)
More articles on Binary Tree
- Insertion in a Binary Tree
- Deletion in a Binary Tree
- Inorder Tree Traversal without Recursion
- Inorder Tree Traversal without recursion and without stack!
- Print Postorder traversal from given Inorder and Preorder traversals
- Find postorder traversal of BST from preorder traversal
Binary Search Tree
Binary Search Tree is a node-based binary tree data structure that has the following properties:
- The left subtree of a node contains only nodes with keys lesser than the node’s key.
- The right subtree of a node contains only nodes with keys greater than the node’s key.
- The left and right subtree each must also be a binary search tree.

The above properties of the Binary Search Tree provide an ordering among keys so that the operations like search, minimum and maximum can be done fast. If there is no order, then we may have to compare every key to search for a given key.
Searching Element
- Start from the root.
- Compare the searching element with root, if less than root, then recurse for left, else recurse for right.
- If the element to search is found anywhere, return true, else return false.
Insertion of a key
- Compare the inserting element with root, if less than root, then recurse for left, else recurse for right.
- After reaching the end, just insert that node at left(if less than current) else right.
More Articles on Binary Search Tree
- Binary Search Tree – Delete Key
- Construct BST from given preorder traversal | Set 1
- Binary Tree to Binary Search Tree Conversion
- Find the node with minimum value in a Binary Search Tree
- A program to check if a binary tree is BST or not
A graph is a nonlinear data structure consisting of nodes and edges. The nodes are sometimes also referred to as vertices and the edges are lines or arcs that connect any two nodes in the graph. More formally a Graph can be defined as a Graph consisting of a finite set of vertices(or nodes) and a set of edges that connect a pair of nodes.
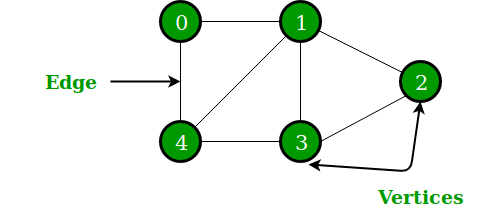
In the above Graph, the set of vertices V = {0,1,2,3,4} and the set of edges E = {01, 12, 23, 34, 04, 14, 13}. The following two are the most commonly used representations of a graph.
Adjacency Matrix
Adjacency list.
Adjacency Matrix is a 2D array of size V x V where V is the number of vertices in a graph. Let the 2D array be adj[][], a slot adj[i][j] = 1 indicates that there is an edge from vertex i to vertex j. The adjacency matrix for an undirected graph is always symmetric. Adjacency Matrix is also used to represent weighted graphs. If adj[i][j] = w, then there is an edge from vertex i to vertex j with weight w.
Vertices of Graph [‘a’, ‘b’, ‘c’, ‘d’, ‘e’, ‘f’] Edges of Graph [(‘a’, ‘c’, 20), (‘a’, ‘e’, 10), (‘b’, ‘c’, 30), (‘b’, ‘e’, 40), (‘c’, ‘a’, 20), (‘c’, ‘b’, 30), (‘d’, ‘e’, 50), (‘e’, ‘a’, 10), (‘e’, ‘b’, 40), (‘e’, ‘d’, 50), (‘e’, ‘f’, 60), (‘f’, ‘e’, 60)] Adjacency Matrix of Graph [[-1, -1, 20, -1, 10, -1], [-1, -1, 30, -1, 40, -1], [20, 30, -1, -1, -1, -1], [-1, -1, -1, -1, 50, -1], [10, 40, -1, 50, -1, 60], [-1, -1, -1, -1, 60, -1]]
An array of lists is used. The size of the array is equal to the number of vertices. Let the array be an array[]. An entry array[i] represents the list of vertices adjacent to the ith vertex. This representation can also be used to represent a weighted graph. The weights of edges can be represented as lists of pairs. Following is the adjacency list representation of the above graph.

Graph Traversal
Breadth-first search or bfs.
Breadth-First Traversal for a graph is similar to Breadth-First Traversal of a tree. The only catch here is, unlike trees, graphs may contain cycles, so we may come to the same node again. To avoid processing a node more than once, we use a boolean visited array. For simplicity, it is assumed that all vertices are reachable from the starting vertex.
For example, in the following graph, we start traversal from vertex 2. When we come to vertex 0, we look for all adjacent vertices of it. 2 is also an adjacent vertex of 0. If we don’t mark visited vertices, then 2 will be processed again and it will become a non-terminating process. A Breadth-First Traversal of the following graph is 2, 0, 3, 1.
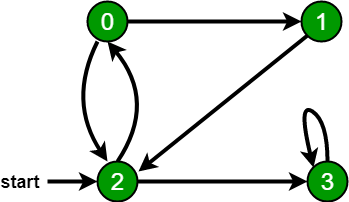
Time Complexity: O(V+E) where V is the number of vertices in the graph and E is the number of edges in the graph.
Depth First Search or DFS
Depth First Traversal for a graph is similar to Depth First Traversal of a tree. The only catch here is, unlike trees, graphs may contain cycles, a node may be visited twice. To avoid processing a node more than once, use a boolean visited array.
- Create a recursive function that takes the index of the node and a visited array.
- Mark the current node as visited and print the node.
- Traverse all the adjacent and unmarked nodes and call the recursive function with the index of the adjacent node.
More articles on graph
- Graph representations using set and hash
- Find Mother Vertex in a Graph
- Iterative Depth First Search
- Count the number of nodes at given level in a tree using BFS
- Count all possible paths between two vertices
The process in which a function calls itself directly or indirectly is called recursion and the corresponding function is called a recursive function . Using the recursive algorithms, certain problems can be solved quite easily. Examples of such problems are Towers of Hanoi (TOH), Inorder/Preorder/Postorder Tree Traversals, DFS of Graph, etc.
What is the base condition in recursion?
