ORIGINAL RESEARCH article
Explainable automated essay scoring: deep learning really has pedagogical value.

- School of Computing and Information Systems, Faculty of Science and Technology, Athabasca University, Edmonton, AB, Canada
Automated essay scoring (AES) is a compelling topic in Learning Analytics for the primary reason that recent advances in AI find it as a good testbed to explore artificial supplementation of human creativity. However, a vast swath of research tackles AES only holistically; few have even developed AES models at the rubric level, the very first layer of explanation underlying the prediction of holistic scores. Consequently, the AES black box has remained impenetrable. Although several algorithms from Explainable Artificial Intelligence have recently been published, no research has yet investigated the role that these explanation models can play in: (a) discovering the decision-making process that drives AES, (b) fine-tuning predictive models to improve generalizability and interpretability, and (c) providing personalized, formative, and fine-grained feedback to students during the writing process. Building on previous studies where models were trained to predict both the holistic and rubric scores of essays, using the Automated Student Assessment Prize’s essay datasets, this study focuses on predicting the quality of the writing style of Grade-7 essays and exposes the decision processes that lead to these predictions. In doing so, it evaluates the impact of deep learning (multi-layer perceptron neural networks) on the performance of AES. It has been found that the effect of deep learning can be best viewed when assessing the trustworthiness of explanation models. As more hidden layers were added to the neural network, the descriptive accuracy increased by about 10%. This study shows that faster (up to three orders of magnitude) SHAP implementations are as accurate as the slower model-agnostic one. It leverages the state-of-the-art in natural language processing, applying feature selection on a pool of 1592 linguistic indices that measure aspects of text cohesion, lexical diversity, lexical sophistication, and syntactic sophistication and complexity. In addition to the list of most globally important features, this study reports (a) a list of features that are important for a specific essay (locally), (b) a range of values for each feature that contribute to higher or lower rubric scores, and (c) a model that allows to quantify the impact of the implementation of formative feedback.
Automated essay scoring (AES) is a compelling topic in Learning Analytics (LA) for the primary reason that recent advances in AI find it as a good testbed to explore artificial supplementation of human creativity. However, a vast swath of research tackles AES only holistically; only a few have even developed AES models at the rubric level, the very first layer of explanation underlying the prediction of holistic scores ( Kumar et al., 2017 ; Taghipour, 2017 ; Kumar and Boulanger, 2020 ). None has attempted to explain the whole decision process of AES, from holistic scores to rubric scores and from rubric scores to writing feature modeling. Although several algorithms from XAI (explainable artificial intelligence) ( Adadi and Berrada, 2018 ; Murdoch et al., 2019 ) have recently been published (e.g., LIME, SHAP) ( Ribeiro et al., 2016 ; Lundberg and Lee, 2017 ), no research has yet investigated the role that these explanation models (trained on top of predictive models) can play in: (a) discovering the decision-making process that drives AES, (b) fine-tuning predictive models to improve generalizability and interpretability, and (c) providing teachers and students with personalized, formative, and fine-grained feedback during the writing process.
One of the key anticipated benefits of AES is the elimination of human bias such as rater fatigue, rater’s expertise, severity/leniency, scale shrinkage, stereotyping, Halo effect, rater drift, perception difference, and inconsistency ( Taghipour, 2017 ). At its turn, AES may suffer from its own set of biases (e.g., imperfections in training data, spurious correlations, overrepresented minority groups), which has incited the research community to look for ways to make AES more transparent, accountable, fair, unbiased, and consequently trustworthy while remaining accurate. This required changing the perception that AES is merely a machine learning and feature engineering task ( Madnani et al., 2017 ; Madnani and Cahill, 2018 ). Hence, researchers have advocated that AES should be seen as a shared task requiring several methodological design decisions along the way such as curriculum alignment, construction of training corpora, reliable scoring process, and rater performance evaluation, where the goal is to build and deploy fair and unbiased scoring models to be used in large-scale assessments and classroom settings ( Rupp, 2018 ; West-Smith et al., 2018 ; Rupp et al., 2019 ). Unfortunately, although these measures are intended to design reliable and valid AES systems, they may still fail to build trust among users, keeping the AES black box impenetrable for teachers and students.
It has been previously recognized that divergence of opinion among human and machine graders has been only investigated superficially ( Reinertsen, 2018 ). So far, researchers investigated the characteristics of essays through qualitative analyses which ended up rejected by AES systems (requiring a human to score them) ( Reinertsen, 2018 ). Others strived to justify predicted scores by identifying essay segments that actually caused the predicted scores. In spite of the fact that these justifications hinted at and quantified the importance of these spatial cues, they did not provide any feedback as to how to improve those suboptimal essay segments ( Mizumoto et al., 2019 ).
Related to this study and the work of Kumar and Boulanger (2020) is Revision Assistant, a commercial AES system developed by Turnitin ( Woods et al., 2017 ; West-Smith et al., 2018 ), which in addition to predicting essays’ holistic scores provides formative, rubric-specific, and sentence-level feedback over multiple drafts of a student’s essay. The implementation of Revision Assistant moved away from the traditional approach to AES, which consists in using a limited set of features engineered by human experts representing only high-level characteristics of essays. Like this study, it rather opted for including a large number of low-level writing features, demonstrating that expert-designed features are not required to produce interpretable predictions. Revision Assistant’s performance was reported on two essay datasets, one of which was the Automated Student Assessment Prize (ASAP) 1 dataset. However, performance on the ASAP dataset was reported in terms of quadratic weighted kappa and this for holistic scores only. Models predicting rubric scores were trained only with the other dataset which was hosted on and collected through Revision Assistant itself.
In contrast to feature-based approaches like the one adopted by Revision Assistant, other AES systems are implemented using deep neural networks where features are learned during model training. For example, Taghipour (2017) in his doctoral dissertation leverages a recurrent neural network to improve accuracy in predicting holistic scores, implement rubric scoring (i.e., organization and argument strength), and distinguish between human-written and computer-generated essays. Interestingly, Taghipour compared the performance of his AES system against other AES systems using the ASAP corpora, but he did not use the ASAP corpora when it came to train rubric scoring models although ASAP provides two corpora provisioning rubric scores (#7 and #8). Finally, research was also undertaken to assess the generalizability of rubric-based models by performing experiments across various datasets. It was found that the predictive power of such rubric-based models was related to how much the underlying feature set covered a rubric’s criteria ( Rahimi et al., 2017 ).
Despite their numbers, rubrics (e.g., organization, prompt adherence, argument strength, essay length, conventions, word choices, readability, coherence, sentence fluency, style, audience, ideas) are usually investigated in isolation and not as a whole, with the exception of Revision Assistant which provides feedback at the same time on the following five rubrics: claim, development, audience, cohesion, and conventions. The literature reveals that rubric-specific automated feedback includes numerical rubric scores as well as recommendations on how to improve essay quality and correct errors ( Taghipour, 2017 ). Again, except for Revision Assistant which undertook a holistic approach to AES including holistic and rubric scoring and provision of rubric-specific feedback at the sentence level, AES has generally not been investigated as a whole or as an end-to-end product. Hence, the AES used in this study and developed by Kumar and Boulanger (2020) is unique in that it uses both deep learning (multi-layer perceptron neural network) and a huge pool of linguistic indices (1592), predicts both holistic and rubric scores, explaining holistic scores in terms of rubric scores, and reports which linguistic indices are the most important by rubric. This study, however, goes one step further and showcases how to explain the decision process behind the prediction of a rubric score for a specific essay, one of the main AES limitations identified in the literature ( Taghipour, 2017 ) that this research intends to address, at least partially.
Besides providing explanations of predictions both globally and individually, this study not only goes one step further toward the automated provision of formative feedback but also does so in alignment with the explanation model and the predictive model, allowing to better map feedback to the actual characteristics of an essay. Woods et al. (2017) succeeded in associating sentence-level expert-derived feedback with strong/weak sentences having the greatest influence on a rubric score based on the rubric, essay score, and the sentence characteristics. While Revision Assistant’s feature space consists of counts and binary occurrence indicators of word unigrams, bigrams and trigrams, character four-grams, and part-of-speech bigrams and trigrams, they are mainly textual and locational indices; by nature they are not descriptive or self-explanative. This research fills this gap by proposing feedback based on a set of linguistic indices that can encompass several sentences at a time. However, the proposed approach omits locational hints, leaving the merging of the two approaches as the next step to be addressed by the research community.
Although this paper proposes to extend the automated provision of formative feedback through an interpretable machine learning method, it rather focuses on the feasibility of automating it in the context of AES instead of evaluating the pedagogical quality (such as the informational and communicational value of feedback messages) or impact on students’ writing performance, a topic that will be kept for an upcoming study. Having an AES system that is capable of delivering real-time formative feedback sets the stage to investigate (1) when feedback is effective, (2) the types of feedback that are effective, and (3) whether there exist different kinds of behaviors in terms of seeking and using feedback ( Goldin et al., 2017 ). Finally, this paper omits describing the mapping between the AES model’s linguistic indices and a pedagogical language that is easily understandable by students and teachers, which is beyond its scope.

Methodology
This study showcases the application of the PDR framework ( Murdoch et al., 2019 ), which provides three pillars to describe interpretations in the context of the data science life cycle: P redictive accuracy, D escriptive accuracy, and R elevancy to human audience(s). It is important to note that in a broader sense both terms “explainable artificial intelligence” and “interpretable machine learning” can be used interchangeably with the following meaning ( Murdoch et al., 2019 ): “the use of machine-learning models for the extraction of relevant knowledge about domain relationships contained in data.” Here “predictive accuracy” refers to the measurement of a model’s ability to fit data; “descriptive accuracy” is the degree at which the relationships learned by a machine learning model can be objectively captured; and “relevant knowledge” implies that a particular audience gets insights into a chosen domain problem that guide its communication, actions, and discovery ( Murdoch et al., 2019 ).
In the context of this article, formative feedback that assesses students’ writing skills and prescribes remedial writing strategies is the relevant knowledge sought for, whose effectiveness on students’ writing performance will be validated in an upcoming study. However, the current study puts forward the tools and evaluates the feasibility to offer this real-time formative feedback. It also measures the predictive and descriptive accuracies of AES and explanation models, two key components to generate trustworthy interpretations ( Murdoch et al., 2019 ). Naturally, the provision of formative feedback is dependent on the speed of training and evaluating new explanation models every time a new essay is ingested by the AES system. That is why this paper investigates the potential of various SHAP implementations for speed optimization without compromising the predictive and descriptive accuracies. This article will show how the insights generated by the explanation model can serve to debug the predictive model and contribute to enhance the feature selection and/or engineering process ( Murdoch et al., 2019 ), laying the foundation for the provision of actionable and impactful pieces of knowledge to educational audiences, whose relevancy will be judged by the human stakeholders and estimated by the magnitude of resulting changes.
Figure 1 overviews all the elements and steps encompassed by the AES system in this study. The following subsections will address each facet of the overall methodology, from hyperparameter optimization to relevancy to both students and teachers.
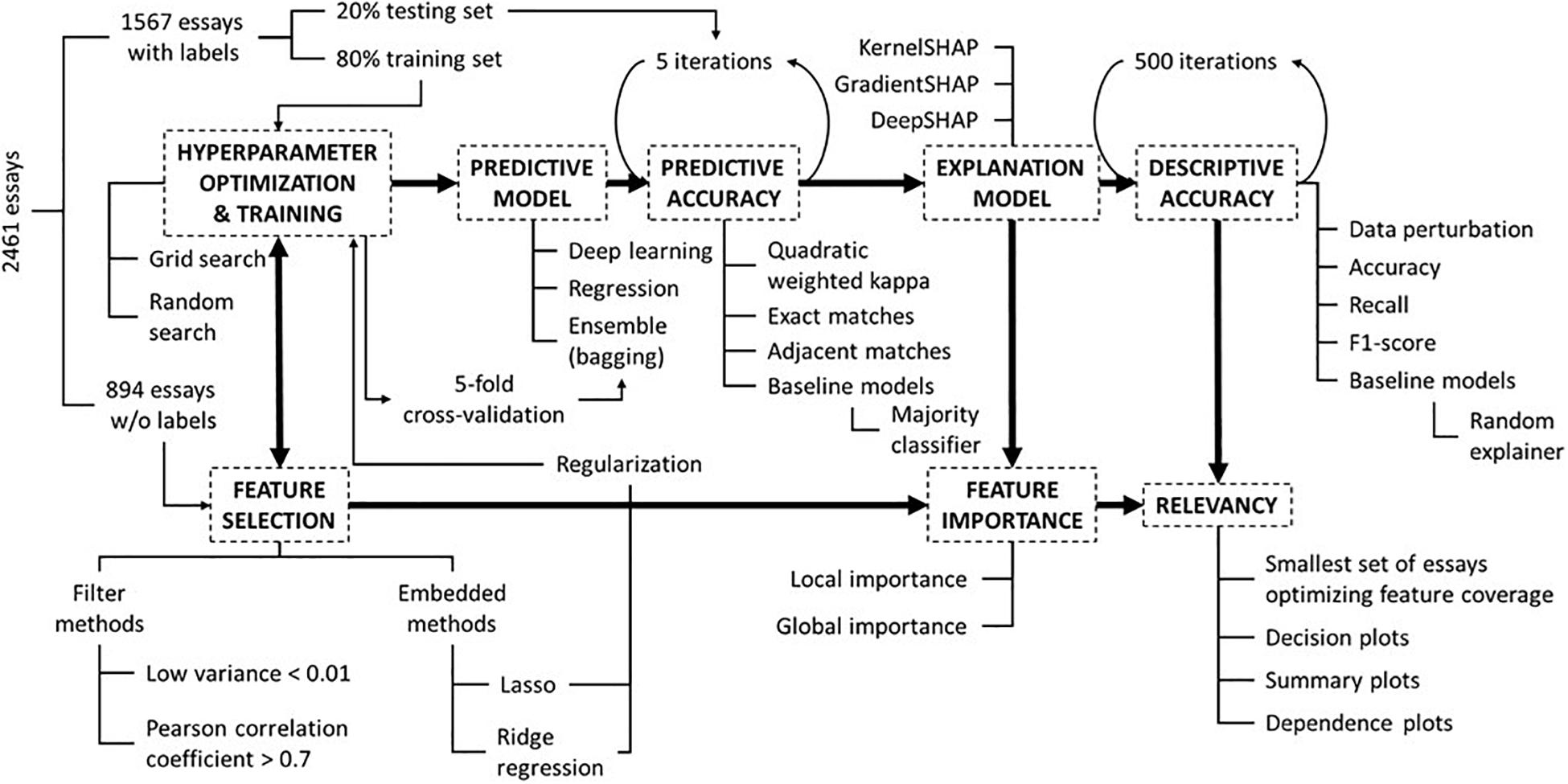
Figure 1. A flow chart exhibiting the sequence of activities to develop an end-to-end AES system and how the various elements work together to produce relevant knowledge to the intended stakeholders.
Automated Essay Scoring System, Dataset, and Feature Selection
As previously mentioned, this paper reuses the AES system developed by Kumar and Boulanger (2020) . The AES models were trained using the ASAP’s seventh essay corpus. These narrative essays were written by Grade-7 students in the setting of state-wide assessments in the United States and had an average length of 171 words. Students were asked to write a story about patience. Kumar and Boulanger’s work consisted in training a predictive model for each of the four rubrics according to which essays were graded: ideas, organization, style, and conventions. Each essay was scored by two human raters on a 0−3 scale (integer scale). Rubric scores were resolved by adding the rubric scores assigned by the two human raters, producing a resolved rubric score between 0 and 6. This paper is a continuation of Boulanger and Kumar (2018 , 2019 , 2020) and Kumar and Boulanger (2020) where the objective is to open the AES black box to explain the holistic and rubric scores that it predicts. Essentially, the holistic score ( Boulanger and Kumar, 2018 , 2019 ) is determined and justified through its four rubrics. Rubric scores, in turn, are investigated to highlight the writing features that play an important role within each rubric ( Kumar and Boulanger, 2020 ). Finally, beyond global feature importance, it is not only indispensable to identify which writing indices are important for a particular essay (local), but also to discover how they contribute to increase or decrease the predicted rubric score, and which feature values are more/less desirable ( Boulanger and Kumar, 2020 ). This paper is a continuation of these previous works by adding the following link to the AES chain: holistic score, rubric scores, feature importance, explanations, and formative feedback. The objective is to highlight the means for transparent and trustable AES while empowering learning analytics practitioners with the tools to debug these models and equip educational stakeholders with an AI companion that will semi-autonomously generate formative feedback to teachers and students. Specifically, this paper analyzes the AES reasoning underlying its assessment of the “style” rubric, which looks for command of language, including effective and compelling word choice and varied sentence structure, that clearly supports the writer’s purpose and audience.
This research’s approach to AES leverages a feature-based multi-layer perceptron (MLP) deep neural network to predict rubric scores. The AES system is fed by 1592 linguistic indices quantitatively measured by the Suite of Automatic Linguistic Analysis Tools 2 (SALAT), which assess aspects of grammar and mechanics, sentiment analysis and cognition, text cohesion, lexical diversity, lexical sophistication, and syntactic sophistication and complexity ( Kumar and Boulanger, 2020 ). The purpose of using such a huge pool of low-level writing features is to let deep learning extract the most important ones; the literature supports this practice since there is evidence that features automatically selected are not less interpretable than those engineered ( Woods et al., 2017 ). However, to facilitate this process, this study opted for a semi-automatic strategy that consisted of both filter and embedded methods. Firstly, the original ASAP’s seventh essay dataset consists of a training set of 1567 essays and a validation and testing sets of 894 essays combined. While the texts of all 2461 essays are still available to the public, only the labels (the rubric scores of two human raters) of the training set have been shared with the public. Yet, this paper reused the unlabeled 894 essays of the validation and testing sets for feature selection, a process that must be carefully carried out by avoiding being informed by essays that will train the predictive model. Secondly, feature data were normalized, and features with variances lower than 0.01 were pruned. Thirdly, the last feature of any pair of features having an absolute Pearson correlation coefficient greater than 0.7 was also pruned (the one that comes last in terms of the column ordering in the datasets). After the application of these filter methods, the number of features was reduced from 1592 to 282. Finally, the Lasso and Ridge regression regularization methods (whose combination is also called ElasticNet) were applied during the training of the rubric scoring models. Lasso is responsible for pruning further features, while Ridge regression is entrusted with eliminating multicollinearity among features.
Hyperparameter Optimization and Training
To ensure a fair evaluation of the potential of deep learning, it is of utmost importance to minimally describe this study’s exploration of the hyperparameter space, a step that is often found to be missing when reporting the outcomes of AES models’ performance ( Kumar and Boulanger, 2020 ). First, a study should list the hyperparameters it is going to investigate by testing for various values of each hyperparameter. For example, Table 1 lists all hyperparameters explored in this study. Note that L 1 and L 2 are two regularization hyperparameters contributing to feature selection. Second, each study should also report the range of values of each hyperparameter. Finally, the strategy to explore the selected hyperparameter subspace should be clearly defined. For instance, given the availability of high-performance computing resources and the time/cost of training AES models, one might favor performing a grid (a systematic testing of all combinations of hyperparameters and hyperparameter values within a subspace) or a random search (randomly selecting a hyperparameter value from a range of values per hyperparameter) or both by first applying random search to identify a good starting candidate and then grid search to test all possible combinations in the vicinity of the starting candidate’s subspace. Of particular interest to this study is the neural network itself, that is, how many hidden layers should a neural network have and how many neurons should compose each hidden layer and the neural network as a whole. These two variables are directly related to the size of the neural network, with the number of hidden layers being a defining trait of deep learning. A vast swath of literature is silent about the application of interpretable machine learning in AES and even more about measuring its descriptive accuracy, the two components of trustworthiness. Hence, this study pioneers the comprehensive assessment of deep learning impact on AES’s predictive and descriptive accuracies.
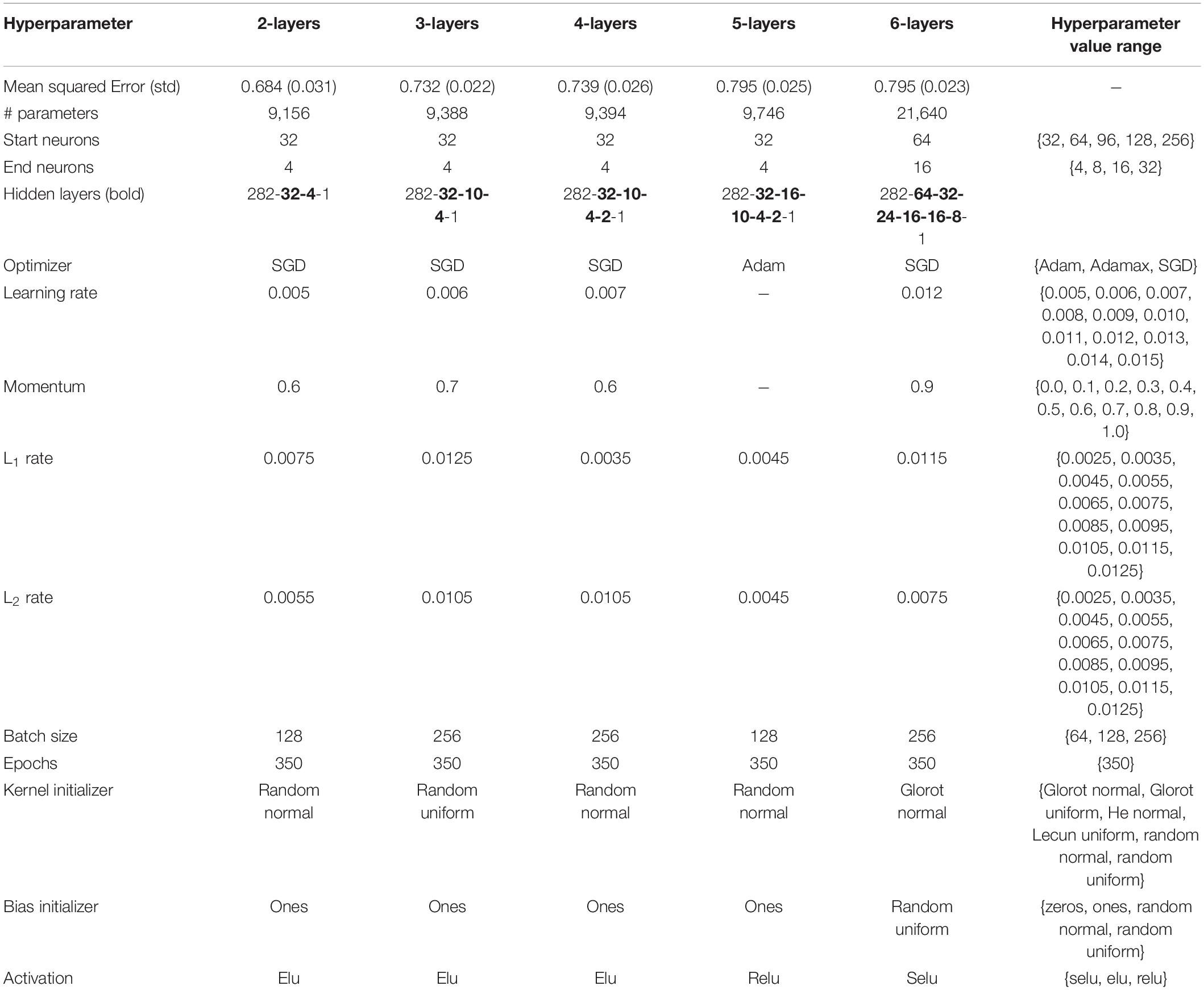
Table 1. Hyperparameter subspace investigated in this article along with best hyperparameter values per neural network architecture.
Consequently, the 1567 labeled essays were divided into a training set (80%) and a testing set (20%). No validation set was put aside; 5-fold cross-validation was rather used for hyperparameter optimization. Table 1 delineates the hyperparameter subspace from which 800 different combinations of hyperparameter values were randomly selected out of a subspace of 86,248,800 possible combinations. Since this research proposes to investigate the potential of deep learning to predict rubric scores, several architectures consisting of 2 to 6 hidden layers and ranging from 9,156 to 119,312 parameters were tested. Table 1 shows the best hyperparameter values per depth of neural networks.
Again, the essays of the testing set were never used during the training and cross-validation processes. In order to retrieve the best predictive models during training, every time the validation loss reached a record low, the model was overwritten. Training stopped when no new record low was reached during 100 epochs. Moreover, to avoid reporting the performance of overfit models, each model was trained five times using the same set of best hyperparameter values. Finally, for each resulting predictive model, a corresponding ensemble model (bagging) was also obtained out of the five models trained during cross-validation.
Predictive Models and Predictive Accuracy
Table 2 delineates the performance of predictive models trained previously by Kumar and Boulanger (2020) on the four scoring rubrics. The first row lists the agreement levels between the resolved and predicted rubric scores measured by the quadratic weighted kappa. The second row is the percentage of accurate predictions; the third row reports the percentages of predictions that are either accurate or off by 1; and the fourth row reports the percentages of predictions that are either accurate or at most off by 2. Prediction of holistic scores is done merely by adding up all rubric scores. Since the scale of rubric scores is 0−6 for every rubric, then the scale of holistic scores is 0−24.
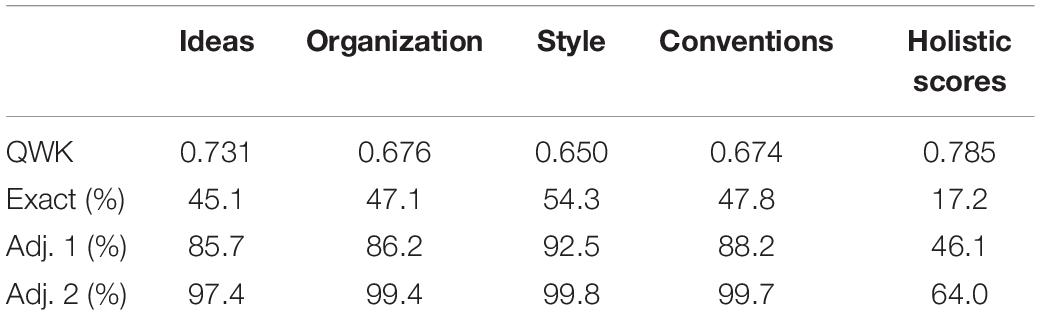
Table 2. Rubric scoring models’ performance on testing set.
While each of these rubric scoring models might suffer from its own systemic bias and hence cancel off each other’s bias by adding up the rubric scores to derive the holistic score, this study (unlike related works) intends to highlight these biases by exposing the decision making process underlying the prediction of rubric scores. Although this paper exclusively focuses on the Style rubric, the methodology put forward to analyze the local and global importance of writing indices and their context-specific contributions to predicted rubric scores is applicable to every rubric and allows to control for these biases one rubric at a time. Comparing and contrasting the role that a specific writing index plays within each rubric context deserves its own investigation, which has been partly addressed in the study led by Kumar and Boulanger (2020) . Moreover, this paper underscores the necessity to measure the predictive accuracy of rubric-based holistic scoring using additional metrics to account for these rubric-specific biases. For example, there exist several combinations of rubric scores to obtain a holistic score of 16 (e.g., 4-4-4-4 vs. 4-3-4-5 vs. 3-5-2-6). Even though the predicted holistic score might be accurate, the rubric scores could all be inaccurate. Similarity or distance metrics (e.g., Manhattan and Euclidean) should then be used to describe the authenticity of the composition of these holistic scores.
According to what Kumar and Boulanger (2020) report on the performance of several state-of-the-art AES systems trained on ASAP’s seventh essay dataset, the AES system they developed and which will be reused in this paper proved competitive while being fully and deeply interpretable, which no other AES system does. They also supply further information about the study setting, essay datasets, rubrics, features, natural language processing (NLP) tools, model training, and evaluation against human performance. Again, this paper showcases the application of explainable artificial intelligence in automated essay scoring by focusing on the decision process of the Rubric #3 (Style) scoring model. Remember that the same methodology is applicable to each rubric.
Explanation Model: SHAP
SH apley A dditive ex P lanations (SHAP) is a theoretically justified XAI framework that can provide simultaneously both local and global explanations ( Molnar, 2020 ); that is, SHAP is able to explain individual predictions taking into account the uniqueness of each prediction, while highlighting the global factors influencing the overall performance of a predictive model. SHAP is of keen interest because it unifies all algorithms of the class of additive feature attribution methods, adhering to a set of three properties that are desirable in interpretable machine learning: local accuracy, missingness, and consistency ( Lundberg and Lee, 2017 ). A key advantage of SHAP is that feature contributions are all expressed in terms of the outcome variable (e.g., rubric scores), providing a same scale to compare the importance of each feature against each other. Local accuracy refers to the fact that no matter the explanation model, the sum of all feature contributions is always equal to the prediction explained by these features. The missingness property implies that the prediction is never explained by unmeasured factors, which are always assigned a contribution of zero. However, the converse is not true; a contribution of zero does not imply an unobserved factor, it can also denote a feature irrelevant to explain the prediction. The consistency property guarantees that a more important feature will always have a greater magnitude than a less important one, no matter how many other features are included in the explanation model. SHAP proves superior to other additive attribution methods such as LIME (Local Interpretable Model-Agnostic Explanations), Shapley values, and DeepLIFT in that they never comply with all three properties, while SHAP does ( Lundberg and Lee, 2017 ). Moreover, the way SHAP assesses the importance of a feature differs from permutation importance methods (e.g., ELI5), measured as the decrease in model performance (accuracy) as a feature is perturbated, in that it is based on how much a feature contributes to every prediction.
Essentially, a SHAP explanation model (linear regression) is trained on top of a predictive model, which in this case is a complex ensemble deep learning model. Table 3 demonstrates a scale explanation model showing how SHAP values (feature contributions) work. In this example, there are five instances and five features describing each instance (in the context of this paper, an instance is an essay). Predictions are listed in the second to last column, and the base value is the mean of all predictions. The base value constitutes the reference point according to which predictions are explained; in other words, reasons are given to justify the discrepancy between the individual prediction and the mean prediction (the base value). Notice that the table does not contain the actual feature values; these are SHAP values that quantify the contribution of each feature to the predicted score. For example, the prediction of Instance 1 is 2.46, while the base value is 3.76. Adding up the feature contributions of Instance 1 to the base value produces the predicted score:
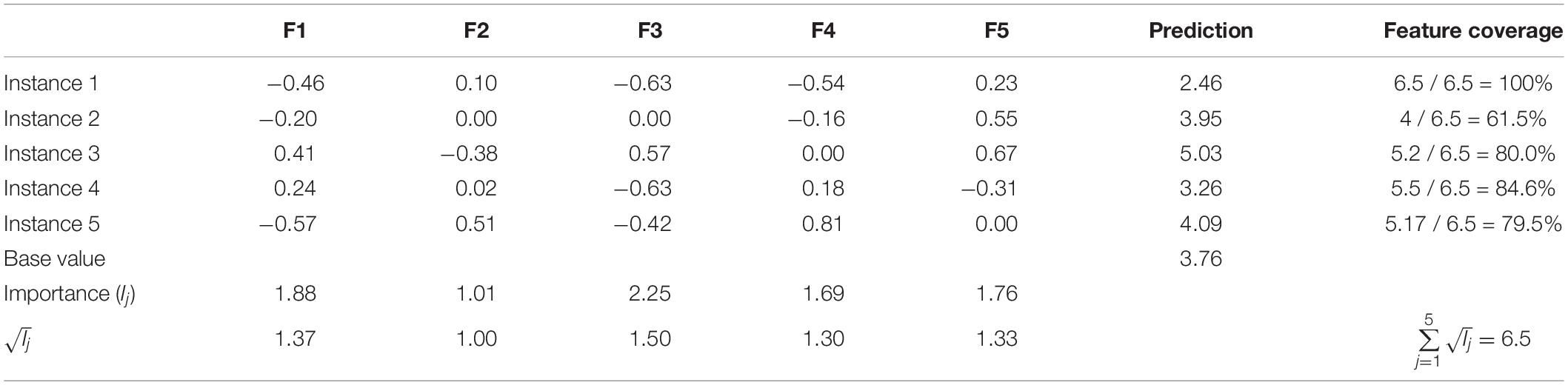
Table 3. Array of SHAP values: local and global importance of features and feature coverage per instance.
Hence, the generic equation of the explanation model ( Lundberg and Lee, 2017 ) is:
where g(x) is the prediction of an individual instance x, σ 0 is the base value, σ i is the feature contribution of feature x i , x i ∈ {0,1} denotes whether feature x i is part of the individual explanation, and j is the total number of features. Furthermore, the global importance of a feature is calculated by adding up the absolute values of its corresponding SHAP values over all instances, where n is the total number of instances and σ i ( j ) is the feature contribution for instance i ( Lundberg et al., 2018 ):
Therefore, it can be seen that Feature 3 is the most globally important feature, while Feature 2 is the least important one. Similarly, Feature 5 is Instance 3’s most important feature at the local level, while Feature 2 is the least locally important. The reader should also note that a feature shall not necessarily be assigned any contribution; some of them are just not part of the explanation such as Feature 2 and Feature 3 in Instance 2. These concepts lay the foundation for the explainable AES system presented in this paper. Just imagine that each instance (essay) will be rather summarized by 282 features and that the explanations of all the testing set’s 314 essays will be provided.
Several implementations of SHAP exist: KernelSHAP, DeepSHAP, GradientSHAP, and TreeSHAP, among others. KernelSHAP is model-agnostic and works for any type of predictive models; however, KernelSHAP is very computing-intensive which makes it undesirable for practical purposes. DeepSHAP and GradientSHAP are two implementations intended for deep learning which takes advantage of the known properties of neural networks (i.e., MLP-NN, CNN, or RNN) to accelerate up to three orders of magnitude the processing time to explain predictions ( Chen et al., 2019 ). Finally, TreeSHAP is the most powerful implementation intended for tree-based models. TreeSHAP is not only fast; it is also accurate. While the three former implementations estimate SHAP values, TreeSHAP computes them exactly. Moreover, TreeSHAP not only measures the contribution of individual features, but it also considers interactions between pairs of features and assigns them SHAP values. Since one of the goals of this paper is to assess the potential of deep learning on the performance of both predictive and explanation models, this research tested the former three implementations. TreeSHAP is recommended for future work since the interaction among features is critical information to consider. Moreover, KernelSHAP, DeepSHAP, and GradientSHAP all require access to the whole original dataset to derive the explanation of a new instance, another constraint TreeSHAP is not subject to.
Descriptive Accuracy: Trustworthiness of Explanation Models
This paper reuses and adapts the methodology introduced by Ribeiro et al. (2016) . Several explanation models will be trained, using different SHAP implementations and configurations, per deep learning predictive model (for each number of hidden layers). The rationale consists in randomly selecting and ignoring 25% of the 282 features feeding the predictive model (e.g., turning them to zero). If it causes the prediction to change beyond a specific threshold (in this study 0.10 and 0.25 were tested), then the explanation model should also reflect the magnitude of this change while ignoring the contributions of these same features. For example, the original predicted rubric score of an essay might be 5; however, when ignoring the information brought in by a subset of 70 randomly selected features (25% of 282), the prediction may turn to 4. On the other side, if the explanation model also predicts a 4 while ignoring the contributions of the same subset of features, then the explanation is considered as trustworthy. This allows to compute the precision, recall, and F1-score of each explanation model (number of true and false positives and true and false negatives). The process is repeated 500 times for every essay to determine the average precision and recall of every explanation model.
Judging Relevancy
So far, the consistency of explanations with predictions has been considered. However, consistent explanations do not imply relevant or meaningful explanations. Put another way, explanations only reflect what predictive models have learned during training. How can the black box of these explanations be opened? Looking directly at the numerical SHAP values of each explanation might seem a daunting task, but there exist tools, mainly visualizations (decision plot, summary plot, and dependence plot), that allow to make sense out of these explanations. However, before visualizing these explanations, another question needs to be addressed: which explanations or essays should be picked for further scrutiny of the AES system? Given the huge number of essays to examine and the tedious task to understand the underpinnings of a single explanation, a small subset of essays should be carefully picked that should represent concisely the state of correctness of the underlying predictive model. Again, this study applies and adapts the methodology in Ribeiro et al. (2016) . A greedy algorithm selects essays whose predictions are explained by as many features of global importance as possible to optimize feature coverage. Ribeiro et al. demonstrated in unrelated studies (i.e., sentiment analysis) that the correctness of a predictive model can be assessed with as few as four or five well-picked explanations.
For example, Table 3 reveals the global importance of five features. The square root of each feature’s global importance is also computed and considered instead to limit the influence of a small group of very influential features. The feature coverage of Instance 1 is 100% because all features are engaged in the explanation of the prediction. On the other hand, Instance 2 has a feature coverage of 61.5% because only Features 1, 4, and 5 are part of the prediction’s explanation. The feature coverage is calculated by summing the square root of each explanation’s feature’s global importance together and dividing by the sum of the square roots of all features’ global importance:
Additionally, it can be seen that Instance 4 does not have any zero-feature value although its feature coverage is only 84.6%. The algorithm was constrained to discard from the explanation any feature whose contribution (local importance) was too close to zero. In the case of Table 3 ’s example, any feature whose absolute SHAP value is less than 0.10 is ignored, hence leading to a feature coverage of:
In this paper’s study, the real threshold was 0.01. This constraint was actually a requirement for the DeepSHAP and GradientSHAP implementations because they only output non-zero SHAP values contrary to KernelSHAP which generates explanations with a fixed number of features: a non-zero SHAP value indicates that the feature is part of the explanation, while a zero value excludes the feature from the explanation. Without this parameter, all 282 features would be part of the explanation although a huge number only has a trivial (very close to zero) SHAP value. Now, a much smaller but variable subset of features makes up each explanation. This is one way in which Ribeiro et al.’s SP-LIME algorithm (SP stands for Submodular Pick) has been adapted to this study’s needs. In conclusion, notice how Instance 4 would be selected in preference to Instance 5 to explain Table 3 ’s underlying predictive model. Even though both instances have four features explaining their prediction, Instance 4’s features are more globally important than Instance 5’s features, and therefore Instance 4 has greater feature coverage than Instance 5.
Whereas Table 3 ’s example exhibits the feature coverage of one instance at a time, this study computes it for a subset of instances, where the absolute SHAP values are aggregated (summed) per candidate subset. When the sum of absolute SHAP values per feature exceeds the set threshold, the feature is then considered as covered by the selected set of instances. The objective in this study was to optimize the feature coverage while minimizing the number of essays to validate the AES model.
Research Questions
One of this article’s objectives is to assess the potential of deep learning in automated essay scoring. The literature has often claimed ( Hussein et al., 2019 ) that there are two approaches to AES, feature-based and deep learning, as though these two approaches were mutually exclusive. Yet, the literature also puts forward that feature-based AES models may be more interpretable than deep learning ones ( Amorim et al., 2018 ). This paper embraces the viewpoint that these two approaches can also be complementary by leveraging the state-of-the-art in NLP and automatic linguistic analysis and harnessing one of the richest pools of linguistic indices put forward in the research community ( Crossley et al., 2016 , 2017 , 2019 ; Kyle, 2016 ; Kyle et al., 2018 ) and applying a thorough feature selection process powered by deep learning. Moreover, the ability of deep learning of modeling complex non-linear relationships makes it particularly well-suited for AES given that the importance of a writing feature is highly dependent on its context, that is, its interactions with other writing features. Besides, this study leverages the SHAP interpretation method that is well-suited to interpret very complex models. Hence, this study elected to work with deep learning models and ensembles to test SHAP’s ability to explain these complex models. Previously, the literature has revealed the difficulty to have at the same time both accurate and interpretable models ( Ribeiro et al., 2016 ; Murdoch et al., 2019 ), where favoring one comes at the expense of the other. However, this research shows how XAI makes it now possible to produce both accurate and interpretable models in the area of AES. Since ensembles have been repeatedly shown to boost the accuracy of predictive models, they were included as part of the tested deep learning architectures to maximize generalizability and accuracy, while making these predictive models interpretable and exploring whether deep learning can even enhance their descriptive accuracy further.
This study investigates the trustworthiness of explanation models, and more specifically, those explaining deep learning predictive models. For instance, does the depth, defined as the number of hidden layers, of an MLP neural network increases the trustworthiness of its SHAP explanation model? The answer to this question will help determine whether it is possible to have very accurate AES models while having competitively interpretable/explainable models, the corner stone for the generation of formative feedback. Remember that formative feedback is defined as “any kind of information provided to students about their actual state of learning or performance in order to modify the learner’s thinking or behavior in the direction of the learning standards” and that formative feedback “conveys where the student is, what are the goals to reach, and how to reach the goals” ( Goldin et al., 2017 ). This notion contrasts with summative feedback which basically is “a justification of the assessment results” ( Hao and Tsikerdekis, 2019 ).
As pointed out in the previous section, multiple SHAP implementations are evaluated in this study. Hence, this paper showcases whether the faster DeepSHAP and GradientSHAP implementations are as reliable as the slower KernelSHAP implementation . The answer to this research question will shed light on the feasibility of providing immediate formative feedback and this multiple times throughout students’ writing processes.
This study also looks at whether a summary of the data produces as trustworthy explanations as those from the original data . This question will be of interest to AES researchers and practitioners because it could allow to significantly decrease the processing time of the computing-intensive and model-agnostic KernelSHAP implementation and test further the potential of customizable explanations.
KernelSHAP allows to specify the total number of features that will shape the explanation of a prediction; for instance, this study experiments with explanations of 16 and 32 features and observes whether there exists a statistically significant difference in the reliability of these explanation models . Knowing this will hint at whether simpler or more complex explanations are more desirable when it comes to optimize their trustworthiness. If there is no statistically significant difference, then AES practitioners are given further flexibility in the selection of SHAP implementations to find the sweet spot between complexity of explanations and speed of processing. For instance, the KernelSHAP implementation allows to customize the number of factors making up an explanation, while the faster DeepSHAP and GradientSHAP do not.
Finally, this paper highlights the means to debug and compare the performance of predictive models through their explanations. Once a model is debugged, the process can be reused to fine-tune feature selection and/or feature engineering to improve predictive models and for the generation of formative feedback to both students and teachers.
The training, validation, and testing sets consist of 1567 essays, each of which has been scored by two human raters, who assigned a score between 0 and 3 per rubric (ideas, organization, style, and conventions). In particular, this article looks at predictive and descriptive accuracy of AES models on the third rubric, style. Note that although each essay has been scored by two human raters, the literature ( Shermis, 2014 ) is not explicit about whether only two or more human raters participated in the scoring of all 1567 essays; given the huge number of essays, it is likely that more than two human raters were involved in the scoring of these essays so that the amount of noise introduced by the various raters’ biases is unknown while probably being at some degree balanced among the two groups of raters. Figure 2 shows the confusion matrices of human raters on Style Rubric. The diagonal elements (dark gray) correspond to exact matches, whereas the light gray squares indicate adjacent matches. Figure 2A delineates the number of essays per pair of ratings, and Figure 2B shows the percentages per pair of ratings. The agreement level between each pair of human raters, measured by the quadratic weighted kappa, is 0.54; the percentage of exact matches is 65.3%; the percentage of adjacent matches is 34.4%; and 0.3% of essays are neither exact nor adjacent matches. Figures 2A,B specify the distributions of 0−3 ratings per group of human raters. Figure 2C exhibits the distribution of resolved scores (a resolved score is the sum of the two human ratings). The mean is 3.99 (with a standard deviation of 1.10), and the median and mode are 4. It is important to note that the levels of predictive accuracy reported in this article are measured on the scale of resolved scores (0−6) and that larger scales tend to slightly inflate quadratic weighted kappa values, which must be taken into account when comparing against the level of agreement between human raters. Comparison of percentages of exact and adjacent matches must also be made with this scoring scale discrepancy in mind.
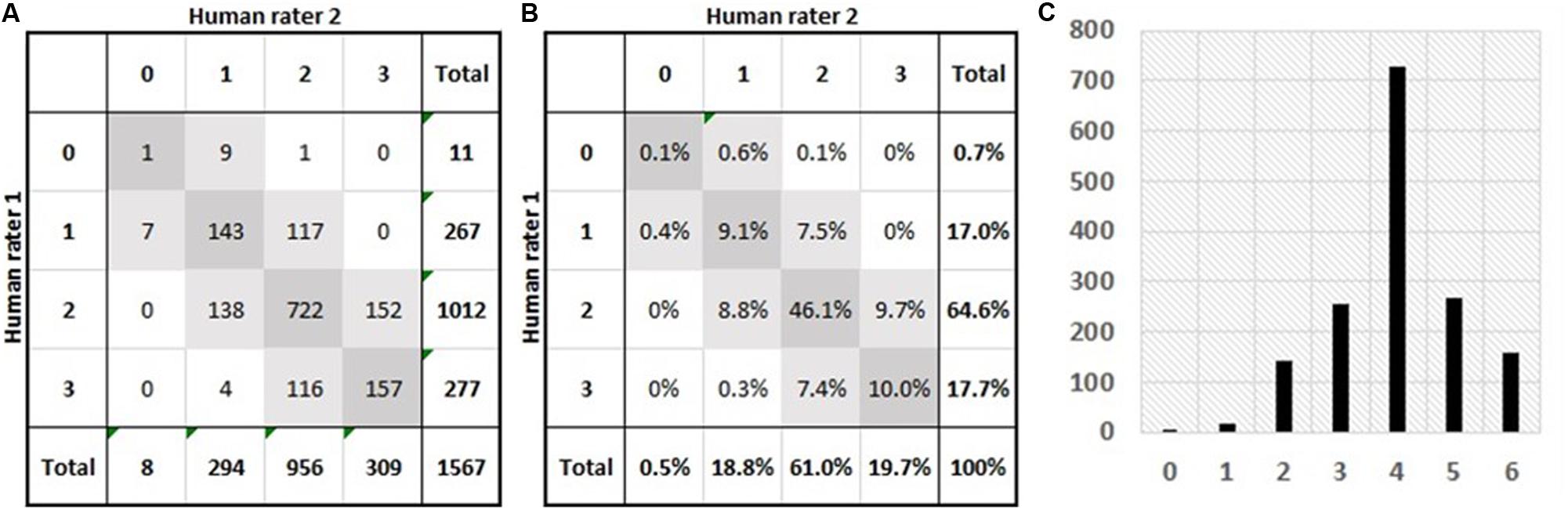
Figure 2. Summary of the essay dataset (1567 Grade-7 narrative essays) investigated in this study. (A) Number of essays per pair of human ratings; the diagonal (dark gray squares) lists the numbers of exact matches while the light-gray squares list the numbers of adjacent matches; and the bottom row and the rightmost column highlight the distributions of ratings for both groups of human raters. (B) Percentages of essays per pair of human ratings; the diagonal (dark gray squares) lists the percentages of exact matches while the light-gray squares list the percentages of adjacent matches; and the bottom row and the rightmost column highlight the distributions (frequencies) of ratings for both groups of human raters. (C) The distribution of resolved rubric scores; a resolved score is the addition of its two constituent human ratings.
Predictive Accuracy and Descriptive Accuracy
Table 4 compiles the performance outcomes of the 10 predictive models evaluated in this study. The reader should remember that the performance of each model was averaged over five iterations and that two models were trained per number of hidden layers, one non-ensemble and one ensemble. Except for the 6-layer models, there is no clear winner among other models. Even for the 6-layer models, they are superior in terms of exact matches, the primary goal for a reliable AES system, but not according to adjacent matches. Nevertheless, on average ensemble models slightly outperform non-ensemble models. Hence, these ensemble models will be retained for the next analysis step. Moreover, given that five ensemble models were trained per neural network depth, the most accurate model among the five is selected and displayed in Table 4 .
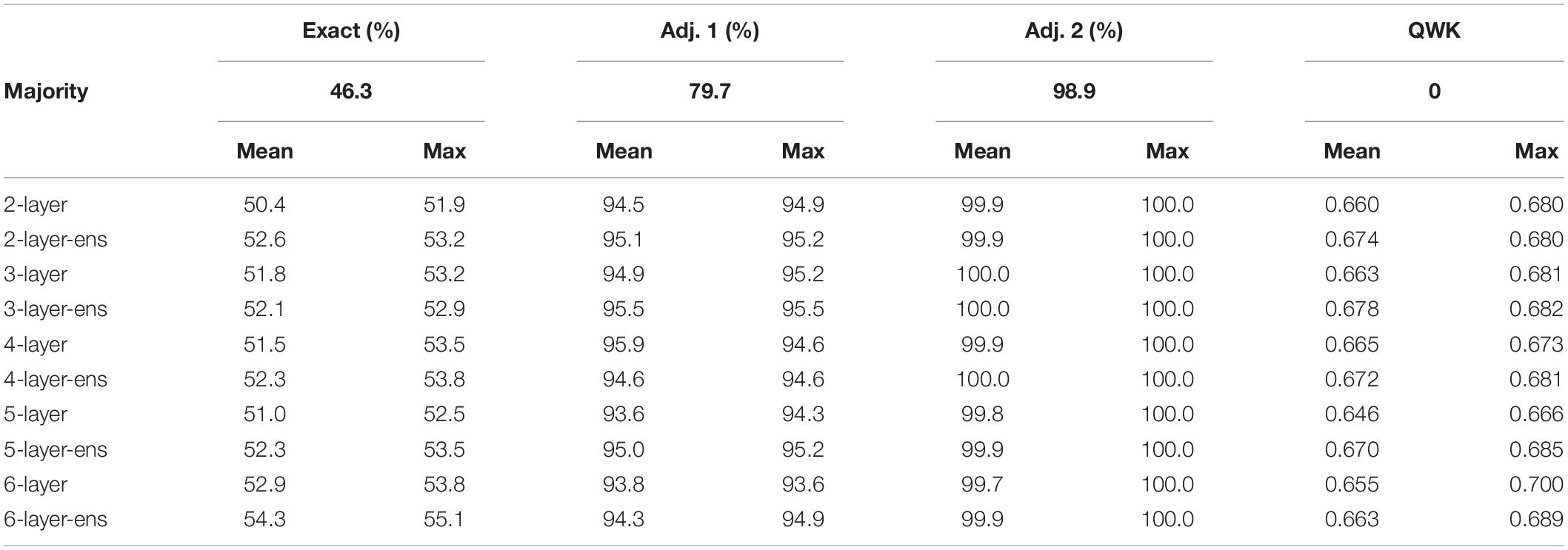
Table 4. Performance of majority classifier and average/maximal performance of trained predictive models.
Next, for each selected ensemble predictive model, several explanation models are trained per predictive model. Every predictive model is explained by the “Deep,” “Grad,” and “Random” explainers, except for the 6-layer model where it was not possible to train a “Deep” explainer apparently due to a bug in the original SHAP code caused by either a unique condition in this study’s data or neural network architecture. However, this was beyond the scope of this study to fix and investigate this issue. As it will be demonstrated, no statistically significant difference exists between the accuracy of these explainers.
The “Random” explainer serves as a baseline model for comparison purpose. Remember that to evaluate the reliability of explanation models, the concurrent impact of randomly selecting and ignoring a subset of features on the prediction and explanation of rubric scores is analyzed. If the prediction changes significantly and its corresponding explanation changes (beyond a set threshold) accordingly (a true positive) or if the prediction remains within the threshold as does the explanation (a true negative), then the explanation is deemed as trustworthy. Hence, in the case of the Random explainer, it simulates random explanations by randomly selecting 32 non-zero features from the original set of 282 features. These random explanations consist only of non-zero features because, according to SHAP’s missingness property, a feature with a zero or a missing value never gets assigned any contribution to the prediction. If at least one of these 32 features is also an element of the subset of the ignored features, then the explanation is considered as untrustworthy, no matter the size of a feature’s contribution.
As for the layer-2 model, six different explanation models are evaluated. Recall that layer-2 models generated the least mean squared error (MSE) during hyperparameter optimization (see Table 1 ). Hence, this specific type of architecture was selected to test the reliability of these various explainers. The “Kernel” explainer is the most computing-intensive and took approximately 8 h of processing. It was trained using the full distributions of feature values in the training set and shaped explanations in terms of 32 features; the “Kernel-16” and “Kernel-32” models were trained on a summary (50 k -means centroids) of the training set to accelerate the processing by about one order of magnitude (less than 1 h). Besides, the “Kernel-16” explainer derived explanations in terms of 16 features, while the “Kernel-32” explainer explained predictions through 32 features. Table 5 exhibits the descriptive accuracy of these various explanation models according to a 0.10 and 0.25 threshold; in other words, by ignoring a subset of randomly picked features, it assesses whether or not the prediction and explanation change simultaneously. Note also how each explanation model, no matter the underlying predictive model, outperforms the “Random” model.
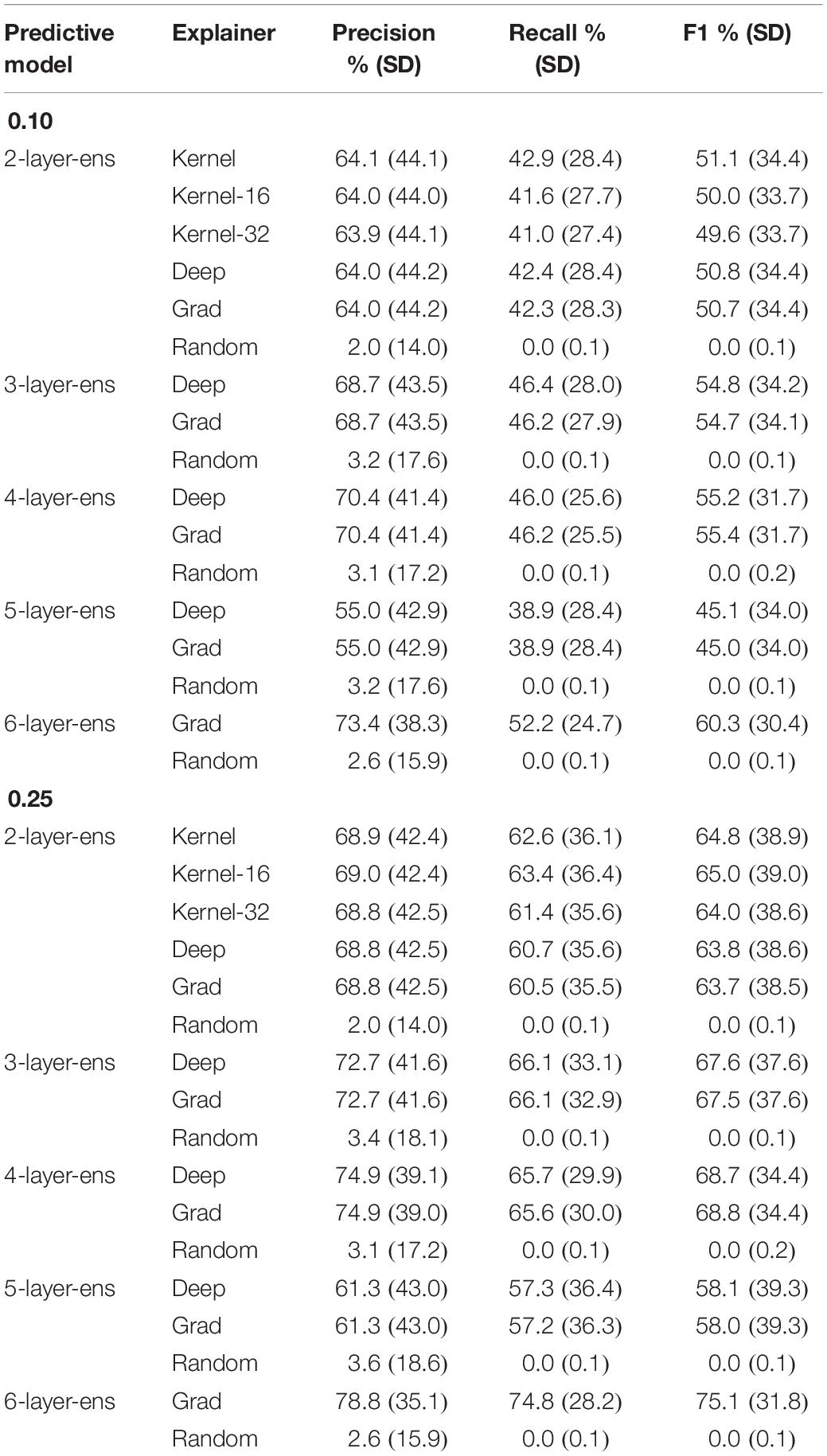
Table 5. Precision, recall, and F1 scores of the various explainers tested per type of predictive model.
The first research question addressed in this subsection asks whether there exists a statistically significant difference between the “Kernel” explainer, which generates 32-feature explanations and is trained on the whole training set, and the “Kernel-32” explainer which also generates 32-feature explanations and is trained on a summary of the training set. To determine this, an independent t-test was conducted using the precision, recall, and F1-score distributions (500 iterations) of both explainers. Table 6 reports the p -values of all the tests and for the 0.10 and 0.25 thresholds. It reveals that there is no statistically significant difference between the two explainers.
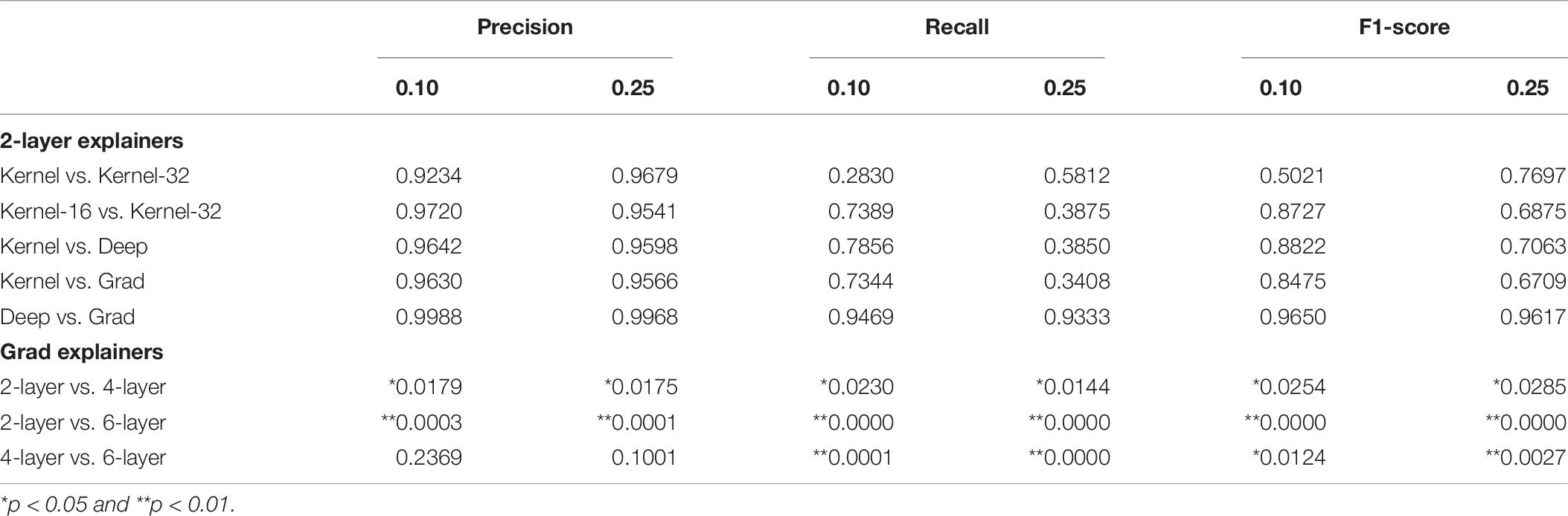
Table 6. p -values of independent t -tests comparing whether there exist statistically significant differences between the mean precisions, recalls, and F1-scores of 2-layer explainers and between those of the 2-layer’s, 4-layer’s, and 6-layer’s Gradient explainers.
The next research question tests whether there exists a difference in the trustworthiness of explainers shaping 16 or 32-feature explanations. Again t-tests were conducted to verify this. Table 6 lists the resulting p -values. Again, there is no statistically significant difference in the average precisions, recalls, and F1-scores of both explainers.
This leads to investigating whether the “Kernel,” “Deep,” and “Grad” explainers are equivalent. Table 6 exhibits the results of the t-tests conducted to verify this and reveals that none of the explainers produce a statistically significantly better performance than the other.
Armed with this evidence, it is now possible to verify whether deeper MLP neural networks produce more trustworthy explanation models. For this purpose, the performance of the “Grad” explainer for each type of predictive model will be compared against each other. The same methodology as previously applied is employed here. Table 6 , again, confirms that the explanation model of the 2-layer predictive model is statistically significantly less trustworthy than the 4-layer’s explanation model; the same can be said of the 4-layer and 6-layer models. The only exception is the difference in average precision between 2-layer and 4-layer models and between 4-layer and 6-layer models; however, there clearly exists a statistically significant difference in terms of precision (and also recall and F1-score) between 2-layer and 6-layer models.
The Best Subset of Essays to Judge AES Relevancy
Table 7 lists the four best essays optimizing feature coverage (93.9%) along with their resolved and predicted scores. Notice how two of the four essays were picked by the adapted SP-LIME algorithm with some strong disagreement between the human and the machine graders, two were picked with short and trivial text, and two were picked exhibiting perfect agreement between the human and machine graders. Interestingly, each pair of longer and shorter essays exposes both strong agreement and strong disagreement between the human and AI agents, offering an opportunity to debug the model and evaluate its ability to detect the presence or absence of more basic (e.g., very small number of words, occurrences of sentence fragments) and more advanced aspects (e.g., cohesion between adjacent sentences, variety of sentence structures) of narrative essay writing and to appropriately reward or penalize them.
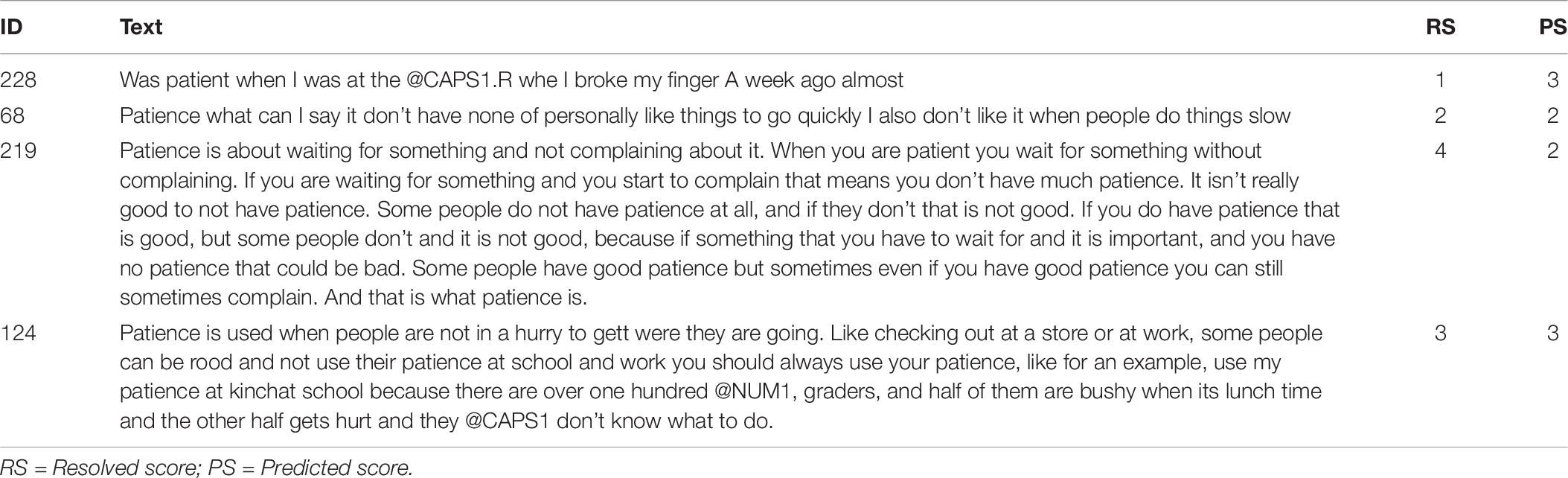
Table 7. Set of best essays to evaluate the correctness of the 6-layer ensemble AES model.
Local Explanation: The Decision Plot
The decision plot lists writing features by order of importance from top to bottom. The line segments display the contribution (SHAP value) of each feature to the predicted rubric score. Note that an actual decision plot consists of all 282 features and that only the top portion of it (20 most important features) can be displayed (see Figure 3 ). A decision plot is read from bottom to top. The line starts at the base value and ends at the predicted rubric score. Given that the “Grad” explainer is the only explainer common to all predictive models, it has been selected to derive all explanations. The decision plots in Figure 3 show the explanations of the four essays in Table 7 ; the dashed line in these plots represents the explanation of the most accurate predictive model, that is the ensemble model with 6 hidden layers which also produced the most trustworthy explanation model. The predicted rubric score of each explanation model is listed in the bottom-right legend. Explanation of the writing features follow in a next subsection.
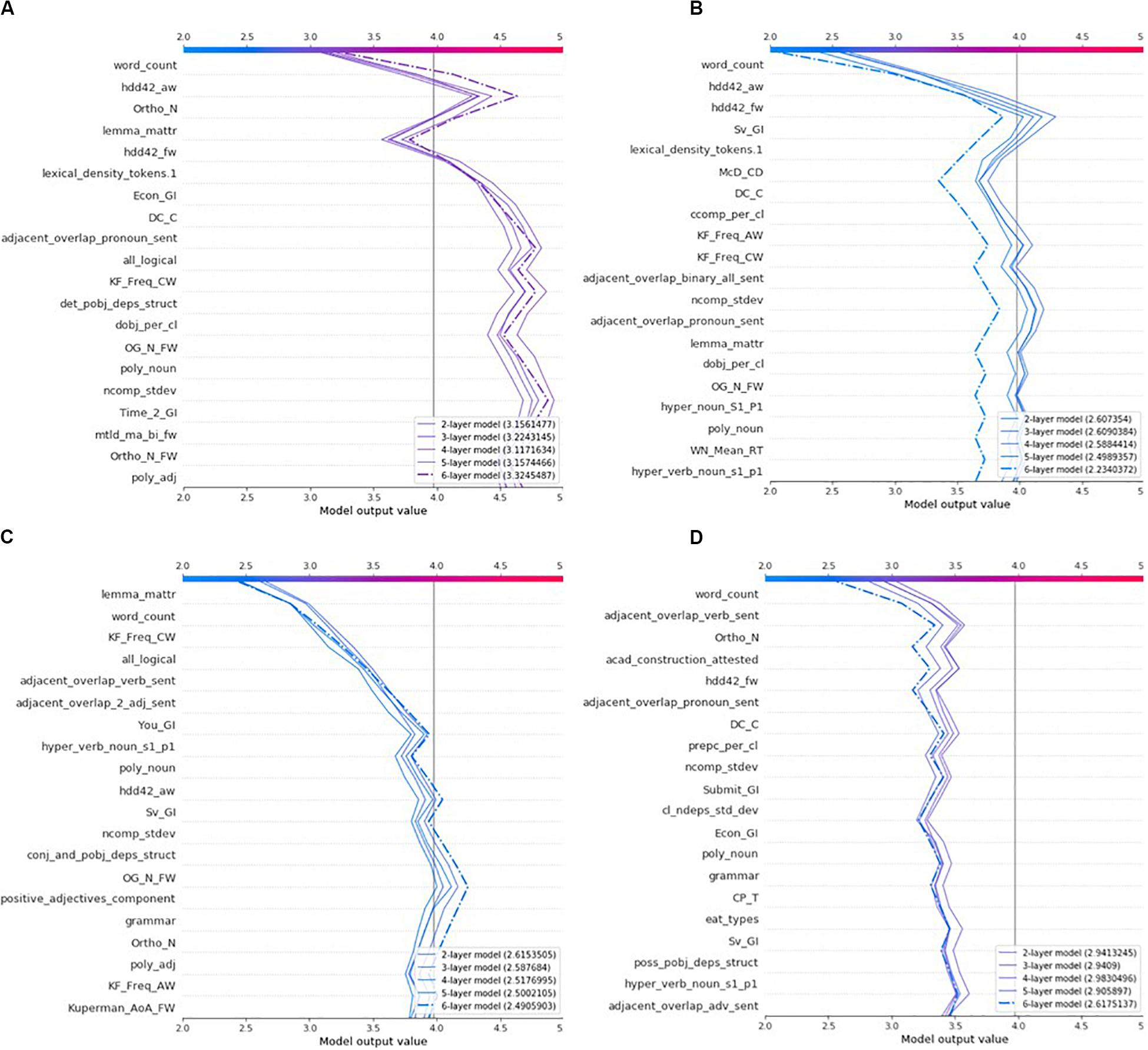
Figure 3. Comparisons of all models’ explanations of the most representative set of four essays: (A) Essay 228, (B) Essay 68, (C) Essay 219, and (D) Essay 124.
Global Explanation: The Summary Plot
It is advantageous to use SHAP to build explanation models because it provides a single framework to discover the writing features that are important to an individual essay (local) or a set of essays (global). While the decision plots list features of local importance, Figure 4 ’s summary plot ranks writing features by order of global importance (from top to bottom). All testing set’s 314 essays are represented as dots in the scatterplot of each writing feature. The position of a dot on the horizontal axis corresponds to the importance (SHAP value) of the writing feature for a specific essay and its color indicates the magnitude of the feature value in relation to the range of all 314 feature values. For example, large or small numbers of words within an essay generally contribute to increase or decrease rubric scores by up to 1.5 and 1.0, respectively. Decision plots can also be used to find the most important features for a small subset of essays; Figure 5 demonstrates the new ordering of writing indices when aggregating the feature contributions (summing the absolute values of SHAP values) of the four essays in Table 7 . Moreover, Figure 5 allows to compare the contributions of a feature to various essays. Note how the orderings in Figures 3 −5 can differ from each other, sharing many features of global importance as well as having their own unique features of local importance.
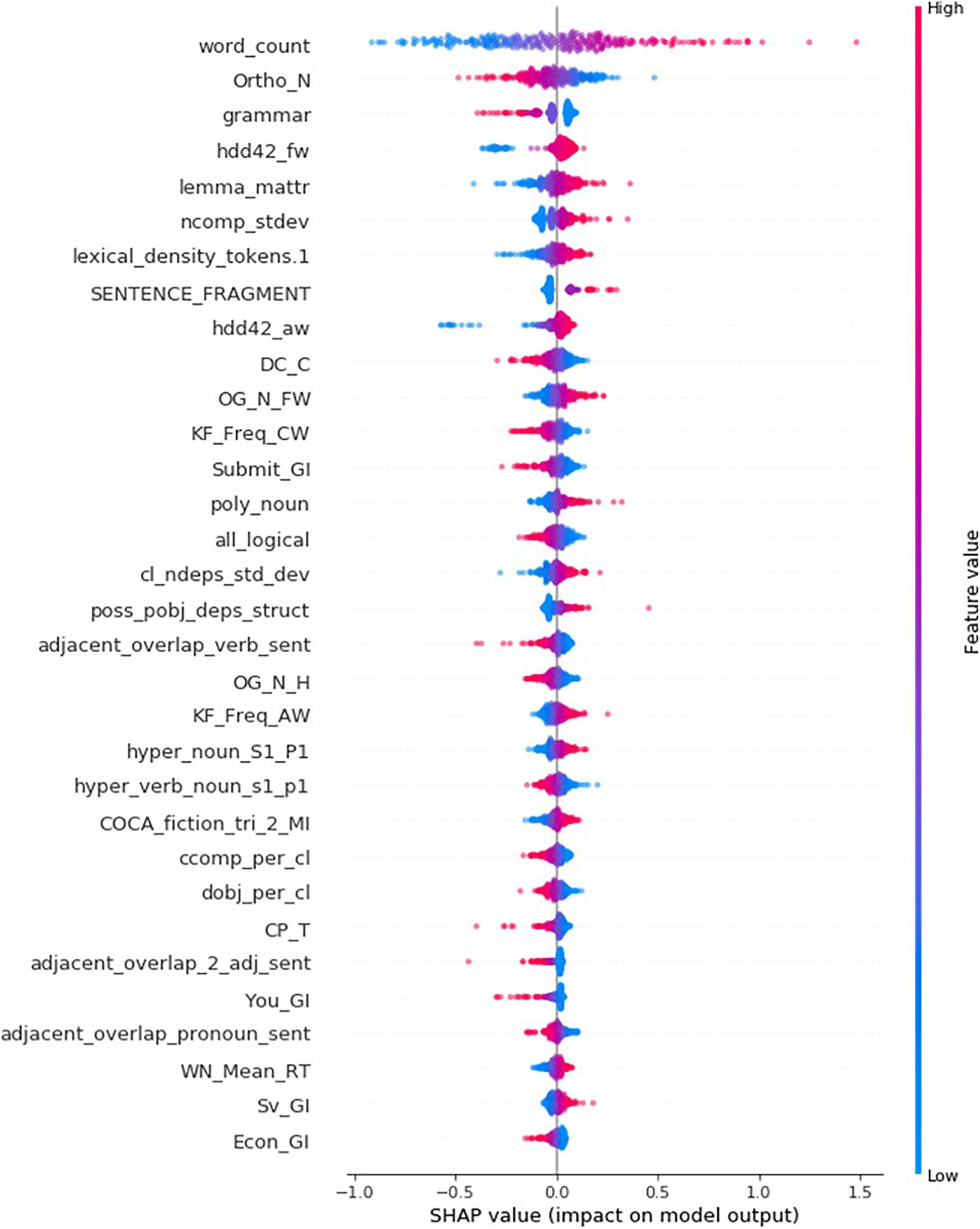
Figure 4. Summary plot listing the 32 most important features globally.
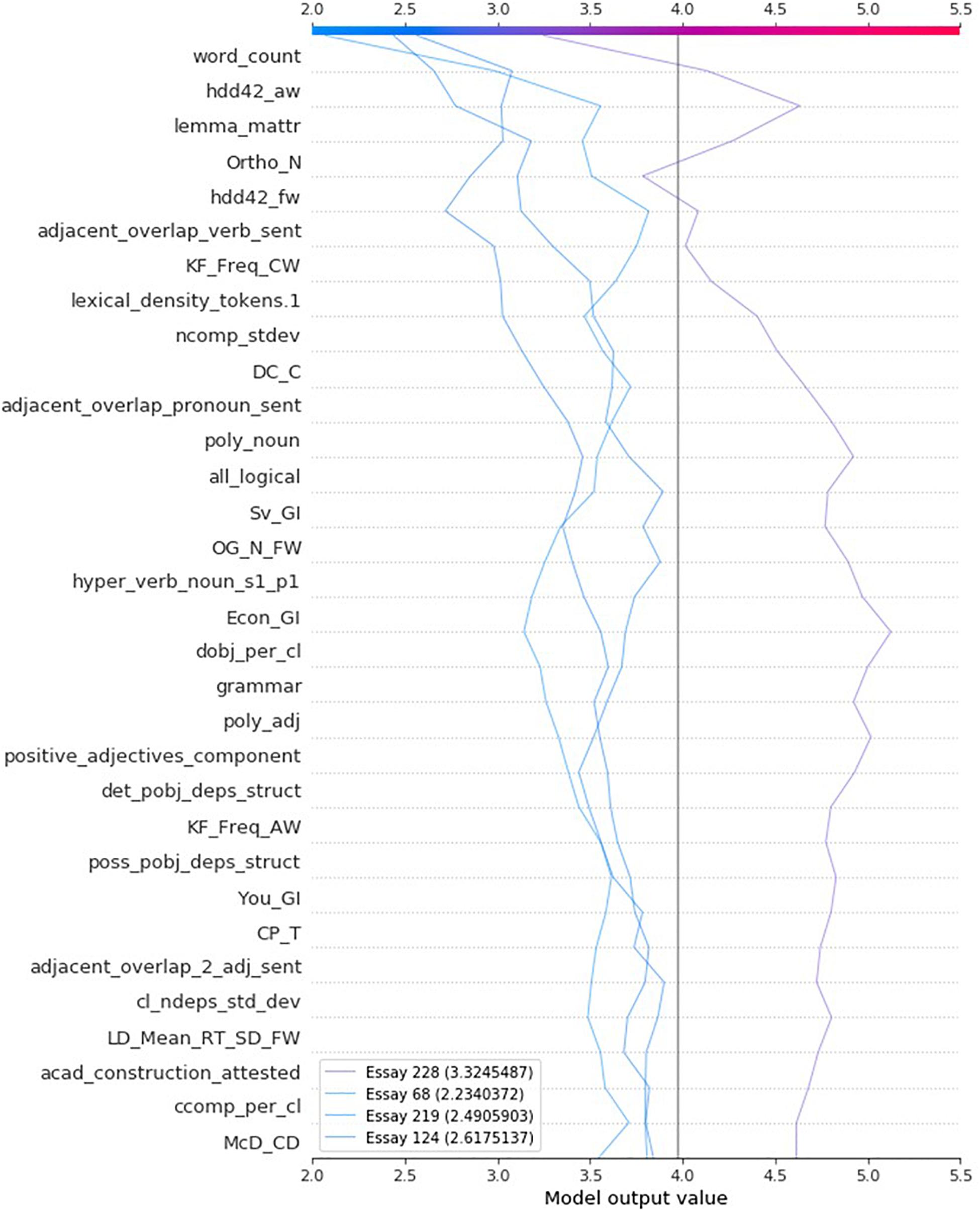
Figure 5. Decision plot delineating the best model’s explanations of Essays 228, 68, 219, and 124 (6-layer ensemble).
Definition of Important Writing Indices
The reader shall understand that it is beyond the scope of this paper to make a thorough description of all writing features. Nevertheless, the summary and decision plots in Figures 4 , 5 allow to identify a subset of features that should be examined in order to validate this study’s predictive model. Supplementary Table 1 combines and describes the 38 features in Figures 4 , 5 .
Dependence Plots
Although the summary plot in Figure 4 is insightful to determine whether small or large feature values are desirable, the dependence plots in Figure 6 prove essential to recommend whether a student should aim at increasing or decreasing the value of a specific writing feature. The dependence plots also reveal whether the student should directly act upon the targeted writing feature or indirectly on other features. The horizontal axis in each of the dependence plots in Figure 6 is the scale of the writing feature and the vertical axis is the scale of the writing feature’s contributions to the predicted rubric scores. Each dot in a dependence plot represents one of the testing set’s 314 essays, that is, the feature value and SHAP value belonging to the essay. The vertical dispersion of the dots on small intervals of the horizontal axis is indicative of interaction with other features ( Molnar, 2020 ). If the vertical dispersion is widespread (e.g., the [50, 100] horizontal-axis interval in the “word_count” dependence plot), then the contribution of the writing feature is most likely at some degree dependent on other writing feature(s).
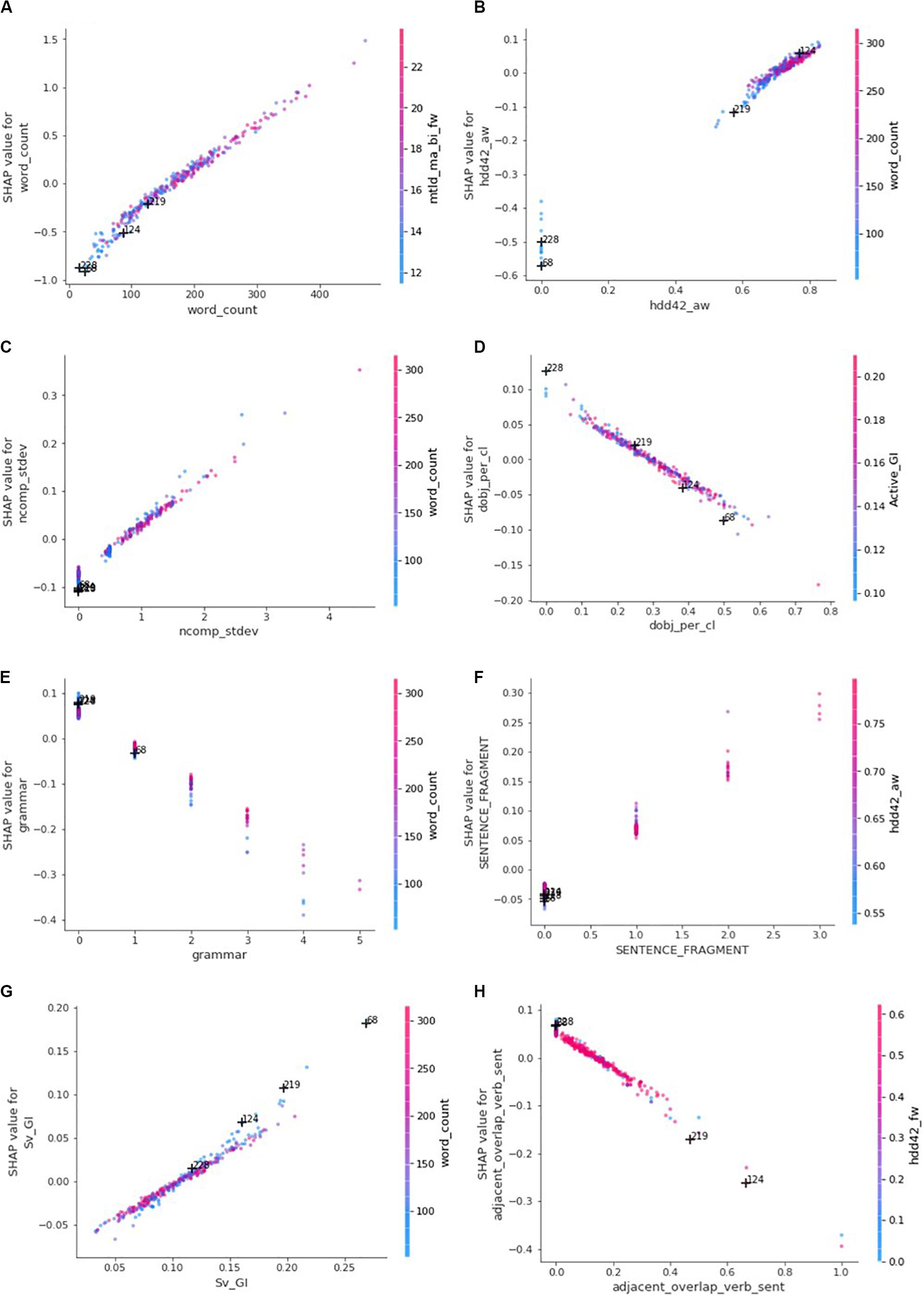
Figure 6. Dependence plots: the horizontal axes represent feature values while vertical axes represent feature contributions (SHAP values). Each dot represents one of the 314 essays of the testing set and is colored according to the value of the feature with which it interacts most strongly. (A) word_count. (B) hdd42_aw. (C) ncomp_stdev. (D) dobj_per_cl. (E) grammar. (F) SENTENCE_FRAGMENT. (G) Sv_GI. (H) adjacent_overlap_verb_sent.
The contributions of this paper can be summarized as follows: (1) it proposes a means (SHAP) to explain individual predictions of AES systems and provides flexible guidelines to build powerful predictive models using more complex algorithms such as ensembles and deep learning neural networks; (2) it applies a methodology to quantitatively assess the trustworthiness of explanation models; (3) it tests whether faster SHAP implementations impact the descriptive accuracy of explanation models, giving insight on the applicability of SHAP in real pedagogical contexts such as AES; (4) it offers a toolkit to debug AES models, highlights linguistic intricacies, and underscores the means to offer formative feedback to novice writers; and more importantly, (5) it empowers learning analytics practitioners to make AI pedagogical agents accountable to the human educator, the ultimate problem holder responsible for the decisions and actions of AI ( Abbass, 2019 ). Basically, learning analytics (which encompasses tools such as AES) is characterized as an ethics-bound, semi-autonomous, and trust-enabled human-AI fusion that recurrently measures and proactively advances knowledge boundaries in human learning.
To exemplify this, imagine an AES system that supports instructors in the detection of plagiarism, gaming behaviors, and the marking of writing activities. As previously mentioned, essays are marked according to a grid of scoring rubrics: ideas, organization, style, and conventions. While an abundance of data (e.g., the 1592 writing metrics) can be collected by the AES tool, these data might still be insufficient to automate the scoring process of certain rubrics (e.g., ideas). Nevertheless, some scoring subtasks such as assessing a student’s vocabulary, sentence fluency, and conventions might still be assigned to AI since the data types available through existing automatic linguistic analysis tools prove sufficient to reliably alleviate the human marker’s workload. Interestingly, learning analytics is key for the accountability of AI agents to the human problem holder. As the volume of writing data (through a large student population, high-frequency capture of learning episodes, and variety of big learning data) accumulate in the system, new AI agents (predictive models) may apply for the job of “automarker.” These AI agents can be quite transparent through XAI ( Arrieta et al., 2020 ) explanation models, and a human instructor may assess the suitability of an agent for the job and hire the candidate agent that comes closest to human performance. Explanations derived from these models could serve as formative feedback to the students.
The AI marker can be assigned to assess the writing activities that are similar to those previously scored by the human marker(s) from whom it learns. Dissimilar and unseen essays can be automatically assigned to the human marker for reliable scoring, and the AI agent can learn from this manual scoring. To ensure accountability, students should be allowed to appeal the AI agent’s marking to the human marker. In addition, the human marker should be empowered to monitor and validate the scoring of select writing rubrics scored by the AI marker. If the human marker does not agree with the machine scores, the writing assignments may be flagged as incorrectly scored and re-assigned to a human marker. These flagged assignments may serve to update predictive models. Moreover, among the essays that are assigned to the machine marker, a small subset can be simultaneously assigned to the human marker for continuous quality control; that is, to continue comparing whether the agreement level between human and machine markers remains within an acceptable threshold. The human marker should be at any time able to “fire” an AI marker or “hire” an AI marker from a pool of potential machine markers.
This notion of a human-AI fusion has been observed in previous AES systems where the human marker’s workload has been found to be significantly alleviated, passing from scoring several hundreds of essays to just a few dozen ( Dronen et al., 2015 ; Hellman et al., 2019 ). As the AES technology matures and as the learning analytics tools continue to penetrate the education market, this alliance of semi-autonomous human and AI agents will lead to better evidence-based/informed pedagogy ( Nelson and Campbell, 2017 ). Such a human-AI alliance can also be guided to autonomously self-regulate its own hypothesis-authoring and data-acquisition processes for purposes of measuring and advancing knowledge boundaries in human learning.
Real-Time Formative Pedagogical Feedback
This paper provides the evidence that deep learning and SHAP can be used not only to score essays automatically but also to offer explanations in real-time. More specifically, the processing time to derive the 314 explanations of the testing set’s essays has been benchmarked for several types of explainers. It was found that the faster DeepSHAP and GradientSHAP implementations, which took only a few seconds of processing, did not produce less accurate explanations than the much slower KernelSHAP. KernelSHAP took approximately 8 h of processing to derive the explanation model of a 2-layer MLP neural network predictive model and 16 h for the 6-layer predictive model.
This finding also holds for various configurations of KernelSHAP, where the number of features (16 vs. 32) shaping the explanation (where all other features are assigned zero contributions) did not produce a statistically significant difference in the reliability of the explanation models. On average, the models had a precision between 63.9 and 64.1% and a recall between 41.0 and 42.9%. This means that after perturbation of the predictive and explanation models, on average 64% of the predictions the explanation model identified as changing were accurate. On the other side, only about 42% of all predictions that changed were detected by the various 2-layer explainers. An explanation was considered as untrustworthy if the sum of its feature contributions, when added to the average prediction (base value), was not within 0.1 from the perturbated prediction. Similarly, the average precision and recall of 2-layer explainers for the 0.25-threshold were about 69% and 62%, respectively.
Impact of Deep Learning on Descriptive Accuracy of Explanations
By analyzing the performance of the various predictive models in Table 4 , no clear conclusion can be reached as to which model should be deemed as the most desirable. Despite the fact that the 6-layer models slightly outperform the other models in terms of accuracy (percentage of exact matches between the resolved [human] and predicted [machine] scores), they are not the best when it comes to the percentages of adjacent (within 1 and 2) matches. Nevertheless, if the selection of the “best” model is based on the quadratic weighted kappas, the decision remains a nebulous one to make. Moreover, ensuring that machine learning actually learned something meaningful remains paramount, especially in contexts where the performance of a majority classifier is close to the human and machine performance. For example, a majority classifier model would get 46.3% of predictions accurate ( Table 4 ), while trained predictive models at best produce accurate predictions between 51.9 and 55.1%.
Since the interpretability of a machine learning model should be prioritized over accuracy ( Ribeiro et al., 2016 ; Murdoch et al., 2019 ) for questions of transparency and trust, this paper investigated whether the impact of the depth of a MLP neural network might be more visible when assessing its interpretability, that is, the trustworthiness of its corresponding SHAP explanation model. The data in Tables 1 , 5 , 6 effectively support the hypothesis that as the depth of the neural network increases, the precision and recall of the corresponding explanation model improve. Besides, this observation is particularly interesting because the 4-layer (Grad) explainer, which has hardly more parameters than the 2-layer model, is also more accurate than the 2-layer model, suggesting that the 6-layer explainer is most likely superior to other explainers not only because of its greater number of parameters, but also because of its number of hidden layers. By increasing the number of hidden layers, it can be seen that the precision and recall of an explanation model can pass on average from approximately 64 to 73% and from 42 to 52%, respectively, for the 0.10-threshold; and for the 0.25-threshold, from 69 to 79% and from 62 to 75%, respectively.
These results imply that the descriptive accuracy of an explanation model is an evidence of effective machine learning, which may exceed the level of agreement between the human and machine graders. Moreover, given that the superiority of a trained predictive model over a majority classifier is not always obvious, the consistency of its associated explanation model demonstrates this better. Note that theoretically the SHAP explanation model of the majority classifier should assign a zero contribution to each writing feature since the average prediction of such a model is actually the most frequent rubric score given by the human raters; hence, the base value is the explanation.
An interesting fact emerges from Figure 3 , that is, all explainers (2-layer to 6-layer) are more or less similar. It appears that they do not contradict each other. More specifically, they all agree on the direction of the contributions of the most important features. In other words, they unanimously determine that a feature should increase or decrease the predicted score. However, they differ from each other on the magnitude of the feature contributions.
To conclude, this study highlights the need to train predictive models that consider the descriptive accuracy of explanations. The idea is that explanation models consider predictions to derive explanations; explanations should be considered when training predictive models. This would not only help train interpretable models the very first time but also potentially break the status quo that may exist among similar explainers to possibly produce more powerful models. In addition, this research calls for a mechanism (e.g., causal diagrams) to allow teachers to guide the training process of predictive models. Put another way, as LA practitioners debug predictive models, their insights should be encoded in a language that will be understood by the machine and that will guide the training process to avoid learning the same errors and to accelerate the training time.
Accountable AES
Now that the superiority of the 6-layer predictive and explanation models has been demonstrated, some aspects of the relevancy of explanations should be examined more deeply, knowing that having an explanation model consistent with its underlying predictive model does not guarantee relevant explanations. Table 7 discloses the set of four essays that optimize the coverage of most globally important features to evaluate the correctness of the best AES model. It is quite intriguing to note that two of the four essays are among the 16 essays that have a major disagreement (off by 2) between the resolved and predicted rubric scores (1 vs. 3 and 4 vs. 2). The AES tool clearly overrated Essay 228, while it underrated Essay 219. Naturally, these two essays offer an opportunity to understand what is wrong with the model and ultimately debug the model to improve its accuracy and interpretability.
In particular, Essay 228 raises suspicion on the positive contributions of features such as “Ortho_N,” “lemma_mattr,” “all_logical,” “det_pobj_deps_struct,” and “dobj_per_cl.” Moreover, notice how the remaining 262 less important features (not visible in the decision plot in Figure 5 ) have already inflated the rubric score beyond the base value, more than any other essay. Given the very short length and very low quality of the essay, whose meaning is seriously undermined by spelling and grammatical errors, it is of utmost importance to verify how some of these features are computed. For example, is the average number of orthographic neighbors (Ortho_N) per token computed for unmeaningful tokens such as “R” and “whe”? Similarly, are these tokens considered as types in the type-token ratio over lemmas (lemma_mattr)? Given the absence of a meaningful grammatical structure conveying a complete idea through well-articulated words, it becomes obvious that the quality of NLP (natural language processing) parsing may become a source of (measurement) bias impacting both the way some writing features are computed and the predicted rubric score. To remedy this, two solutions are proposed: (1) enhancing the dataset with the part-of-speech sequence or the structure of dependency relationships along with associated confidence levels, or (2) augmenting the essay dataset with essays enclosing various types of non-sensical content to improve the learning of these feature contributions.
Note that all four essays have a text length smaller than the average: 171 words. Notice also how the “hdd42_aw” and “hdd42_fw” play a significant role to decrease the predicted score of Essays 228 and 68. The reader should note that these metrics require a minimum of 42 tokens in order to compute a non-zero D index, a measure of lexical diversity as explained in Supplementary Table 1 . Figure 6B also shows how zero “hdd42_aw” values are heavily penalized. This is extra evidence that supports the strong role that the number of words plays in determining these rubric scores, especially for very short essays where it is one of the few observations that can be reliably recorded.
Two other issues with the best trained AES model were identified. First, in the eyes of the model, the lowest the average number of direct objects per clause (dobj_per_cl), as seen in Figure 6D , the best it is. This appears to contradict one of the requirements of the “Style” rubric, which looks for a variety of sentence structures. Remember that direct objects imply the presence of transitive verbs (action verbs) and that the balanced usage of linking verbs and action verbs as well as of transitive and intransitive verbs is key to meet the requirement of variety of sentence structures. Moreover, note that the writing feature is about counting the number of direct objects per clause, not by sentence. Only one direct object is therefore possible per clause. On the other side, a sentence may contain several clauses, which determines if the sentence is a simple, compound, or a complex sentence. This also means that a sentence may have multiple direct objects and that a high ratio of direct objects per clause is indicative of sentence complexity. Too much complexity is also undesirable. Hence, it is fair to conclude that the higher range of feature values has reasonable feature contributions (SHAP values), while the lower range does not capture well the requirements of the rubric. The dependence plot should rather display a positive peak somewhere in the middle. Notice how the poor quality of Essay 228’s single sentence prevented the proper detection of the single direct object, “broke my finger,” and the so-called absence of direct objects was one of the reasons to wrongfully improve the predicted rubric score.
The model’s second issue discussed here is the presence of sentence fragments, a type of grammatical errors. Essentially, a sentence fragment is a clause that misses one of three critical components: a subject, a verb, or a complete idea. Figure 6E shows the contribution model of grammatical errors, all types combined, while Figure 6F shows specifically the contribution model of sentence fragments. It is interesting to see how SHAP further penalizes larger numbers of grammatical errors and that it takes into account the length of the essay (red dots represent essays with larger numbers of words; blue dots represent essays with smaller numbers of words). For example, except for essays with no identified grammatical errors, longer essays are less penalized than shorter ones. This is particularly obvious when there are 2−4 grammatical errors. The model increases the predicted rubric score only when there is no grammatical error. Moreover, the model tolerates longer essays with only one grammatical error, which sounds quite reasonable. On the other side, the model finds desirable high numbers of sentence fragments, a non-trivial type of grammatical errors. Even worse, the model decreases the rubric score of essays having no sentence fragment. Although grammatical issues are beyond the scope of the “Style” rubric, the model has probably included these features because of their impact on the quality of assessment of vocabulary usage and sentence fluency. The reader should observe how the very poor quality of an essay can even prevent the detection of such fundamental grammatical errors such as in the case of Essay 228, where the AES tool did not find any grammatical error or sentence fragment. Therefore, there should be a way for AES systems to detect a minimum level of text quality before attempting to score an essay. Note that the objective of this section was not to undertake thorough debugging of the model, but rather to underscore the effectiveness of SHAP in doing so.