In the recursive program, the solution to the base case is provided and the solution of the bigger problem is expressed in terms of smaller problems.
In the above example, base case for n < = 1 is defined and larger value of number can be solved by converting to smaller one till base case is reached.
How memory is allocated to different function calls in recursion?
When any function is called from main(), the memory is allocated to it on the stack. A recursive function calls itself, the memory for a called function is allocated on top of memory allocated to the calling function and a different copy of local variables is created for each function call. When the base case is reached, the function returns its value to the function by whom it is called and memory is de-allocated and the process continues.
Let us take the example of how recursion works by taking a simple function.
The memory stack has been shown in below diagram.
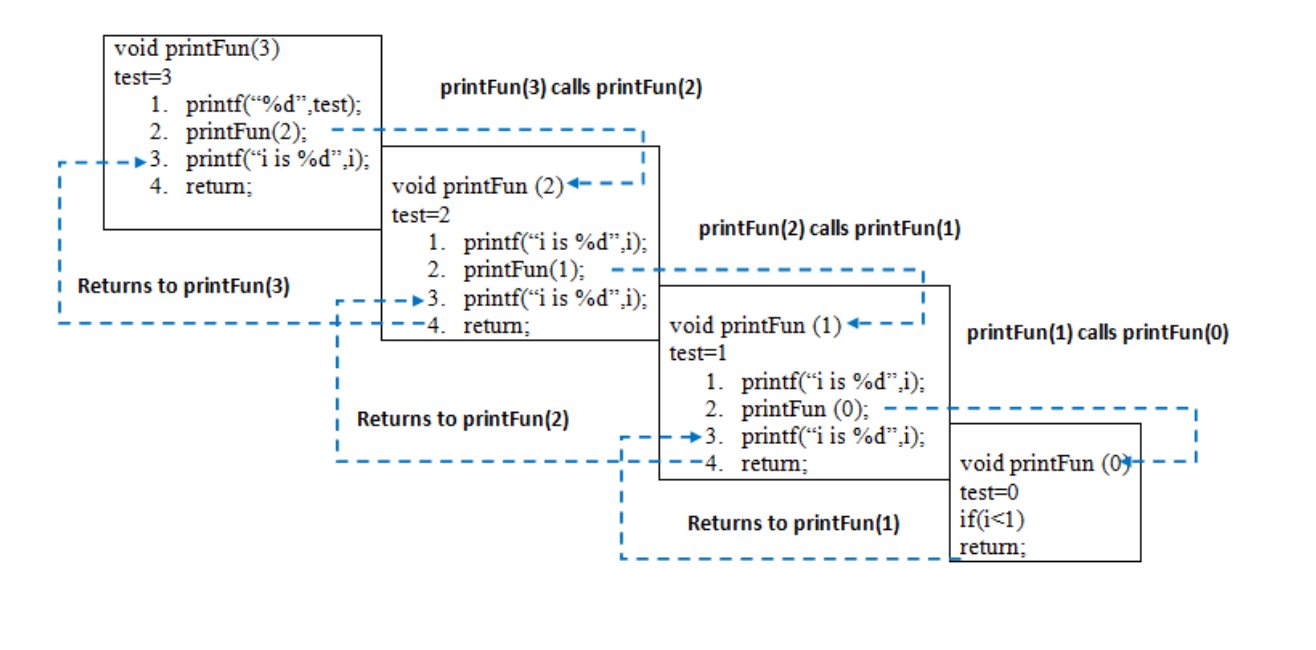
More articles on Recursion
- Recursion in Python
- Practice Questions for Recursion | Set 1
- Practice Questions for Recursion | Set 2
- Practice Questions for Recursion | Set 3
- Practice Questions for Recursion | Set 4
- Practice Questions for Recursion | Set 5
- Practice Questions for Recursion | Set 6
- Practice Questions for Recursion | Set 7
Dynamic Programming
Dynamic Programming is mainly an optimization over plain recursion. Wherever we see a recursive solution that has repeated calls for same inputs, we can optimize it using Dynamic Programming. The idea is to simply store the results of subproblems, so that we do not have to re-compute them when needed later. This simple optimization reduces time complexities from exponential to polynomial. For example, if we write simple recursive solution for Fibonacci Numbers, we get exponential time complexity and if we optimize it by storing solutions of subproblems, time complexity reduces to linear.
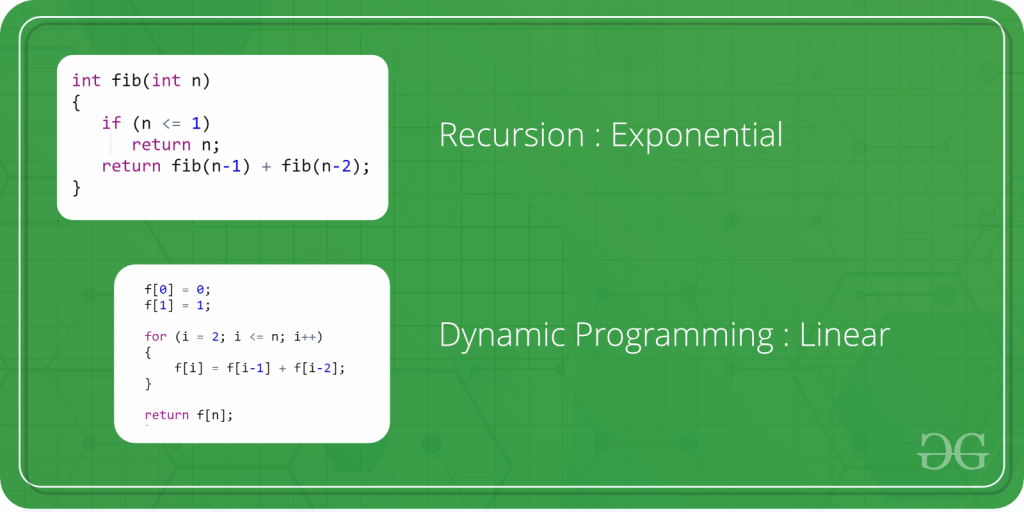
Tabulation vs Memoization
There are two different ways to store the values so that the values of a sub-problem can be reused. Here, will discuss two patterns of solving dynamic programming (DP) problem:
- Tabulation: Bottom Up
- Memoization: Top Down
As the name itself suggests starting from the bottom and accumulating answers to the top. Let’s discuss in terms of state transition.