Formative Feedback
Once an AES model is considered reasonably valid, SHAP can be a suitable formalism to empower the machine to provide formative feedback. For instance, the explanation of Essay 124, which has been assigned a rubric score of 3 by both human and machine markers, indicates that the top two factors contributing to decreasing the predicted rubric score are: (1) the essay length being smaller than average, and (2) the average number of verb lemma types occurring at least once in the next sentence (adjacent_overlap_verb_sent). Figures 6A,H give the overall picture in which the realism of the contributions of these two features can be analyzed. More specifically, Essay 124 is one of very few essays ( Figure 6H ) that makes redundant usage of the same verbs across adjacent sentences. Moreover, the essay displays poor sentence fluency where everything is only expressed in two sentences. To understand more accurately the impact of “adjacent_overlap_verb_sent” on the prediction, a few spelling errors have been corrected and the text has been divided in four sentences instead of two. Revision 1 in Table 8 exhibits the corrections made to the original essay. The decision plot’s dashed line in Figure 3D represents the original explanation of Essay 124, while Figure 7A demonstrates the new explanation of the revised essay. It can be seen that the “adjacent_overlap_verb_sent” feature is still the second most important feature in the new explanation of Essay 124, with a feature value of 0.429, still considered as very poor according to the dependence plot in Figure 6H .
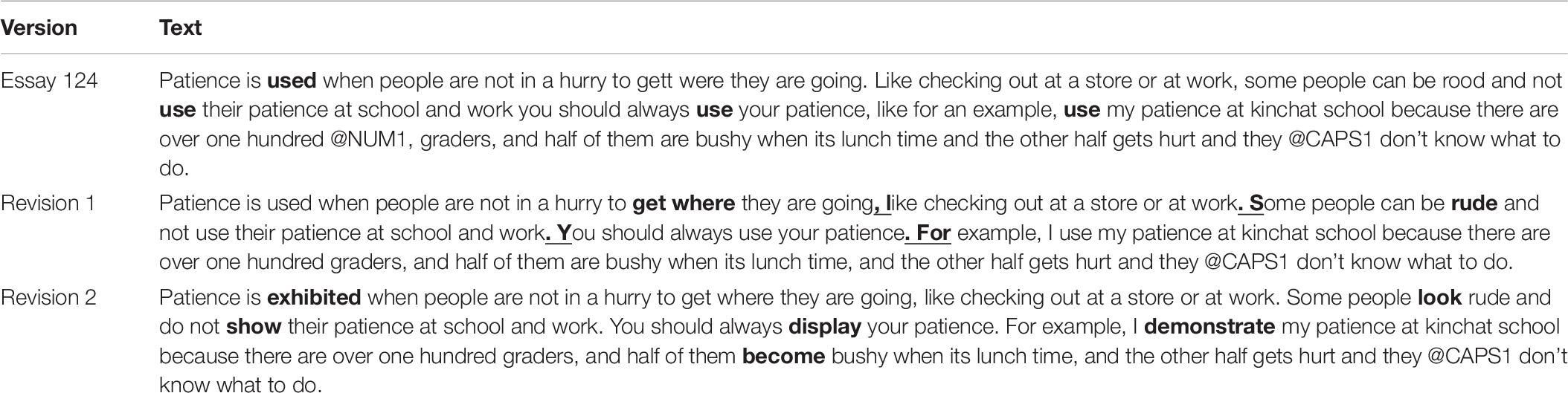
Table 8. Revisions of Essay 124: improvement of sentence splitting, correction of some spelling errors, and elimination of redundant usage of same verbs (bold for emphasis in Essay 124’s original version; corrections in bold for Revisions 1 and 2).
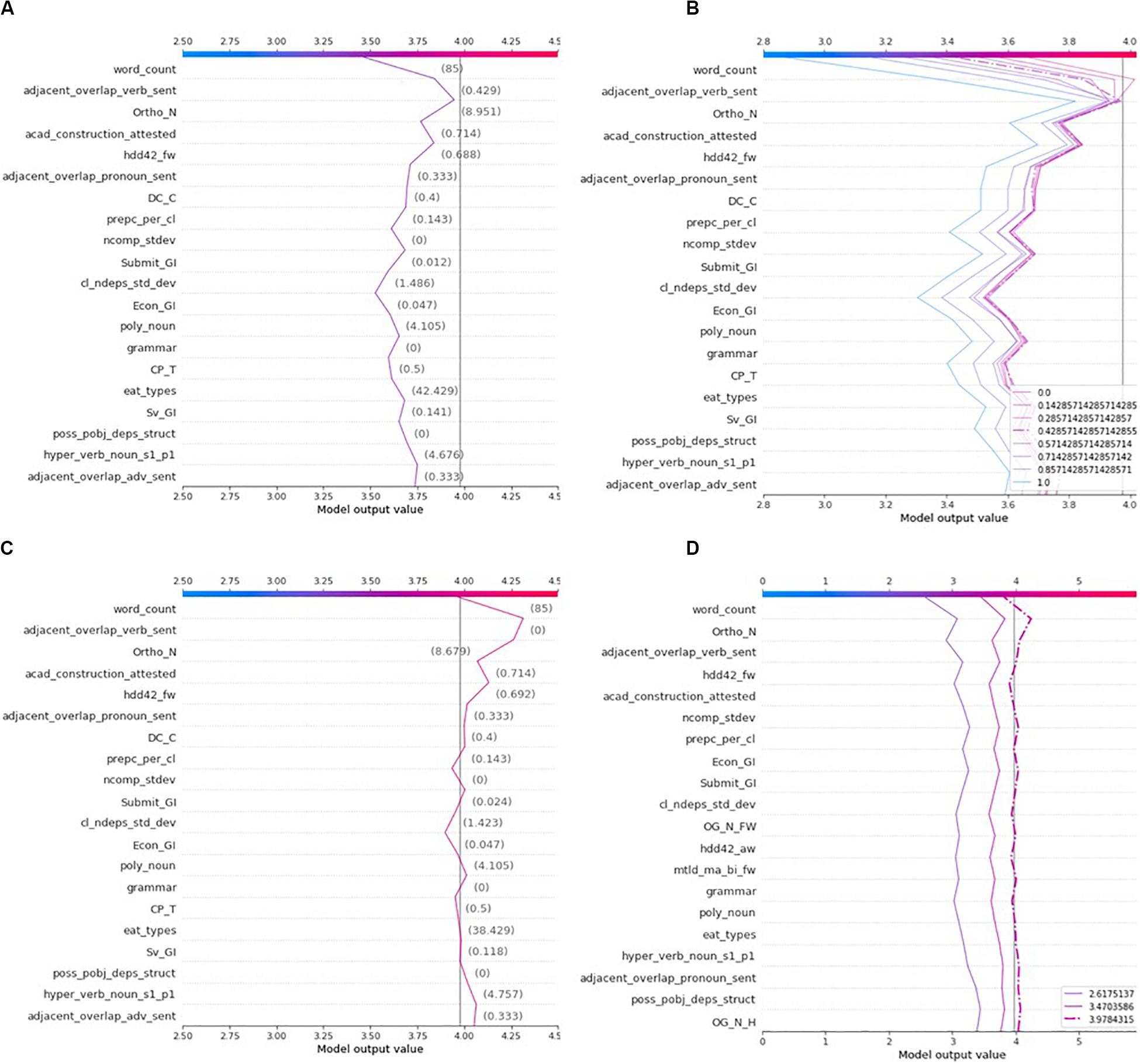
Figure 7. Explanations of the various versions of Essay 124 and evaluation of feature effect for a range of feature values. (A) Explanation of Essay 124’s first revision. (B) Forecasting the effect of changing the ‘adjacent_overlap_verb_sent’ feature on the rubric score. (C) Explanation of Essay 124’s second revision. (D) Comparison of the explanations of all Essay 124’s versions.
To show how SHAP could be leveraged to offer remedial formative feedback, the revised version of Essay 124 will be explained again for eight different values of “adjacent_overlap_verb_sent” (0, 0.143, 0.286, 0.429, 0.571, 0.714, 0.857, 1.0), while keeping the values of all other features constant. The set of these eight essays are explained by a newly trained SHAP explainer (Gradient), producing new SHAP values for each feature and each “revised” essay. Notice how the new model, called the feedback model, allows to foresee by how much a novice writer can hope to improve his/her score according to the “Style” rubric. If the student employs different verbs at every sentence, the feedback model estimates that the rubric score could be improved from 3.47 up to 3.65 ( Figure 7B ). Notice that the dashed line represents Revision 1, while other lines simulate one of the seven other altered essays. Moreover, it is important to note how changing the value of a single feature may influence the contributions that other features may have on the predicted score. Again, all explanations look similar in terms of direction, but certain features differ in terms of the magnitude of their contributions. However, the reader should observe how the targeted feature varies not only in terms of magnitude, but also of direction, allowing the student to ponder the relevancy of executing the recommended writing strategy.
Thus, upon receiving this feedback, assume that a student sets the goal to improve the effectiveness of his/her verb choice by eliminating any redundant verb, producing Revision 2 in Table 8 . The student submits his essay again to the AES system, which finally gives a new rubric score of 3.98, a significant improvement from the previous 3.47, allowing the student to get a 4 instead of a 3. Figure 7C exhibits the decision plot of Revision 2. To better observe how the various revisions of the student’s essay changed over time, their respective explanations have been plotted in the same decision plot ( Figure 7D ). Notice this time that the ordering of the features has changed to list the features of common importance to all of the essay’s versions. The feature ordering in Figures 7A−C complies with the same ordering as in Figure 3D , the decision plot of the original essay. These figures underscore the importance of tracking the interaction between the various features so that the model understands well the impact that changing one feature has on the others. TreeSHAP, an implementation for tree-based models, offers this capability and its potential on improving the quality of feedback provided to novice writers will be tested in a future version of this AES system.
This paper serves as a proof of concept of the applicability of XAI techniques in automated essay scoring, providing learning analytics practitioners and educators with a methodology on how to “hire” AI markers and make them accountable to their human counterparts. In addition to debug predictive models, SHAP explanation models can serve as some formalism of a broader learning analytics platform, where aspects of prescriptive analytics (provision of remedial formative feedback) can be added on top of the more pervasive predictive analytics.
However, the main weakness of the approach put forward in this paper consists in omitting many types of spatio-temporal data. In other words, it ignores precious information inherent to the writing process, which may prove essential to guess the intent of the student, especially in contexts of poor sentence structures and high grammatical inaccuracy. Hence, this paper calls for adapting current NLP technologies to educational purposes, where the quality of writing may be suboptimal, which is contrary to many utopian scenarios where NLP is used for content analysis, opinion mining, topic modeling, or fact extraction trained on corpora of high-quality texts. By capturing the writing process preceding a submission of an essay to an AES tool, other kinds of explanation models can also be trained to offer feedback not only from a linguistic perspective but also from a behavioral one (e.g., composing vs. revising); that is, the AES system could inform novice writers about suboptimal and optimal writing strategies (e.g., planning a revision phase after bursts of writing).
In addition, associating sections of text with suboptimal writing features, those whose contributions lower the predicted score, would be much more informative. This spatial information would not only allow to point out what is wrong and but also where it is wrong, answering more efficiently the question why an essay is wrong. This problem could be simply approached through a multiple-inputs and mixed-data feature-based (MLP) neural network architecture fed by both linguistic indices and textual data ( n -grams), where the SHAP explanation model would assign feature contributions to both types of features and any potential interaction between them. A more complex approach could address the problem through special types of recurrent neural networks such as Ordered-Neurons LSTMs (long short-term memory), which are well adapted to the parsing of natural language, and where the natural sequence of text is not only captured but also its hierarchy of constituents ( Shen et al., 2018 ). After all, this paper highlights the fact that the potential of deep learning can reach beyond the training of powerful predictive models and be better visible in the higher trustworthiness of explanation models. This paper also calls for optimizing the training of predictive models by considering the descriptive accuracy of explanations and the human expert’s qualitative knowledge (e.g., indicating the direction of feature contributions) during the training process.
Data Availability Statement
The datasets and code of this study can be found in these Open Science Framework’s online repositories: https://osf.io/fxvru/ .
Author Contributions
VK architected the concept of an ethics-bound, semi-autonomous, and trust-enabled human-AI fusion that measures and advances knowledge boundaries in human learning, which essentially defines the key traits of learning analytics. DB was responsible for its implementation in the area of explainable automated essay scoring and for the training and validation of the predictive and explanation models. Together they offer an XAI-based proof of concept of a prescriptive model that can offer real-time formative remedial feedback to novice writers. Both authors contributed to the article and approved its publication.
Research reported in this article was supported by the Academic Research Fund (ARF) publication grant of Athabasca University under award number (24087).
Conflict of Interest
The authors declare that the research was conducted in the absence of any commercial or financial relationships that could be construed as a potential conflict of interest.
Supplementary Material
The Supplementary Material for this article can be found online at: https://www.frontiersin.org/articles/10.3389/feduc.2020.572367/full#supplementary-material
- ^ https://www.kaggle.com/c/asap-aes
- ^ https://www.linguisticanalysistools.org/
Abbass, H. A. (2019). Social integration of artificial intelligence: functions, automation allocation logic and human-autonomy trust. Cogn. Comput. 11, 159–171. doi: 10.1007/s12559-018-9619-0
CrossRef Full Text | Google Scholar
Adadi, A., and Berrada, M. (2018). Peeking inside the black-box: a survey on explainable artificial intelligence (XAI). IEEE Access 6, 52138–52160. doi: 10.1109/ACCESS.2018.2870052
Amorim, E., Cançado, M., and Veloso, A. (2018). “Automated essay scoring in the presence of biased ratings,” in Proceedings of the 2018 Conference of the North American Chapter of the Association for Computational Linguistics: Human Language Technologies , New Orleans, LA, 229–237.
Google Scholar
Arrieta, A. B., Díaz-Rodríguez, N., Ser, J., Del Bennetot, A., Tabik, S., Barbado, A., et al. (2020). Explainable Artificial Intelligence (XAI): concepts, taxonomies, opportunities and challenges toward responsible AI. Inform. Fusion 58, 82–115. doi: 10.1016/j.inffus.2019.12.012
Balota, D. A., Yap, M. J., Hutchison, K. A., Cortese, M. J., Kessler, B., Loftis, B., et al. (2007). The English lexicon project. Behav. Res. Methods 39, 445–459. doi: 10.3758/BF03193014
PubMed Abstract | CrossRef Full Text | Google Scholar
Boulanger, D., and Kumar, V. (2018). “Deep learning in automated essay scoring,” in Proceedings of the International Conference of Intelligent Tutoring Systems , eds R. Nkambou, R. Azevedo, and J. Vassileva (Cham: Springer International Publishing), 294–299. doi: 10.1007/978-3-319-91464-0_30
Boulanger, D., and Kumar, V. (2019). “Shedding light on the automated essay scoring process,” in Proceedings of the International Conference on Educational Data Mining , 512–515.
Boulanger, D., and Kumar, V. (2020). “SHAPed automated essay scoring: explaining writing features’ contributions to English writing organization,” in Intelligent Tutoring Systems , eds V. Kumar and C. Troussas (Cham: Springer International Publishing), 68–78. doi: 10.1007/978-3-030-49663-0_10
Chen, H., Lundberg, S., and Lee, S.-I. (2019). Explaining models by propagating Shapley values of local components. arXiv [Preprint]. Available online at: https://arxiv.org/abs/1911.11888 (accessed September 22, 2020).
Crossley, S. A., Bradfield, F., and Bustamante, A. (2019). Using human judgments to examine the validity of automated grammar, syntax, and mechanical errors in writing. J. Writ. Res. 11, 251–270. doi: 10.17239/jowr-2019.11.02.01
Crossley, S. A., Kyle, K., and McNamara, D. S. (2016). The tool for the automatic analysis of text cohesion (TAACO): automatic assessment of local, global, and text cohesion. Behav. Res. Methods 48, 1227–1237. doi: 10.3758/s13428-015-0651-7
Crossley, S. A., Kyle, K., and McNamara, D. S. (2017). Sentiment analysis and social cognition engine (SEANCE): an automatic tool for sentiment, social cognition, and social-order analysis. Behav. Res. Methods 49, 803–821. doi: 10.3758/s13428-016-0743-z
Dronen, N., Foltz, P. W., and Habermehl, K. (2015). “Effective sampling for large-scale automated writing evaluation systems,” in Proceedings of the Second (2015) ACM Conference on Learning @ Scale , 3–10.
Goldin, I., Narciss, S., Foltz, P., and Bauer, M. (2017). New directions in formative feedback in interactive learning environments. Int. J. Artif. Intellig. Educ. 27, 385–392. doi: 10.1007/s40593-016-0135-7
Hao, Q., and Tsikerdekis, M. (2019). “How automated feedback is delivered matters: formative feedback and knowledge transfer,” in Proceedings of the 2019 IEEE Frontiers in Education Conference (FIE) , Covington, KY, 1–6.
Hellman, S., Rosenstein, M., Gorman, A., Murray, W., Becker, L., Baikadi, A., et al. (2019). “Scaling up writing in the curriculum: batch mode active learning for automated essay scoring,” in Proceedings of the Sixth (2019) ACM Conference on Learning @ Scale , (New York, NY: Association for Computing Machinery).
Hussein, M. A., Hassan, H., and Nassef, M. (2019). Automated language essay scoring systems: a literature review. PeerJ Comput. Sci. 5:e208. doi: 10.7717/peerj-cs.208
Kumar, V., and Boulanger, D. (2020). Automated essay scoring and the deep learning black box: how are rubric scores determined? Int. J. Artif. Intellig. Educ. doi: 10.1007/s40593-020-00211-5
Kumar, V., Fraser, S. N., and Boulanger, D. (2017). Discovering the predictive power of five baseline writing competences. J. Writ. Anal. 1, 176–226.
Kyle, K. (2016). Measuring Syntactic Development In L2 Writing: Fine Grained Indices Of Syntactic Complexity And Usage-Based Indices Of Syntactic Sophistication. Dissertation, Georgia State University, Atlanta, GA.
Kyle, K., Crossley, S., and Berger, C. (2018). The tool for the automatic analysis of lexical sophistication (TAALES): version 2.0. Behav. Res. Methods 50, 1030–1046. doi: 10.3758/s13428-017-0924-4
Lundberg, S. M., Erion, G. G., and Lee, S.-I. (2018). Consistent individualized feature attribution for tree ensembles. arXiv [Preprint]. Available online at: https://arxiv.org/abs/1802.03888 (accessed September 22, 2020).
Lundberg, S. M., and Lee, S.-I. (2017). “A unified approach to interpreting model predictions,” in Advances in Neural Information Processing Systems , eds I. Guyon, U. V. Luxburg, S. Bengio, H. Wallach, R. Fergus, S. Vishwanathan, et al. (Red Hook, NY: Curran Associates, Inc), 4765–4774.
Madnani, N., and Cahill, A. (2018). “Automated scoring: beyond natural language processing,” in Proceedings of the 27th International Conference on Computational Linguistics , (Santa Fe: Association for Computational Linguistics), 1099–1109.
Madnani, N., Loukina, A., von Davier, A., Burstein, J., and Cahill, A. (2017). “Building better open-source tools to support fairness in automated scoring,” in Proceedings of the First (ACL) Workshop on Ethics in Natural Language Processing , (Valencia: Association for Computational Linguistics), 41–52.
McCarthy, P. M., and Jarvis, S. (2010). MTLD, vocd-D, and HD-D: a validation study of sophisticated approaches to lexical diversity assessment. Behav. Res. Methods 42, 381–392. doi: 10.3758/brm.42.2.381
Mizumoto, T., Ouchi, H., Isobe, Y., Reisert, P., Nagata, R., Sekine, S., et al. (2019). “Analytic score prediction and justification identification in automated short answer scoring,” in Proceedings of the Fourteenth Workshop on Innovative Use of NLP for Building Educational Applications , Florence, 316–325.
Molnar, C. (2020). Interpretable Machine Learning . Abu Dhabi: Lulu
Murdoch, W. J., Singh, C., Kumbier, K., Abbasi-Asl, R., and Yu, B. (2019). Definitions, methods, and applications in interpretable machine learning. Proc. Natl. Acad. Sci. U.S.A. 116, 22071–22080. doi: 10.1073/pnas.1900654116
Nelson, J., and Campbell, C. (2017). Evidence-informed practice in education: meanings and applications. Educ. Res. 59, 127–135. doi: 10.1080/00131881.2017.1314115
Rahimi, Z., Litman, D., Correnti, R., Wang, E., and Matsumura, L. C. (2017). Assessing students’ use of evidence and organization in response-to-text writing: using natural language processing for rubric-based automated scoring. Int. J. Artif. Intellig. Educ. 27, 694–728. doi: 10.1007/s40593-017-0143-2
Reinertsen, N. (2018). Why can’t it mark this one? A qualitative analysis of student writing rejected by an automated essay scoring system. English Austral. 53:52.
Ribeiro, M. T., Singh, S., and Guestrin, C. (2016). “Why should i trust you?”: explaining the predictions of any classifier. CoRR, abs/1602.0. arXiv [Preprint]. Available online at: http://arxiv.org/abs/1602.04938 (accessed September 22, 2020).
Rupp, A. A. (2018). Designing, evaluating, and deploying automated scoring systems with validity in mind: methodological design decisions. Appl. Meas. Educ. 31, 191–214. doi: 10.1080/08957347.2018.1464448
Rupp, A. A., Casabianca, J. M., Krüger, M., Keller, S., and Köller, O. (2019). Automated essay scoring at scale: a case study in Switzerland and Germany. ETS Res. Rep. Ser. 2019, 1–23. doi: 10.1002/ets2.12249
Shen, Y., Tan, S., Sordoni, A., and Courville, A. C. (2018). Ordered Neurons: Integrating Tree Structures into Recurrent Neural Networks. CoRR, abs/1810.0. arXiv [Preprint]. Available online at: http://arxiv.org/abs/1810.09536 (accessed September 22, 2020).
Shermis, M. D. (2014). State-of-the-art automated essay scoring: competition, results, and future directions from a United States demonstration. Assess. Writ. 20, 53–76. doi: 10.1016/j.asw.2013.04.001
Taghipour, K. (2017). Robust Trait-Specific Essay Scoring using Neural Networks and Density Estimators. Dissertation, National University of Singapore, Singapore.
West-Smith, P., Butler, S., and Mayfield, E. (2018). “Trustworthy automated essay scoring without explicit construct validity,” in Proceedings of the 2018 AAAI Spring Symposium Series , (New York, NY: ACM).
Woods, B., Adamson, D., Miel, S., and Mayfield, E. (2017). “Formative essay feedback using predictive scoring models,” in Proceedings of the 23rd ACM SIGKDD International Conference on Knowledge Discovery and Data Mining , (New York, NY: ACM), 2071–2080.
Keywords : explainable artificial intelligence, SHAP, automated essay scoring, deep learning, trust, learning analytics, feedback, rubric
Citation: Kumar V and Boulanger D (2020) Explainable Automated Essay Scoring: Deep Learning Really Has Pedagogical Value. Front. Educ. 5:572367. doi: 10.3389/feduc.2020.572367
Received: 14 June 2020; Accepted: 09 September 2020; Published: 06 October 2020.
Reviewed by:
Copyright © 2020 Kumar and Boulanger. This is an open-access article distributed under the terms of the Creative Commons Attribution License (CC BY) . The use, distribution or reproduction in other forums is permitted, provided the original author(s) and the copyright owner(s) are credited and that the original publication in this journal is cited, in accordance with accepted academic practice. No use, distribution or reproduction is permitted which does not comply with these terms.
*Correspondence: David Boulanger, [email protected]
Disclaimer: All claims expressed in this article are solely those of the authors and do not necessarily represent those of their affiliated organizations, or those of the publisher, the editors and the reviewers. Any product that may be evaluated in this article or claim that may be made by its manufacturer is not guaranteed or endorsed by the publisher.
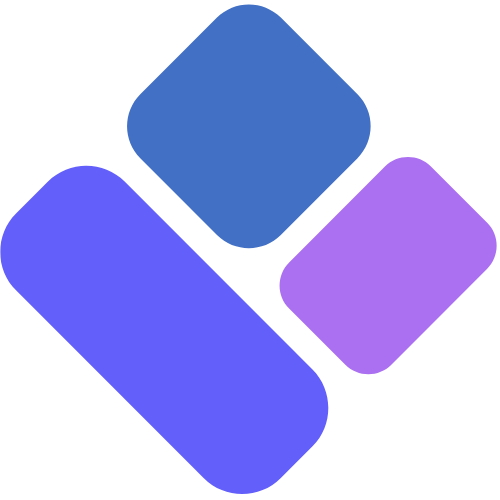
minutes minute
seconds second
Revolutionizing Assessment: AI’s Automated Grading & Feedback – Unlocking Efficiency, Objectivity, and Personalized Learning
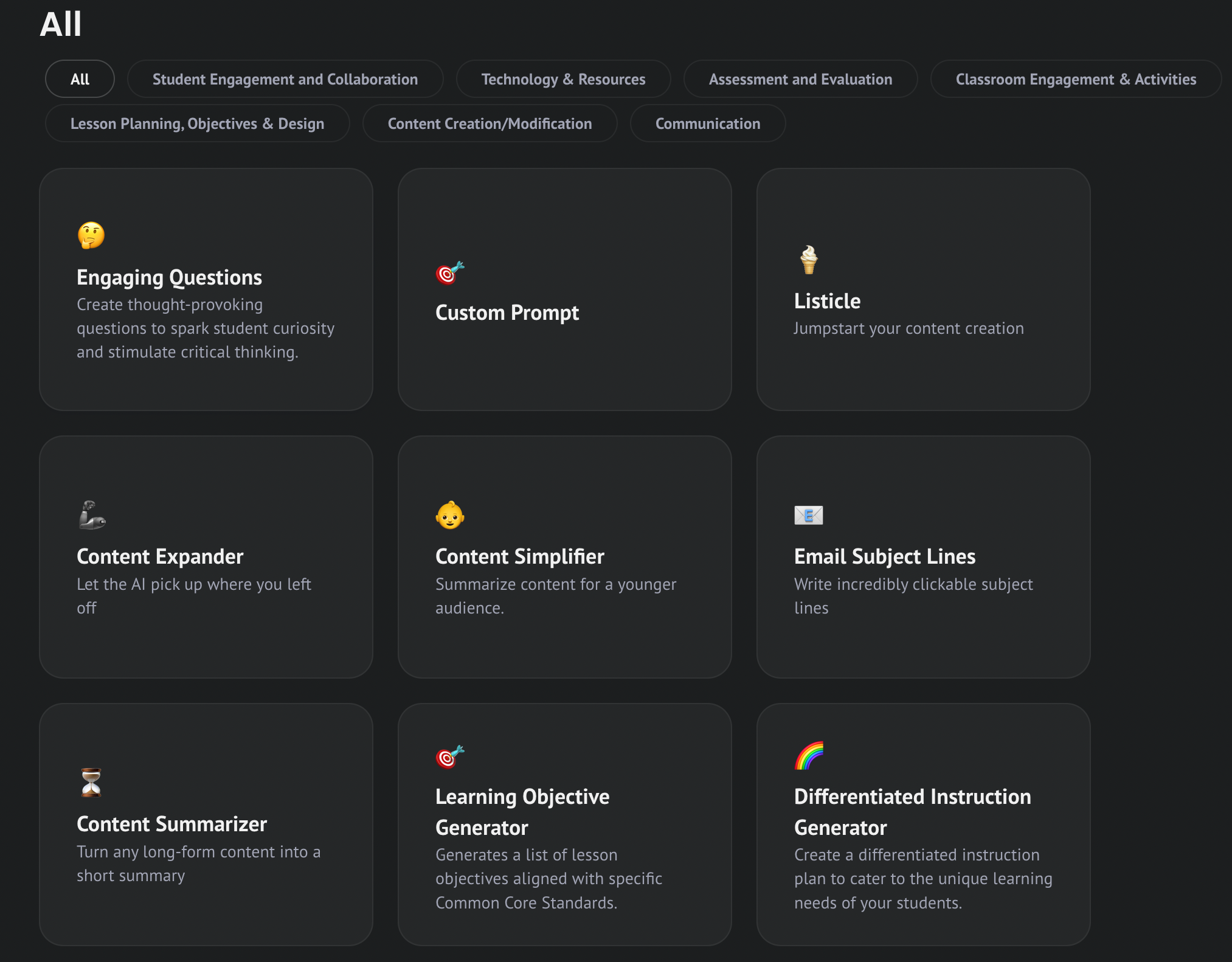
- Date: 11/23/2022
Welcome to our blog post on revolutionizing assessment through AI’s automated grading and feedback. In today’s rapidly evolving digital era, technology has permeated every aspect of our lives, including education. With the advent of artificial intelligence (AI), assessment methods have undergone a transformation, unlocking efficiency, objectivity, and personalized learning like never before.
In this blog post, we will delve into the world of AI in assessment, specifically focusing on automated grading and feedback. We will begin by providing a comprehensive definition and overview of AI in assessment, highlighting its significance and the benefits it brings to the table.
Additionally, we will explore the history and evolution of AI in assessment, shedding light on how this technology has evolved over time. Furthermore, we will introduce you to major platforms and tools that utilize AI-based grading and feedback systems, showcasing the wide range of options available to educators and institutions.
Moving on, we will dive into the process of automated grading, outlining how it works and the different types of assessments suitable for this approach. We will discuss the role of machine learning algorithms, their contribution to accurate grading, and the reliability of automated grading systems. To provide a comprehensive understanding, we will present case studies and examples of successful implementation.
Next, we will shift our focus to AI-powered feedback generation. Feedback plays a crucial role in the learning process, and AI-generated feedback has the potential to revolutionize the way students receive guidance. We will explore the techniques and methodologies used for generating feedback, emphasizing the benefits of customization and personalization. However, we will also address the ethical considerations and challenges associated with AI-generated feedback.
In the following section, we will examine the advantages and challenges of using AI in assessment. We will explore the efficiency and time-saving benefits, the consistency and objectivity in grading, and the enhanced quality and quantity of feedback that AI brings to the table. However, we will also discuss the challenges, such as bias and fairness concerns, complex and subjective assessments, technical limitations, and the impact on human grading and the role of educators.
Finally, we will discuss future trends and implications of AI in assessment. We will explore emerging trends and advancements in AI-based assessment, as well as their potential impact on educational institutions and educators. Ethical considerations and guidelines for AI in assessment will be explored, along with a discussion on the future of assessment and the role of AI.
In conclusion, this blog post aims to provide a comprehensive understanding of AI’s automated grading and feedback in revolutionizing assessment. We will explore the benefits, challenges, and future implications of this technology, ultimately highlighting its potential to unlock efficiency, objectivity, and personalized learning in the educational landscape. So, let’s embark on this exciting journey to discover the transformative power of AI in assessment.
AI in Assessment: Automated Grading and Feedback
Artificial intelligence (AI) has emerged as a game-changer in various industries, and education is no exception. In recent years, the integration of AI in assessment has revolutionized the way we evaluate and provide feedback to learners. Automated grading and feedback systems powered by AI offer a host of benefits, from increased efficiency to enhanced objectivity and personalized learning experiences.
Introduction to AI in Assessment
AI in assessment refers to the utilization of artificial intelligence technologies to automate the grading and feedback generation processes in educational settings. This innovative approach replaces or assists human evaluators by leveraging machine learning algorithms, natural language processing, and data analysis techniques. The goal is to streamline assessment procedures, provide timely feedback, and enhance the overall learning experience for students.
Importance and Benefits of Automated Grading and Feedback
Automated grading and feedback have gained significant importance due to the growing need for efficient and effective assessment processes in education. Traditionally, manual grading was time-consuming and prone to subjective biases. With AI, educators can now save valuable time and resources by automating the grading process, enabling them to focus more on instructional activities.
One of the key advantages of automated grading is its ability to provide consistent and objective evaluations. Unlike human graders who may be influenced by personal biases or fatigue, AI-based systems evaluate assignments based on predefined criteria, ensuring fairness and impartiality. This objectivity helps establish a standardized assessment process for all learners, promoting transparency and equity in education.
Furthermore, automated grading and feedback systems generate immediate and personalized feedback for students. Timely feedback is crucial for learners to identify their strengths and areas for improvement. AI algorithms analyze student responses and provide detailed feedback, enabling learners to understand their mistakes, clarify misconceptions, and make necessary adjustments to enhance their learning outcomes.
Brief History and Evolution of AI in Assessment
The use of AI in assessment has a rich history that dates back several decades. Early attempts at automated grading can be traced back to the 1960s when computer-based assessments were first introduced. However, the limited computing power and lack of sophisticated algorithms hindered their widespread adoption.
Over the years, advancements in AI technologies, particularly in machine learning and natural language processing, have propelled the development of more robust automated grading and feedback systems. Researchers and educators have explored various approaches, such as rule-based systems, statistical modeling, and neural networks, to improve the accuracy and reliability of automated assessment.
Today, AI-based assessment platforms have become increasingly sophisticated, leveraging big data analytics and predictive modeling to provide comprehensive insights into student performance. These platforms are continuously evolving, integrating advanced techniques like deep learning and adaptive algorithms to enhance their capabilities further.
Introduction to Major Platforms and Tools in AI-based Grading and Feedback
A variety of platforms and tools have emerged in the market, offering AI-based grading and feedback solutions for educators and institutions. These platforms harness the power of machine learning and natural language processing algorithms to automate the grading process and generate personalized feedback.
One prominent platform in this space is Turnitin, which combines plagiarism detection with automated grading capabilities. It utilizes AI algorithms to assess the originality and quality of students’ written assignments, providing educators with an efficient and reliable grading system. Turnitin’s feedback studio enables instructors to deliver personalized feedback, enhancing the learning experience for students.
Another notable platform is Gradescope, which simplifies the grading workflow for educators. It enables instructors to create assignments, automatically grade them using AI, and provide detailed feedback to students. Gradescope’s machine learning models can accurately evaluate handwritten responses, making it suitable for subjects that require mathematical equations or diagrams.
Additionally, there are open-source tools like Open edX and Moodle that offer AI-based assessment features. These platforms provide educators with the flexibility to customize assessments and leverage AI algorithms to automate grading and generate feedback. Open edX incorporates AI-powered tools like Open Response Assessment to evaluate subjective responses, while Moodle integrates plugins like the Feedback Grading Rubric to facilitate automated grading.
These major platforms and tools are just a glimpse of the vast landscape of AI-based grading and feedback systems available to educators. Their diverse functionalities cater to different educational needs, empowering institutions to adopt automated assessment solutions that align with their specific requirements.
The Process of Automated Grading
Automated grading is a complex process that involves the use of AI algorithms to evaluate student assessments, such as exams, quizzes, essays, and coding assignments. Understanding the underlying process is crucial to grasp the mechanics and potential of AI in assessment.
Understanding the Automated Grading Process
The automated grading process typically consists of several steps, starting with the submission of student assessments through a digital platform or learning management system. Once the assessments are received, AI algorithms take over and analyze the content using predefined criteria and rules.
Machine learning algorithms play a pivotal role in automated grading. These algorithms are trained using large datasets that include both correct and incorrect solutions, essays, or programming codes. By analyzing these datasets, the algorithms learn patterns and establish a baseline for evaluating future assessments.
During the evaluation phase, the AI algorithms compare the student’s responses with the learned patterns and criteria. The algorithms consider various factors such as grammar, vocabulary, syntax, logic, and subject-specific knowledge to determine the quality and correctness of the assessment. This process allows for a comprehensive evaluation that goes beyond simple keyword matching.
The output of the automated grading process is a numerical score or a qualitative assessment of the student’s performance. Depending on the platform or tool used, the automated grading system may also generate detailed feedback to help students understand their strengths and weaknesses.
Types of Assessments Suitable for Automated Grading
While automated grading is not suitable for all types of assessments, it can be effectively applied to certain formats. Objective assessments, such as multiple-choice questions, true/false questions, and fill-in-the-blank exercises, are well-suited for automated grading. These types of assessments have clear and unambiguous answers that can be easily evaluated by AI algorithms.
Subjective assessments, such as essays, short-answer questions, and coding assignments, pose a greater challenge for automated grading due to their subjective nature and open-ended responses. However, advancements in natural language processing and machine learning techniques have improved the accuracy and reliability of automated grading for subjective assessments as well.
For essays, AI algorithms analyze factors such as grammar, sentence structure, coherence, and content relevance to assign a score. Some platforms even incorporate sentiment analysis to gauge the overall tone and quality of the essay. Similarly, for coding assignments, algorithms evaluate the correctness of the code, adherence to coding standards, and efficiency of the solution.
It’s important to note that while automated grading can handle a significant portion of assessments, there may still be a need for human intervention in certain cases. Complex assessments that require subjective judgment, creativity, or critical thinking may still require the expertise of human graders to provide a comprehensive evaluation.
The Role of Machine Learning Algorithms in Automated Grading
Machine learning algorithms are at the heart of automated grading systems. These algorithms are trained using large datasets that contain both correctly and incorrectly assessed assignments. The training process allows the algorithms to learn patterns, identify common errors, and establish a baseline for evaluating future assessments.
One common approach in machine learning-based grading systems is supervised learning, where algorithms are trained on labeled datasets. Human graders assess a subset of student assignments and provide the correct scores or qualitative assessments. The algorithm then learns from these labeled examples and generalizes the knowledge to evaluate new assignments.
Another approach is unsupervised learning, where algorithms analyze unlabelled datasets to discover patterns and similarities in student assessments. This approach is particularly useful for assessments without predefined correct answers, such as essays or open-ended questions. Unsupervised learning algorithms can identify common themes, evaluate the coherence of arguments, and provide feedback based on the identified patterns.
In recent years, deep learning algorithms, particularly neural networks, have gained popularity in automated grading systems. Deep learning models can process large amounts of data, extract complex features, and make sophisticated judgments. These models have shown promising results in assessing subjective assignments, such as essays or creative writing.
The use of machine learning algorithms in automated grading facilitates scalability, as the algorithms can handle large volumes of assessments efficiently. Moreover, these algorithms can adapt and improve over time as they continue to learn from new examples and feedback provided by human graders.
Accuracy and Reliability of Automated Grading
The accuracy and reliability of automated grading systems have been a subject of extensive research and discussion. While these systems have made significant advancements, they are not without limitations. The performance of automated grading depends on various factors, including the quality of training data, the complexity of the assessment, and the algorithms employed.
In objective assessments, such as multiple-choice questions, automated grading systems can achieve high levels of accuracy. The algorithms can precisely match student responses with the correct answers, ensuring consistent and objective evaluations. However, errors can still occur due to ambiguous questions or incorrect answer keys.
Subjective assessments pose a greater challenge for automated grading systems. Evaluating essays, for example, involves assessing the structure, coherence, grammar, and content relevance. While AI algorithms have improved in analyzing these aspects, they may still struggle with understanding nuanced arguments, creativity, or cultural context, which can impact the accuracy of the evaluation.
To address these challenges, automated grading systems often incorporate human-in-the-loop mechanisms. In hybrid systems, human graders review a subset of assignments, providing a benchmark for the automated grading system. The system then compares its evaluations with those of human graders, allowing for continuous improvement and calibration.
Continuous research and development efforts are focused on improving the accuracy and reliability of automated grading systems. Ongoing advancements in natural language processing, machine learning, and deep learning techniques hold promise for further enhancing the capabilities of automated grading and ensuring consistent and fair assessments.
Case Studies and Examples Showcasing Successful Implementation
Numerous case studies and examples highlight the successful implementation of automated grading systems across different educational levels and disciplines. These real-world applications demonstrate the benefits, challenges, and potential of AI in assessment.
One notable example is the Automated Student Assessment Prize (ASAP), organized by the Hewlett Foundation. The competition invited participants to develop automated grading systems for essays in high-stakes exams. The winning solutions demonstrated high accuracy and reliability, comparable to human graders, showcasing the potential of AI in large-scale assessments.
In higher education, several universities and institutions have adopted automated grading systems to streamline assessment processes and improve feedback delivery. The University of Michigan, for instance, implemented the ECoach system, which utilizes AI to provide personalized feedback to students on their writing assignments. The system’s algorithm analyzes student responses and generates tailored feedback, helping learners improve their writing skills.
Automated grading systems have also found success in coding and programming assignments. Platforms like CodeSignal and HackerRank employ AI algorithms to evaluate students’ coding skills, providing detailed assessments and feedback. These systems not only save time for educators but also offer a standardized and objective evaluation of programming abilities.
Furthermore, the use of automated grading systems has expanded beyond traditional academic settings. Massive Open Online Courses (MOOCs) and online learning platforms have embraced automated grading as a means to handle the large number of assessments from global learners. Platforms like Coursera and edX utilize AI algorithms to evaluate assessments from thousands of students simultaneously, ensuring timely feedback and assessment results.
These case studies and examples highlight the successful adoption of automated grading systems across diverse educational contexts. They demonstrate the potential of AI in assessment to improve efficiency, objectivity, and feedback delivery, ultimately enhancing the learning experience for students.
AI-powered Feedback Generation
Feedback is a critical component of the learning process, providing students with valuable insights into their performance and guiding them towards improvement. AI-powered feedback generation takes this process to the next level by leveraging advanced algorithms to provide personalized and meaningful feedback to learners.
Importance of Feedback in the Learning Process
Feedback is an integral part of effective learning. It helps students identify their strengths, weaknesses, and areas for improvement. Timely and constructive feedback not only boosts motivation but also enables students to make adjustments, refine their understanding, and enhance their learning outcomes.
Traditionally, providing personalized feedback to each student has been a time-consuming task for educators, especially in large classes. However, AI-based feedback generation offers a scalable solution, enabling educators to deliver tailored feedback on a larger scale.
Overview of AI-generated Feedback and its Potential Benefits
AI-generated feedback refers to the use of artificial intelligence algorithms to analyze student assessments and generate customized feedback. These algorithms can evaluate various aspects of student work, such as content accuracy, coherence, organization, language proficiency, and critical thinking skills.
One of the significant advantages of AI-generated feedback is its ability to provide immediate and consistent feedback. Unlike human graders who may experience variations in timing and availability, AI algorithms can process assessments quickly and deliver instant feedback. This immediate feedback empowers students to reflect on their performance and take corrective actions promptly.
In addition to timing, AI-generated feedback offers consistency and objectivity. The algorithms evaluate assessments based on predefined criteria, ensuring that all students receive fair and unbiased feedback. This consistency helps establish a standardized assessment process, where all students are evaluated on the same parameters, regardless of their location or the availability of human graders.
Moreover, AI-generated feedback can be highly personalized. By analyzing student responses and patterns, AI algorithms can identify specific areas of improvement for each learner. This personalized feedback enables students to focus on their individual learning needs and make targeted efforts to enhance their skills and knowledge.
Techniques and Methodologies Used for Generating Feedback
AI-generated feedback relies on a variety of techniques and methodologies to analyze student assessments and generate meaningful feedback. Natural language processing (NLP) plays a crucial role in understanding and interpreting the content of written responses.
NLP techniques, such as part-of-speech tagging, syntactic parsing, and sentiment analysis, enable algorithms to analyze the structure, grammar, coherence, and overall quality of written responses. These techniques help identify specific areas where students excel and areas that require improvement, allowing for targeted feedback.
Machine learning algorithms also play a significant role in feedback generation. Supervised learning algorithms, trained on annotated datasets, can identify common errors, misconceptions, and areas of improvement in student work. By leveraging these algorithms, AI-generated feedback can provide detailed suggestions, point out specific errors, and offer alternative approaches to problem-solving.
Additionally, natural language generation (NLG) techniques enable AI systems to generate feedback in a human-readable format. NLG algorithms use predefined templates, rules, and linguistic rules to construct coherent and contextually appropriate feedback sentences. This ensures that the generated feedback is not only accurate but also easily understandable by students.
Customization and Personalization of Feedback
One of the key advantages of AI-generated feedback is its ability to be customized and personalized. AI algorithms can adapt to individual student needs, considering their unique learning styles, strengths, and weaknesses.
Customization involves tailoring feedback based on the specific requirements of an assessment or subject. For example, in a mathematics assignment, AI algorithms can provide detailed explanations of incorrect calculations or suggest alternative problem-solving methods. In an English essay, the algorithms can focus on aspects such as grammar, vocabulary, and logical coherence.
Personalization takes customization a step further by considering individual student profiles and learning histories. AI algorithms can analyze a student’s prior work, performance patterns, and learning objectives to provide feedback that aligns with their specific needs. This personalized feedback helps students understand their progress, identify recurring mistakes, and set specific goals for improvement.
The customization and personalization of feedback contribute to a more individualized and adaptive learning experience. By addressing students’ specific needs and providing targeted guidance, AI-generated feedback fuels their motivation, enhances their engagement, and facilitates self-directed learning.
Ethical Considerations and Challenges of AI-generated Feedback
While AI-generated feedback offers numerous benefits, it also raises ethical considerations and challenges that need to be addressed. One significant concern is the potential for bias in AI algorithms. If the training data used to develop these algorithms are biased, the generated feedback may perpetuate existing biases or reinforce stereotypes. It is crucial to ensure that the training datasets are diverse, inclusive, and representative of the student population to avoid such biases.
Another challenge lies in maintaining a balance between automation and human involvement in the feedback process. While AI algorithms can analyze and generate feedback at scale, human input is essential to provide nuanced and context-specific guidance. Educators should play an active role in reviewing and supplementing AI-generated feedback to ensure its accuracy and relevance.
Furthermore, the ethical use of AI-generated feedback involves transparency and informed consent. Students should be aware that their assessments are being evaluated by AI algorithms and should have the opportunity to understand how their data is used. Institutions and educators must ensure clear communication about the purpose, limitations, and implications of AI-generated feedback and obtain appropriate consent from students.
Addressing these ethical considerations and challenges requires ongoing research, collaboration between educators and technologists, and the development of ethical guidelines and best practices for AI-generated feedback in education.
Advantages and Challenges of AI in Assessment
The integration of AI in assessment brings forth a plethora of advantages that have the potential to transform the educational landscape. However, alongside these advantages, there are also challenges that need to be addressed to ensure the effective and ethical implementation of AI in assessment.
Advantages of Using AI in Assessment
Efficiency and Time-saving Benefits: Automated grading and feedback systems powered by AI significantly reduce the time and effort required for manual grading. Educators can focus more on providing personalized instruction, engaging with students, and addressing individual learning needs.
Consistency and Objectivity in Grading: AI-based assessment systems offer consistent and objective evaluations by adhering to predefined criteria. This eliminates subjective biases that may arise from human grading and ensures fairness in the assessment process.
Enhanced Feedback Quality and Quantity: AI-generated feedback can provide detailed and constructive insights into student performance. With the ability to analyze multiple aspects of assessments, AI algorithms can offer personalized feedback that goes beyond what is feasible with manual grading.
Scalability and Handling Large Volumes of Assessments: AI-powered assessment systems can handle vast quantities of assessments, making them ideal for massive open online courses (MOOCs) and online learning platforms. This scalability enables educators to provide timely feedback to a large number of students, regardless of class size.
Challenges and Limitations of AI in Assessment
Bias and Fairness Concerns: AI algorithms are only as unbiased as the data they are trained on. If training datasets are biased or lack diversity, AI-generated grading and feedback may perpetuate existing biases or disadvantage certain groups of students. It is crucial to continually monitor and address these biases to ensure fair and equitable assessment practices.
Complex and Subjective Assessments: While AI-powered systems have made significant progress in evaluating subjective assessments, such as essays and open-ended questions, there are inherent challenges in assessing creativity, critical thinking, and nuanced arguments. Human judgment and expertise may still be required to evaluate these complex aspects accurately.
Technical Limitations and Implementation Challenges: Implementing AI-based assessment systems requires robust technical infrastructure, including powerful computing resources, secure data storage, and efficient algorithms. Institutions and educators may face challenges in acquiring and maintaining the necessary resources and expertise to effectively integrate AI into assessment practices.
Impact on Human Grading and the Role of Educators: The adoption of AI in assessment raises concerns about the potential displacement of human graders. While AI systems can automate certain aspects of grading, human intervention and expertise remain crucial for contextual understanding, providing qualitative feedback, and addressing the individual needs of students. Educators should focus on leveraging AI as a tool to enhance their role rather than replacing them.
Addressing these challenges requires a multidisciplinary approach that combines the expertise of educators, technologists, and policymakers. Collaboration is needed to develop ethical guidelines, improve the transparency of AI algorithms, and ensure that AI-based assessment systems are fair, inclusive, and aligned with educational goals.
As AI technology continues to advance, the advantages of using AI in assessment are expected to outweigh the challenges. With ongoing research, development, and collaboration, AI-powered assessment has the potential to revolutionize education, providing more accurate, efficient, and personalized evaluations while preserving the essential role of human educators.
Future Trends and Implications of AI in Assessment
As AI continues to advance and shape various industries, the future of assessment holds exciting possibilities. The integration of AI in assessment is expected to evolve further, bringing about transformative changes in educational institutions and the role of educators. Let’s explore some future trends and implications of AI in assessment.
Emerging Trends and Advancements in AI-based Assessment
Adaptive Assessments: AI-powered assessment systems have the potential to adapt to individual learner’s needs and provide tailored assessments. By analyzing a student’s performance and learning patterns, AI algorithms can dynamically adjust the difficulty level and content of assessments, ensuring an optimal learning experience.
Multimodal Assessments: With the advancements in natural language processing and computer vision, AI systems can evaluate student work beyond traditional text-based assessments. This opens up possibilities for assessing multimedia assignments, such as videos, audio recordings, and images, enabling a more comprehensive evaluation of student skills and creativity.
Real-time Feedback and Intervention: AI algorithms can provide real-time feedback during the learning process, guiding students in the right direction. Through continuous assessment and feedback, AI-powered systems can identify areas of improvement and intervene with personalized recommendations, ensuring timely support for students.
Data-driven Insights and Predictive Analytics: AI-based assessment systems can generate valuable insights by analyzing large datasets. These insights can inform educational stakeholders about learning trends, strengths and weaknesses of curricula, and student performance patterns. Predictive analytics can also help identify students at risk of falling behind, allowing for early intervention and personalized support.
Potential Impact on Educational Institutions and Educators
Efficiency and Resource Optimization: AI-powered assessments can streamline administrative tasks, allowing educators to focus more on instructional activities and individual student support. This leads to increased efficiency and resource optimization within educational institutions.
Personalized Learning: AI-generated feedback and adaptive assessments enable personalized learning experiences for students. Educators can leverage AI insights to tailor instruction, identify individual learning needs, and provide targeted interventions, fostering student engagement and academic success.
Skill Development and Lifelong Learning: AI-based assessments can go beyond traditional subject-based evaluations and focus on evaluating essential skills such as critical thinking, problem-solving, and creativity. This shift supports the development of 21st-century skills necessary for success in a rapidly evolving job market.
Redefining the Role of Educators: The integration of AI in assessment redefines the role of educators from mere evaluators to facilitators of learning. Educators become guides, leveraging AI-generated insights to personalize instruction, foster critical thinking, and nurture students’ unique talents.
Ethical Considerations and Guidelines for AI in Assessment
As AI becomes more prevalent in assessment practices, it is crucial to address ethical considerations and establish guidelines to ensure responsible and fair use of AI technologies. Some key considerations include:
Transparency and Explainability: AI algorithms should be transparent, and students should understand how their assessments are being evaluated. Institutions should provide clear information about the use of AI in assessment and ensure that students have access to explanations of how their grades and feedback are generated.
Data Privacy and Security: Institutions must prioritize data privacy and security when implementing AI-based assessment systems. Students’ personal information and assessment data should be protected, and strict protocols should be in place to safeguard sensitive data.
Bias Mitigation: Steps should be taken to address and mitigate biases in AI algorithms. Datasets used for training should be diverse and representative of the student population to ensure fair and equitable assessment practices.
Continuous Monitoring and Improvement: Institutions should continually monitor and evaluate the performance of AI-based assessment systems to address any shortcomings or biases. Regular audits and assessments should be conducted to ensure the accuracy, fairness, and reliability of AI-generated grading and feedback.
The Future of Assessment and the Role of AI
AI in assessment represents a paradigm shift in how we evaluate learning outcomes. It has the potential to enhance the efficiency, objectivity, and personalization of assessments, enabling educators to provide timely feedback and support to students. However, it is essential to strike a balance between AI-powered automation and the expertise of educators, ensuring that AI serves as a tool to augment and empower the human element in education.
As AI technology continues to advance, ongoing research, collaboration, and ethical considerations will shape the future of assessment. The integration of AI in assessment holds immense promise in preparing students for a future that demands adaptability, critical thinking, and lifelong learning. By leveraging AI’s capabilities responsibly, educational institutions can unlock new possibilities and optimize the learning experience for generations to come.
The integration of AI in assessment has the potential to reshape the educational landscape, offering new possibilities and transforming traditional assessment practices. As we look ahead, several key trends and implications emerge, highlighting the future of AI in assessment.
Emerging Trends in AI-based Assessment
Gamification and Immersive Assessments: The future of AI in assessment may witness the integration of gamification elements and immersive technologies. Gamified assessments can engage students through interactive simulations, virtual reality, and augmented reality experiences, providing a more authentic and engaging evaluation of their skills and knowledge.
Natural Language Understanding and Generation: Advancements in natural language processing and understanding will enhance AI’s ability to analyze and generate feedback for complex assessments. AI algorithms will become more proficient in understanding nuanced arguments, context, and individual writing styles, leading to more accurate and meaningful feedback.
Emotion and Sentiment Analysis: AI-powered assessment systems may evolve to incorporate emotion and sentiment analysis to understand the emotional state of students during assessments. By detecting emotions such as frustration, confusion, or engagement, AI algorithms can provide tailored support and interventions to enhance the learning experience.
Collaborative and Social Assessments: AI-based assessment systems can facilitate collaborative and social learning experiences. Students can engage in group projects, peer assessments, and collaborative problem-solving activities, with AI algorithms providing feedback on individual and group contributions, promoting teamwork and collaboration skills.
Implications for Educational Institutions and Educators
Redefined Assessment Strategies: The integration of AI in assessment will prompt educational institutions to reconsider their assessment strategies and frameworks. Traditional methods may give way to more dynamic, adaptive, and competency-based assessments that align with the evolving needs of learners and the demands of the future workforce.
Data-Informed Decision Making: AI-powered assessment systems generate a wealth of data that can inform educational stakeholders about student performance, learning trends, and instructional effectiveness. Educational institutions can leverage this data to make data-informed decisions, optimize curriculum design, and personalize learning experiences.
Shift in Educator Roles: The role of educators will evolve from traditional evaluators to learning facilitators and data interpreters. Educators will leverage AI-generated insights to tailor instruction, provide targeted interventions, and foster deeper engagement, focusing on individual student growth and development.
Enhanced Accessibility and Inclusivity: AI-powered assessment systems have the potential to address accessibility and inclusivity challenges. By leveraging natural language processing and adaptive technologies, assessments can be tailored to accommodate diverse learning needs, ensuring equal opportunities for all students.
As AI becomes more prevalent in assessment practices, ethical considerations and guidelines become paramount. To ensure responsible and fair use of AI in assessment, institutions should consider the following:
Ethical Use of Data: Institutions must prioritize data privacy and security, ensuring that student data is protected and used responsibly. Clear policies should be in place to govern data collection, storage, and usage, adhering to legal and ethical standards.
Explainability and Transparency: AI algorithms used in assessment should be transparent, and students should have the opportunity to understand how their assessments are evaluated. Institutions should provide clear explanations of the criteria and processes used by AI systems to generate grades and feedback.
Addressing Bias and Fairness: Institutions should actively address biases in AI algorithms to ensure fair and equitable evaluations. Regular audits and evaluations should be conducted to identify and rectify any biases that may arise from the training data or algorithmic decision-making processes.
Ethical Governance and Accountability: Institutions should establish clear governance frameworks to ensure accountability in the development, implementation, and use of AI in assessment. Regular monitoring, audits, and reviews should be conducted to uphold ethical standards and address any issues that arise.
The future of assessment lies in a dynamic and symbiotic relationship between AI and human educators. AI-powered assessment systems have the potential to enhance efficiency, objectivity, and personalization. Educators play a crucial role in leveraging AI-generated insights and feedback to provide personalized instruction, support individual learning needs, and foster critical thinking skills.
As AI technology continues to advance, ongoing research, collaboration, and ethical considerations will shape the future of assessment. The integration of AI in assessment holds immense promise in preparing students for a future that demands adaptability, critical thinking, and lifelong learning. By embracing AI responsibly, educational institutions can unlock new possibilities and optimize the learning experience for generations to come.
Final Thoughts on the Potential Benefits and Challenges of AI in Assessment
The integration of AI in assessment, particularly in automated grading and feedback, holds immense potential to revolutionize the educational landscape. The benefits of AI in assessment are vast, ranging from increased efficiency and objectivity to enhanced feedback quality and scalability. However, it is crucial to acknowledge and address the challenges and limitations that come with this technology.
AI-based assessment systems have the power to save educators valuable time and resources by automating the grading process. This allows educators to focus more on providing personalized instruction and support to students, fostering a more engaging and impactful learning experience. The consistency and objectivity offered by AI algorithms ensure fair evaluations, eliminating subjective biases and promoting transparency in assessments.
Furthermore, AI-generated feedback provides students with detailed and timely insights into their performance. By analyzing various aspects of their work, AI algorithms can offer personalized feedback that goes beyond what is feasible with manual grading. This enables students to understand their strengths and weaknesses, make targeted improvements, and take ownership of their learning journey.
The scalability of AI-powered assessment systems is another significant advantage. With the ability to handle large volumes of assessments, AI systems are well-suited for online learning platforms, massive open online courses (MOOCs), and global educational initiatives. This scalability ensures that students receive timely feedback regardless of class size or geographical location.
However, alongside these benefits, there are challenges and limitations that need to be considered. AI algorithms are only as unbiased as the data they are trained on. Biases in training data can lead to biased evaluations and feedback, perpetuating existing inequalities. It is essential to continually monitor and address these biases to ensure fair and equitable assessment practices.
Subjective assessments, such as essays and open-ended questions, pose challenges for AI algorithms due to their complexity and the need for human judgment. While AI systems have made significant progress in evaluating subjective assessments, there may still be instances where human graders are required to provide nuanced and context-specific feedback.
Technical limitations and implementation challenges also need to be considered. Implementing AI-based assessment systems requires robust technical infrastructure, secure data storage, and efficient algorithms. Institutions and educators may face challenges in acquiring and maintaining the necessary resources and expertise to effectively integrate AI into assessment practices.
Furthermore, the role of educators should not be undermined or replaced by AI. While AI can automate certain aspects of grading and feedback, human intervention and expertise are crucial for contextual understanding, providing qualitative feedback, and addressing the individual needs of students. Educators should embrace AI as a tool that enhances their role, allowing them to focus on personalized instruction, mentorship, and fostering critical thinking skills.
In conclusion, AI in assessment, specifically automated grading and feedback, has the potential to transform education by increasing efficiency, objectivity, and personalization. The benefits of AI in assessment are vast, but it is important to address challenges such as biases, technical limitations, and the role of educators. By leveraging AI responsibly, educational institutions can unlock the full potential of this technology, empowering students and educators alike to thrive in the evolving educational landscape.
The Potential of AI in School Mining and Mineral Studies
The impact of ai on school environmental studies and conservation.
Comments are closed
Recent Posts
- AI and School Physical Therapy and Rehabilitation September 17, 2023
- AI and School Health Communication and Media Studies September 17, 2023
- The Potential of AI in School Media and Communication Studies September 16, 2023
About Teachflow
Follow us on social media, important links, contact info.
- 2222 Ponce de Leon, Miami, FL 33134
- [email protected]
- +1 (855) 592 0524
newsletter signup