Let’s describe a state for our DP problem to be dp[x] with dp[0] as base state and dp[n] as our destination state. So, we need to find the value of destination state i.e dp[n].
If we start our transition from our base state i.e dp[0] and follow our state transition relation to reach our destination state dp[n], we call it the Bottom-Up approach as it is quite clear that we started our transition from the bottom base state and reached the topmost desired state.
Now, Why do we call it tabulation method?
To know this let’s first write some code to calculate the factorial of a number using bottom up approach. Once, again as our general procedure to solve a DP we first define a state. In this case, we define a state as dp[x], where dp[x] is to find the factorial of x.
Now, it is quite obvious that dp[x+1] = dp[x] * (x+1)
Memoization
Once, again let’s describe it in terms of state transition. If we need to find the value for some state say dp[n] and instead of starting from the base state that i.e dp[0] we ask our answer from the states that can reach the destination state dp[n] following the state transition relation, then it is the top-down fashion of DP.
Here, we start our journey from the top most destination state and compute its answer by taking in count the values of states that can reach the destination state, till we reach the bottom-most base state.
Once again, let’s write the code for the factorial problem in the top-down fashion
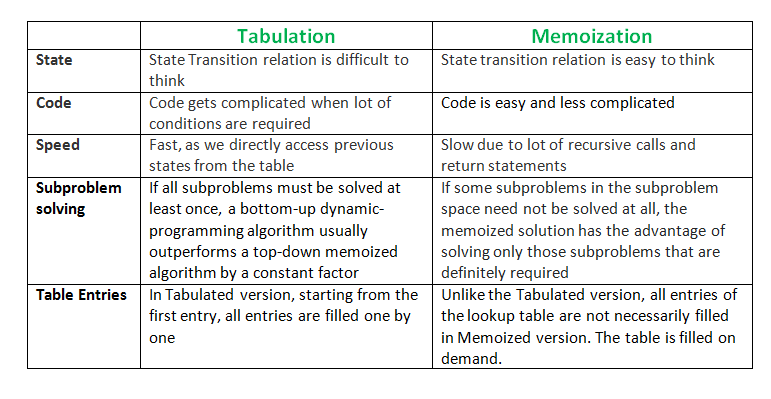
More articles on Dynamic Programming
- Optimal Substructure Property
- Overlapping Subproblems Property
- Fibonacci numbers
- Subset with sum divisible by m
- Maximum Sum Increasing Subsequence
- Longest Common Substring
Searching Algorithms
Linear search.
- Start from the leftmost element of arr[] and one by one compare x with each element of arr[]
- If x matches with an element, return the index.
- If x doesn’t match with any of the elements, return -1.
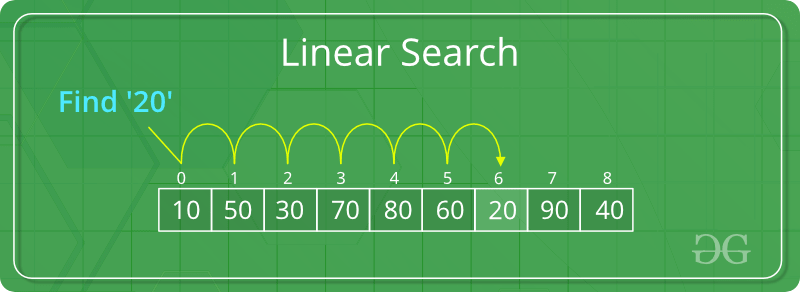
The time complexity of the above algorithm is O(n).
For more information, refer to Linear Search .
Binary Search
Search a sorted array by repeatedly dividing the search interval in half. Begin with an interval covering the whole array. If the value of the search key is less than the item in the middle of the interval, narrow the interval to the lower half. Otherwise, narrow it to the upper half. Repeatedly check until the value is found or the interval is empty.

The time complexity of the above algorithm is O(log(n)).
For more information, refer to Binary Search .
Sorting Algorithms
Selection sort.
The selection sort algorithm sorts an array by repeatedly finding the minimum element (considering ascending order) from unsorted part and putting it at the beginning. In every iteration of selection sort, the minimum element (considering ascending order) from the unsorted subarray is picked and moved to the sorted subarray.