- Industry News
- Beyond Multiple Choice
- 2024 Winners and Finalists
- 2023 Winners and Finalists
- 2022 Winners and Finalists
- 2021 Winners & Finalists
- Consultants Directory
- 2023 Conference Rewind Pass
- AI Special Interest Group
- Online Proctoring Special Interest Group
- Sponsorship Opportunities
Revolutionising essay grading with AI: future of assessment in education

A blog by Manjinder Kainth, PhD. CEO/CO-founder Graide
Gone are the days when teachers had to spend countless hours reading and evaluating stacks of essays. AI-powered essay grading systems are now capable of analysing and assessing a multitude of factors, such as grammar, structure, content, and more, with remarkable speed and precision. By leveraging machine learning algorithms, AI systems not only provide quick feedback to students but also enable educators to identify patterns and trends within the essays.
Furthermore, AI-based essay grading systems eliminate human biases and inconsistencies, levelling the playing field for students. These applications leverage advanced natural language processing techniques to analyse essays and provide constructive suggestions for improvement.
As technology continues to advance, AI is poised to shape the future of education, offering tremendous benefits to both educators and students. So, let’s explore how AI is revolutionising essay grading and opening up new possibilities for a more effective and personalised learning experience.
Traditional methods of essay grading
Every educator knows the drill: piles of essays waiting to be graded, hours spent poring over each one, and the constant challenge of providing meaningful feedback. Grading is an essential part of the educational process, ensuring students understand the material and receive valuable feedback to improve. However, the traditional grading system is fraught with challenges, from the sheer time it consumes to the inconsistency that can arise from human error.
The shortcomings of traditional essay grading
Traditional grading methods, while tried and tested, have inherent limitations. First and foremost, they are time-consuming. Educators often spend hours, if not days, grading a single batch of essays. This not only leads to fatigue but can also result in inconsistent grading as the teacher’s concentration wanes.
Moreover, no two educators grade identically. What one teacher might consider an ‘A’ essay, another might deem a ‘B+’. This lack of standardisation can be confusing for students and can even impact their academic trajectory.
Some might argue that the solution lies in fully automated grading systems. However, these systems often lack the nuance and understanding required to grade complex subjects, especially in subjects like literature or philosophy. They fail to capture the essence of an argument or the subtleties of a well-crafted essay. In short, while they might offer speed, they compromise on quality.
AI essay grading solution
With the traditional grading issues enters the AI essay grading system like Graide. Graide was born out of a need identified at the University of Birmingham, Graide sought to bridge the gap between speed and quality. Recognizing that fully automated solutions were falling short, the team at Graide embarked on a mission to create a system that combined the best of both worlds.
The result? An AI-driven grading system that learns from minimal data points. Instead of requiring vast amounts of data to understand and grade an essay, Graide’s system can quickly adapt and provide accurate, consistent feedback. It’s a game-changer, not just in terms of efficiency but also in the quality of feedback provided.
Case study of AI-powered essay grading
In collaboration with Oxbridge Ltd, the Graide AI essay tool was used to grade essays on complex subjects like Shakespeare and poetry. The results were nothing short of astounding. With minimal data input, the AI was able to understand and grade these intricate essays with remarkable accuracy.
For educators, this means a drastic reduction in the hours spent grading. But more than that, it promises consistent and precise feedback for students, ensuring they receive the guidance they need to improve.
For students, the benefits are manifold. With the potential for automated feedback on practice essays, they can receive feedback almost instantly, allowing for more touchpoints and opportunities to refine their skills.
Implementing AI-powered essay grading in educational institutions
To successfully implement AI-powered essay grading in educational institutions, a thoughtful and strategic approach is key. It is crucial to involve stakeholders, including teachers, students, and administrators, in the decision-making process. Their input can help identify specific needs and concerns, ensuring the successful integration of AI systems into existing educational frameworks.
Training and professional development programmes should be provided to educators to familiarise them with AI-powered grading systems. Educators need to understand the capabilities and limitations of the systems, enabling them to effectively leverage AI-generated feedback and tailor their instruction accordingly. This collaborative approach ensures that AI is used as a tool to enhance teaching and learning, rather than replace human interaction.
Additionally, ongoing monitoring and evaluation of AI systems should be conducted to ensure their effectiveness and address any unforeseen challenges. Regular feedback from educators and students can help refine and improve the algorithms, making them more accurate and reliable over time.
Final thoughts
AI is revolutionising higher education by transforming the learning experience. From personalised learning paths to intelligent tutoring systems to faster feedback, AI is reshaping traditional educational models and making education more accessible and effective. By leveraging AI, institutions can deliver personalised learning experiences, enhance student assessments and feedback, streamline administrative tasks, and gain valuable insights through learning analytics. However, as AI continues to advance, ethical considerations and challenges should be addressed to ensure fairness, privacy, and the preservation of human interaction in education.
Artificial intelligence will power education in the future. If you’re an educator or institution looking to revolutionise your grading system, to provide consistent, accurate feedback, and free up invaluable time, take a look at Graide’s AI essay grading system.

News categories
- Press Releases

Upcoming Events
Iacat conference 2024, the 25th mwalt conference.
- Privacy Policy
e-rater ® Scoring Engine
Evaluates students’ writing proficiency with automatic scoring and feedback
Selection an option below to learn more.
About the e-rater Scoring Engine
The e-rater automated scoring engine uses AI technology and Natural Language Processing (NLP) to evaluate the writing proficiency of student essays by providing automatic scoring and feedback. The engine provides descriptive feedback on the writer’s grammar, mechanics, word use and complexity, style, organization and more.
Who uses the e-rater engine and why?
Companies and institutions use this patented technology to power their custom applications.
The e-rater engine is used within the Criterion ® Online Writing Evaluation Service . Students use the e-rater engine's feedback to evaluate their essay-writing skills and to identify areas that need improvement. Teachers use the Criterion service to help their students develop their writing skills independently and receive automated, constructive feedback. The e-rater engine is also used in other low-stakes practice tests include TOEFL ® Practice Online and GRE ® ScoreItNow!™.
In high-stakes settings, the engine is used in conjunction with human ratings for both the Issue and Argument prompts of the GRE test's Analytical Writing section and the TOEFL iBT ® test's Independent and Integrated Writing prompts. ETS research has shown that combining automated and human essay scoring demonstrates assessment score reliability and measurement benefits.
For more information about the use of the e-rater engine, read E-rater as a Quality Control on Human Scores (PDF) .
How does the e-rater engine grade essays?
The e-rater engine provides a holistic score for an essay that has been entered into the computer electronically. It also provides real-time diagnostic feedback about grammar, usage, mechanics, style and organization, and development. This feedback is based on NLP research specifically tailored to the analysis of student responses and is detailed in ETS's research publications (PDF) .
How does the e-rater engine compare to human raters?
The e-rater engine uses NLP to identify features relevant to writing proficiency in training essays and their relationship with human scores. The resulting scoring model, which assigns weights to each observed feature, is stored offline in a database that can then be used to score new essays according to the same formula.
The e-rater engine doesn’t have the ability to read so it can’t evaluate essays the same way that human raters do. However, the features used in e-rater scoring have been developed to be as substantively meaningful as they can be, given the state of the art in NLP. They also have been developed to demonstrate strong reliability — often greater reliability than human raters themselves.
Learn more about how it works .
About Natural Language Processing
The e-rater engine is an artificial intelligence engine that uses Natural Language Processing (NLP), a field of computer science and linguistics that uses computational methods to analyze characteristics of a text. NLP methods support such burgeoning application areas as machine translation, speech recognition and information retrieval.
Ready to begin? Contact us to learn how the e-rater service can enhance your existing program.

Breadcrumbs Section. Click here to navigate to respective pages.