Flowchart of the Selection Sort:
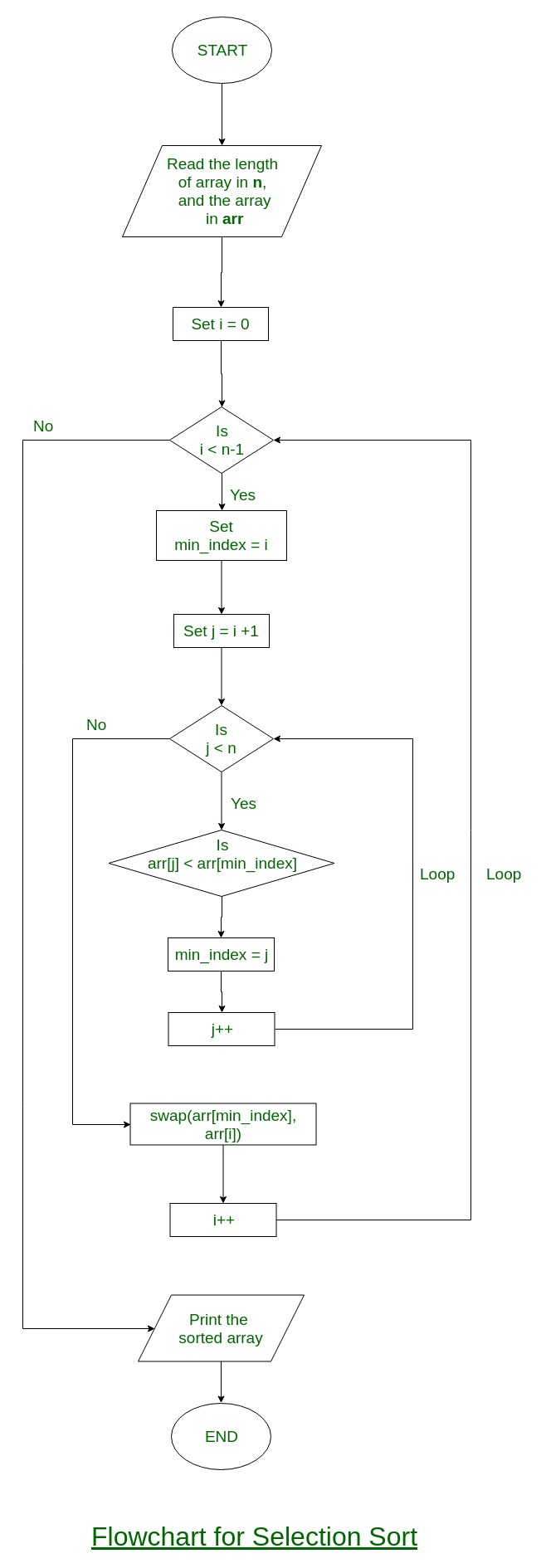
Time Complexity: O(n 2 ) as there are two nested loops.
Auxiliary Space: O(1)
Bubble Sort
Bubble Sort is the simplest sorting algorithm that works by repeatedly swapping the adjacent elements if they are in wrong order.
Illustration :
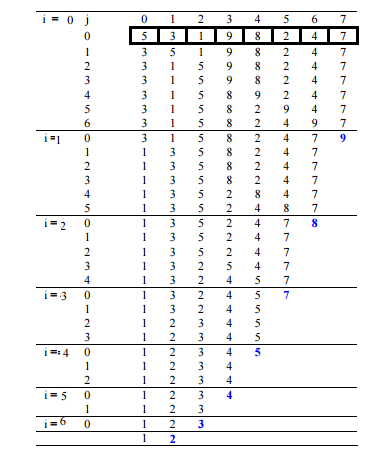
Time Complexity: O(n 2 )
Insertion Sort
To sort an array of size n in ascending order using insertion sort :
- Iterate from arr[1] to arr[n] over the array.
- Compare the current element (key) to its predecessor.
- If the key element is smaller than its predecessor, compare it to the elements before. Move the greater elements one position up to make space for the swapped element.
Illustration:
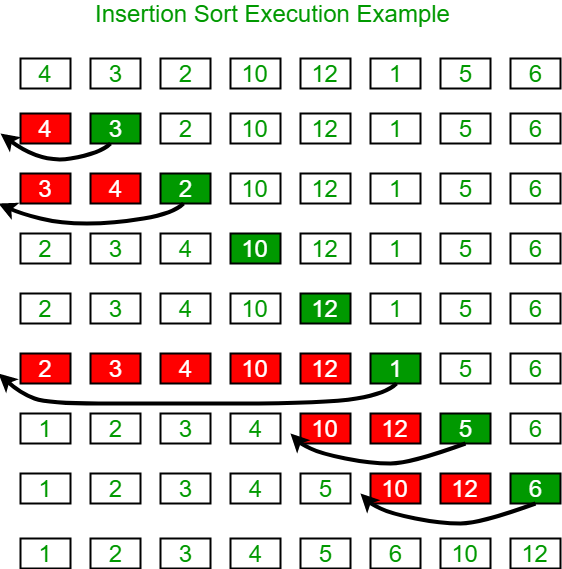
Time Complexity: O(n 2 ))
Like QuickSort, Merge Sort is a Divide and Conquer algorithm. It divides the input array into two halves, calls itself for the two halves, and then merges the two sorted halves. The merge() function is used for merging two halves. The merge(arr, l, m, r) is a key process that assumes that arr[l..m] and arr[m+1..r] are sorted and merges the two sorted sub-arrays into one.
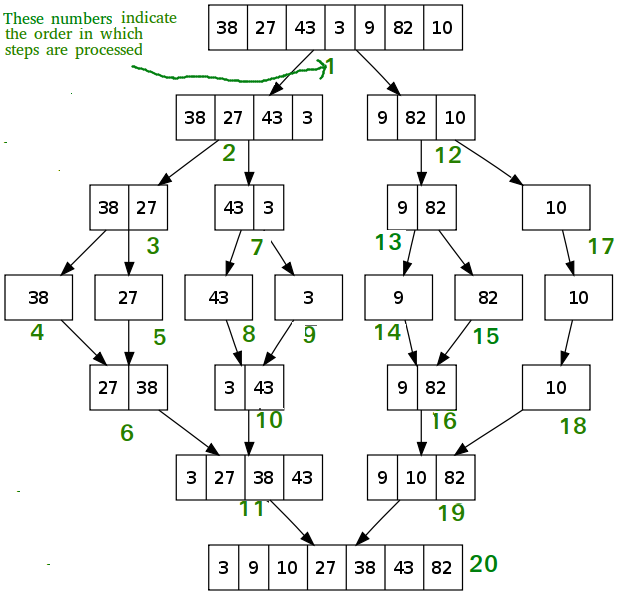
Time Complexity: O(n(logn))
Like Merge Sort, QuickSort is a Divide and Conquer algorithm. It picks an element as pivot and partitions the given array around the picked pivot. There are many different versions of quickSort that pick pivot in different ways.
Always pick first element as pivot.
- Always pick last element as pivot (implemented below)
- Pick a random element as pivot.
- Pick median as pivot.
The key process in quickSort is partition(). Target of partitions is, given an array and an element x of array as pivot, put x at its correct position in sorted array and put all smaller elements (smaller than x) before x, and put all greater elements (greater than x) after x. All this should be done in linear time.
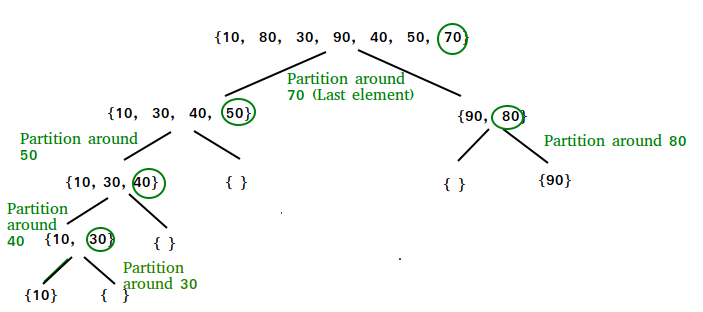
Partition Algorithm
There can be many ways to do partition, following pseudo code adopts the method given in CLRS book. The logic is simple, we start from the leftmost element and keep track of index of smaller (or equal to) elements as i. While traversing, if we find a smaller element, we swap current element with arr[i]. Otherwise we ignore current element.
ShellSort is mainly a variation of Insertion Sort. In insertion sort, we move elements only one position ahead. When an element has to be moved far ahead, many movements are involved. The idea of shellSort is to allow the exchange of far items. In shellSort, we make the array h-sorted for a large value of h. We keep reducing the value of h until it becomes 1. An array is said to be h-sorted if all sublists of every h th element is sorted.
Time Complexity: O(n 2 ).
Please Login to comment...
Similar reads, improve your coding skills with practice.
What kind of Experience do you want to share?
Learn Algorithms and Data Structures in Python
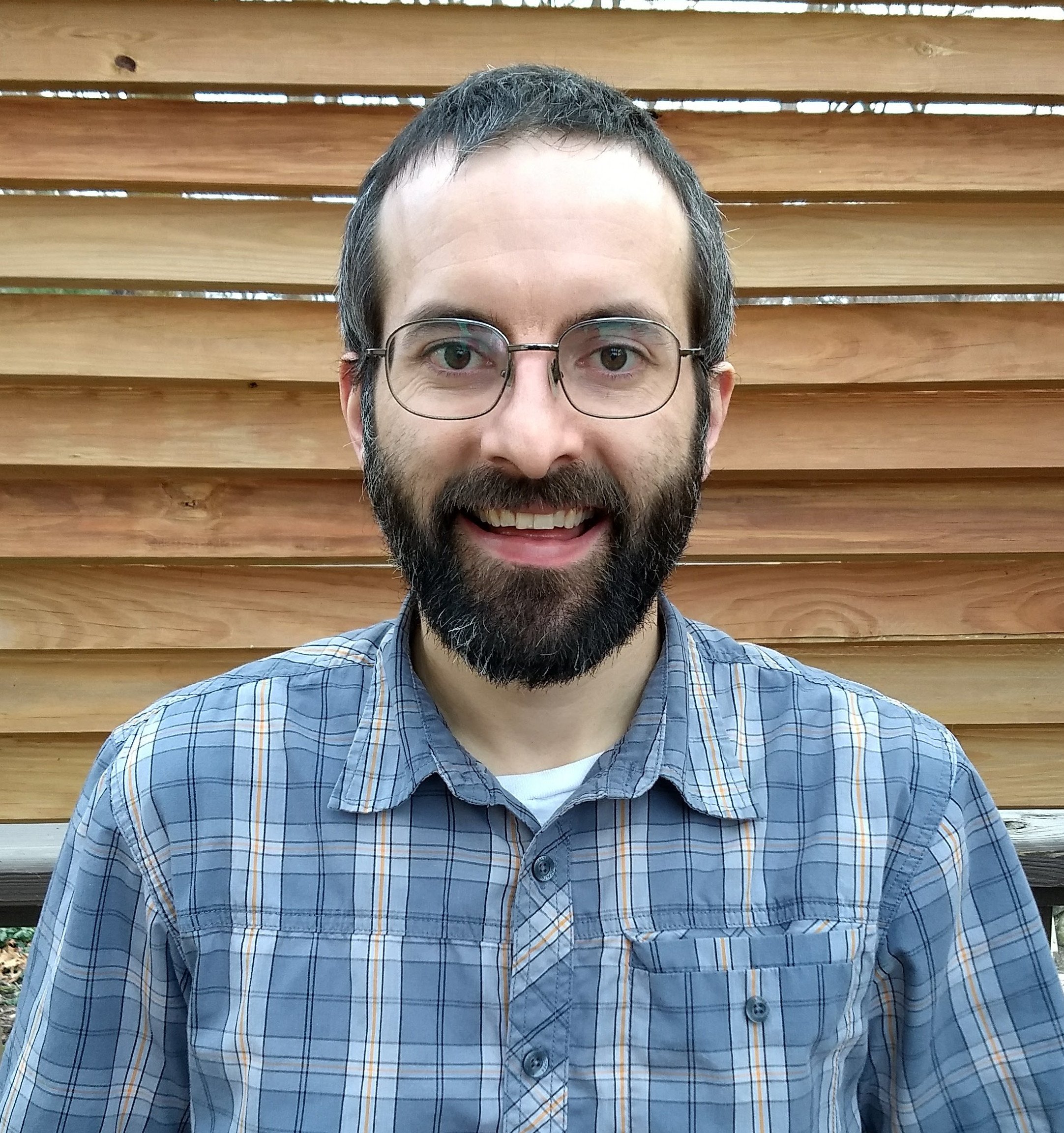
Algorithms and data structures are important for most programmers to understand.