Automated Essay Scoring
DOI link for Automated Essay Scoring
Get Citation
This new volume is the first to focus entirely on automated essay scoring and evaluation. It is intended to provide a comprehensive overview of the evolution and state-of-the-art of automated essay scoring and evaluation technology across several disciplines, including education, testing and measurement, cognitive science, computer science, and computational linguistics. The development of this technology has led to many questions and concerns. Automated Essay Scoring attempts to address some of these questions including: *How can automated scoring and evaluation supplement classroom instruction? *How does the technology actually work? *Can it improve students' writing? *How reliable is the technology? *How can these computing methods be used to develop evaluation tools? *What are the state-of the-art essay evaluation technologies and automated scoring systems? Divided into four parts, the first part reviews the teaching of writing and how computers can contribute to it. Part II analyzes actual automated essay scorers including e-raterTM, Intellimetric , and the Intelligent Essay Assessor . The third part analyzes related psychometric issues, and the final part reviews innovations in the field. This book is ideal for researchers and advanced students interested in automated essay scoring from the fields of testing and measurement, education, cognitive science, language, and computational linguistics.
TABLE OF CONTENTS
Part i | 2 pages, teaching of writing, chapter 1 | 16 pages, what can computers and aes contribute to a k-12 writing program, part ii | 2 pages, psychometric issues in performance assessment, chapter 2 | 16 pages, issues in the reliability and validity of automated scoring of constructed responses, part iii | 2 pages, automated essay scorers, chapter 3 | 12 pages, project essay grade: peg, chapter 4 | 16 pages, a text categorization approach to automated essay grading, chapter 5 | 15 pages, intellimetric™: from here to validity, chapter 6 | 25 pages, automated scoring and annotation of essays with the intelligent essay assessor™, chapter 7 | 9 pages, the e-rater® scoring engine: automated essay scoring with natural language processing, part iv | 2 pages, psychometric issues in automated essay scoring, chapter 8 | 22 pages, the concept of reliability in the context of automated essay scoring, chapter 9 | 21 pages, validity of automated essay scoring systems, chapter 10 | 11 pages, norming and scaling for automated essay scoring, chapter 11 | 12 pages, bayesian analysis of essay grading, part v | 2 pages, current innovation in automated essay evaluation, chapter 12 | 14 pages, automated grammatical error detection, chapter 13 | 20 pages, automated evaluation of discourse structure in student essays.
- Privacy Policy
- Terms & Conditions
- Cookie Policy
- Taylor & Francis Online
- Taylor & Francis Group
- Students/Researchers
- Librarians/Institutions
Connect with us
Registered in England & Wales No. 3099067 5 Howick Place | London | SW1P 1WG © 2024 Informa UK Limited

An official website of the United States government
The .gov means it’s official. Federal government websites often end in .gov or .mil. Before sharing sensitive information, make sure you’re on a federal government site.
The site is secure. The https:// ensures that you are connecting to the official website and that any information you provide is encrypted and transmitted securely.
- Publications
- Account settings
The PMC website is updating on October 15, 2024. Learn More or Try it out now .
- Advanced Search
- Journal List
- Springer Nature - PMC COVID-19 Collection

An automated essay scoring systems: a systematic literature review
Dadi ramesh.
1 School of Computer Science and Artificial Intelligence, SR University, Warangal, TS India
2 Research Scholar, JNTU, Hyderabad, India
Suresh Kumar Sanampudi
3 Department of Information Technology, JNTUH College of Engineering, Nachupally, Kondagattu, Jagtial, TS India
Associated Data
Assessment in the Education system plays a significant role in judging student performance. The present evaluation system is through human assessment. As the number of teachers' student ratio is gradually increasing, the manual evaluation process becomes complicated. The drawback of manual evaluation is that it is time-consuming, lacks reliability, and many more. This connection online examination system evolved as an alternative tool for pen and paper-based methods. Present Computer-based evaluation system works only for multiple-choice questions, but there is no proper evaluation system for grading essays and short answers. Many researchers are working on automated essay grading and short answer scoring for the last few decades, but assessing an essay by considering all parameters like the relevance of the content to the prompt, development of ideas, Cohesion, and Coherence is a big challenge till now. Few researchers focused on Content-based evaluation, while many of them addressed style-based assessment. This paper provides a systematic literature review on automated essay scoring systems. We studied the Artificial Intelligence and Machine Learning techniques used to evaluate automatic essay scoring and analyzed the limitations of the current studies and research trends. We observed that the essay evaluation is not done based on the relevance of the content and coherence.
Supplementary Information
The online version contains supplementary material available at 10.1007/s10462-021-10068-2.
Introduction
Due to COVID 19 outbreak, an online educational system has become inevitable. In the present scenario, almost all the educational institutions ranging from schools to colleges adapt the online education system. The assessment plays a significant role in measuring the learning ability of the student. Most automated evaluation is available for multiple-choice questions, but assessing short and essay answers remain a challenge. The education system is changing its shift to online-mode, like conducting computer-based exams and automatic evaluation. It is a crucial application related to the education domain, which uses natural language processing (NLP) and Machine Learning techniques. The evaluation of essays is impossible with simple programming languages and simple techniques like pattern matching and language processing. Here the problem is for a single question, we will get more responses from students with a different explanation. So, we need to evaluate all the answers concerning the question.
Automated essay scoring (AES) is a computer-based assessment system that automatically scores or grades the student responses by considering appropriate features. The AES research started in 1966 with the Project Essay Grader (PEG) by Ajay et al. ( 1973 ). PEG evaluates the writing characteristics such as grammar, diction, construction, etc., to grade the essay. A modified version of the PEG by Shermis et al. ( 2001 ) was released, which focuses on grammar checking with a correlation between human evaluators and the system. Foltz et al. ( 1999 ) introduced an Intelligent Essay Assessor (IEA) by evaluating content using latent semantic analysis to produce an overall score. Powers et al. ( 2002 ) proposed E-rater and Intellimetric by Rudner et al. ( 2006 ) and Bayesian Essay Test Scoring System (BESTY) by Rudner and Liang ( 2002 ), these systems use natural language processing (NLP) techniques that focus on style and content to obtain the score of an essay. The vast majority of the essay scoring systems in the 1990s followed traditional approaches like pattern matching and a statistical-based approach. Since the last decade, the essay grading systems started using regression-based and natural language processing techniques. AES systems like Dong et al. ( 2017 ) and others developed from 2014 used deep learning techniques, inducing syntactic and semantic features resulting in better results than earlier systems.
Ohio, Utah, and most US states are using AES systems in school education, like Utah compose tool, Ohio standardized test (an updated version of PEG), evaluating millions of student's responses every year. These systems work for both formative, summative assessments and give feedback to students on the essay. Utah provided basic essay evaluation rubrics (six characteristics of essay writing): Development of ideas, organization, style, word choice, sentence fluency, conventions. Educational Testing Service (ETS) has been conducting significant research on AES for more than a decade and designed an algorithm to evaluate essays on different domains and providing an opportunity for test-takers to improve their writing skills. In addition, they are current research content-based evaluation.
The evaluation of essay and short answer scoring should consider the relevance of the content to the prompt, development of ideas, Cohesion, Coherence, and domain knowledge. Proper assessment of the parameters mentioned above defines the accuracy of the evaluation system. But all these parameters cannot play an equal role in essay scoring and short answer scoring. In a short answer evaluation, domain knowledge is required, like the meaning of "cell" in physics and biology is different. And while evaluating essays, the implementation of ideas with respect to prompt is required. The system should also assess the completeness of the responses and provide feedback.
Several studies examined AES systems, from the initial to the latest AES systems. In which the following studies on AES systems are Blood ( 2011 ) provided a literature review from PEG 1984–2010. Which has covered only generalized parts of AES systems like ethical aspects, the performance of the systems. Still, they have not covered the implementation part, and it’s not a comparative study and has not discussed the actual challenges of AES systems.
Burrows et al. ( 2015 ) Reviewed AES systems on six dimensions like dataset, NLP techniques, model building, grading models, evaluation, and effectiveness of the model. They have not covered feature extraction techniques and challenges in features extractions. Covered only Machine Learning models but not in detail. This system not covered the comparative analysis of AES systems like feature extraction, model building, and level of relevance, cohesion, and coherence not covered in this review.
Ke et al. ( 2019 ) provided a state of the art of AES system but covered very few papers and not listed all challenges, and no comparative study of the AES model. On the other hand, Hussein et al. in ( 2019 ) studied two categories of AES systems, four papers from handcrafted features for AES systems, and four papers from the neural networks approach, discussed few challenges, and did not cover feature extraction techniques, the performance of AES models in detail.
Klebanov et al. ( 2020 ). Reviewed 50 years of AES systems, listed and categorized all essential features that need to be extracted from essays. But not provided a comparative analysis of all work and not discussed the challenges.
This paper aims to provide a systematic literature review (SLR) on automated essay grading systems. An SLR is an Evidence-based systematic review to summarize the existing research. It critically evaluates and integrates all relevant studies' findings and addresses the research domain's specific research questions. Our research methodology uses guidelines given by Kitchenham et al. ( 2009 ) for conducting the review process; provide a well-defined approach to identify gaps in current research and to suggest further investigation.
We addressed our research method, research questions, and the selection process in Sect. 2 , and the results of the research questions have discussed in Sect. 3 . And the synthesis of all the research questions addressed in Sect. 4 . Conclusion and possible future work discussed in Sect. 5 .
Research method
We framed the research questions with PICOC criteria.
Population (P) Student essays and answers evaluation systems.
Intervention (I) evaluation techniques, data sets, features extraction methods.
Comparison (C) Comparison of various approaches and results.
Outcomes (O) Estimate the accuracy of AES systems,
Context (C) NA.
Research questions
To collect and provide research evidence from the available studies in the domain of automated essay grading, we framed the following research questions (RQ):
RQ1 what are the datasets available for research on automated essay grading?
The answer to the question can provide a list of the available datasets, their domain, and access to the datasets. It also provides a number of essays and corresponding prompts.
RQ2 what are the features extracted for the assessment of essays?
The answer to the question can provide an insight into various features so far extracted, and the libraries used to extract those features.
RQ3, which are the evaluation metrics available for measuring the accuracy of algorithms?
The answer will provide different evaluation metrics for accurate measurement of each Machine Learning approach and commonly used measurement technique.
RQ4 What are the Machine Learning techniques used for automatic essay grading, and how are they implemented?
It can provide insights into various Machine Learning techniques like regression models, classification models, and neural networks for implementing essay grading systems. The response to the question can give us different assessment approaches for automated essay grading systems.
RQ5 What are the challenges/limitations in the current research?
The answer to the question provides limitations of existing research approaches like cohesion, coherence, completeness, and feedback.
Search process
We conducted an automated search on well-known computer science repositories like ACL, ACM, IEEE Explore, Springer, and Science Direct for an SLR. We referred to papers published from 2010 to 2020 as much of the work during these years focused on advanced technologies like deep learning and natural language processing for automated essay grading systems. Also, the availability of free data sets like Kaggle (2012), Cambridge Learner Corpus-First Certificate in English exam (CLC-FCE) by Yannakoudakis et al. ( 2011 ) led to research this domain.
Search Strings : We used search strings like “Automated essay grading” OR “Automated essay scoring” OR “short answer scoring systems” OR “essay scoring systems” OR “automatic essay evaluation” and searched on metadata.
Selection criteria
After collecting all relevant documents from the repositories, we prepared selection criteria for inclusion and exclusion of documents. With the inclusion and exclusion criteria, it becomes more feasible for the research to be accurate and specific.
Inclusion criteria 1 Our approach is to work with datasets comprise of essays written in English. We excluded the essays written in other languages.
Inclusion criteria 2 We included the papers implemented on the AI approach and excluded the traditional methods for the review.
Inclusion criteria 3 The study is on essay scoring systems, so we exclusively included the research carried out on only text data sets rather than other datasets like image or speech.
Exclusion criteria We removed the papers in the form of review papers, survey papers, and state of the art papers.
Quality assessment
In addition to the inclusion and exclusion criteria, we assessed each paper by quality assessment questions to ensure the article's quality. We included the documents that have clearly explained the approach they used, the result analysis and validation.
The quality checklist questions are framed based on the guidelines from Kitchenham et al. ( 2009 ). Each quality assessment question was graded as either 1 or 0. The final score of the study range from 0 to 3. A cut off score for excluding a study from the review is 2 points. Since the papers scored 2 or 3 points are included in the final evaluation. We framed the following quality assessment questions for the final study.
Quality Assessment 1: Internal validity.
Quality Assessment 2: External validity.
Quality Assessment 3: Bias.
The two reviewers review each paper to select the final list of documents. We used the Quadratic Weighted Kappa score to measure the final agreement between the two reviewers. The average resulted from the kappa score is 0.6942, a substantial agreement between the reviewers. The result of evolution criteria shown in Table Table1. 1 . After Quality Assessment, the final list of papers for review is shown in Table Table2. 2 . The complete selection process is shown in Fig. Fig.1. 1 . The total number of selected papers in year wise as shown in Fig. Fig.2. 2 .
Quality assessment analysis
Number of papers | Quality assessment score |
---|---|
50 | 3 |
12 | 2 |
59 | 1 |
23 | 0 |
Final list of papers
Data base | Paper count |
---|---|
ACL | 28 |
ACM | 5 |
IEEE Explore | 19 |
Springer | 5 |
Other | 5 |
Total | 62 |

Selection process

Year wise publications
What are the datasets available for research on automated essay grading?
To work with problem statement especially in Machine Learning and deep learning domain, we require considerable amount of data to train the models. To answer this question, we listed all the data sets used for training and testing for automated essay grading systems. The Cambridge Learner Corpus-First Certificate in English exam (CLC-FCE) Yannakoudakis et al. ( 2011 ) developed corpora that contain 1244 essays and ten prompts. This corpus evaluates whether a student can write the relevant English sentences without any grammatical and spelling mistakes. This type of corpus helps to test the models built for GRE and TOFEL type of exams. It gives scores between 1 and 40.
Bailey and Meurers ( 2008 ), Created a dataset (CREE reading comprehension) for language learners and automated short answer scoring systems. The corpus consists of 566 responses from intermediate students. Mohler and Mihalcea ( 2009 ). Created a dataset for the computer science domain consists of 630 responses for data structure assignment questions. The scores are range from 0 to 5 given by two human raters.
Dzikovska et al. ( 2012 ) created a Student Response Analysis (SRA) corpus. It consists of two sub-groups: the BEETLE corpus consists of 56 questions and approximately 3000 responses from students in the electrical and electronics domain. The second one is the SCIENTSBANK(SemEval-2013) (Dzikovska et al. 2013a ; b ) corpus consists of 10,000 responses on 197 prompts on various science domains. The student responses ladled with "correct, partially correct incomplete, Contradictory, Irrelevant, Non-domain."
In the Kaggle (2012) competition, released total 3 types of corpuses on an Automated Student Assessment Prize (ASAP1) (“ https://www.kaggle.com/c/asap-sas/ ” ) essays and short answers. It has nearly 17,450 essays, out of which it provides up to 3000 essays for each prompt. It has eight prompts that test 7th to 10th grade US students. It gives scores between the [0–3] and [0–60] range. The limitations of these corpora are: (1) it has a different score range for other prompts. (2) It uses statistical features such as named entities extraction and lexical features of words to evaluate essays. ASAP + + is one more dataset from Kaggle. It is with six prompts, and each prompt has more than 1000 responses total of 10,696 from 8th-grade students. Another corpus contains ten prompts from science, English domains and a total of 17,207 responses. Two human graders evaluated all these responses.
Correnti et al. ( 2013 ) created a Response-to-Text Assessment (RTA) dataset used to check student writing skills in all directions like style, mechanism, and organization. 4–8 grade students give the responses to RTA. Basu et al. ( 2013 ) created a power grading dataset with 700 responses for ten different prompts from US immigration exams. It contains all short answers for assessment.
The TOEFL11 corpus Blanchard et al. ( 2013 ) contains 1100 essays evenly distributed over eight prompts. It is used to test the English language skills of a candidate attending the TOFEL exam. It scores the language proficiency of a candidate as low, medium, and high.
International Corpus of Learner English (ICLE) Granger et al. ( 2009 ) built a corpus of 3663 essays covering different dimensions. It has 12 prompts with 1003 essays that test the organizational skill of essay writing, and13 prompts, each with 830 essays that examine the thesis clarity and prompt adherence.
Argument Annotated Essays (AAE) Stab and Gurevych ( 2014 ) developed a corpus that contains 102 essays with 101 prompts taken from the essayforum2 site. It tests the persuasive nature of the student essay. The SCIENTSBANK corpus used by Sakaguchi et al. ( 2015 ) available in git-hub, containing 9804 answers to 197 questions in 15 science domains. Table Table3 3 illustrates all datasets related to AES systems.
ALL types Datasets used in Automatic scoring systems
Data Set | Language | Total responses | Number of prompts |
---|---|---|---|
Cambridge Learner Corpus-First Certificate in English exam (CLC-FCE) | English | 1244 | |
CREE | English | 566 | |
CS | English | 630 | |
SRA | English | 3000 | 56 |
SCIENTSBANK(SemEval-2013) | English | 10,000 | 197 |
ASAP-AES | English | 17,450 | 8 |
ASAP-SAS | English | 17,207 | 10 |
ASAP + + | English | 10,696 | 6 |
power grading | English | 700 | |
TOEFL11 | English | 1100 | 8 |
International Corpus of Learner English (ICLE) | English | 3663 |
Features play a major role in the neural network and other supervised Machine Learning approaches. The automatic essay grading systems scores student essays based on different types of features, which play a prominent role in training the models. Based on their syntax and semantics and they are categorized into three groups. 1. statistical-based features Contreras et al. ( 2018 ); Kumar et al. ( 2019 ); Mathias and Bhattacharyya ( 2018a ; b ) 2. Style-based (Syntax) features Cummins et al. ( 2016 ); Darwish and Mohamed ( 2020 ); Ke et al. ( 2019 ). 3. Content-based features Dong et al. ( 2017 ). A good set of features appropriate models evolved better AES systems. The vast majority of the researchers are using regression models if features are statistical-based. For Neural Networks models, researches are using both style-based and content-based features. The following table shows the list of various features used in existing AES Systems. Table Table4 4 represents all set of features used for essay grading.
Types of features
Statistical features | Style based features | Content based features |
---|---|---|
Essay length with respect to the number of words | Sentence structure | Cohesion between sentences in a document |
Essay length with respect to sentence | POS | Overlapping (prompt) |
Average sentence length | Punctuation | Relevance of information |
Average word length | Grammatical | Semantic role of words |
N-gram | Logical operators | Correctness |
Vocabulary | Consistency | |
Sentence expressing key concepts |
We studied all the feature extracting NLP libraries as shown in Fig. Fig.3. that 3 . that are used in the papers. The NLTK is an NLP tool used to retrieve statistical features like POS, word count, sentence count, etc. With NLTK, we can miss the essay's semantic features. To find semantic features Word2Vec Mikolov et al. ( 2013 ), GloVe Jeffrey Pennington et al. ( 2014 ) is the most used libraries to retrieve the semantic text from the essays. And in some systems, they directly trained the model with word embeddings to find the score. From Fig. Fig.4 4 as observed that non-content-based feature extraction is higher than content-based.