We just released a course on the freeCodeCamp YouTube channel that is a beginner-friendly introduction to common data structures (linked lists, stacks, queues, graphs) and algorithms (search, sorting, recursion, dynamic programming) in Python.
This course will help you prepare for coding interviews and assessments. In this course, you will:
- Watch live hands-on coding-focused video tutorials
- Practice coding with cloud Jupyter notebooks
- Solve questions from real programming interviews
Aakash N S teaches this course. He is the co-founder and CEO of Jovian and has created many popular courses about machine learning and programming.
The course is broken up into a series of lessons, assignments, and projects. There are Jupyter Notebook files to go along with each section.
Here is what is covered in the course:
Lesson 1 - Binary Search, Linked Lists and Complexity
- Linear and Binary Search
- Complexity and Big O Notation
- Linked Lists using Python Classes
Assignment 1 - Binary Search Practice
- Understand and solve a problem systematically
- Implement linear search and analyze it
- Optimize the solution using binary search
Lesson 2 - Binary Search Trees, Traversals and Recursion
- Binary trees, traversals, and recursion
- Binary search trees & common operations
- Balanced binary trees and optimizations
Assignment 2 - Hash Tables and Python Dictionaries
- Hash tables from scratch in Python
- Handling collisions using linear probing
- Replicating Python dictionaries
Lesson 3 - Sorting Algorithms and Divide & Conquer
- Bubble sort and Insertion Sort
- Merge sort using Divide & Conquer
- Quicksort and average complexity
Assignment 3 - Divide and Conquer Practice
- Implement polynomial multiplication
- Optimize using divide and conquer
- Analyze time and space complexity
Lesson 4 - Recursion and Dynamic Programming
- Recursion and memoization
- Subsequence and knapsack problems
- Backtracking and pruning
Lesson 5 - Graph Algorithms (BFS, DFS & Shortest Paths)
- Graphs, trees, and adjacency lists
- Breadth-first and depth-first search
- Shortest paths and directed graphs
Project - Step-by-Step Solution to a Programming Problem
- Pick an interesting coding problem
- Solve the problem step-by-step
- Document and present the solution
Lesson 6 - Python Interview Questions, Tips & Advice
- Practice questions and solutions
- Tips for solving coding challenges
- Advice for cracking coding interviews
Watch the course below or on the freeCodeCamp.org YouTube channel (13-hour watch).
I'm a teacher and developer with freeCodeCamp.org. I run the freeCodeCamp.org YouTube channel.
If this article was helpful, share it .
Learn to code for free. freeCodeCamp's open source curriculum has helped more than 40,000 people get jobs as developers. Get started
Discover the Top 75 Free Courses for August
Udemy Announces Layoffs Without Saying ‘Layoffs’
Udemy’s latest ‘Strategic Business Update’ uses corporate euphemisms to signal job cuts while pivoting to enterprise clients.
- 7 Best Sketch Courses for 2024
- 8 Best Free Geology Courses for 2024
- 7 Best Climate Change Courses for 2024: Exploring the Science
- [2024] 110+ Hours of Free LinkedIn Learning Courses with Free Certification
- 7 Best Free Haskell Courses for 2024
600 Free Google Certifications
Most common
- cyber security
Popular subjects
Computer Science
Communication Skills
Project Management
Popular courses
Molecular Biology - Part 1: DNA Replication and Repair
Introduction to Classical Music
Learning How to Learn: Powerful mental tools to help you master tough subjects
Organize and share your learning with Class Central Lists.
View our Lists Showcase
Class Central is learner-supported. When you buy through links on our site, we may earn an affiliate commission.
Data Structures and Algorithms in Python - Full Course for Beginners
via freeCodeCamp Help
Introduction. Binary Search Linked Lists and Complexity. Introduction. Problem. The Method. Solution. Complexity and Big O notation. Binary Search vs Linear Search. Generic Binary Search. Summary and Conclusion. Assignment Walkthrough. Introduction. Problem- Rotated Lists. The Method. Solution. Summary and Conclusion. Binary Search Trees Python Tutorial. Introduction. Problem. The Method. Binary tree. Traversing Binary Tree. Binary Search Tree. Self-Balancing Binary Trees and AVL Trees. Summary and Conclusion. Hash Tables and Python Dictionaries. Introduction. Problem. Data List. Hash Function. Basic Hash Table Implementation. Handling Collisions with Linear Probing. Summary and Conclusion. Sorting Algorithms and Divide & Conquer. Introduction. Problem. The Method. Custom Comparison Functions. Summary and Conclusion. Recursion Memoization & Dynamic Programming. Introduction. Problem. The Method. Solution. Knapsack Problems. The Method. Solution. Summary and Conclusion. Graph Algorithms BFS, DFS & Shortest Paths. Introduction. Graph Data Structure. Graph Algorithms - Breadth-First Search. Depth-First Search. Shortest Paths. Summary and Conclusion. Python Interview Questions Tips & Advice. Introduction. The Method. Solution. Summary and Conclusion.
freeCodeCamp.org
Related Courses
Data structures and algorithms in python, data structures and algorithms with visualizations – full course (java), learn data structures and algorithms with python, data structures and algorithms in javascript, master technical interviews – full course, related articles, 10 best data structures & algorithms courses.