Usages of tools

Number of papers on content based features
RQ3 which are the evaluation metrics available for measuring the accuracy of algorithms?
The majority of the AES systems are using three evaluation metrics. They are (1) quadrated weighted kappa (QWK) (2) Mean Absolute Error (MAE) (3) Pearson Correlation Coefficient (PCC) Shehab et al. ( 2016 ). The quadratic weighted kappa will find agreement between human evaluation score and system evaluation score and produces value ranging from 0 to 1. And the Mean Absolute Error is the actual difference between human-rated score to system-generated score. The mean square error (MSE) measures the average squares of the errors, i.e., the average squared difference between the human-rated and the system-generated scores. MSE will always give positive numbers only. Pearson's Correlation Coefficient (PCC) finds the correlation coefficient between two variables. It will provide three values (0, 1, − 1). "0" represents human-rated and system scores that are not related. "1" represents an increase in the two scores. "− 1" illustrates a negative relationship between the two scores.
RQ4 what are the Machine Learning techniques being used for automatic essay grading, and how are they implemented?
After scrutinizing all documents, we categorize the techniques used in automated essay grading systems into four baskets. 1. Regression techniques. 2. Classification model. 3. Neural networks. 4. Ontology-based approach.
All the existing AES systems developed in the last ten years employ supervised learning techniques. Researchers using supervised methods viewed the AES system as either regression or classification task. The goal of the regression task is to predict the score of an essay. The classification task is to classify the essays belonging to (low, medium, or highly) relevant to the question's topic. Since the last three years, most AES systems developed made use of the concept of the neural network.
Regression based models
Mohler and Mihalcea ( 2009 ). proposed text-to-text semantic similarity to assign a score to the student essays. There are two text similarity measures like Knowledge-based measures, corpus-based measures. There eight knowledge-based tests with all eight models. They found the similarity. The shortest path similarity determines based on the length, which shortest path between two contexts. Leacock & Chodorow find the similarity based on the shortest path's length between two concepts using node-counting. The Lesk similarity finds the overlap between the corresponding definitions, and Wu & Palmer algorithm finds similarities based on the depth of two given concepts in the wordnet taxonomy. Resnik, Lin, Jiang&Conrath, Hirst& St-Onge find the similarity based on different parameters like the concept, probability, normalization factor, lexical chains. In corpus-based likeness, there LSA BNC, LSA Wikipedia, and ESA Wikipedia, latent semantic analysis is trained on Wikipedia and has excellent domain knowledge. Among all similarity scores, correlation scores LSA Wikipedia scoring accuracy is more. But these similarity measure algorithms are not using NLP concepts. These models are before 2010 and basic concept models to continue the research automated essay grading with updated algorithms on neural networks with content-based features.
Adamson et al. ( 2014 ) proposed an automatic essay grading system which is a statistical-based approach in this they retrieved features like POS, Character count, Word count, Sentence count, Miss spelled words, n-gram representation of words to prepare essay vector. They formed a matrix with these all vectors in that they applied LSA to give a score to each essay. It is a statistical approach that doesn’t consider the semantics of the essay. The accuracy they got when compared to the human rater score with the system is 0.532.
Cummins et al. ( 2016 ). Proposed Timed Aggregate Perceptron vector model to give ranking to all the essays, and later they converted the rank algorithm to predict the score of the essay. The model trained with features like Word unigrams, bigrams, POS, Essay length, grammatical relation, Max word length, sentence length. It is multi-task learning, gives ranking to the essays, and predicts the score for the essay. The performance evaluated through QWK is 0.69, a substantial agreement between the human rater and the system.
Sultan et al. ( 2016 ). Proposed a Ridge regression model to find short answer scoring with Question Demoting. Question Demoting is the new concept included in the essay's final assessment to eliminate duplicate words from the essay. The extracted features are Text Similarity, which is the similarity between the student response and reference answer. Question Demoting is the number of repeats in a student response. With inverse document frequency, they assigned term weight. The sentence length Ratio is the number of words in the student response, is another feature. With these features, the Ridge regression model was used, and the accuracy they got 0.887.
Contreras et al. ( 2018 ). Proposed Ontology based on text mining in this model has given a score for essays in phases. In phase-I, they generated ontologies with ontoGen and SVM to find the concept and similarity in the essay. In phase II from ontologies, they retrieved features like essay length, word counts, correctness, vocabulary, and types of word used, domain information. After retrieving statistical data, they used a linear regression model to find the score of the essay. The accuracy score is the average of 0.5.
Darwish and Mohamed ( 2020 ) proposed the fusion of fuzzy Ontology with LSA. They retrieve two types of features, like syntax features and semantic features. In syntax features, they found Lexical Analysis with tokens, and they construct a parse tree. If the parse tree is broken, the essay is inconsistent—a separate grade assigned to the essay concerning syntax features. The semantic features are like similarity analysis, Spatial Data Analysis. Similarity analysis is to find duplicate sentences—Spatial Data Analysis for finding Euclid distance between the center and part. Later they combine syntax features and morphological features score for the final score. The accuracy they achieved with the multiple linear regression model is 0.77, mostly on statistical features.
Süzen Neslihan et al. ( 2020 ) proposed a text mining approach for short answer grading. First, their comparing model answers with student response by calculating the distance between two sentences. By comparing the model answer with student response, they find the essay's completeness and provide feedback. In this approach, model vocabulary plays a vital role in grading, and with this model vocabulary, the grade will be assigned to the student's response and provides feedback. The correlation between the student answer to model answer is 0.81.
Classification based Models
Persing and Ng ( 2013 ) used a support vector machine to score the essay. The features extracted are OS, N-gram, and semantic text to train the model and identified the keywords from the essay to give the final score.
Sakaguchi et al. ( 2015 ) proposed two methods: response-based and reference-based. In response-based scoring, the extracted features are response length, n-gram model, and syntactic elements to train the support vector regression model. In reference-based scoring, features such as sentence similarity using word2vec is used to find the cosine similarity of the sentences that is the final score of the response. First, the scores were discovered individually and later combined two features to find a final score. This system gave a remarkable increase in performance by combining the scores.
Mathias and Bhattacharyya ( 2018a ; b ) Proposed Automated Essay Grading Dataset with Essay Attribute Scores. The first concept features selection depends on the essay type. So the common attributes are Content, Organization, Word Choice, Sentence Fluency, Conventions. In this system, each attribute is scored individually, with the strength of each attribute identified. The model they used is a random forest classifier to assign scores to individual attributes. The accuracy they got with QWK is 0.74 for prompt 1 of the ASAS dataset ( https://www.kaggle.com/c/asap-sas/ ).
Ke et al. ( 2019 ) used a support vector machine to find the response score. In this method, features like Agreeability, Specificity, Clarity, Relevance to prompt, Conciseness, Eloquence, Confidence, Direction of development, Justification of opinion, and Justification of importance. First, the individual parameter score obtained was later combined with all scores to give a final response score. The features are used in the neural network to find whether the sentence is relevant to the topic or not.
Salim et al. ( 2019 ) proposed an XGBoost Machine Learning classifier to assess the essays. The algorithm trained on features like word count, POS, parse tree depth, and coherence in the articles with sentence similarity percentage; cohesion and coherence are considered for training. And they implemented K-fold cross-validation for a result the average accuracy after specific validations is 68.12.
Neural network models
Shehab et al. ( 2016 ) proposed a neural network method that used learning vector quantization to train human scored essays. After training, the network can provide a score to the ungraded essays. First, we should process the essay to remove Spell checking and then perform preprocessing steps like Document Tokenization, stop word removal, Stemming, and submit it to the neural network. Finally, the model will provide feedback on the essay, whether it is relevant to the topic. And the correlation coefficient between human rater and system score is 0.7665.
Kopparapu and De ( 2016 ) proposed the Automatic Ranking of Essays using Structural and Semantic Features. This approach constructed a super essay with all the responses. Next, ranking for a student essay is done based on the super-essay. The structural and semantic features derived helps to obtain the scores. In a paragraph, 15 Structural features like an average number of sentences, the average length of sentences, and the count of words, nouns, verbs, adjectives, etc., are used to obtain a syntactic score. A similarity score is used as semantic features to calculate the overall score.
Dong and Zhang ( 2016 ) proposed a hierarchical CNN model. The model builds two layers with word embedding to represents the words as the first layer. The second layer is a word convolution layer with max-pooling to find word vectors. The next layer is a sentence-level convolution layer with max-pooling to find the sentence's content and synonyms. A fully connected dense layer produces an output score for an essay. The accuracy with the hierarchical CNN model resulted in an average QWK of 0.754.
Taghipour and Ng ( 2016 ) proposed a first neural approach for essay scoring build in which convolution and recurrent neural network concepts help in scoring an essay. The network uses a lookup table with the one-hot representation of the word vector of an essay. The final efficiency of the network model with LSTM resulted in an average QWK of 0.708.
Dong et al. ( 2017 ). Proposed an Attention-based scoring system with CNN + LSTM to score an essay. For CNN, the input parameters were character embedding and word embedding, and it has attention pooling layers and used NLTK to obtain word and character embedding. The output gives a sentence vector, which provides sentence weight. After CNN, it will have an LSTM layer with an attention pooling layer, and this final layer results in the final score of the responses. The average QWK score is 0.764.
Riordan et al. ( 2017 ) proposed a neural network with CNN and LSTM layers. Word embedding, given as input to a neural network. An LSTM network layer will retrieve the window features and delivers them to the aggregation layer. The aggregation layer is a superficial layer that takes a correct window of words and gives successive layers to predict the answer's sore. The accuracy of the neural network resulted in a QWK of 0.90.
Zhao et al. ( 2017 ) proposed a new concept called Memory-Augmented Neural network with four layers, input representation layer, memory addressing layer, memory reading layer, and output layer. An input layer represents all essays in a vector form based on essay length. After converting the word vector, the memory addressing layer takes a sample of the essay and weighs all the terms. The memory reading layer takes the input from memory addressing segment and finds the content to finalize the score. Finally, the output layer will provide the final score of the essay. The accuracy of essay scores is 0.78, which is far better than the LSTM neural network.
Mathias and Bhattacharyya ( 2018a ; b ) proposed deep learning networks using LSTM with the CNN layer and GloVe pre-trained word embeddings. For this, they retrieved features like Sentence count essays, word count per sentence, Number of OOVs in the sentence, Language model score, and the text's perplexity. The network predicted the goodness scores of each essay. The higher the goodness scores, means higher the rank and vice versa.
Nguyen and Dery ( 2016 ). Proposed Neural Networks for Automated Essay Grading. In this method, a single layer bi-directional LSTM accepting word vector as input. Glove vectors used in this method resulted in an accuracy of 90%.
Ruseti et al. ( 2018 ) proposed a recurrent neural network that is capable of memorizing the text and generate a summary of an essay. The Bi-GRU network with the max-pooling layer molded on the word embedding of each document. It will provide scoring to the essay by comparing it with a summary of the essay from another Bi-GRU network. The result obtained an accuracy of 0.55.
Wang et al. ( 2018a ; b ) proposed an automatic scoring system with the bi-LSTM recurrent neural network model and retrieved the features using the word2vec technique. This method generated word embeddings from the essay words using the skip-gram model. And later, word embedding is used to train the neural network to find the final score. The softmax layer in LSTM obtains the importance of each word. This method used a QWK score of 0.83%.
Dasgupta et al. ( 2018 ) proposed a technique for essay scoring with augmenting textual qualitative Features. It extracted three types of linguistic, cognitive, and psychological features associated with a text document. The linguistic features are Part of Speech (POS), Universal Dependency relations, Structural Well-formedness, Lexical Diversity, Sentence Cohesion, Causality, and Informativeness of the text. The psychological features derived from the Linguistic Information and Word Count (LIWC) tool. They implemented a convolution recurrent neural network that takes input as word embedding and sentence vector, retrieved from the GloVe word vector. And the second layer is the Convolution Layer to find local features. The next layer is the recurrent neural network (LSTM) to find corresponding of the text. The accuracy of this method resulted in an average QWK of 0.764.
Liang et al. ( 2018 ) proposed a symmetrical neural network AES model with Bi-LSTM. They are extracting features from sample essays and student essays and preparing an embedding layer as input. The embedding layer output is transfer to the convolution layer from that LSTM will be trained. Hear the LSRM model has self-features extraction layer, which will find the essay's coherence. The average QWK score of SBLSTMA is 0.801.
Liu et al. ( 2019 ) proposed two-stage learning. In the first stage, they are assigning a score based on semantic data from the essay. The second stage scoring is based on some handcrafted features like grammar correction, essay length, number of sentences, etc. The average score of the two stages is 0.709.
Pedro Uria Rodriguez et al. ( 2019 ) proposed a sequence-to-sequence learning model for automatic essay scoring. They used BERT (Bidirectional Encoder Representations from Transformers), which extracts the semantics from a sentence from both directions. And XLnet sequence to sequence learning model to extract features like the next sentence in an essay. With this pre-trained model, they attained coherence from the essay to give the final score. The average QWK score of the model is 75.5.
Xia et al. ( 2019 ) proposed a two-layer Bi-directional LSTM neural network for the scoring of essays. The features extracted with word2vec to train the LSTM and accuracy of the model in an average of QWK is 0.870.
Kumar et al. ( 2019 ) Proposed an AutoSAS for short answer scoring. It used pre-trained Word2Vec and Doc2Vec models trained on Google News corpus and Wikipedia dump, respectively, to retrieve the features. First, they tagged every word POS and they found weighted words from the response. It also found prompt overlap to observe how the answer is relevant to the topic, and they defined lexical overlaps like noun overlap, argument overlap, and content overlap. This method used some statistical features like word frequency, difficulty, diversity, number of unique words in each response, type-token ratio, statistics of the sentence, word length, and logical operator-based features. This method uses a random forest model to train the dataset. The data set has sample responses with their associated score. The model will retrieve the features from both responses like graded and ungraded short answers with questions. The accuracy of AutoSAS with QWK is 0.78. It will work on any topics like Science, Arts, Biology, and English.
Jiaqi Lun et al. ( 2020 ) proposed an automatic short answer scoring with BERT. In this with a reference answer comparing student responses and assigning scores. The data augmentation is done with a neural network and with one correct answer from the dataset classifying reaming responses as correct or incorrect.
Zhu and Sun ( 2020 ) proposed a multimodal Machine Learning approach for automated essay scoring. First, they count the grammar score with the spaCy library and numerical count as the number of words and sentences with the same library. With this input, they trained a single and Bi LSTM neural network for finding the final score. For the LSTM model, they prepared sentence vectors with GloVe and word embedding with NLTK. Bi-LSTM will check each sentence in both directions to find semantic from the essay. The average QWK score with multiple models is 0.70.
Ontology based approach
Mohler et al. ( 2011 ) proposed a graph-based method to find semantic similarity in short answer scoring. For the ranking of answers, they used the support vector regression model. The bag of words is the main feature extracted in the system.
Ramachandran et al. ( 2015 ) also proposed a graph-based approach to find lexical based semantics. Identified phrase patterns and text patterns are the features to train a random forest regression model to score the essays. The accuracy of the model in a QWK is 0.78.
Zupanc et al. ( 2017 ) proposed sentence similarity networks to find the essay's score. Ajetunmobi and Daramola ( 2017 ) recommended an ontology-based information extraction approach and domain-based ontology to find the score.
Speech response scoring
Automatic scoring is in two ways one is text-based scoring, other is speech-based scoring. This paper discussed text-based scoring and its challenges, and now we cover speech scoring and common points between text and speech-based scoring. Evanini and Wang ( 2013 ), Worked on speech scoring of non-native school students, extracted features with speech ratter, and trained a linear regression model, concluding that accuracy varies based on voice pitching. Loukina et al. ( 2015 ) worked on feature selection from speech data and trained SVM. Malinin et al. ( 2016 ) used neural network models to train the data. Loukina et al. ( 2017 ). Proposed speech and text-based automatic scoring. Extracted text-based features, speech-based features and trained a deep neural network for speech-based scoring. They extracted 33 types of features based on acoustic signals. Malinin et al. ( 2017 ). Wu Xixin et al. ( 2020 ) Worked on deep neural networks for spoken language assessment. Incorporated different types of models and tested them. Ramanarayanan et al. ( 2017 ) worked on feature extraction methods and extracted punctuation, fluency, and stress and trained different Machine Learning models for scoring. Knill et al. ( 2018 ). Worked on Automatic speech recognizer and its errors how its impacts the speech assessment.
The state of the art
This section provides an overview of the existing AES systems with a comparative study w. r. t models, features applied, datasets, and evaluation metrics used for building the automated essay grading systems. We divided all 62 papers into two sets of the first set of review papers in Table Table5 5 with a comparative study of the AES systems.
State of the art
System | Approach | Dataset | Features applied | Evaluation metric and results |
---|---|---|---|---|
Mohler and Mihalcea in ( ) | shortest path similarity, LSA regression model | Word vector | Finds the shortest path | |
Niraj Kumar and Lipika Dey. In ( ) | Word-Graph | ASAP Kaggle | Content and style-based features | 63.81% accuracy |
Alex Adamson et al. in ( ) | LSA regression model | ASAP Kaggle | Statistical features | QWK 0.532 |
Nguyen and Dery ( ) | LSTM (single layer bidirectional) | ASAP Kaggle | Statistical features | 90% accuracy |
Keisuke Sakaguchi et al. in ( ) | Classification model | ETS (educational testing services) | Statistical, Style based features | QWK is 0.69 |
Ramachandran et al. in ( ) | regression model | ASAP Kaggle short Answer | Statistical and style-based features | QWK 0.77 |
Sultan et al. in ( ) | Ridge regression model | SciEntBank answers | Statistical features | RMSE 0.887 |
Dong and Zhang ( ) | CNN neural network | ASAP Kaggle | Statistical features | QWK 0.734 |
Taghipour and Ngl in ( ) | CNN + LSTM neural network | ASAP Kaggle | Lookup table (one hot representation of word vector) | QWK 0.761 |
Shehab et al. in ( ) | Learning vector quantization neural network | Mansoura University student's essays | Statistical features | correlation coefficient 0.7665 |
Cummins et al. in ( ) | Regression model | ASAP Kaggle | Statistical features, style-based features | QWK 0.69 |
Kopparapu and De ( ) | Neural network | ASAP Kaggle | Statistical features, Style based | |
Dong, et al. in ( ) | CNN + LSTM neural network | ASAP Kaggle | Word embedding, content based | QWK 0.764 |
Ajetunmobi and Daramola ( ) | WuPalmer algorithm | Statistical features | ||
Siyuan Zhao et al. in ( ) | LSTM (memory network) | ASAP Kaggle | Statistical features | QWK 0.78 |
Mathias and Bhattacharyya ( ) | Random Forest Classifier a classification model | ASAP Kaggle | Style and Content based features | Classified which feature set is required |
Brian Riordan et al. in ( ) | CNN + LSTM neural network | ASAP Kaggle short Answer | Word embeddings | QWK 0.90 |
Tirthankar Dasgupta et al. in ( ) | CNN -bidirectional LSTMs neural network | ASAP Kaggle | Content and physiological features | QWK 0.786 |
Wu and Shih ( ) | Classification model | SciEntBank answers | unigram_recall | Squared correlation coefficient 59.568 |
unigram_precision | ||||
unigram_F_measure | ||||
log_bleu_recall | ||||
log_bleu_precision | ||||
log_bleu_F_measure BLUE features | ||||
Yucheng Wang, etc.in ( ) | Bi-LSTM | ASAP Kaggle | Word embedding sequence | QWK 0.724 |
Anak Agung Putri Ratna et al. in ( ) | Winnowing ALGORITHM | 86.86 accuracy | ||
Sharma and Jayagopi ( ) | Glove, LSTM neural network | ASAP Kaggle | Hand written essay images | QWK 0.69 |
Jennifer O. Contreras et al. in ( ) | OntoGen (SVM) Linear Regression | University of Benghazi data set | Statistical, style-based features | |
Mathias, Bhattacharyya ( ) | GloVe,LSTM neural network | ASAP Kaggle | Statistical features, style features | Predicted Goodness score for essay |
Stefan Ruseti, et al. in ( ) | BiGRU Siamese architecture | Amazon Mechanical Turk online research service. Collected summaries | Word embedding | Accuracy 55.2 |
Zining wang, et al. in ( ) | LSTM (semantic) HAN (hierarchical attention network) neural network | ASAP Kaggle | Word embedding | QWK 0.83 |
Guoxi Liang et al. ( ) | Bi-LSTM | ASAP Kaggle | Word embedding, coherence of sentence | QWK 0.801 |
Ke et al. in ( ) | Classification model | ASAP Kaggle | Content based | Pearson’s Correlation Coefficient (PC)-0.39 ME-0.921 |
Tsegaye Misikir Tashu and Horváth in ( ) | Unsupervised learning–Locality Sensitivity Hashing | ASAP Kaggle | Statistical features | root mean squared error |
Kumar and Dey ( ) | Random Forest CNN, RNN neural network | ASAP Kaggle short Answer | Style and content-based features | QWK 0.82 |
Pedro Uria Rodriguez et al. ( ) | BERT, Xlnet | ASAP Kaggle | Error correction, sequence learning | QWK 0.755 |
Jiawei Liu et al. ( ) | CNN, LSTM, BERT | ASAP Kaggle | semantic data, handcrafted features like grammar correction, essay length, number of sentences, etc | QWK 0.709 |
Darwish and Mohamed ( ) | Multiple Linear Regression | ASAP Kaggle | Style and content-based features | QWK 0.77 |
Jiaqi Lun et al. ( ) | BERT | SemEval-2013 | Student Answer, Reference Answer | Accuracy 0.8277 (2-way) |
Süzen, Neslihan, et al. ( ) | Text mining | introductory computer science class in the University of North Texas, Student Assignments | Sentence similarity | Correlation score 0.81 |
Wilson Zhu and Yu Sun in ( ) | RNN (LSTM, Bi-LSTM) | ASAP Kaggle | Word embedding, grammar count, word count | QWK 0.70 |
Salim Yafet et al. ( ) | XGBoost machine learning classifier | ASAP Kaggle | Word count, POS, parse tree, coherence, cohesion, type token ration | Accuracy 68.12 |
Andrzej Cader ( ) | Deep Neural Network | University of Social Sciences in Lodz students’ answers | asynchronous feature | Accuracy 0.99 |
Tashu TM, Horváth T ( ) | Rule based algorithm, Similarity based algorithm | ASAP Kaggle | Similarity based | Accuracy 0.68 |
Masaki Uto(B) and Masashi Okano ( ) | Item Response Theory Models (CNN-LSTM,BERT) | ASAP Kaggle | QWK 0.749 |
Comparison of all approaches
In our study, we divided major AES approaches into three categories. Regression models, classification models, and neural network models. The regression models failed to find cohesion and coherence from the essay because it trained on BoW(Bag of Words) features. In processing data from input to output, the regression models are less complicated than neural networks. There are unable to find many intricate patterns from the essay and unable to find sentence connectivity. If we train the model with BoW features in the neural network approach, the model never considers the essay's coherence and coherence.
First, to train a Machine Learning algorithm with essays, all the essays are converted to vector form. We can form a vector with BoW and Word2vec, TF-IDF. The BoW and Word2vec vector representation of essays represented in Table Table6. 6 . The vector representation of BoW with TF-IDF is not incorporating the essays semantic, and it’s just statistical learning from a given vector. Word2vec vector comprises semantic of essay in a unidirectional way.
Vector representation of essays
Essay | BoW << vector >> | Word2vec << vector >> | |
---|---|---|---|
Student 1 response | I believe that using computers will benefit us in many ways like talking and becoming friends will others through websites like facebook and mysace | << 0.00000 0.00000 0.165746 0.280633 … 0.00000 0.280633 0.280633 0.280633 >> | << 3.9792988e-03 − 1.9810481e-03 1.9830784e-03 9.0381579e-04 − 2.9438005e-03 2.1778699e-03 4.4950014e-03 2.9508960e -03 − 2.2331756e-03 − 3.8774475e-03 3.5967759e- 03 − 4.0194849e-03 − 3.0412588e-03 − 2.4055617e-03 4.8296354e-03 2.4813593e-03… − 2.7158875e-03 − 1.4563646e-03 1.4072991e-03 − 5.2228488e-04 − 2.3597316e-03 6.2979700e-04 − 3.0249553e-03 4.4125126e-04 2.1633594e-03 − 4.9487003e-03 9.9755758e-05 − 2.4388896e-03 >> |
Student 2 response | More and more people use computers, but not everyone agrees that this benefits society. Those who support advances in technology believe that computers have a positive effect on people | << 0.26043 0.26043 0.153814 0.000000 … 0.26043 0.000000 0.000000 0.000000 > > | << 3.9792988e-03 − 1.9810481e- 03 1.9830784e-03 9.0381579e-04 − 2.9438005e-03 2.1778699e-03 4.4950014e-03 2.9508960e-03 − 2.2331756e-03 − 3.8774475e-03 3.5967759e-03 − 4.0194849e-03… − 2.7158875e-03 − 1.4563646e-03 1.4072991e-03 − 5.2228488e-04 − 2.3597316e-03 6.2979700e-04 − 3.0249553e-03 4.4125126e-04 3.7868773e-03 − 4.4193151e-03 3.0735810e-03 2.5546195e-03 2.1633594e-03 − 4.9487003e-03 9.9755758e-05 − 2.4388896e-03 >> |
In BoW, the vector contains the frequency of word occurrences in the essay. The vector represents 1 and more based on the happenings of words in the essay and 0 for not present. So, in BoW, the vector does not maintain the relationship with adjacent words; it’s just for single words. In word2vec, the vector represents the relationship between words with other words and sentences prompt in multiple dimensional ways. But word2vec prepares vectors in a unidirectional way, not in a bidirectional way; word2vec fails to find semantic vectors when a word has two meanings, and the meaning depends on adjacent words. Table Table7 7 represents a comparison of Machine Learning models and features extracting methods.
Comparison of models
BoW | Word2vec | |
---|---|---|
Regression models/classification models | The system implemented with Bow features and regression or classification algorithms will have low cohesion and coherence | The system implemented with Word2vec features and regression or classification algorithms will have low to medium cohesion and coherence |
Neural Networks (LSTM) | The system implemented with BoW features and neural network models will have low cohesion and coherence | The system implemented with Word2vec features and neural network model (LSTM) will have medium to high cohesion and coherence |
In AES, cohesion and coherence will check the content of the essay concerning the essay prompt these can be extracted from essay in the vector from. Two more parameters are there to access an essay is completeness and feedback. Completeness will check whether student’s response is sufficient or not though the student wrote correctly. Table Table8 8 represents all four parameters comparison for essay grading. Table Table9 9 illustrates comparison of all approaches based on various features like grammar, spelling, organization of essay, relevance.
Comparison of all models with respect to cohesion, coherence, completeness, feedback
Authors | Cohesion | Coherence | Completeness | Feed Back |
---|---|---|---|---|
Mohler and Mihalcea ( ) | Low | Low | Low | Low |
Mohler et al. ( ) | Medium | Low | Medium | Low |
Persing and Ng ( ) | Medium | Low | Low | Low |
Adamson et al. ( ) | Low | Low | Low | Low |
Ramachandran et al. ( ) | Medium | Medium | Low | Low |
Sakaguchi et al.. ( ), | Medium | Low | Low | Low |
Cummins et al. ( ) | Low | Low | Low | Low |
Sultan et al. ( ) | Medium | Medium | Low | Low |
Shehab et al. ( ) | Low | Low | Low | Low |
Kopparapu and De ( ) | Medium | Medium | Low | Low |
Dong an Zhang ( ) | Medium | Low | Low | Low |
Taghipour and Ng ( ) | Medium | Medium | Low | Low |
Zupanc et al. ( ) | Medium | Medium | Low | Low |
Dong et al. ( ) | Medium | Medium | Low | Low |
Riordan et al. ( ) | Medium | Medium | Medium | Low |
Zhao et al. ( ) | Medium | Medium | Low | Low |
Contreras et al. ( ) | Medium | Low | Low | Low |
Mathias and Bhattacharyya ( ; ) | Medium | Medium | Low | Low |
Mathias and Bhattacharyya ( ; ) | Medium | Medium | Low | Low |
Nguyen and Dery ( ) | Medium | Medium | Medium | Medium |
Ruseti et al. ( ) | Medium | Low | Low | Low |
Dasgupta et al. ( ) | Medium | Medium | Low | Low |
Liu et al.( ) | Low | Low | Low | Low |
Wang et al. ( ) | Medium | Low | Low | Low |
Guoxi Liang et al. ( ) | High | High | Low | Low |
Wang et al. ( ) | Medium | Medium | Low | Low |
Chen and Li ( ) | Medium | Medium | Low | Low |
Li et al. ( ) | Medium | Medium | Low | Low |
Alva-Manchego et al.( ) | Low | Low | Low | Low |
Jiawei Liu et al. ( ) | High | High | Medium | Low |
Pedro Uria Rodriguez et al. ( ) | Medium | Medium | Medium | Low |
Changzhi Cai( ) | Low | Low | Low | Low |
Xia et al. ( ) | Medium | Medium | Low | Low |
Chen and Zhou ( ) | Low | Low | Low | Low |
Kumar et al. ( ) | Medium | Medium | Medium | Low |
Ke et al. ( ) | Medium | Low | Medium | Low |
Andrzej Cader( ) | Low | Low | Low | Low |
Jiaqi Lun et al. ( ) | High | High | Low | Low |
Wilson Zhu and Yu Sun ( ) | Medium | Medium | Low | Low |
Süzen, Neslihan et al. ( ) | Medium | Low | Medium | Low |
Salim Yafet et al. ( ) | High | Medium | Low | Low |
Darwish and Mohamed ( ) | Medium | Low | Low | Low |
Tashu and Horváth ( ) | Medium | Medium | Low | Medium |
Tashu ( ) | Medium | Medium | Low | Low |
Masaki Uto(B) and Masashi Okano( ) | Medium | Medium | Medium | Medium |
Panitan Muangkammuen and Fumiyo Fukumoto( ) | Medium | Medium | Medium | Low |
comparison of all approaches on various features
Approaches | Grammar | Style (Word choice, sentence structure) | Mechanics (Spelling, punctuation, capitalization) | Development | BoW (tf-idf) | relevance |
---|---|---|---|---|---|---|
Mohler and Mihalcea ( ) | No | No | No | No | Yes | No |
Mohler et al. ( ) | Yes | No | No | No | Yes | No |
Persing and Ng ( ) | Yes | Yes | Yes | No | Yes | Yes |
Adamson et al. ( ) | Yes | No | Yes | No | Yes | No |
Ramachandran et al. ( ) | Yes | No | Yes | Yes | Yes | Yes |
Sakaguchi et al. ( ), | No | No | Yes | Yes | Yes | Yes |
Cummins et al. ( ) | Yes | No | Yes | No | Yes | No |
Sultan et al. ( ) | No | No | No | No | Yes | Yes |
Shehab et al. ( ) | Yes | Yes | Yes | No | Yes | No |
Kopparapu and De ( ) | No | No | No | No | Yes | No |
Dong and Zhang ( ) | Yes | No | Yes | No | Yes | Yes |
Taghipour and Ng ( ) | Yes | No | No | No | Yes | Yes |
Zupanc et al. ( ) | No | No | No | No | Yes | No |
Dong et al. ( ) | No | No | No | No | No | Yes |
Riordan et al. ( ) | No | No | No | No | No | Yes |
Zhao et al. ( ) | No | No | No | No | No | Yes |
Contreras et al. ( ) | Yes | No | No | No | Yes | Yes |
Mathias and Bhattacharyya ( , ) | No | Yes | Yes | No | No | Yes |
Mathias and Bhattacharyya ( , ) | Yes | No | Yes | No | Yes | Yes |
Nguyen and Dery ( ) | No | No | No | No | Yes | Yes |
Ruseti et al. ( ) | No | No | No | Yes | No | Yes |
Dasgupta et al. ( ) | Yes | Yes | Yes | Yes | No | Yes |
Liu et al.( ) | Yes | Yes | No | No | Yes | No |
Wang et al. ( ) | No | No | No | No | No | Yes |
Guoxi Liang et al. ( ) | No | No | No | No | No | Yes |
Wang et al. ( ) | No | No | No | No | No | Yes |
Chen and Li ( ) | No | No | No | No | No | Yes |
Li et al. ( ) | Yes | No | No | No | No | Yes |
Alva-Manchego et al. ( ) | Yes | No | No | Yes | No | Yes |
Jiawei Liu et al. ( ) | Yes | No | No | Yes | No | Yes |
Pedro Uria Rodriguez et al. ( ) | No | No | No | No | Yes | Yes |
Changzhi Cai( ) | No | No | No | No | No | Yes |
Xia et al. ( ) | No | No | No | No | No | Yes |
Chen and Zhou ( ) | No | No | No | No | No | Yes |
Kumar et al. ( ) | Yes | Yes | No | Yes | Yes | Yes |
Ke et al. ( ) | No | Yes | No | Yes | Yes | Yes |
Andrzej Cader( ) | No | No | No | No | No | Yes |
Jiaqi Lun et al. ( ) | No | No | No | No | No | Yes |
Wilson Zhu and Yu Sun ( ) | No | No | No | No | No | Yes |
Süzen, Neslihan, et al. ( ) | No | No | No | No | Yes | Yes |
Salim Yafet et al. ( ) | Yes | Yes | Yes | No | Yes | Yes |
Darwish and Mohamed ( ) | Yes | Yes | No | No | No | Yes |
What are the challenges/limitations in the current research?
From our study and results discussed in the previous sections, many researchers worked on automated essay scoring systems with numerous techniques. We have statistical methods, classification methods, and neural network approaches to evaluate the essay automatically. The main goal of the automated essay grading system is to reduce human effort and improve consistency.
The vast majority of essay scoring systems are dealing with the efficiency of the algorithm. But there are many challenges in automated essay grading systems. One should assess the essay by following parameters like the relevance of the content to the prompt, development of ideas, Cohesion, Coherence, and domain knowledge.
No model works on the relevance of content, which means whether student response or explanation is relevant to the given prompt or not if it is relevant to how much it is appropriate, and there is no discussion about the cohesion and coherence of the essays. All researches concentrated on extracting the features using some NLP libraries, trained their models, and testing the results. But there is no explanation in the essay evaluation system about consistency and completeness, But Palma and Atkinson ( 2018 ) explained coherence-based essay evaluation. And Zupanc and Bosnic ( 2014 ) also used the word coherence to evaluate essays. And they found consistency with latent semantic analysis (LSA) for finding coherence from essays, but the dictionary meaning of coherence is "The quality of being logical and consistent."
Another limitation is there is no domain knowledge-based evaluation of essays using Machine Learning models. For example, the meaning of a cell is different from biology to physics. Many Machine Learning models extract features with WordVec and GloVec; these NLP libraries cannot convert the words into vectors when they have two or more meanings.
Other challenges that influence the Automated Essay Scoring Systems.
All these approaches worked to improve the QWK score of their models. But QWK will not assess the model in terms of features extraction and constructed irrelevant answers. The QWK is not evaluating models whether the model is correctly assessing the answer or not. There are many challenges concerning students' responses to the Automatic scoring system. Like in evaluating approach, no model has examined how to evaluate the constructed irrelevant and adversarial answers. Especially the black box type of approaches like deep learning models provides more options to the students to bluff the automated scoring systems.
The Machine Learning models that work on statistical features are very vulnerable. Based on Powers et al. ( 2001 ) and Bejar Isaac et al. ( 2014 ), the E-rater was failed on Constructed Irrelevant Responses Strategy (CIRS). From the study of Bejar et al. ( 2013 ), Higgins and Heilman ( 2014 ), observed that when student response contain irrelevant content or shell language concurring to prompt will influence the final score of essays in an automated scoring system.
In deep learning approaches, most of the models automatically read the essay's features, and some methods work on word-based embedding and other character-based embedding features. From the study of Riordan Brain et al. ( 2019 ), The character-based embedding systems do not prioritize spelling correction. However, it is influencing the final score of the essay. From the study of Horbach and Zesch ( 2019 ), Various factors are influencing AES systems. For example, there are data set size, prompt type, answer length, training set, and human scorers for content-based scoring.
Ding et al. ( 2020 ) reviewed that the automated scoring system is vulnerable when a student response contains more words from prompt, like prompt vocabulary repeated in the response. Parekh et al. ( 2020 ) and Kumar et al. ( 2020 ) tested various neural network models of AES by iteratively adding important words, deleting unimportant words, shuffle the words, and repeating sentences in an essay and found that no change in the final score of essays. These neural network models failed to recognize common sense in adversaries' essays and give more options for the students to bluff the automated systems.
Other than NLP and ML techniques for AES. From Wresch ( 1993 ) to Madnani and Cahill ( 2018 ). discussed the complexity of AES systems, standards need to be followed. Like assessment rubrics to test subject knowledge, irrelevant responses, and ethical aspects of an algorithm like measuring the fairness of student response.
Fairness is an essential factor for automated systems. For example, in AES, fairness can be measure in an agreement between human score to machine score. Besides this, From Loukina et al. ( 2019 ), the fairness standards include overall score accuracy, overall score differences, and condition score differences between human and system scores. In addition, scoring different responses in the prospect of constructive relevant and irrelevant will improve fairness.
Madnani et al. ( 2017a ; b ). Discussed the fairness of AES systems for constructed responses and presented RMS open-source tool for detecting biases in the models. With this, one can change fairness standards according to their analysis of fairness.
From Berzak et al.'s ( 2018 ) approach, behavior factors are a significant challenge in automated scoring systems. That helps to find language proficiency, word characteristics (essential words from the text), predict the critical patterns from the text, find related sentences in an essay, and give a more accurate score.
Rupp ( 2018 ), has discussed the designing, evaluating, and deployment methodologies for AES systems. They provided notable characteristics of AES systems for deployment. They are like model performance, evaluation metrics for a model, threshold values, dynamically updated models, and framework.
First, we should check the model performance on different datasets and parameters for operational deployment. Selecting Evaluation metrics for AES models are like QWK, correlation coefficient, or sometimes both. Kelley and Preacher ( 2012 ) have discussed three categories of threshold values: marginal, borderline, and acceptable. The values can be varied based on data size, model performance, type of model (single scoring, multiple scoring models). Once a model is deployed and evaluates millions of responses every time for optimal responses, we need a dynamically updated model based on prompt and data. Finally, framework designing of AES model, hear a framework contains prompts where test-takers can write the responses. One can design two frameworks: a single scoring model for a single methodology and multiple scoring models for multiple concepts. When we deploy multiple scoring models, each prompt could be trained separately, or we can provide generalized models for all prompts with this accuracy may vary, and it is challenging.
Our Systematic literature review on the automated essay grading system first collected 542 papers with selected keywords from various databases. After inclusion and exclusion criteria, we left with 139 articles; on these selected papers, we applied Quality assessment criteria with two reviewers, and finally, we selected 62 writings for final review.
Our observations on automated essay grading systems from 2010 to 2020 are as followed:
- The implementation techniques of automated essay grading systems are classified into four buckets; there are 1. regression models 2. Classification models 3. Neural networks 4. Ontology-based methodology, but using neural networks, the researchers are more accurate than other techniques, and all the methods state of the art provided in Table Table3 3 .
- The majority of the regression and classification models on essay scoring used statistical features to find the final score. It means the systems or models trained on such parameters as word count, sentence count, etc. though the parameters extracted from the essay, the algorithm are not directly training on essays. The algorithms trained on some numbers obtained from the essay and hear if numbers matched the composition will get a good score; otherwise, the rating is less. In these models, the evaluation process is entirely on numbers, irrespective of the essay. So, there is a lot of chance to miss the coherence, relevance of the essay if we train our algorithm on statistical parameters.
- In the neural network approach, the models trained on Bag of Words (BoW) features. The BoW feature is missing the relationship between a word to word and the semantic meaning of the sentence. E.g., Sentence 1: John killed bob. Sentence 2: bob killed John. In these two sentences, the BoW is "John," "killed," "bob."
- In the Word2Vec library, if we are prepared a word vector from an essay in a unidirectional way, the vector will have a dependency with other words and finds the semantic relationship with other words. But if a word has two or more meanings like "Bank loan" and "River Bank," hear bank has two implications, and its adjacent words decide the sentence meaning; in this case, Word2Vec is not finding the real meaning of the word from the sentence.
- The features extracted from essays in the essay scoring system are classified into 3 type's features like statistical features, style-based features, and content-based features, which are explained in RQ2 and Table Table3. 3 . But statistical features, are playing a significant role in some systems and negligible in some systems. In Shehab et al. ( 2016 ); Cummins et al. ( 2016 ). Dong et al. ( 2017 ). Dong and Zhang ( 2016 ). Mathias and Bhattacharyya ( 2018a ; b ) Systems the assessment is entirely on statistical and style-based features they have not retrieved any content-based features. And in other systems that extract content from the essays, the role of statistical features is for only preprocessing essays but not included in the final grading.
- In AES systems, coherence is the main feature to be considered while evaluating essays. The actual meaning of coherence is to stick together. That is the logical connection of sentences (local level coherence) and paragraphs (global level coherence) in a story. Without coherence, all sentences in a paragraph are independent and meaningless. In an Essay, coherence is a significant feature that is explaining everything in a flow and its meaning. It is a powerful feature in AES system to find the semantics of essay. With coherence, one can assess whether all sentences are connected in a flow and all paragraphs are related to justify the prompt. Retrieving the coherence level from an essay is a critical task for all researchers in AES systems.
- In automatic essay grading systems, the assessment of essays concerning content is critical. That will give the actual score for the student. Most of the researches used statistical features like sentence length, word count, number of sentences, etc. But according to collected results, 32% of the systems used content-based features for the essay scoring. Example papers which are on content-based assessment are Taghipour and Ng ( 2016 ); Persing and Ng ( 2013 ); Wang et al. ( 2018a , 2018b ); Zhao et al. ( 2017 ); Kopparapu and De ( 2016 ), Kumar et al. ( 2019 ); Mathias and Bhattacharyya ( 2018a ; b ); Mohler and Mihalcea ( 2009 ) are used content and statistical-based features. The results are shown in Fig. Fig.3. 3 . And mainly the content-based features extracted with word2vec NLP library, but word2vec is capable of capturing the context of a word in a document, semantic and syntactic similarity, relation with other terms, but word2vec is capable of capturing the context word in a uni-direction either left or right. If a word has multiple meanings, there is a chance of missing the context in the essay. After analyzing all the papers, we found that content-based assessment is a qualitative assessment of essays.
- On the other hand, Horbach and Zesch ( 2019 ); Riordan Brain et al. ( 2019 ); Ding et al. ( 2020 ); Kumar et al. ( 2020 ) proved that neural network models are vulnerable when a student response contains constructed irrelevant, adversarial answers. And a student can easily bluff an automated scoring system by submitting different responses like repeating sentences and repeating prompt words in an essay. From Loukina et al. ( 2019 ), and Madnani et al. ( 2017b ). The fairness of an algorithm is an essential factor to be considered in AES systems.
- While talking about speech assessment, the data set contains audios of duration up to one minute. Feature extraction techniques are entirely different from text assessment, and accuracy varies based on speaking fluency, pitching, male to female voice and boy to adult voice. But the training algorithms are the same for text and speech assessment.
- Once an AES system evaluates essays and short answers accurately in all directions, there is a massive demand for automated systems in the educational and related world. Now AES systems are deployed in GRE, TOEFL exams; other than these, we can deploy AES systems in massive open online courses like Coursera(“ https://coursera.org/learn//machine-learning//exam ”), NPTEL ( https://swayam.gov.in/explorer ), etc. still they are assessing student performance with multiple-choice questions. In another perspective, AES systems can be deployed in information retrieval systems like Quora, stack overflow, etc., to check whether the retrieved response is appropriate to the question or not and can give ranking to the retrieved answers.
Conclusion and future work
As per our Systematic literature review, we studied 62 papers. There exist significant challenges for researchers in implementing automated essay grading systems. Several researchers are working rigorously on building a robust AES system despite its difficulty in solving this problem. All evaluating methods are not evaluated based on coherence, relevance, completeness, feedback, and knowledge-based. And 90% of essay grading systems are used Kaggle ASAP (2012) dataset, which has general essays from students and not required any domain knowledge, so there is a need for domain-specific essay datasets to train and test. Feature extraction is with NLTK, WordVec, and GloVec NLP libraries; these libraries have many limitations while converting a sentence into vector form. Apart from feature extraction and training Machine Learning models, no system is accessing the essay's completeness. No system provides feedback to the student response and not retrieving coherence vectors from the essay—another perspective the constructive irrelevant and adversarial student responses still questioning AES systems.
Our proposed research work will go on the content-based assessment of essays with domain knowledge and find a score for the essays with internal and external consistency. And we will create a new dataset concerning one domain. And another area in which we can improve is the feature extraction techniques.
This study includes only four digital databases for study selection may miss some functional studies on the topic. However, we hope that we covered most of the significant studies as we manually collected some papers published in useful journals.
Below is the link to the electronic supplementary material.
Not Applicable.
Publisher's Note
Springer Nature remains neutral with regard to jurisdictional claims in published maps and institutional affiliations.
Contributor Information
Dadi Ramesh, Email: moc.liamg@44hsemaridad .
Suresh Kumar Sanampudi, Email: ni.ca.hutnj@idupmanashserus .
- Adamson, A., Lamb, A., & December, R. M. (2014). Automated Essay Grading.
- Ajay HB, Tillett PI, Page EB (1973) Analysis of essays by computer (AEC-II) (No. 8-0102). Washington, DC: U.S. Department of Health, Education, and Welfare, Office of Education, National Center for Educational Research and Development
- Ajetunmobi SA, Daramola O (2017) Ontology-based information extraction for subject-focussed automatic essay evaluation. In: 2017 International Conference on Computing Networking and Informatics (ICCNI) p 1–6. IEEE
- Alva-Manchego F, et al. (2019) EASSE: Easier Automatic Sentence Simplification Evaluation.” ArXiv abs/1908.04567 (2019): n. pag
- Bailey S, Meurers D (2008) Diagnosing meaning errors in short answers to reading comprehension questions. In: Proceedings of the Third Workshop on Innovative Use of NLP for Building Educational Applications (Columbus), p 107–115
- Basu S, Jacobs C, Vanderwende L. Powergrading: a clustering approach to amplify human effort for short answer grading. Trans Assoc Comput Linguist (TACL) 2013; 1 :391–402. doi: 10.1162/tacl_a_00236. [ CrossRef ] [ Google Scholar ]
- Bejar, I. I., Flor, M., Futagi, Y., & Ramineni, C. (2014). On the vulnerability of automated scoring to construct-irrelevant response strategies (CIRS): An illustration. Assessing Writing, 22, 48-59.
- Bejar I, et al. (2013) Length of Textual Response as a Construct-Irrelevant Response Strategy: The Case of Shell Language. Research Report. ETS RR-13-07.” ETS Research Report Series (2013): n. pag
- Berzak Y, et al. (2018) “Assessing Language Proficiency from Eye Movements in Reading.” ArXiv abs/1804.07329 (2018): n. pag
- Blanchard D, Tetreault J, Higgins D, Cahill A, Chodorow M (2013) TOEFL11: A corpus of non-native English. ETS Research Report Series, 2013(2):i–15, 2013
- Blood, I. (2011). Automated essay scoring: a literature review. Studies in Applied Linguistics and TESOL, 11(2).
- Burrows S, Gurevych I, Stein B. The eras and trends of automatic short answer grading. Int J Artif Intell Educ. 2015; 25 :60–117. doi: 10.1007/s40593-014-0026-8. [ CrossRef ] [ Google Scholar ]
- Cader, A. (2020, July). The Potential for the Use of Deep Neural Networks in e-Learning Student Evaluation with New Data Augmentation Method. In International Conference on Artificial Intelligence in Education (pp. 37–42). Springer, Cham.
- Cai C (2019) Automatic essay scoring with recurrent neural network. In: Proceedings of the 3rd International Conference on High Performance Compilation, Computing and Communications (2019): n. pag.
- Chen M, Li X (2018) "Relevance-Based Automated Essay Scoring via Hierarchical Recurrent Model. In: 2018 International Conference on Asian Language Processing (IALP), Bandung, Indonesia, 2018, p 378–383, doi: 10.1109/IALP.2018.8629256
- Chen Z, Zhou Y (2019) "Research on Automatic Essay Scoring of Composition Based on CNN and OR. In: 2019 2nd International Conference on Artificial Intelligence and Big Data (ICAIBD), Chengdu, China, p 13–18, doi: 10.1109/ICAIBD.2019.8837007
- Contreras JO, Hilles SM, Abubakar ZB (2018) Automated essay scoring with ontology based on text mining and NLTK tools. In: 2018 International Conference on Smart Computing and Electronic Enterprise (ICSCEE), 1-6
- Correnti R, Matsumura LC, Hamilton L, Wang E. Assessing students’ skills at writing analytically in response to texts. Elem Sch J. 2013; 114 (2):142–177. doi: 10.1086/671936. [ CrossRef ] [ Google Scholar ]
- Cummins, R., Zhang, M., & Briscoe, E. (2016, August). Constrained multi-task learning for automated essay scoring. Association for Computational Linguistics.
- Darwish SM, Mohamed SK (2020) Automated essay evaluation based on fusion of fuzzy ontology and latent semantic analysis. In: Hassanien A, Azar A, Gaber T, Bhatnagar RF, Tolba M (eds) The International Conference on Advanced Machine Learning Technologies and Applications
- Dasgupta T, Naskar A, Dey L, Saha R (2018) Augmenting textual qualitative features in deep convolution recurrent neural network for automatic essay scoring. In: Proceedings of the 5th Workshop on Natural Language Processing Techniques for Educational Applications p 93–102
- Ding Y, et al. (2020) "Don’t take “nswvtnvakgxpm” for an answer–The surprising vulnerability of automatic content scoring systems to adversarial input." In: Proceedings of the 28th International Conference on Computational Linguistics
- Dong F, Zhang Y (2016) Automatic features for essay scoring–an empirical study. In: Proceedings of the 2016 Conference on Empirical Methods in Natural Language Processing p 1072–1077
- Dong F, Zhang Y, Yang J (2017) Attention-based recurrent convolutional neural network for automatic essay scoring. In: Proceedings of the 21st Conference on Computational Natural Language Learning (CoNLL 2017) p 153–162
- Dzikovska M, Nielsen R, Brew C, Leacock C, Gi ampiccolo D, Bentivogli L, Clark P, Dagan I, Dang HT (2013a) Semeval-2013 task 7: The joint student response analysis and 8th recognizing textual entailment challenge
- Dzikovska MO, Nielsen R, Brew C, Leacock C, Giampiccolo D, Bentivogli L, Clark P, Dagan I, Trang Dang H (2013b) SemEval-2013 Task 7: The Joint Student Response Analysis and 8th Recognizing Textual Entailment Challenge. *SEM 2013: The First Joint Conference on Lexical and Computational Semantics
- Educational Testing Service (2008) CriterionSM online writing evaluation service. Retrieved from http://www.ets.org/s/criterion/pdf/9286_CriterionBrochure.pdf .
- Evanini, K., & Wang, X. (2013, August). Automated speech scoring for non-native middle school students with multiple task types. In INTERSPEECH (pp. 2435–2439).
- Foltz PW, Laham D, Landauer TK (1999) The Intelligent Essay Assessor: Applications to Educational Technology. Interactive Multimedia Electronic Journal of Computer-Enhanced Learning, 1, 2, http://imej.wfu.edu/articles/1999/2/04/ index.asp
- Granger, S., Dagneaux, E., Meunier, F., & Paquot, M. (Eds.). (2009). International corpus of learner English. Louvain-la-Neuve: Presses universitaires de Louvain.
- Higgins D, Heilman M. Managing what we can measure: quantifying the susceptibility of automated scoring systems to gaming behavior” Educ Meas Issues Pract. 2014; 33 :36–46. doi: 10.1111/emip.12036. [ CrossRef ] [ Google Scholar ]
- Horbach A, Zesch T. The influence of variance in learner answers on automatic content scoring. Front Educ. 2019; 4 :28. doi: 10.3389/feduc.2019.00028. [ CrossRef ] [ Google Scholar ]
- https://www.coursera.org/learn/machine-learning/exam/7pytE/linear-regression-with-multiple-variables/attempt
- Hussein, M. A., Hassan, H., & Nassef, M. (2019). Automated language essay scoring systems: A literature review. PeerJ Computer Science, 5, e208. [ PMC free article ] [ PubMed ]
- Ke Z, Ng V (2019) “Automated essay scoring: a survey of the state of the art.” IJCAI
- Ke, Z., Inamdar, H., Lin, H., & Ng, V. (2019, July). Give me more feedback II: Annotating thesis strength and related attributes in student essays. In Proceedings of the 57th Annual Meeting of the Association for Computational Linguistics (pp. 3994-4004).
- Kelley K, Preacher KJ. On effect size. Psychol Methods. 2012; 17 (2):137–152. doi: 10.1037/a0028086. [ PubMed ] [ CrossRef ] [ Google Scholar ]
- Kitchenham B, Brereton OP, Budgen D, Turner M, Bailey J, Linkman S. Systematic literature reviews in software engineering–a systematic literature review. Inf Softw Technol. 2009; 51 (1):7–15. doi: 10.1016/j.infsof.2008.09.009. [ CrossRef ] [ Google Scholar ]
- Klebanov, B. B., & Madnani, N. (2020, July). Automated evaluation of writing–50 years and counting. In Proceedings of the 58th Annual Meeting of the Association for Computational Linguistics (pp. 7796–7810).
- Knill K, Gales M, Kyriakopoulos K, et al. (4 more authors) (2018) Impact of ASR performance on free speaking language assessment. In: Interspeech 2018.02–06 Sep 2018, Hyderabad, India. International Speech Communication Association (ISCA)
- Kopparapu SK, De A (2016) Automatic ranking of essays using structural and semantic features. In: 2016 International Conference on Advances in Computing, Communications and Informatics (ICACCI), p 519–523
- Kumar, Y., Aggarwal, S., Mahata, D., Shah, R. R., Kumaraguru, P., & Zimmermann, R. (2019, July). Get it scored using autosas—an automated system for scoring short answers. In Proceedings of the AAAI Conference on Artificial Intelligence (Vol. 33, No. 01, pp. 9662–9669).
- Kumar Y, et al. (2020) “Calling out bluff: attacking the robustness of automatic scoring systems with simple adversarial testing.” ArXiv abs/2007.06796
- Li X, Chen M, Nie J, Liu Z, Feng Z, Cai Y (2018) Coherence-Based Automated Essay Scoring Using Self-attention. In: Sun M, Liu T, Wang X, Liu Z, Liu Y (eds) Chinese Computational Linguistics and Natural Language Processing Based on Naturally Annotated Big Data. CCL 2018, NLP-NABD 2018. Lecture Notes in Computer Science, vol 11221. Springer, Cham. 10.1007/978-3-030-01716-3_32
- Liang G, On B, Jeong D, Kim H, Choi G. Automated essay scoring: a siamese bidirectional LSTM neural network architecture. Symmetry. 2018; 10 :682. doi: 10.3390/sym10120682. [ CrossRef ] [ Google Scholar ]
- Liua, H., Yeb, Y., & Wu, M. (2018, April). Ensemble Learning on Scoring Student Essay. In 2018 International Conference on Management and Education, Humanities and Social Sciences (MEHSS 2018). Atlantis Press.
- Liu J, Xu Y, Zhao L (2019) Automated Essay Scoring based on Two-Stage Learning. ArXiv, abs/1901.07744
- Loukina A, et al. (2015) Feature selection for automated speech scoring.” BEA@NAACL-HLT
- Loukina A, et al. (2017) “Speech- and Text-driven Features for Automated Scoring of English-Speaking Tasks.” SCNLP@EMNLP 2017
- Loukina A, et al. (2019) The many dimensions of algorithmic fairness in educational applications. BEA@ACL
- Lun J, Zhu J, Tang Y, Yang M (2020) Multiple data augmentation strategies for improving performance on automatic short answer scoring. In: Proceedings of the AAAI Conference on Artificial Intelligence, 34(09): 13389-13396
- Madnani, N., & Cahill, A. (2018, August). Automated scoring: Beyond natural language processing. In Proceedings of the 27th International Conference on Computational Linguistics (pp. 1099–1109).
- Madnani N, et al. (2017b) “Building better open-source tools to support fairness in automated scoring.” EthNLP@EACL
- Malinin A, et al. (2016) “Off-topic response detection for spontaneous spoken english assessment.” ACL
- Malinin A, et al. (2017) “Incorporating uncertainty into deep learning for spoken language assessment.” ACL
- Mathias S, Bhattacharyya P (2018a) Thank “Goodness”! A Way to Measure Style in Student Essays. In: Proceedings of the 5th Workshop on Natural Language Processing Techniques for Educational Applications p 35–41
- Mathias S, Bhattacharyya P (2018b) ASAP++: Enriching the ASAP automated essay grading dataset with essay attribute scores. In: Proceedings of the Eleventh International Conference on Language Resources and Evaluation (LREC 2018).
- Mikolov T, et al. (2013) “Efficient Estimation of Word Representations in Vector Space.” ICLR
- Mohler M, Mihalcea R (2009) Text-to-text semantic similarity for automatic short answer grading. In: Proceedings of the 12th Conference of the European Chapter of the ACL (EACL 2009) p 567–575
- Mohler M, Bunescu R, Mihalcea R (2011) Learning to grade short answer questions using semantic similarity measures and dependency graph alignments. In: Proceedings of the 49th annual meeting of the association for computational linguistics: Human language technologies p 752–762
- Muangkammuen P, Fukumoto F (2020) Multi-task Learning for Automated Essay Scoring with Sentiment Analysis. In: Proceedings of the 1st Conference of the Asia-Pacific Chapter of the Association for Computational Linguistics and the 10th International Joint Conference on Natural Language Processing: Student Research Workshop p 116–123
- Nguyen, H., & Dery, L. (2016). Neural networks for automated essay grading. CS224d Stanford Reports, 1–11.
- Palma D, Atkinson J. Coherence-based automatic essay assessment. IEEE Intell Syst. 2018; 33 (5):26–36. doi: 10.1109/MIS.2018.2877278. [ CrossRef ] [ Google Scholar ]
- Parekh S, et al (2020) My Teacher Thinks the World Is Flat! Interpreting Automatic Essay Scoring Mechanism.” ArXiv abs/2012.13872 (2020): n. pag
- Pennington, J., Socher, R., & Manning, C. D. (2014, October). Glove: Global vectors for word representation. In Proceedings of the 2014 conference on empirical methods in natural language processing (EMNLP) (pp. 1532–1543).
- Persing I, Ng V (2013) Modeling thesis clarity in student essays. In:Proceedings of the 51st Annual Meeting of the Association for Computational Linguistics (Volume 1: Long Papers) p 260–269
- Powers DE, Burstein JC, Chodorow M, Fowles ME, Kukich K. Stumping E-Rater: challenging the validity of automated essay scoring. ETS Res Rep Ser. 2001; 2001 (1):i–44. [ Google Scholar ]
- Powers DE, Burstein JC, Chodorow M, Fowles ME, Kukich K. Stumping e-rater: challenging the validity of automated essay scoring. Comput Hum Behav. 2002; 18 (2):103–134. doi: 10.1016/S0747-5632(01)00052-8. [ CrossRef ] [ Google Scholar ]
- Ramachandran L, Cheng J, Foltz P (2015) Identifying patterns for short answer scoring using graph-based lexico-semantic text matching. In: Proceedings of the Tenth Workshop on Innovative Use of NLP for Building Educational Applications p 97–106
- Ramanarayanan V, et al. (2017) “Human and Automated Scoring of Fluency, Pronunciation and Intonation During Human-Machine Spoken Dialog Interactions.” INTERSPEECH
- Riordan B, Horbach A, Cahill A, Zesch T, Lee C (2017) Investigating neural architectures for short answer scoring. In: Proceedings of the 12th Workshop on Innovative Use of NLP for Building Educational Applications p 159–168
- Riordan B, Flor M, Pugh R (2019) "How to account for misspellings: Quantifying the benefit of character representations in neural content scoring models."In: Proceedings of the Fourteenth Workshop on Innovative Use of NLP for Building Educational Applications
- Rodriguez P, Jafari A, Ormerod CM (2019) Language models and Automated Essay Scoring. ArXiv, abs/1909.09482
- Rudner, L. M., & Liang, T. (2002). Automated essay scoring using Bayes' theorem. The Journal of Technology, Learning and Assessment, 1(2).
- Rudner, L. M., Garcia, V., & Welch, C. (2006). An evaluation of IntelliMetric™ essay scoring system. The Journal of Technology, Learning and Assessment, 4(4).
- Rupp A. Designing, evaluating, and deploying automated scoring systems with validity in mind: methodological design decisions. Appl Meas Educ. 2018; 31 :191–214. doi: 10.1080/08957347.2018.1464448. [ CrossRef ] [ Google Scholar ]
- Ruseti S, Dascalu M, Johnson AM, McNamara DS, Balyan R, McCarthy KS, Trausan-Matu S (2018) Scoring summaries using recurrent neural networks. In: International Conference on Intelligent Tutoring Systems p 191–201. Springer, Cham
- Sakaguchi K, Heilman M, Madnani N (2015) Effective feature integration for automated short answer scoring. In: Proceedings of the 2015 conference of the North American Chapter of the association for computational linguistics: Human language technologies p 1049–1054
- Salim, Y., Stevanus, V., Barlian, E., Sari, A. C., & Suhartono, D. (2019, December). Automated English Digital Essay Grader Using Machine Learning. In 2019 IEEE International Conference on Engineering, Technology and Education (TALE) (pp. 1–6). IEEE.
- Shehab A, Elhoseny M, Hassanien AE (2016) A hybrid scheme for Automated Essay Grading based on LVQ and NLP techniques. In: 12th International Computer Engineering Conference (ICENCO), Cairo, 2016, p 65-70
- Shermis MD, Mzumara HR, Olson J, Harrington S. On-line grading of student essays: PEG goes on the World Wide Web. Assess Eval High Educ. 2001; 26 (3):247–259. doi: 10.1080/02602930120052404. [ CrossRef ] [ Google Scholar ]
- Stab C, Gurevych I (2014) Identifying argumentative discourse structures in persuasive essays. In: Proceedings of the 2014 Conference on Empirical Methods in Natural Language Processing (EMNLP) p 46–56
- Sultan MA, Salazar C, Sumner T (2016) Fast and easy short answer grading with high accuracy. In: Proceedings of the 2016 Conference of the North American Chapter of the Association for Computational Linguistics: Human Language Technologies p 1070–1075
- Süzen, N., Gorban, A. N., Levesley, J., & Mirkes, E. M. (2020). Automatic short answer grading and feedback using text mining methods. Procedia Computer Science, 169, 726–743.
- Taghipour K, Ng HT (2016) A neural approach to automated essay scoring. In: Proceedings of the 2016 conference on empirical methods in natural language processing p 1882–1891
- Tashu TM (2020) "Off-Topic Essay Detection Using C-BGRU Siamese. In: 2020 IEEE 14th International Conference on Semantic Computing (ICSC), San Diego, CA, USA, p 221–225, doi: 10.1109/ICSC.2020.00046
- Tashu TM, Horváth T (2019) A layered approach to automatic essay evaluation using word-embedding. In: McLaren B, Reilly R, Zvacek S, Uhomoibhi J (eds) Computer Supported Education. CSEDU 2018. Communications in Computer and Information Science, vol 1022. Springer, Cham
- Tashu TM, Horváth T (2020) Semantic-Based Feedback Recommendation for Automatic Essay Evaluation. In: Bi Y, Bhatia R, Kapoor S (eds) Intelligent Systems and Applications. IntelliSys 2019. Advances in Intelligent Systems and Computing, vol 1038. Springer, Cham
- Uto M, Okano M (2020) Robust Neural Automated Essay Scoring Using Item Response Theory. In: Bittencourt I, Cukurova M, Muldner K, Luckin R, Millán E (eds) Artificial Intelligence in Education. AIED 2020. Lecture Notes in Computer Science, vol 12163. Springer, Cham
- Wang Z, Liu J, Dong R (2018a) Intelligent Auto-grading System. In: 2018 5th IEEE International Conference on Cloud Computing and Intelligence Systems (CCIS) p 430–435. IEEE.
- Wang Y, et al. (2018b) “Automatic Essay Scoring Incorporating Rating Schema via Reinforcement Learning.” EMNLP
- Zhu W, Sun Y (2020) Automated essay scoring system using multi-model Machine Learning, david c. wyld et al. (eds): mlnlp, bdiot, itccma, csity, dtmn, aifz, sigpro
- Wresch W. The Imminence of Grading Essays by Computer-25 Years Later. Comput Compos. 1993; 10 :45–58. doi: 10.1016/S8755-4615(05)80058-1. [ CrossRef ] [ Google Scholar ]
- Wu, X., Knill, K., Gales, M., & Malinin, A. (2020). Ensemble approaches for uncertainty in spoken language assessment.
- Xia L, Liu J, Zhang Z (2019) Automatic Essay Scoring Model Based on Two-Layer Bi-directional Long-Short Term Memory Network. In: Proceedings of the 2019 3rd International Conference on Computer Science and Artificial Intelligence p 133–137
- Yannakoudakis H, Briscoe T, Medlock B (2011) A new dataset and method for automatically grading ESOL texts. In: Proceedings of the 49th annual meeting of the association for computational linguistics: human language technologies p 180–189
- Zhao S, Zhang Y, Xiong X, Botelho A, Heffernan N (2017) A memory-augmented neural model for automated grading. In: Proceedings of the Fourth (2017) ACM Conference on Learning@ Scale p 189–192
- Zupanc K, Bosnic Z (2014) Automated essay evaluation augmented with semantic coherence measures. In: 2014 IEEE International Conference on Data Mining p 1133–1138. IEEE.
- Zupanc K, Savić M, Bosnić Z, Ivanović M (2017) Evaluating coherence of essays using sentence-similarity networks. In: Proceedings of the 18th International Conference on Computer Systems and Technologies p 65–72
- Dzikovska, M. O., Nielsen, R., & Brew, C. (2012, June). Towards effective tutorial feedback for explanation questions: A dataset and baselines. In Proceedings of the 2012 Conference of the North American Chapter of the Association for Computational Linguistics: Human Language Technologies (pp. 200-210).
- Kumar, N., & Dey, L. (2013, November). Automatic Quality Assessment of documents with application to essay grading. In 2013 12th Mexican International Conference on Artificial Intelligence (pp. 216–222). IEEE.
- Wu, S. H., & Shih, W. F. (2018, July). A short answer grading system in chinese by support vector approach. In Proceedings of the 5th Workshop on Natural Language Processing Techniques for Educational Applications (pp. 125-129).
- Agung Putri Ratna, A., Lalita Luhurkinanti, D., Ibrahim I., Husna D., Dewi Purnamasari P. (2018). Automatic Essay Grading System for Japanese Language Examination Using Winnowing Algorithm, 2018 International Seminar on Application for Technology of Information and Communication, 2018, pp. 565–569. 10.1109/ISEMANTIC.2018.8549789.
- Sharma A., & Jayagopi D. B. (2018). Automated Grading of Handwritten Essays 2018 16th International Conference on Frontiers in Handwriting Recognition (ICFHR), 2018, pp 279–284. 10.1109/ICFHR-2018.2018.00056