4.7 rating, based on 37 Class Central reviews
Select rating
Start your review of Data Structures and Algorithms in Python - Full Course for Beginners
- Sailesh Tuniki 1 month ago The "Data Structures and Algorithms in Python - Full Course for Beginners" by freeCodeCamp is an excellent resource for anyone looking to gain a solid foundation in computer science. This comprehensive course covers a wide range of fundamental topic… Read more The "Data Structures and Algorithms in Python - Full Course for Beginners" by freeCodeCamp is an excellent resource for anyone looking to gain a solid foundation in computer science. This comprehensive course covers a wide range of fundamental topics, including arrays, linked lists, stacks, queues, trees, and graphs, as well as essential algorithms such as sorting and searching. The instructor explains each concept in a clear and concise manner, making it easy for beginners to understand and follow along. The use of Python, a popular and beginner-friendly programming language, enhances the learning experience. Practical coding exercises and examples are provided throughout the course, allowing learners to apply what they've learned and reinforce their understanding. Additionally, the course emphasizes the importance of algorithmic thinking and problem-solving skills, which are crucial for any aspiring programmer. Overall, this course is a valuable resource for anyone looking to master data structures and algorithms using Python, providing a solid foundation for further studies and practical applications in software development. Helpful
- Ram Narayana @Narayana 1 month ago The course was very knowledgeable and clear and would definitely visit it again to brush up the concepts and have a deeper understanding Helpful
- KRISHNADHARSHINI L 7 months ago The data structures and algorithm in python course was very helpful.My foundation has become stronger and i am confident in my basics than before learning this course.The "Data Structures and Algorithms in Python" course for beginners is a comprehen… Read more The data structures and algorithm in python course was very helpful.My foundation has become stronger and i am confident in my basics than before learning this course.The "Data Structures and Algorithms in Python" course for beginners is a comprehensive and well-structured learning resource. It covers fundamental concepts with clarity, offering a solid foundation for understanding data structures and algorithms. The instructor effectively communicates complex ideas, making it accessible for beginners. The course incorporates practical examples and coding exercises, enhancing hands-on learning. The pacing is suitable for beginners, ensuring a gradual and thorough understanding. Overall, it's a commendable course that combines theoretical knowledge with practical implementation, making it an excellent choice for those looking to grasp the essentials of data structures and algorithms in Python. Helpful
- Adirala Uday 2 weeks ago "I just completed the 'Data Structures and Algorithms in Python - Full Course for Beginners' on freeCodeCamp, and I'm blown away by the quality of the content! As a beginner, I found the explanations clear, concise, and easy to follow. The course c… Read more "I just completed the 'Data Structures and Algorithms in Python - Full Course for Beginners' on freeCodeCamp, and I'm blown away by the quality of the content! As a beginner, I found the explanations clear, concise, and easy to follow. The course covers a wide range of topics, from basic data structures like arrays and linked lists to advanced algorithms like dynamic programming and greedy algorithms. The best part? The course is entirely hands-on, with plenty of coding challenges and projects to help reinforce your understanding. I loved the interactive coding environment, which made it easy to write and test my code. The instructors are knowledgeable and enthusiastic, making even the most complex concepts seem approachable. I appreciated the emphasis on problem-solving strategies and critical thinking. Whether you're new to programming or looking to brush up on your skills, this course is an excellent resource. I feel confident in my ability to tackle real-world problems and excited to continue learning. Thanks, freeCodeCamp, for another fantastic course!" Helpful
- AA Anonymous 1 week ago the data structures and algorithm in python course was very helpful.My foundation has become stronger and i am confident in my basics than before learning this course.The "Data Structures and Algorithms in Python" course for beginners is a comprehen… Read more the data structures and algorithm in python course was very helpful.My foundation has become stronger and i am confident in my basics than before learning this course.The "Data Structures and Algorithms in Python" course for beginners is a comprehensive and well-structured learning resource. It covers fundamental concepts with clarity, offering a solid foundation for understanding data structures and algorithms. The instructor effectively communicates complex ideas, making it accessible for beginners. The course incorporates practical examples and coding exercises, enhancing hands-on learning. The pacing is suitable for beginners, ensuring a gradual and thorough understanding. Overall, it's a commendable course that combines theoretical knowledge with practical implementation, making it an excellent choice for those looking to grasp the essentials of data structures and algorithms in Python. Helpful
- ANIKETH MOHITE 2 months ago The "Data Structures and Algorithms in Python - Full Course for Beginners" provides a comprehensive introduction to fundamental concepts essential for anyone starting their journey in programming. With clear explanations and practical examples, this course covers various data structures like arrays, linked lists, stacks, queues, trees, and graphs, as well as essential algorithms like sorting, searching, and recursion. The instructor's teaching style is engaging and easy to follow, making complex topics accessible even for beginners. Overall, this course is an excellent resource for building a solid foundation in data structures and algorithms using Python, setting learners up for success in their programming endeavors. Helpful
- Madala Mahesh 2 weeks ago Thanks to free code camp, this course on data structures and algorithms in python is very helpful and useful to me ans many others, it covers essential topics necessary for understanding and implementing efficient solutions to real world problems. The course content is generally comprehensive, covering a wide range of data structures such as arrays, linked list, stacks,trees, heaps, and graphs.It also dives into various algorithms,it providing code examples and exercises to reinforce learning, and it also provides interview questions and answers. Helpful
- VK Vendra Venkat Krishna 1 month ago I would definitely recommend this course to my friends. The 'DSA in Python for Beginners' course is an excellent starting point for anyone looking to delve into the world of data structures and algorithms. Its well-structured curriculum introduces complex concepts in an easy-to-understand manner, making it accessible even to those new to programming. Helpful
- GG Gobinath G 2 weeks ago I recently completed the "Data Structures and Algorithms in Python" course, and I found it to be highly informative and well-structured. The course provides a comprehensive overview of key concepts, including arrays, linked lists, stacks, queues, trees, and graphs, along with detailed explanations of various algorithms like sorting and searching. Helpful
- Anushri Anil Rajeshirke 4 days ago Thank you for a great course. Great presentation style with lots of opportunities to ask questions and talk about real life examples which all made for a really enjoyable and informative course. This has more than met my expectations. A wonderfully practical course. Helpful
- Dhanyamraju Laasya Priya 2 months ago It was a very helpful for a student who is curious about learning data structures and algorithms. It is an effective way to learn data structures and algorithms by completing the assignments provided in videos. this course made easy for self-taught learners. Helpful
- Barath Raja. M 1 week ago Comprehensive guide to data structures and algorithms in Python. Clear explanations, practical examples, and exercises make it an excellent resource for beginners and experienced programmers. Nice experience with more knowledge Helpful
- VR Varikuti Ramyasri 2 weeks ago Course contents are good but coming to explain if there is some basic information related to the topics along with practical. Everything was good but when explaining the content they should consider the beginning people also Helpful
- Janani Jn 4 days ago Gained More knowledge about data structure in python, clearly explained each and every concept. It was very easy to understand.More practical questions have explained clearly.without any doubt Helpful
- N Surya Vamshi Babu 3 weeks ago overall its a very good course for me to know in depth about the data structures and algorithms and i liked it a lot and gained some good grip on these data structures and algorithms in python.. Helpful
- Uday Kiran 3 weeks ago This course helped me to improve my skills and added weightage to my resume to achieve to my goals. It was in a beginner manner, so that i was able to understand the course in a proper way. Helpful
- KS KOTHA SHIVANI 3 weeks ago As a beginner to learn the Data structures,the course is very helpful and informative to understand and perform algorithms in python. I gain a knowledge on DSA which I am aware if. Helpful
- Kendyala Supriya 3 weeks ago Comprehensive course on Data Structures & Algorithms in Python. Clear explanations, practical exercises, and supportive community. Highly recommended for all levels. Helpful
- PV Puvvala Vedasri 2 weeks ago Helpful for my career and I had learn about data structures and algorithms.it is a wonderful experience to learn this ..I had learn a lot of new things... Helpful
- Ajay Govindula 3 weeks ago The course is very informative which helps a person to improve their DSA skills.And provides a good knowledge on how to work with algorithms. Helpful
Never Stop Learning.
Get personalized course recommendations, track subjects and courses with reminders, and more.
Navigation Menu
Search code, repositories, users, issues, pull requests..., provide feedback.
We read every piece of feedback, and take your input very seriously.
Saved searches
Use saved searches to filter your results more quickly.
To see all available qualifiers, see our documentation .
- Notifications You must be signed in to change notification settings

IMAGES
VIDEO
COMMENTS
This repository contains week wise solutions to Coursera assignments given by University of Michigan - vennela03/Python-Data-Structures-University-of-Michigan
Class notes. Contribute to ed-lau/python-for-everybody development by creating an account on GitHub.
8.4 Open the file romeo.txt and read it line by line. For each line, split the line into a list of words using the split () method. The program should build a list of words. For each word on each line check to see if the word is already in the list and if not append it to the list. When the program completes,
Python Data Structures Assignment 8.4 Solution [Coursera] | Assignment 8.4 Python Data Structures Codeshala 19.7K subscribers 156 19K views 3 years ago PYTHON DATA STRUCTURES [University OF Michigan]
Learn how to choose and use Python's data structures, such as lists, tuples, dictionaries, sets, and more, with this practical tutorial.
Python For Everybody Assignment 8.4 | split () | romeo.txt |append, sort, print in alphabetical order Go Geeks 659 subscribers Subscribed 62 4.6K views 2 years ago #alphabeticalorder #split #print
About Press Copyright Contact us Creators Advertise Developers Terms Privacy Policy & Safety How YouTube works Test new features NFL Sunday Ticket © 2024 Google LLC
Data Structures — Python 3.12.4 documentation. 5. Data Structures ¶. This chapter describes some things you've learned about already in more detail, and adds some new things as well. 5.1. More on Lists ¶. The list data type has some more methods. Here are all of the methods of list objects:
Assignment 7.2. Write a program that prompts for a file name, then opens that file and reads through the file, looking for lines of the form: X-DSPAM-Confidence: 0.8475. Count these lines and extract the floating point values from each of the lines and compute the average of those values and produce an output as shown below.
In this article, we will discuss the Data Structures in the Python Programming Language and how they are related to some specific Python Data Types. We will discuss all the in-built data structures like list tuples, dictionaries, etc. as well as some advanced data structures like trees, graphs, etc.
This data structure exercise is for beginners to understand and practice data structure in Python. When you complete each question, you get more familiar with List, Dictionary, set, and tuple.
This tutorial is a beginner-friendly guide for learning data structures and algorithms using Python. In this article, we will discuss the in-built data structures such as lists, tuples, dictionaries, etc, and some user-defined data structures such as linked lists, trees, graphs, etc, and traversal as well as searching and sorting algorithms with the help of good and well-explained examples and ...
Python for everybody: data structures assignment 8.4 요해인 17 subscribers Subscribed 5 397 views 3 years ago #pythonforeverybody
Coursera courses for the Python for Everybody Specialization. - Python-for-Everybody-Coursera/Python Data Structures/Chapter 8/Assignment 8.4.py at master · atse0612/Python-for-Everybody-Coursera
Coursera-Data_Structures_and_Algorithms This repository contains my solutions to the Data Structures and Algorithms assignments offered by the University of California, San Diego (UCSD) and the National Research University Higher School of Economics (HSE) on Coursera. All of the problems from courses 1 through 6 have been solved using Python.
Get started. Algorithms and data structures are important for most programmers to understand. We just released a course on the freeCodeCamp YouTube channel that is a beginner-friendly introduction to common data structures (linked lists, stacks, queues, graphs) and algorithms (search, sorting, recursion, dynamic programming) in Python.
Coursera: 8.4 Assignment// python data structures assignment 8.4 solution ManoranjanVibe 579 subscribers Subscribed 42 2.9K views 4 years ago INDIA
A beginner-friendly introduction to common data structures (linked lists, stacks, queues, graphs) and algorithms (search, sorting, recursion, dynamic programming) in Python. This course will help you prepare for coding interviews and assessments.
Here is All Weeks Assignment for Python Data Structures Course on Coursera - Coursera---Python-Data-Structures/Week-4/Assignment8.5.py at master · PragneshRamani ...