Top 25 AI-Powered Online Grading System Options for Faster Feedback
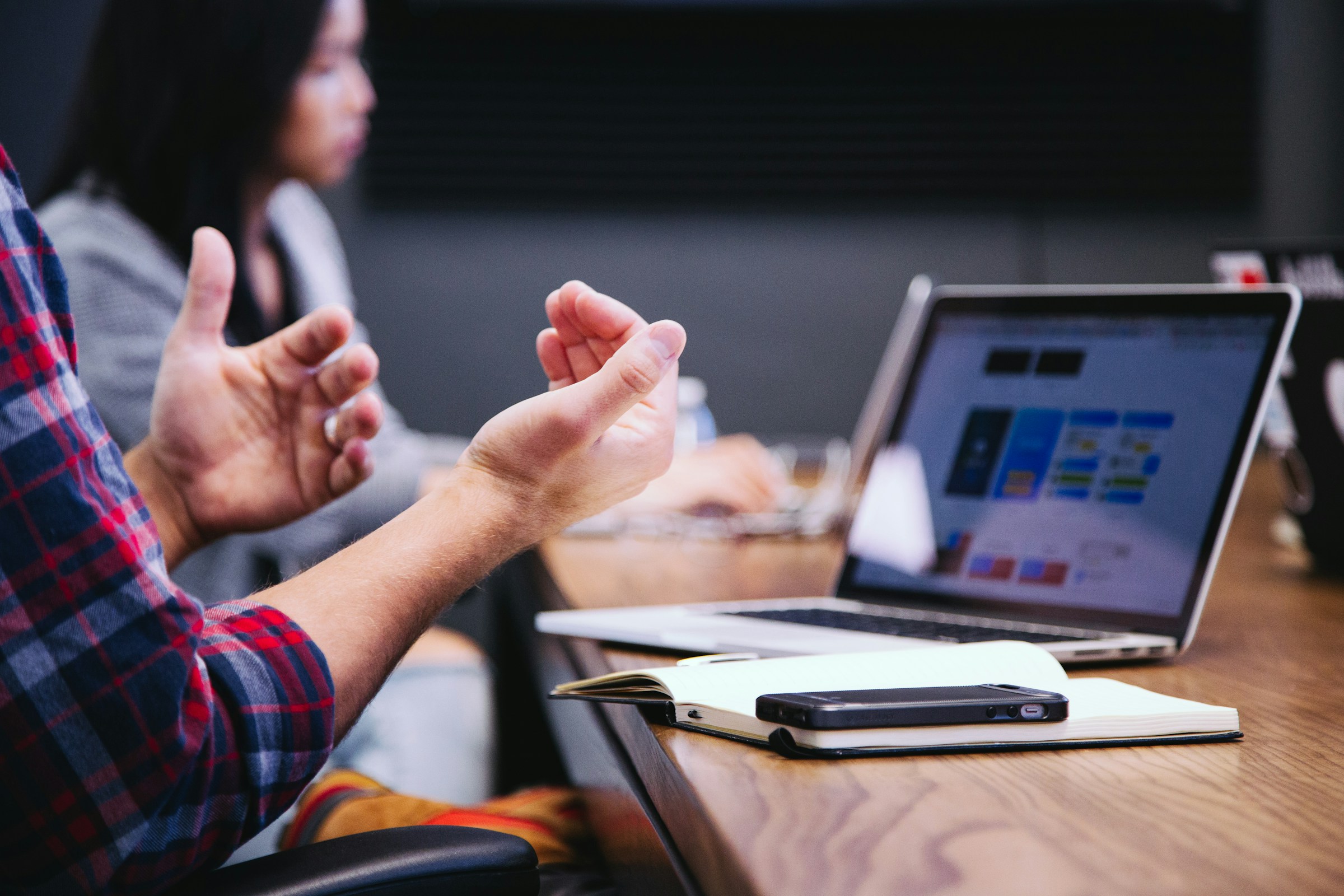
Imagine you're a teacher, overwhelmed by a pile of essays and assignments with overdue feedback. Sound familiar? Online grading systems can ease your workload and boost efficiency. With the integration of AI in the classroom , tasks like grading and personalized feedback become even more streamlined, allowing educators to focus on instruction rather than administrative duties. This article will guide you to an AI-powered grading system that speeds up feedback, saves time, and simplifies student performance evaluations. EssayGrader.ai is a powerful grading software for teachers designed to streamline assessments so you can focus on what truly matters: teaching and guiding your students.
What Is an Online Grading System?
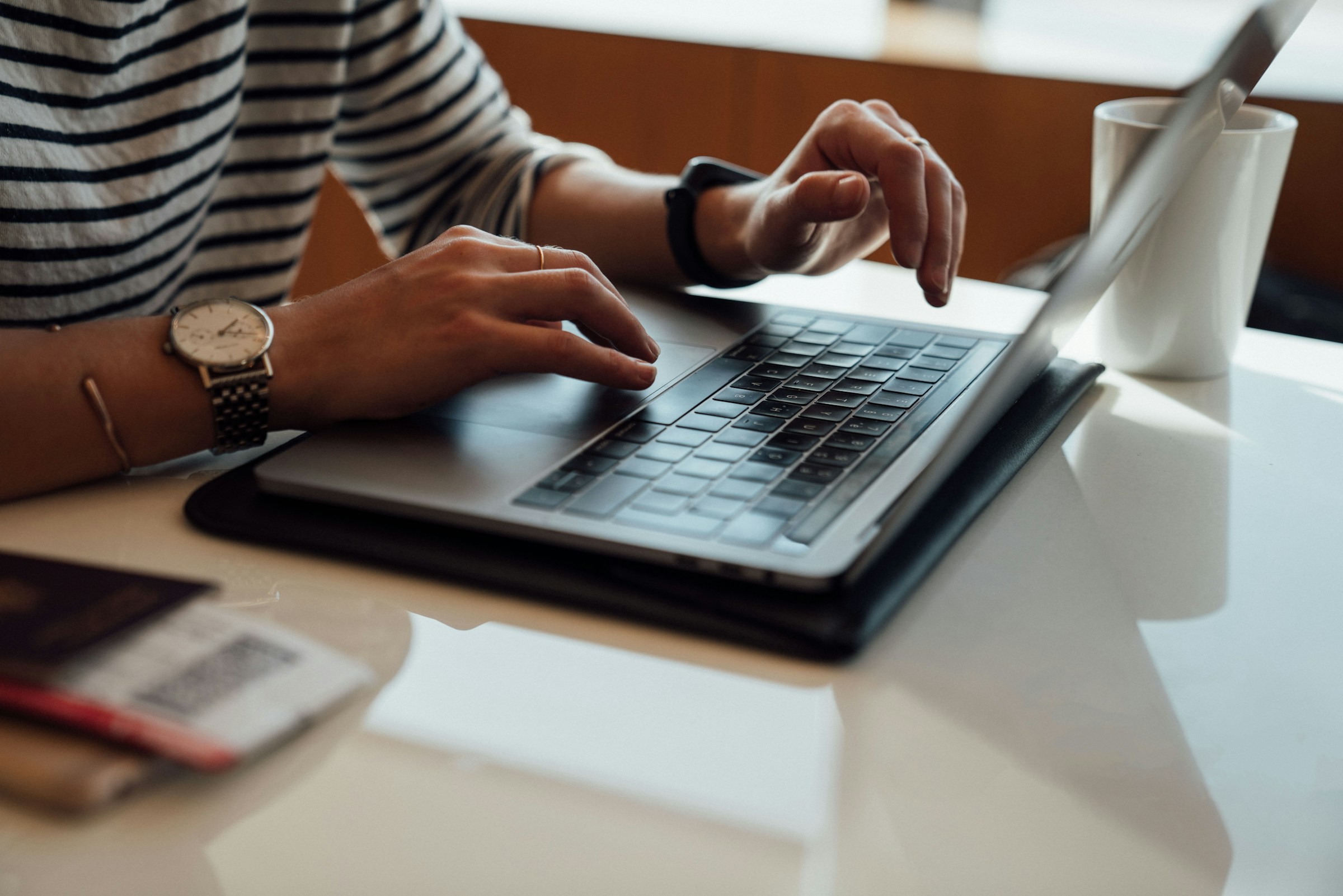
Online grading systems are transforming how teachers manage academic performance. These digital platforms streamline the grading process, allowing educators to enter, track quickly, and report grades. They go beyond numbers, helping teachers manage:
- Assignments
- Communication with students and parents
With everything in one place, teachers can focus on teaching which matters most.
Real-Time Feedback: Keeping Everyone in the Loop
One of the standout features of online gradebooks is the ability to update grades instantly . Teachers can record and share their scores as soon as students complete assignments. This real-time feedback keeps students and parents informed. Students can see where they excel and where they need to improve, giving them time to make changes before the next big test. Parents can stay connected to their child's progress, offering support when needed.
Accessibility and Organization: A Teacher's Dream
Online gradebooks offer easy accessibility and organization. Teachers can access grades from anywhere, whether at school, home, or on the go. This flexibility makes it easy to keep everything organized and up to date. With a few clicks, teachers can sort and filter grades, making it simple to see how students are doing in specific areas. This level of organization helps teachers make informed decisions about instruction and support.
Promoting Student Empowerment and Parental Involvement
Online gradebooks empower students to take charge of their learning by providing real-time updates and easy access to grades. When students know where they stand, they can set goals and work towards achieving them. Parents can also play a more active role in their child's education, offering encouragement and guidance as needed. This level of involvement can make a big difference in a student's success.
Streamlining Communication With Parents and Students
Online gradebooks make communication between teachers, students, and parents a breeze. With a centralized platform , teachers can:
- Send messages
- Share feedback
- Provide updates with ease
This level of communication helps build solid relationships and ensures everyone is on the same page. When teachers, students, and parents work together, students can succeed better.
Related Reading
- Different Grading Systems
- How Do Teachers Grade
- LMS Gradebook
- Evidence Based Grading
- Technology In The Classroom
- Constructive Criticism Examples For Students
- Digital Gradebook
- Education Workflow Automation
- Grading Software For Teachers
AI's Role in Online Grading Systems

When grading is manual, a teacher’s mood, personal preferences, or unconscious biases can seep in. Such subjectivity risks unfair evaluations and inconsistent results. Plus, traditional grading is a time drain. Teachers slog through tests and assignments when they could be crafting lessons or guiding struggling students. And feedback? Limited. Students often get vague notes like “ Good job ” or “ Needs improvement ,” which leaves them in the dark about their strengths and shortcomings.
AI as a Grading Game-Changer
AI is the superhero that saves the day for educators. Its automated grading systems can handle multiple-choice questions and other tasks in the blink of an eye. That means students get feedback faster , grasping what they need to improve.
AI’s objectivity is also a boon. It removes the risk of human biases tainting the results, ensuring a fair evaluation. And its scalability is a godsend. AI can handle thousands of exams with consistent assessments, eliminating the inconsistency of having multiple human graders.
Personalized Feedback Revolution
The magic of AI grading lies in its ability to tailor feedback to each student. Analyzing student responses can identify strengths and weaknesses and provide specific guidance to help them improve.
This personalized feedback empowers students by showing them where to focus their efforts and giving them a roadmap for improvement. It also frees teachers from the burden of creating individualized guidance for every student, allowing them to focus on more meaningful interactions.
AI's Role in Making It Happen
AI powers automated grading systems with natural language processing algorithms that can decipher written responses. It uses pattern recognition to identify common mistakes or misconceptions, providing insights into where students need help.
Its adaptive learning capabilities allow it to evolve and improve, generating more accurate and helpful feedback. The result is a grading process that’s fast, fair, and effective, allowing teachers to focus on the human aspect of education.
How Does an AI-Powered Online Grading System Work?
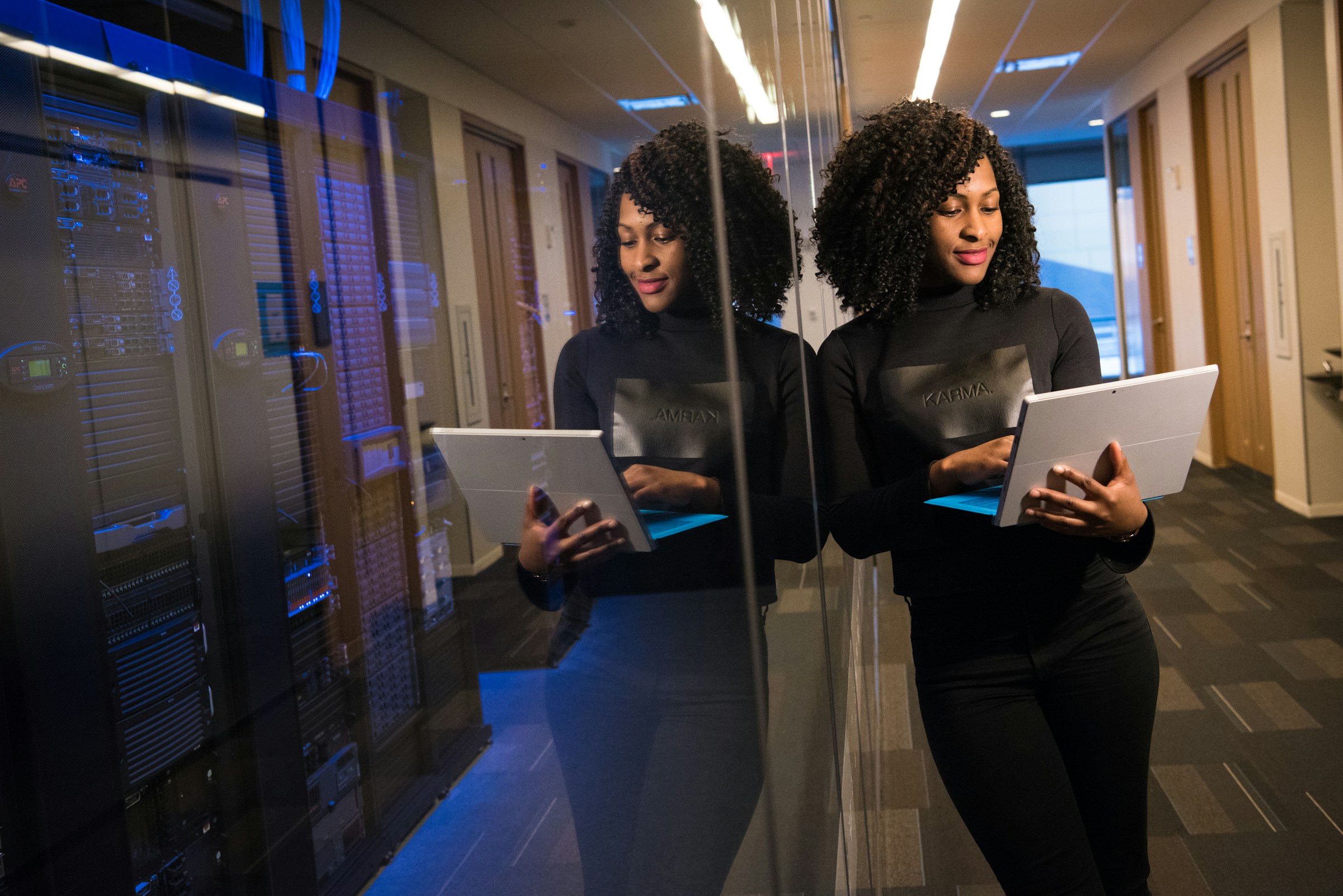
NLP: The Secret of AI Grading
AI-powered grading systems lean heavily on Natural Language Processing (NLP) to understand student responses. NLP algorithms break down text to assess its:
These systems use machine learning techniques to measure a student’s grasp of the subject matter. This analysis gives educators insights and helps students know where they stand. It’s like having an assistant who reads and understands text with the finesse of a human but at warp speed.
Pattern Recognition: Spotting Issues Before They Snowball
AI doesn’t just read; it recognizes patterns in student responses . Identifying common errors or misconceptions highlights areas where students struggle. This allows educators to adjust their teaching strategies, focusing on what matters. It’s like having a radar that picks up on trouble spots before they become real problems.
Adaptive Learning: Tailored Education for Every Student
As AI-powered grading systems process student data, they adapt and improve. They tailor feedback and recommendations, giving each learner a unique experience. This personalized approach helps students progress at their own pace. It’s like having a personal tutor that grows smarter with every interaction.
Feedback Generation: Detailed Insights Without the Hassle
AI algorithms can generate detailed feedback automatically. They highlight strengths and areas for improvement, providing guidance that’s as specific and actionable as what a teacher might give. This saves educators time, allowing them to focus on teaching rather than grading. It’s like having a time-saving assistant who ensures every student gets their needed help.
Revolutionize Your Grading With EssayGrader
Save 95% of your time grading school work with our tool to get high-quality, specific, and accurate writing feedback for essays in seconds with EssayGrader's grading software for teachers . Get started for free today!
8 Benefits of Online Gradebooks
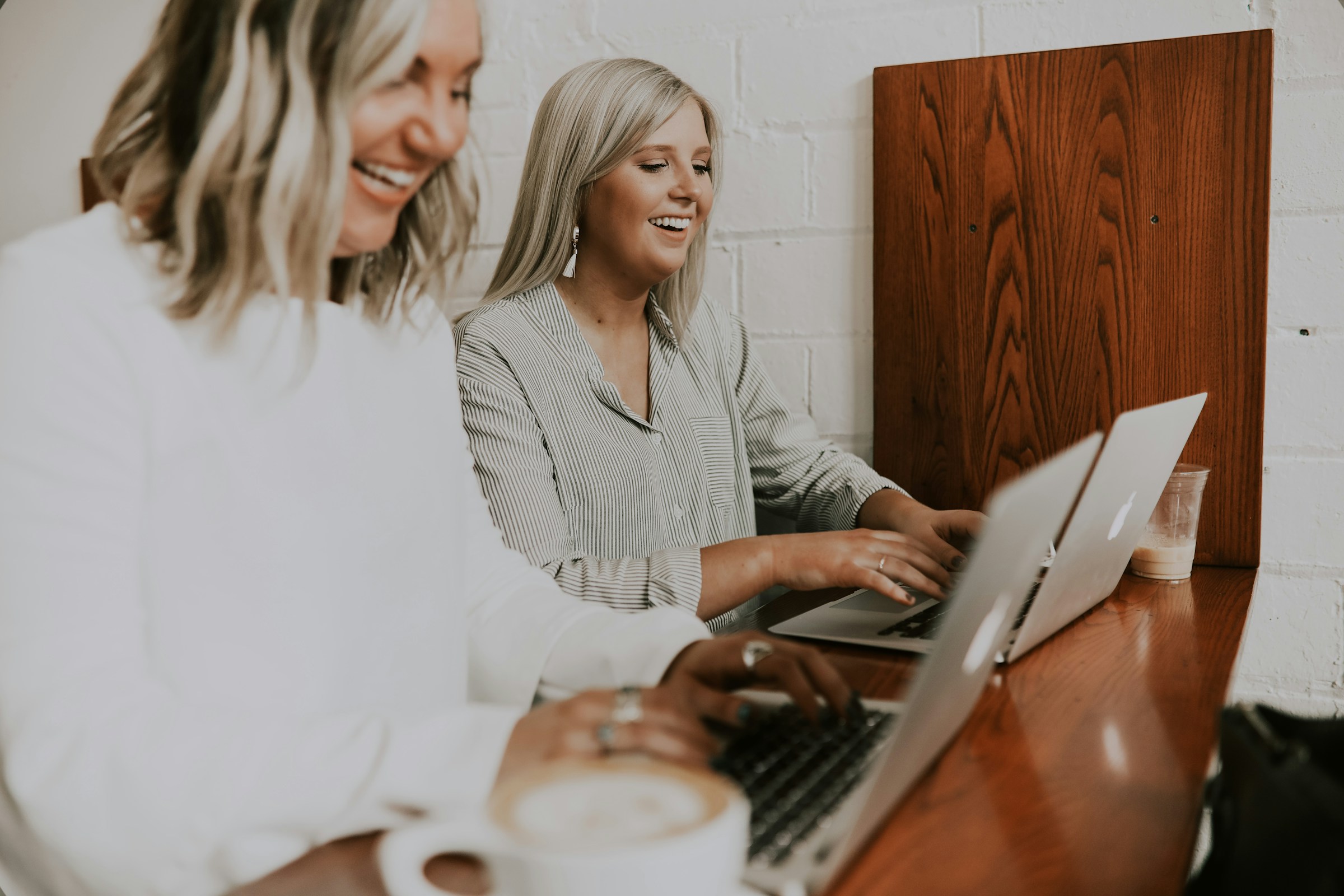
1. Enhanced Accessibility and Convenience
Online grading systems revolutionize how educators, students, and parents interact with academic information. With just a few clicks, anyone can access real-time grades and feedback from anywhere with an internet connection. This accessibility breaks down barriers and fosters open communication between teachers and parents. No more waiting for report cards to know how a student is doing; everyone can stay informed and engaged.
2. Customization and Flexibility
Traditional grading systems often force schools into rigid 10-point scales. Online gradebooks break free from this mold, offering the flexibility to customize grading criteria and assessments. This innovation is especially beneficial for charter schools, which may have unique academic standards. Schools can create grading scales to reflect their assessment systems accurately, leading to a more personalized and fair educational experience.
3. Real-time Updates
Gone are the days of waiting for progress reports. Online gradebooks provide real-time access and updates for parents and students. With traditional paper systems, everyone had to wait for the following report to see grades and feedback. Now, online gradebooks allow parents and students to log in anytime to view:
- Teacher feedback
This transparency leads to better communication and keeps everyone on the same page regarding academic progress.
4. Data-driven Decision Making
Online grading systems offer a wealth of data and analytics for educators to uncover:
- Areas for improvement
By analyzing this data, schools can make informed decisions about curriculum adjustments, teaching methods, and student support initiatives. These data-driven decisions lead to a more effective and personalized student educational experience.
5. Streamlined Communications
Online gradebooks streamline communication between educators, students, and parents. Educators ensure that everyone stays informed and engaged by effortlessly sharing:
- Assignment specifics
- Grading criteria
- Constructive feedback
This transparent communication fosters a supportive learning environment and strengthens the bond between schools and families.
6. Integration With School Management Systems
Online gradebook platforms often seamlessly integrate with existing Learning Management Systems (LMS) or School Management Systems (SMS). This integration creates a cohesive educational ecosystem, allowing educators to align the following with the LMS:
- Assessments
This centralizes all academic activities and streamlines processes for everyone involved.
7. Promoting Accountability and Responsibility
Online grading systems empower students to take responsibility for their learning journey. With the ability to review their grades and assignments, students are motivated to:
- Establish objectives
- Monitor their advancement
- Reach out for extra assistance when necessary
This cultivation of responsibility nurtures a proactive mindset towards learning.
8. Efficiency and Cost Savings
One of the benefits of online gradebooks is that they have the potential to enhance efficiency and save money for schools. By simplifying the grading process and offering immediate access for parents and students, teachers can allocate more time to teaching and less to administrative duties. This can also lead to cost savings for schools, as they may need fewer staff members to manage grading and reporting.
25 Best AI-Powered Online Grading System Options
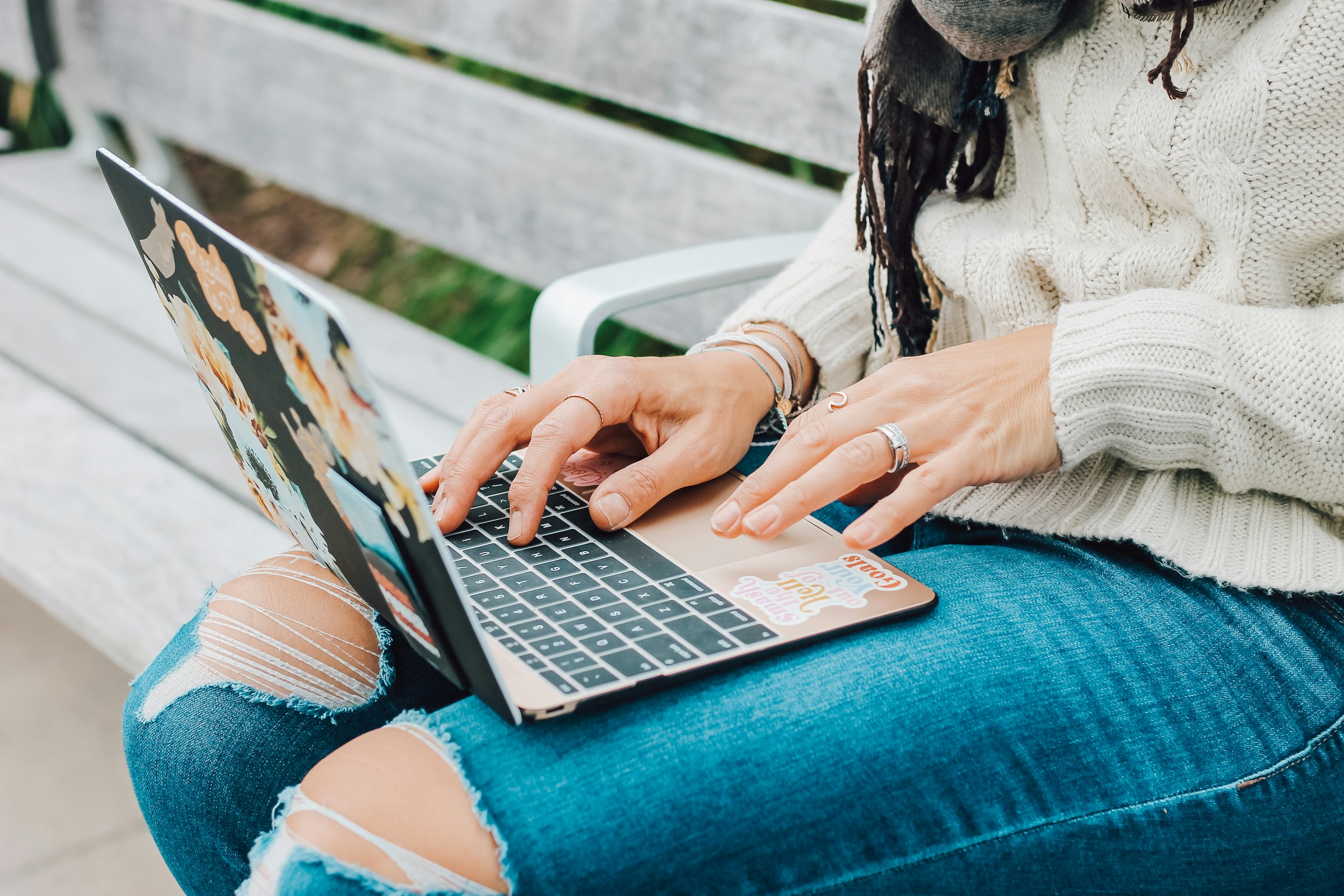
1. EssayGrader
Essaygrader is the most accurate AI grading platform, trusted by over 60,000 educators. It cuts grading time from 10 minutes to 30 seconds. Features include:
- Custom rubrics
- Bulk uploads
- AI detectors
- Used at all educational levels
2. Gradescope
A versatile application by Turnitin for grading and analyzing assessments. It supports the following:
- Online assignments
- Programming
- Bubble sheets
Students can upload work for evaluation.
3. Zipgrade
A mobile app for scanning and grading multiple-choice tests. Teachers can:
- Print answer sheets
- Create custom keys
- Share reports with students and parents
4. Co-Grader
AI-guided grading system for work imported from Google Classroom. It supports rubric-based grading and allows teachers to define criteria using templates.
Popular LMS with real-time assessment features. Automatically grades student assessments and provides detailed reports and analytics dashboards.
AI Grader evaluates essays based on the following:
- Plagiarism detection
- Grammar checking
- Readability
Offers feedback and suggestions, highlighting errors and improvements.
7. MagicSchool
Offers over 60 tools for educators , including a Rubric Generator and Diagnostic Assessment Generator for multiple-choice assessments.
Provides over 100 educational resources, including assessment tools. Offers “lesson seeds” with resources to enhance lesson plans, including:
9. ClassCompanion
AI tool that assesses student writing and provides real-time feedback. It helps teachers identify areas for improvement and track progress.
10. Feedback Studio
By Turnitin, offers a range of feedback tools, including:
- Drag-and-drop “QuickMarks”
- Voice comments
- Automatic grammar checking
Annotations and rubrics help focus feedback.
11. EnglightenAI
It syncs with Google Classroom to provide quick feedback. This allows teachers to train the AI to understand their grading scale and pedagogical focus.
12. Graded Pro
Fully integrated with Google Classroom, it automates the retrieval of student submissions and offers AI-assisted grading for various subjects.
13. Happy Grader
Created by a veteran teacher, it uses pattern recognition for grading exams and provides predictive scoring and feedback for paragraph responses.
14. Timely Grader
It streamlines grading from rubric creation to grade pass-back. It offers explanations for AI grading suggestions and provides personalized feedback for students.
15. Kangaroo AI Essay Grader
In beta mode, it offers instant grading with customizable rubrics. It provides 24/7 support through an AI assistant and ensures data safety.
An advanced system that gives detailed grading evaluations and personalized feedback. It offers comprehensive reports on student performance.
17. Coursebox
All-in-one platform with AI capabilities for creating and grading courses. Suitable for students of all levels.
AI-assisted tool for checking open-ended questions and providing detailed feedback.
19. ChatGPT
It can be used for grading with customized models for exams and essays. It offers detailed feedback and tips for improvement.
Provides immediate feedback and suggestions for improvement. It is suitable for essays, short texts, and math exams.
21. Progressay
Ideal for a hands-off approach. It grades assignments and highlights errors in the following:
- Sentence structure
22. Marking.ai
A comprehensive tool for creating and grading assignments. Promises to save significant time each week.
23. Crowdmark
It allows students to scan assignments for online grading. It offers tools like annotations and comment libraries for efficient grading.
Gradebook collects grades from all course activities. Provides automatic grading for quizzes and customizable scales for manual grading.
25. Edmentum
Grading service with qualified assistants to grade and provide detailed feedback. Frees up teachers’ time for teaching.
What Is the Role of Teachers in an AI-Driven Grading Environment
.jpeg)
Co-Designing Learning Experiences
In the AI age, teachers aren’t just dispensers of knowledge. They’re co-designers of learning experiences . AI tools can help students explore their passions while nurturing problem-solving and critical thinking. By integrating AI into lesson plans, educators empower students to connect with real-world scenarios and build the skills they need for the future.
Curating AI-Driven Content: The New Role of Teachers
AI is everywhere, offering an overwhelming amount of educational content. Teachers become curators, selecting and adapting AI-generated materials to suit their students' needs. They ensure content aligns with academic goals and encourages deep understanding. By sifting through AI resources, educators can tailor instruction to learners' strengths and interests.
Embracing Collaboration: Teachers as Partners in Learning
AI brings new chances for teamwork and collaboration. Educators now act as facilitators, connecting students with external organizations and experts. These collaborative experiences teach students valuable skills like teamwork and adaptability. At the same time, teachers must be learners, continuously growing alongside their students and modeling the importance of curiosity.
Building Learning Communities: The Human Touch in the Digital Age
Human connection is more crucial than ever in a world dominated by technology. Teachers create supportive environments by fostering relationships with students and nurturing their emotional well-being. AI can provide personalized feedback , but it’s the face-to-face interactions that genuinely make a difference. Educators build communities where students feel valued and motivated to learn.
Preparing Students for AI-Driven Careers: A New Educational Focus
As AI transforms industries, students need new skills to succeed. Teachers help them develop:
- Critical thinking
- Ethical decision-making
Educators incorporate AI into the curriculum to help students understand its societal impacts and become responsible users and creators.
- How To Grade In Google Classroom
- Automation In Education
- Magic School AI
- Grading Writing
- Teacher Apps
- Apps For Teachers
- AI Tools For Education
- Technology Tools For The Classroom
- Google Classroom Alternatives
- Standards Based Grading Systems
Is AI-Assisted Grading a Magic Wand or a Pandora’s Box?
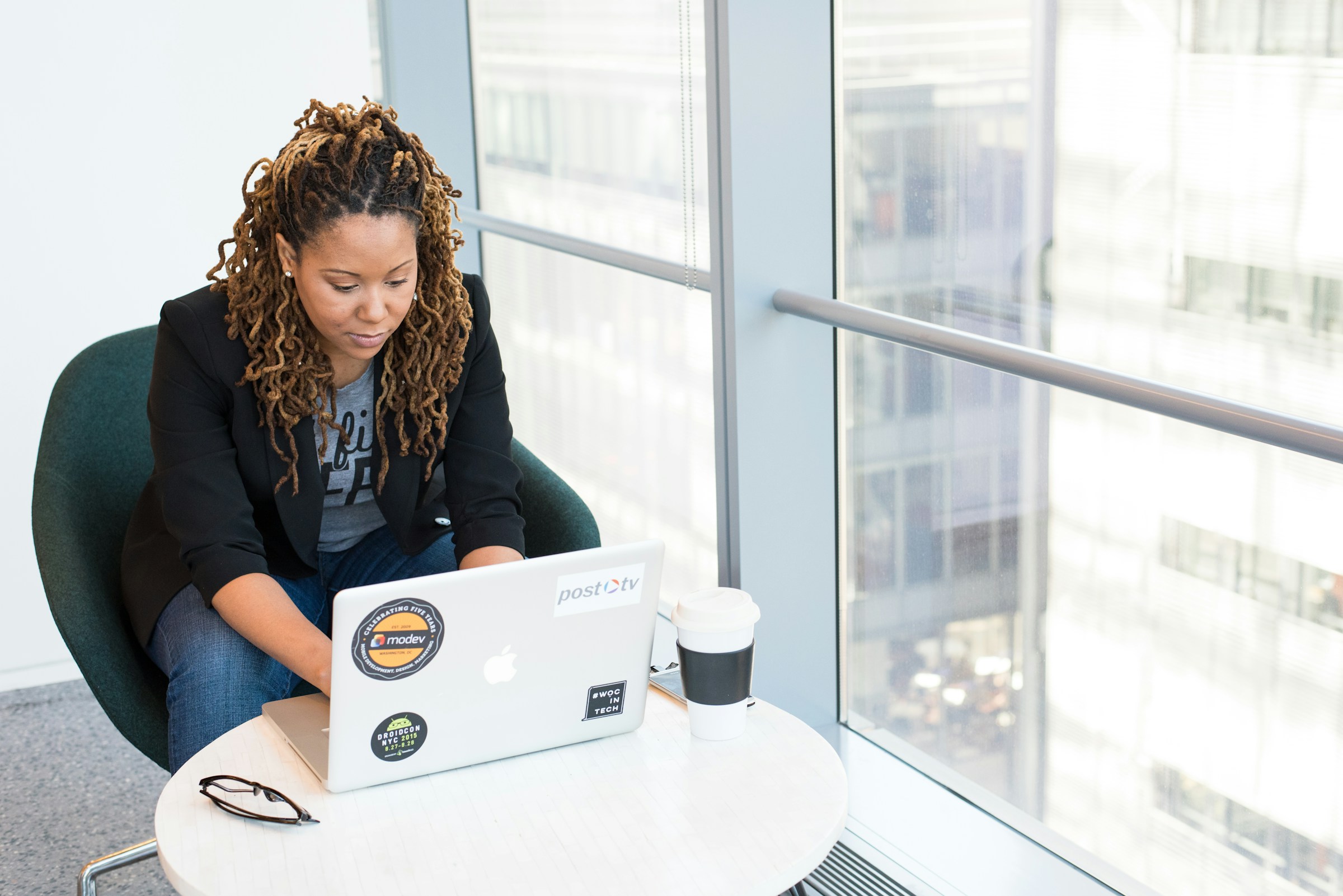
Imagine giving your students a finance problem set and having an AI grading system quickly assess their numerical responses. The AI would deliver immediate feedback, letting students correct mistakes and learn in real-time.
This process frees you to focus on complex and subjective tasks, like guiding struggling students or designing engaging activities. AI can assess grammar and coherence in writing courses, enabling you to concentrate on more advanced aspects like argumentation.
The Risks of Overreliance on AI Grading
While AI can handle structured tasks, it struggles with nuanced assignments. Take a strategic management course. When students create a business strategy , they apply:
- Contextual understanding
These elements are often beyond the reach of AI, which might offer superficial or formulaic feedback. Relying too much on AI could undermine learning and erode trust between students and teachers.
Bias and Fairness Concerns
AI grading systems are only as good as the data they're trained on. If a system learns from a biased sample, it can perpetuate or amplify existing inequities. For instance, an AI trained mainly on business plans from male-led startups might unfairly penalize projects addressing women’s challenges. This bias can hinder the development of strategic thinking skills needed for leadership.

Responsible Use of AI in Education
To harness AI’s power responsibly:
- Use it to complement human judgment , not replace it.
- Protect student privacy by anonymizing data and using secure tools.
- Be transparent with students about AI’s role in grading.
- Regularly audit the AI for accuracy and fairness.
- Adjust practices based on student outcomes and feedback.
These principles help you leverage AI’s benefits while mitigating its risks.
5 Tips for Grading Ethically With AI
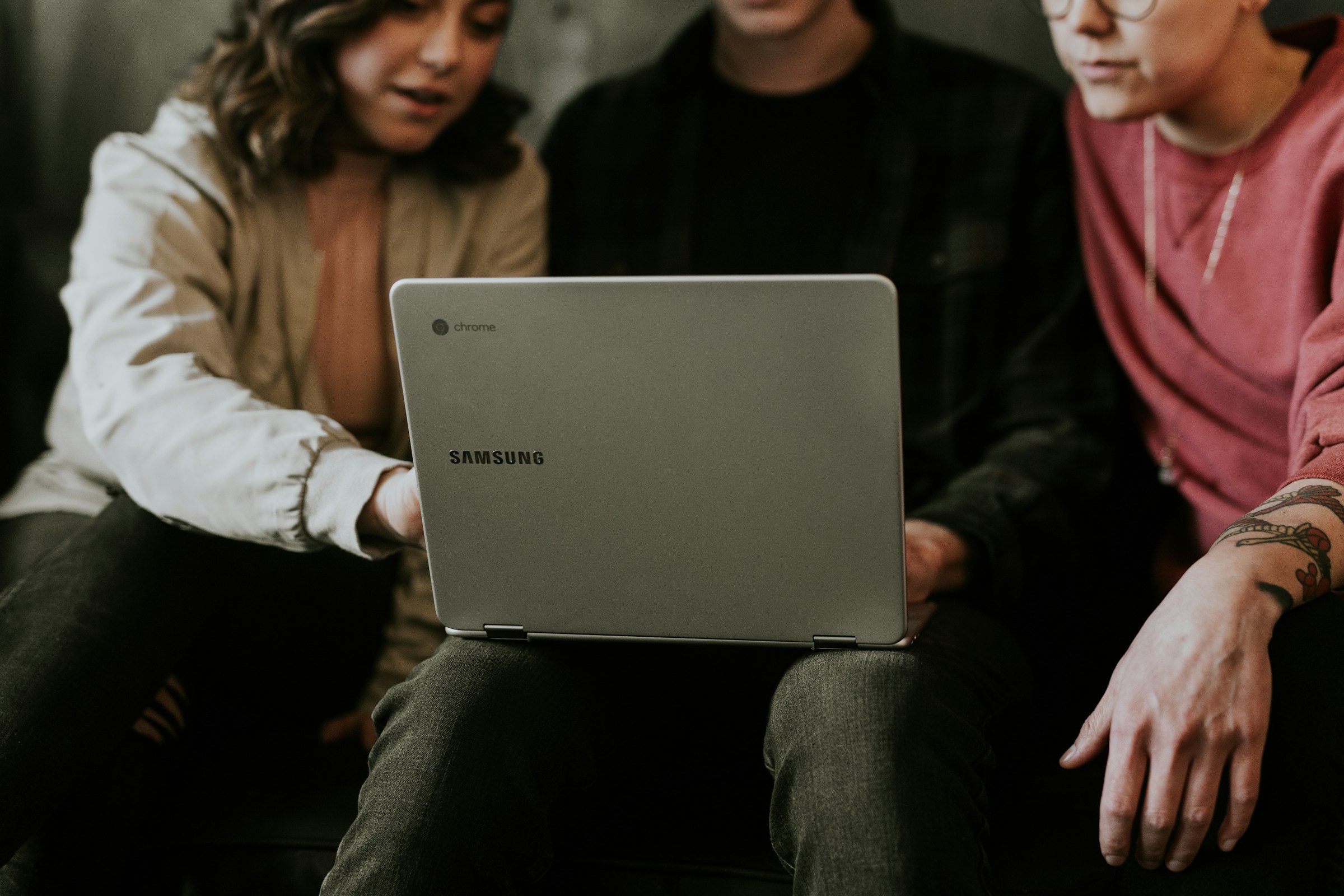
1. Clarity and Openness: Be Transparent with Students
Transparency is essential when integrating AI into grading. Start by adding a detailed AI statement to your syllabus. Explain to students that AI assists in grading but doesn't replace your evaluation. Make it clear that AI offers more comprehensive and timely feedback, enhancing the learning experience. Encourage students to provide feedback on this grading method and assure them of a safe space to ask questions or voice concerns.
Here's a sample statement for your syllabus:
" In this course, AI is encouraged for certain tasks with attribution: You can use AI tools to brainstorm assignments or revise work. I will use AI to assist in evaluating work against rubrics, identifying strengths or areas needing growth, and providing feedback. Rest assured, I review all work and feedback personally. If you have questions about AI's role in grading or need a second evaluation, feel free to reach out. "
2. Consistency is Key: Grade Against a Rubric
A rubric brings consistency to grading and sets clear expectations. When using AI for grading, input the rubric first, asking AI to measure success against these criteria. Teach students to self-assess their work using AI, empowering them to take charge of their learning. Always allow students to request a reevaluation of their work. This practice shows you care and are willing to address any concerns. Invite students to request a manual reevaluation or additional help if needed.
3. Quality Control: Ensure Quality of Feedback—Every Time
AI can be a fantastic tool, but it's not infallible. Review and edit AI-generated feedback to ensure it is accurate and relevant, and be cautious of potential biases within AI systems. To mitigate bias, test specific prompts beforehand and input your evaluative measures. For example, instead of asking the AI to "Determine whether this essay is well written," input your rubric and say: "Evaluate this essay using the rubric provided. Determine strengths and areas for improvement based on the rubric points."
4. Prioritize Learning: Focus on Feedback Over Letter Grades
AI tools can help analyze patterns in student work, but assigning letter grades isn't recommended. Traditional grading is subjective, and AI cannot assess skill mastery. Instead, use AI to produce qualitative feedback.
Alternative grading methods can prioritize feedback over letter grades. When adopting inclusive grading approaches, students can help create rubrics or assignments, fostering a more equitable learning environment.
5. Seek Input: Run It by Your Colleagues
If you need clarification on whether AI-assisted grading is ethical , open a dialogue with colleagues. Bring it up in meetings or ask your department head for their thoughts. Consider questions like:
- Does AI-assisted grading align with our institution's policies?
- Is grading with AI equitable?
- Do its benefits outweigh ethical concerns?
Your colleagues' insights can help gauge whether AI is appropriate in your setting. Ask yourself: Will AI help me serve my students better? Will it enhance or diminish learning?
- Gradebook Software
- Best Online Gradebook
- Free Gradebooks For Teachers
- Grading Software For Schools
- Automatic Grading
- Grading Management Software
- How To Grade Student
Save Time While Grading Schoolwork with EssayGrader's Grading Software for Teachers
Imagine cutting down essay grading time from 10 minutes to just 30 seconds. EssayGrader delivers this efficiency boost while maintaining accuracy. Trusted by 60,000 educators globally, it saves teachers countless hours. This AI tool lets teachers:
- Replicate their grading rubrics
- Set up custom criteria and more
The result is high-quality, specific, and accurate feedback delivered in seconds. What would you do with all that extra time?
Replicate and Customize Your Grading Rubrics
Why start from scratch when you can use what you already have? EssayGrader allows teachers to replicate their existing grading rubrics, so the AI doesn’t have to guess the criteria. You can also set up custom rubrics, ensuring grades align perfectly with your expectations. This flexibility means you can tailor the grading experience to fit any assignment.
Grade Essays by Class and Bulk Upload
Handling essays for an entire class can be overwhelming, but EssayGrader streamlines the process. You can grade essays by class, making organizing and managing your workload easy. The bulk upload feature lets you add multiple essays simultaneously, saving you even more time. Spend less time organizing and more time teaching.
Catch Essays Written by AI With Our Detector
In an age where AI can write essays, ensuring students submit their work is essential. EssayGrader includes an AI detector to catch essays written by machines. This feature gives you peace of mind, knowing that the job you’re grading is original. Protect academic integrity without adding to your workload.
Summarize Essays Quickly and Easily
Need a quick overview without reading an entire essay? The EssayGrader summarizer provides concise summaries, giving you the gist without the fluff. This feature is perfect for understanding a student's work without spending much time. Stay informed and efficient with this handy tool.
Try EssayGrader for Free Today
Want to see how much time you can save with grading software for teachers ? Get started with EssayGrader for free. Discover how this AI tool can transform your grading process and give you more time to focus on teaching. Join the 60,000 educators who already trust EssayGrader and experience the benefits for yourself.
Start grading today
Save hours by grading essays in 30 seconds or less.
Related blogs
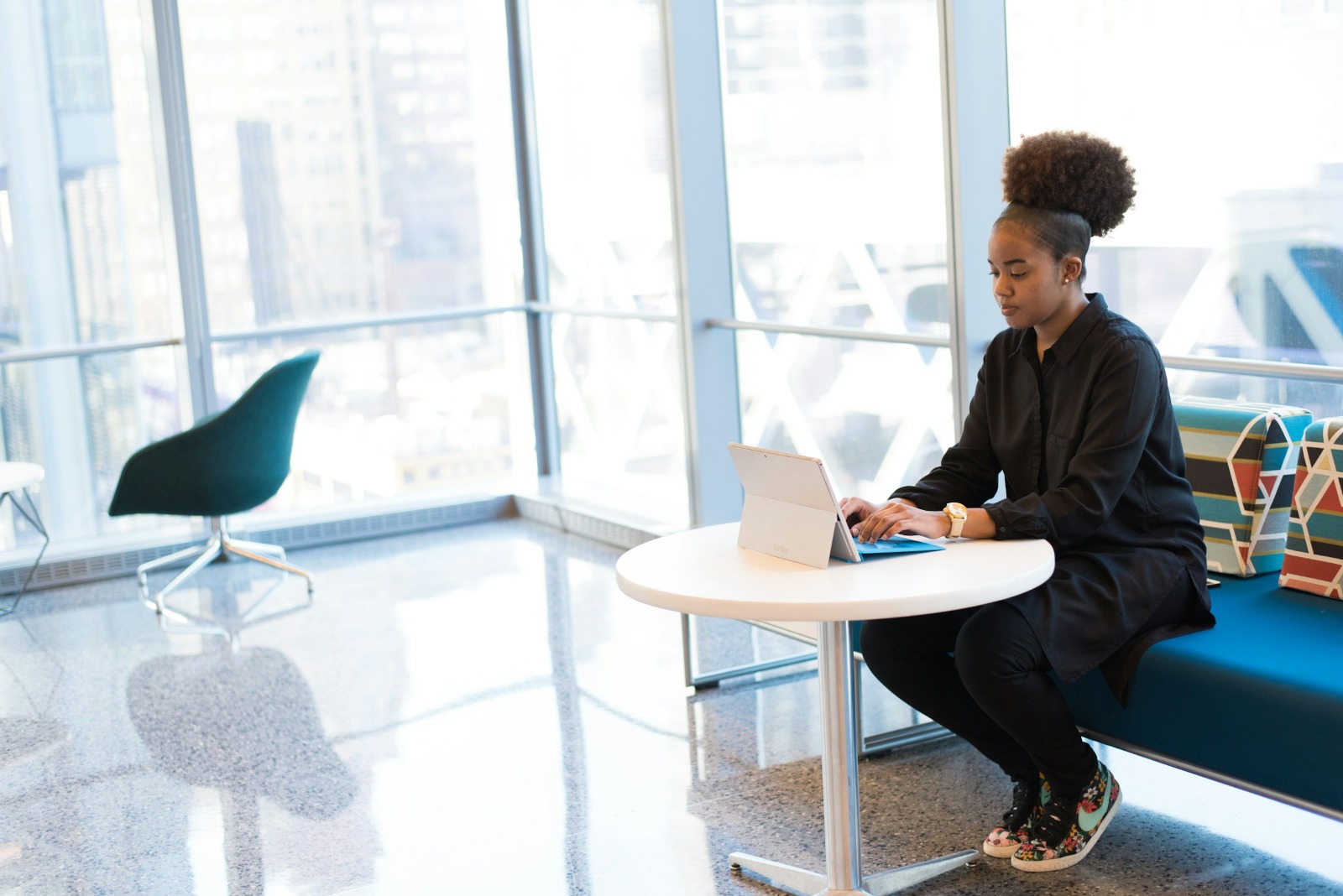
How to Grade Students Efficiently & Get off the Grading Hamster Wheel
Get off the grading hamster wheel! This guide reveals how to grade students efficiently, allowing you to focus on teaching rather than paperwork.

Top 27 Grading Management Software Tools for Smarter Assessments
Upgrade your grading process with these top 27 tools designed to simplify assessments and boost classroom efficiency.
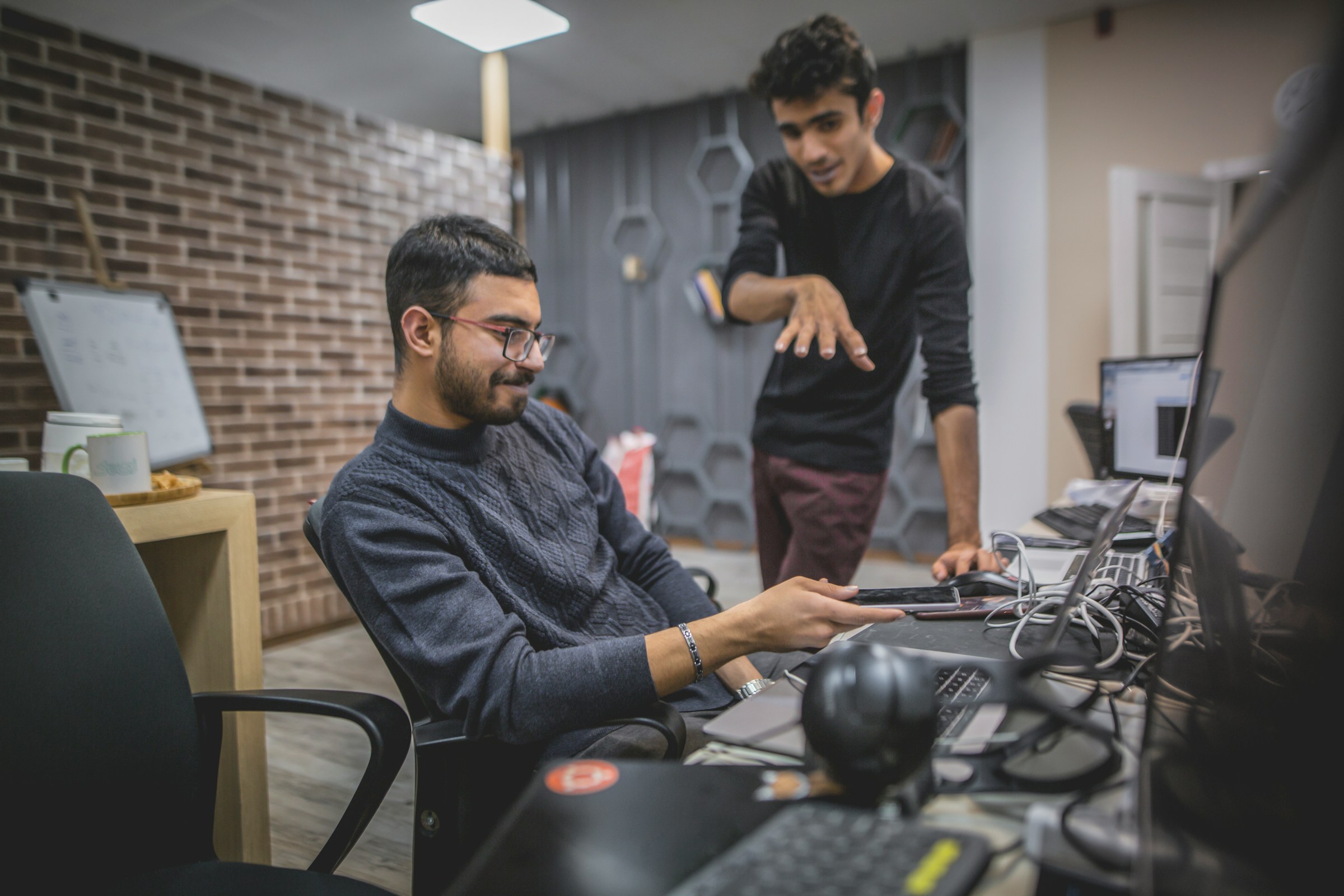
31 Best Automatic Grading Tools to Enhance Classroom Efficiency
These 31 automatic grading tools enhance classroom efficiency, helping teachers save time while delivering accurate student assessments.
Automated Essay Scoring Systems
- Reference work entry
- Open Access
- First Online: 01 January 2023
- pp 1057–1071
- Cite this reference work entry
You have full access to this open access reference work entry
- Dirk Ifenthaler 3 , 4
22k Accesses
2 Citations
Essays are scholarly compositions with a specific focus on a phenomenon in question. They provide learners the opportunity to demonstrate in-depth understanding of a subject matter; however, evaluating, grading, and providing feedback on written essays are time consuming and labor intensive. Advances in automated assessment systems may facilitate the feasibility, objectivity, reliability, and validity of the evaluation of written prose as well as providing instant feedback during learning processes. Measurements of written text include observable components such as content, style, organization, and mechanics. As a result, automated essay scoring systems generate a single score or detailed evaluation of predefined assessment features. This chapter describes the evolution and features of automated scoring systems, discusses their limitations, and concludes with future directions for research and practice.
You have full access to this open access chapter, Download reference work entry PDF
Similar content being viewed by others
An automated essay scoring systems: a systematic literature review
Automated Essay Feedback Generation in the Learning of Writing: A Review of the Field
- Automated essay scoring
- Essay grading system
- Writing assessment
- Natural language processing
- Educational measurement
- Technology-enhanced assessment
- Automated writing evaluation
Introduction
Educational assessment is a systematic method of gathering information or artifacts about a learner and learning processes to draw inferences of the persons’ dispositions (E. Baker, Chung, & Cai, 2016 ). Various forms of assessments exist, including single- and multiple-choice, selection/association, hot spot, knowledge mapping, or visual identification. However, using natural language (e.g., written prose or essays) is regarded as the most useful and valid technique for assessing higher-order learning processes and learning outcomes (Flower & Hayes, 1981 ). Essays are scholarly analytical or interpretative compositions with a specific focus on a phenomenon in question. Valenti, Neri, and Cucchiarelli ( 2003 ) as well as Zupanc and Bosnic ( 2015 ) note that written essays provide learners the opportunity to demonstrate higher order thinking skills and in-depth understanding of a subject matter. However, evaluating, grading, and providing feedback on written essays are time consuming, labor intensive, and possibly biased by an unfair human rater.
For more than 50 years, the concept of developing and implementing computer-based systems, which may support automated assessment and feedback of written prose, has been discussed (Page, 1966 ). Technology-enhanced assessment systems enriched standard or paper-based assessment approaches, some of which hold much promise for supporting learning processes and learning outcomes (Webb, Gibson, & Forkosh-Baruch, 2013 ; Webb & Ifenthaler, 2018 ). While much effort in institutional and national systems is focused on harnessing the power of technology-enhanced assessment approaches in order to reduce costs and increase efficiency (Bennett, 2015 ), a range of different technology-enhanced assessment scenarios have been the focus of educational research and development, however, often at small scale (Stödberg, 2012 ). For example, technology-enhanced assessments may involve a pedagogical agent for providing feedback during a learning process (Johnson & Lester, 2016 ). Other scenarios of technology-enhanced assessments include analyses of a learners’ decisions and interactions during game-based learning (Bellotti, Kapralos, Lee, Moreno-Ger, & Berta, 2013 ; Kim & Ifenthaler, 2019 ), scaffolding for dynamic task selection including related feedback (Corbalan, Kester, & van Merriënboer, 2009 ), remote asynchronous expert feedback on collaborative problem-solving tasks (Rissanen et al., 2008 ), or semantic rich and personalized feedback as well as adaptive prompts for reflection through data-driven assessments (Ifenthaler & Greiff, 2021 ; Schumacher & Ifenthaler, 2021 ).
It is expected that such technology-enhanced assessment systems meet a number of specific requirements, such as (a) adaptability to different subject domains, (b) flexibility for experimental as well as learning and teaching settings, (c) management of huge amounts of data, (d) rapid analysis of complex and unstructured data, (e) immediate feedback for learners and educators, as well as (f) generation of automated reports of results for educational decision-making.
Given the on-going developments in computer technology, data analytics, and artificial intelligence, there are advances in automated assessment systems, which may facilitate the feasibility, objectivity, reliability, and validity of the assessment of written prose as well as providing instant feedback during learning processes (Whitelock & Bektik, 2018 ). Accordingly, automated essay grading (AEG) systems, or automated essay scoring (AES systems, are defined as a computer-based process of applying standardized measurements on open-ended or constructed-response text-based test items. Measurements of written text include observable components such as content, style, organization, mechanics, and so forth (Shermis, Burstein, Higgins, & Zechner, 2010 ). As a result, the AES system generates a single score or detailed evaluation of predefined assessment features (Ifenthaler, 2016 ).
This chapter describes the evolution and features of automated scoring systems, discusses their limitations, and concludes with future directions for research and practice.
Synopsis of Automated Scoring Systems
The first widely known automated scoring system, Project Essay Grader (PEG), was conceptualized by Ellis Battan Page in late 1960s (Page, 1966 , 1968 ). PEG relies on proxy measures, such as average word length, essay length, number of certain punctuation marks, and so forth, to determine the quality of an open-ended response item. Despite the promising findings from research on PEG, acceptance and use of the system remained limited (Ajay, Tillett, & Page, 1973 ; Page, 1968 ). The advent of the Internet in the 1990s and related advances in hard- and software introduced a further interest in designing and implementing AES systems. The developers primarily aimed to address concerns with time, cost, reliability, and generalizability regarding the assessment of writing. AES systems have been used as a co-rater in large-scale standardized writing assessments since the late 1990s (e.g., e-rater by Educational Testing Service). While initial systems focused on English language, a wide variety of languages have been included in further developments, such as Arabic (Azmi, Al-Jouie, & Hussain, 2019 ), Bahasa Malay (Vantage Learning, 2002 ), Hebrew (Vantage Learning, 2001 ), German (Pirnay-Dummer & Ifenthaler, 2011 ), or Japanese (Kawate-Mierzejewska, 2003 ). More recent developments of AES systems utilize advanced machine learning approaches and elaborated natural language processing algorithms (Glavas, Ganesh, & Somasundaran, 2021 ).
For almost 60 years, different terms related to automated assessment of written prose have been used mostly interchangeably. Most frequently used terms are automated essay scoring (AES) and automated essay grading (AEG); however, more recent research used the term automated writing evaluation (AWE) and automated essay evaluation (AEE) (Zupanc & Bosnic, 2015 ). While the above-mentioned system focuses on written prose including several hundred words, another field developed focusing on short answers referred to as automatic short answer grading (ASAG) (Burrows, Gurevych, & Stein, 2015 ).
Functions of Automated Scoring Systems
AES systems mimic human evaluation of written prose by using various methods of scoring, that is, statistics, machine learning, and natural language processing (NLP) techniques. Implemented features of AES systems vary widely, yet they are mostly trained with large sets of expert-rated sample open-ended assessment items to internalize features that are relevant to human scoring. AES systems compare the features in training sets to those in new test items to find similarities between high/low scoring training and high/low scoring new ones and then apply scoring information gained from training sets to new item responses (Ifenthaler, 2016 ).
The underlying methodology of AES systems varies; however, recent research mainly focuses on natural language processing approaches (Glavas et al., 2021 ). AES systems focusing on content use Latent Semantic Analysis (LSA) which assumes that terms or words with similar meaning occur in similar parts of written text (Wild, 2016 ). Other content-related approaches include Pattern Matching Techniques (PMT). The idea of depicting semantic structures, which include concepts and relations between the concepts, has its source in two fields: semantics (especially propositional logic) and linguistics. Semantic oriented approaches include Ontologies and Semantic Networks (Pirnay-Dummer, Ifenthaler, & Seel, 2012 ). A semantic network represents information in terms of a collection of objects (nodes) and binary associations (directed labeled edges), the former standing for individuals (or concepts of some sort), and the latter standing for binary relations over these. Accordingly, a representation of knowledge in a written text by means of a semantic network corresponds with a graphical representation where each node denotes an object or concept, and each labeled being one of the relations used in the knowledge representation. Despite the differences between semantic networks, three types of edges are usually contained in all network representation schemas (Pirnay-Dummer et al., 2012 ): (a) Generalization: connects a concept with a more general one. The generalization relation between concepts is a partial order and organizes concepts into a hierarchy. (b) Individualization: connects an individual (token) with its generic type. (c) Aggregation: connects an object with its attributes (parts, functions) (e.g., wings – part of – bird). Another method of organizing semantic networks is partitioning which involves grouping objects and elements or relations into partitions that are organized hierarchically, so that if partition A is below partition B, everything visible or present in B is also visible in A unless otherwise specified (Hartley & Barnden, 1997 ).
From an information systems perspective, understood as a set of interrelated components that accumulate, process, store, and distribute information to support decision making, several preconditions and processes are required for a functioning AES system (Burrows et al., 2015 ; Pirnay-Dummer & Ifenthaler, 2010 ):
Assessment scenario: The assessment task with a specific focus on written prose needs to be designed and implemented. Written text is being collected from learners and from experts (being used as a reference for later evaluation).
Preparation: The written text may contain characters which could disturb the evaluation process. Thus, a specific character set is expected. All other characters may be deleted. Tags may be also deleted, as are other expected metadata within each text.
Tokenizing: The prepared text gets split into sentences and tokens. Tokens are words, punctuation marks, quotation marks, and so on. Tokenizing is somewhat language dependent, which means that different tokenizing methods are required for different languages.
Tagging: There are different approaches and heuristics for tagging sentences and tokens. A combination of rule-based and corpus-based tagging seems most feasible when the subject domain of the content is unknown to the AES system. Tagging and the rules for it is a quite complex field of linguistic methods (Brill, 1995 ).
Stemming: Specific assessment attributes may require that flexions of a word will be treated as one (e.g., the singular and plural forms “door” and “doors”). Stemming reduces all words to their word stems.
Analytics: Using further natural language processing (NLP) approaches, the prepared text is analyzed regarding predefined assessment attributes (see below), resulting in models and statistics.
Prediction: Further algorithms produce scores or other output variables based on the analytics results.
Veracity: Based on available historical data or reference data, the analytics scores are compared in order to build trust and validity in the AES result.
Common assessment attributes of AES have been identified by Zupanc and Bosnic ( 2017 ) including linguistic (lexical, grammar, mechanics), style, and content attributes. Among 28 lexical attributes, frequencies of characters, words, sentences are commonly used. More advanced lexical attributes include average sentence length, use of stopwords, variation in sentence length, or the variation of specific words. Other lexical attributes focus on readability or lexical diversity utilizing specific measures such as Gunning Fox index, Nominal ratio, Type-token-ratio (DuBay, 2007 ). Another 37 grammar attributes are frequently implemented, such as number of grammar errors, complexity of sentence tree structure, use of prepositions and forms of adjectives, adverbs, nouns, verbs. A few attributes focus on mechanics, for example, the number of spellchecking errors, the number of capitalization errors, or punctuation errors. Attributes that focus on content include similarities with source or reference texts or content-related patterns (Attali, 2011 ). Specific semantic attributes have been described as concept matching and proposition matching (Ifenthaler, 2014 ). Both attributes are based on similarity measures (Tversky, 1977 ). Concept matching compares the sets of concepts (single words) within a written text to determine the use of terms. This measure is especially important for different assessments which operate in the same domain. Propositional matching compares only fully identical propositions between two knowledge representations. It is a good measure for quantifying complex semantic relations in a specific subject domain. Balanced semantic matching measure uses both concepts and propositions to match the semantic potential between the knowledge representations. Such content or semantic oriented attributes focus on the correctness of content and its meaning (Ifenthaler, 2014 ).
Overview of Automated Scoring Systems
Instructional applications of automated scoring systems are developed to facilitate the process of scoring and feedback in writing classrooms. These AES systems mimic human scoring by using various attributes; however, implemented attributes vary widely.
The market of commercial and open-source AES systems has seen a steady growth since the introduction of PEG. The majority of available AES systems extract a set of attributes from written prose and analyze it using some algorithm to generate a final output. Several overviews document the distinct features of AES systems (Dikli, 2011 ; Ifenthaler, 2016 ; Ifenthaler & Dikli, 2015 ; Zupanc & Bosnic, 2017 ). Burrows et al. ( 2015 ) identified five eras throughout the almost 60 years of research in AES: (1) concept mapping, (2) information extraction, (3) corpus-based methods, (4) machine learning, and (5) evaluation.
Zupanc and Bosnic ( 2017 ) note that four commercial AES systems have been predominant in application: PEG, e-rater, IEA, and IntelliMetric. Open access or open code systems have been available for research purposes (e.g., AKOVIA); however, they are yet to be made available to the general public. Table 1 provides an overview of current AES systems, including a short description of the applied assessment methodology, output features, information about test quality, and specific requirements. The overview is far from being complete; however, it includes major systems which have been reported in previous summaries and systematic literature reviews on AES systems (Burrows et al., 2015 ; Dikli, 2011 ; Ifenthaler, 2016 ; Ifenthaler & Dikli, 2015 ; Ramesh & Sanampudi, 2021 ; Zupanc & Bosnic, 2017 ). Several AES systems also have instructional versions for classroom use. In addition to their instant scoring capacity on a holistic scale, the instructional AES systems are capable of generating diagnostic feedback and scoring on an analytic scale as well. The majority of AES systems use focus on style or content-quality and use NLP algorithms in combination with variations of regression models. Depending on the methodology, AES system requires training samples for building a reference for future comparisons. However, the test quality, precision, or accuracy of several AES systems is publicly not available or has not been reported in rigorous empirical research (Wilson & Rodrigues, 2020 ).
Open Questions and Directions for Research
There are several concerns regarding the precision of AES systems and the lack of semantic interpretation capabilities of underlying algorithms. Reliability and validity of AES systems have been extensively investigated (Landauer, Laham, & Foltz, 2003 ; Shermis et al., 2010 ). The correlations and agreement rates between AES systems and expert human raters have been found to be fairly high; however, the agreement rate is not at the desired level yet (Gierl, Latifi, Lai, Boulais, & Champlain, 2014 ). It should be noted that many of these studies highlight the results of adjacent agreement between humans and AES systems rather than those of exact agreement (Ifenthaler & Dikli, 2015 ). Exact agreement is harder to achieve as it requires two or more raters to assign the same exact score on an essay while adjacent agreement requires two or more raters to assign a score within one scale point of each other. It should also be noted that correlation studies are mostly conducted at high-stakes assessment settings rather than classroom settings; therefore, AES versus human inter-rater reliability rates may not be the same in specific assessment settings. The rate is expected to be lower in the latter since the content of an essay is likely to be more important in low-stakes assessment contexts.
The validity of AES systems has been critically reflected since the introduction of the initial applications (Page, 1966 ). A common approach for testing validity is the comparison of scores from AES systems with those of human experts (Attali & Burstein, 2006 ). Accordingly, questions arise about the role of AES systems promoting purposeful writing or authentic open-ended assessment responses, because the underlying algorithms view writing as a formulaic act and allows writers to concentrate more on the formal aspects of language such as origin, vocabulary, grammar, and text length with little or no attention to the meaning of the text (Ifenthaler, 2016 ). Validation of AES systems may include the correct use of specific assessment attributes, the openness of algorithms, and underlying aggregation and analytics techniques, as well as a combination of human and automated approaches before communicating results to learners (Attali, 2013 ). Closely related to the issue of validity is the concern regarding reliability of AES systems. In this context, reliability assumes that AES systems produce repeatedly consistent scores within and across different assessment conditions (Zupanc & Bosnic, 2015 ). Another concern is the bias of underlying algorithms, that is, algorithms have their source in a human programmer which may introduce additional error structures or even features of discrimination (e.g., cultural bias based on selective text corpora). Criticism has been put toward commercial marketing of AES systems for speakers of English as a second or foreign language (ESL/EFL) when the underlying methodology has been developed based on English language with native-English speakers in mind. In an effort to assist ESL/EFL speakers in writing classrooms, many developers have incorporated a multilingual feedback function in the instructional versions of AES systems. Receiving feedback in the first language has proven benefits, yet it may not be sufficient for ESL/EFL speakers to improve their writing in English. It would be more beneficial for non-native speakers of English if developers take common ESL/EFL errors into consideration when they build algorithms in AES systems. Another area of concern is that writers can trick AES systems. For instance, if the written text produced is long and includes certain type of vocabulary that the AES system is familiar with, an essay can receive a higher score from AES regardless of the quality of its content. Therefore, developers have been trying to prevent cheating by users through incorporating additional validity algorithms (e.g., flagging written text with unusual elements for human scoring) (Ifenthaler & Dikli, 2015 ). The validity and reliability concerns result in speculations regarding the credibility of AES systems considering that the majority of the research on AES is conducted or sponsored by the developing companies. Hence, there is a need for more research that addresses the validity and reliability issues raised above and preferably those conducted by independent researchers (Kumar & Boulanger, 2020 ).
Despite the above-mentioned concerns and limitation, educational organizations choose to incorporate instructional applications of AES systems in classrooms, mainly to increase student motivation toward writing and reducing workload of involved teachers. They assume that if AES systems assist students with the grammatical errors in their writings, teachers will have more time to focus on content related issues. Still, research on students’ perception on AES systems and the effect on motivation as well as on learning processes and learning outcomes is scarce (Stephen, Gierl, & King, 2021 ). In contrast, educational organizations are hesitant in implementing AES systems mainly because of validity issues related to domain knowledge-based evaluation. As Ramesh and Sanampudi ( 2021 ) exemplify, the domain-specific meaning of “cell” may be different in biology or physics. Other concerns that may lower the willingness to adopt of AES systems in educational organizations include fairness, consistency, transparency, privacy, security, and ethical issues (Ramineni & Williamson, 2013 ; Shermis, 2010 ).
AES systems can make the result of an assessment available instantly and may produce immediate feedback whenever the learner needs it. Such instant feedback provides autonomy to the learner during the learning process, that is, learners are not depended on possibly delayed feedback from teachers. Several attributes implemented in AES systems can produce an automated score, for instance, correctness of syntactic aspects. Still, the automated and informative feedback regarding content and semantics is limited. Alternative feedback mechanisms have been suggested, for example, Automated Knowledge Visualization and Assessment (AKOVIA) provides automated graphical feedback models, generated on the fly, which have been successfully tested for preflection and reflection in problem-based writing tasks (Lehmann, Haehnlein, & Ifenthaler, 2014 ). Other studies using AKOVIA feedback models highlight the benefits of availability of informative feedback whenever the learner needs it and its identical impact on problem solving when compared with feedback models created by domain experts (Ifenthaler, 2014 ).
Questions for future research focusing on AES systems may focus on (a) construct validity (i.e., comparing AES systems with other systems or human rater results), (b) interindividual and intraindividual consistency and robustness of AES scores obtained (e.g., in comparison with different assessment tasks), (c) correlative nature of AES scores with other pedagogical or psychological measures (e.g., interest, intelligence, prior knowledge), (d) fairness and transparency of AES systems and related scores, as well as (e) ethical concerns related to AES systems, (f) (Elliot & Williamson, 2013 ). From a technological perspective, (f) the feasibility of the automated scoring system (including training of AES using prescored, expert/reference, comparison) is still a key issue with regard to the quality of assessment results. Other requirements include the (g) instant availability, accuracy, and confidence of the automated assessment. From a pedagogical perspective, (h) the form of the open-ended or constructed-response test needs to be considered. The (i) assessment capabilities of the AES system, such as the assessment of different languages, content-oriented assessment, coherence assessment (e.g., writing style, syntax, spelling), domain-specific features assessment, and plagiarism detection, are critical for a large-scale implementation. Further, (j) the form of feedback generated by the automated scoring system might include simple scoring but also rich semantic and graphical feedback. Finally, (k) the integration of an AES system into existing applications, such as learning management systems, needs to be further investigated by developers, researchers, and practitioners.
Implications for Open, Distance, and Digital Education
The evolution of Massive Open Online Courses (MOOCs) nurtured important questions about online education and its automated assessment (Blackmon & Major, 2017 ; White, 2014 ). Education providers such as Coursera, edX, and Udacity dominantly apply so-called auto-graded assessments (e.g., single- or multiple-choice assessments). Implementing automated scoring for open-ended assessments is still on the agenda of such provides, however, not fully developed yet (Corbeil, Khan, & Corbeil, 2018 ).
With the increased availability of vast and highly varied amounts of data from learners, teachers, learning environments, and administrative systems within educational settings, further opportunities arise for advancing AES systems in open, distance, and digital education. Analytics-enhanced assessment enlarges standard methods of AES systems through harnessing formative as well as summative data from learners and their contexts in order to facilitate learning processes in near real-time and help decision-makers to improve learning environments. Hence, analytics-enhanced assessment may provide multiple benefits for students, schools, and involved stakeholders. However, as noted by Ellis ( 2013 ), analytics currently fail to make full use of educational data for assessment.
Interest in collecting and mining large sets of educational data on student background and performance has grown over the past years and is generally referred to as learning analytics (R. S. Baker & Siemens, 2015 ). In recent years, the incorporation of learning analytics into educational practices and research has further developed. However, while new applications and approaches have brought forth new insights, there is still a shortage of research addressing the effectiveness and consequences with regard to AES systems. Learning analytics, which refers to the use of static and dynamic data from learners and their contexts for (1) the understanding of learning and the discovery of traces of learning and (2) the support of learning processes and educational decision-making (Ifenthaler, 2015 ), offers a range of opportunities for formative and summative assessment of written text. Hence, the primary goal of learning analytics is to better meet students’ needs by offering individual learning paths, adaptive assessments and recommendations, or adaptive and just-in-time feedback (Gašević, Dawson, & Siemens, 2015 ; McLoughlin & Lee, 2010 ), ideally, tailored to learners’ motivational states, individual characteristics, and learning goals (Schumacher & Ifenthaler, 2018 ). From an assessment perspective focusing on AES systems, learning analytics for formative assessment focuses on the generation and interpretation of evidence about learner performance by teachers, learners, and/or technology to make assisted decisions about the next steps in learning and instruction (Ifenthaler, Greiff, & Gibson, 2018 ; Spector et al., 2016 ). In this context, real- or near-time data are extremely valuable because of their benefits in ongoing learning interactions. Learning analytics for written text from a summative assessment perspective is utilized to make judgments that are typically based on standards or benchmarks (Black & Wiliam, 1998 ).
In conclusion, analytics-enhanced assessments of written essays may reveal personal information and insights into an individual learning history; however, they are not accredited and far from being unbiased, comprehensive, and fully valid at this point in time. Much remains to be done to mitigate these shortcomings in a way that learners will truly benefit from AES systems.
Cross-References
Artificial Intelligence in Education and Ethics
Evolving Learner Support Systems
Introduction to Design, Delivery, and Assessment in ODDE
Learning Analytics in Open, Distance, and Digital Education (ODDE)
Ajay, H. B., Tillett, P. I., & Page, E. B. (1973). The analysis of essays by computer (AEC-II). Final report . Storrs, CT: University of Connecticut.
Google Scholar
Attali, Y. (2011). A differential word use measure for content analysis in automated essay scoring . ETS Research Report Series , 36.
Attali, Y. (2013). Validity and reliability of automated essay scoring. In M. D. Shermis & J. Burstein (Eds.), Handbook of automated essay evaluation: Current applications and new directions (pp. 181–198). New York, NY: Routledge.
Attali, Y., & Burstein, J. (2006). Automated essay scoring with e-rater V. 2. The Journal of Technology, Learning and Assessment, 4 (3), 3–29. https://doi.org/10.1002/j.2333-8504.2004.tb01972.x .
Article Google Scholar
Azmi, A., Al-Jouie, M. F., & Hussain, M. (2019). AAEE – Automated evaluation of students‘ essays in Arabic language. Information Processing & Management, 56 (5), 1736–1752. https://doi.org/10.1016/j.ipm.2019.05.008 .
Baker, E., Chung, G., & Cai, L. (2016). Assessment, gaze, refraction, and blur: The course of achievement testing in the past 100 years. Review of Research in Education, 40 , 94–142. https://doi.org/10.3102/0091732X16679806 .
Baker, R. S., & Siemens, G. (2015). Educational data mining and learning analytics. In R. K. Sawyer (Ed.), The Cambridge handbook of the learning sciences (2nd ed., pp. 253–272). Cambridge, UK: Cambridge University Press.
Bellotti, F., Kapralos, B., Lee, K., Moreno-Ger, P., & Berta, R. (2013). Assessment in and of serious games: An overview. Advances in Human-Computer Interaction, 2013 , 136864. https://doi.org/10.1155/2013/136864 .
Bennett, R. E. (2015). The changing nature of educational assessment. Review of Research in Education, 39 (1), 370–407. https://doi.org/10.3102/0091732x14554179 .
Black, P., & Wiliam, D. (1998). Assessment and classroom learning. Assessment in Education: Principles, Policy & Practice, 5 (1), 7–74. https://doi.org/10.1080/0969595980050102 .
Blackmon, S. J., & Major, C. H. (2017). Wherefore art thou MOOC?: Defining massive open online courses. Online Learning Journal, 21 (4), 195–221. https://doi.org/10.24059/olj.v21i4.1272 .
Brill, E. (1995). Unsupervised learning of dismabiguation rules for part of speech tagging. Paper presented at the Second Workshop on Very Large Corpora, WVLC-95, Boston. Paper presentation retrieved from
Burrows, S., Gurevych, I., & Stein, B. (2015). The eras and trends of automatic short answer grading. International Journal of Artificial Intelligence in Education, 25 (1), 60–117. https://doi.org/10.1007/s40593-014-0026-8 .
Corbalan, G., Kester, L., & van Merriënboer, J. J. G. (2009). Dynamic task selection: Effects of feedback and learner control on efficiency and motivation. Learning and Instruction, 19 (6), 455–465. https://doi.org/10.1016/j.learninstruc.2008.07.002 .
Corbeil, J. R., Khan, B. H., & Corbeil, M. E. (2018). MOOCs revisited: Still transformative or passing fad? Asian Journal of University Education, 14 (2), 1–12.
Dikli, S. (2011). The nature of automated essay scoring feedback. CALICO Journal, 28 (1), 99–134. https://doi.org/10.11139/cj.28.1.99-134 .
DuBay, W. H. (2007). Smart language: Readers, readability, and the grading of text . Costa Mesa, CA, USA: BookSurge Publishing.
Elliot, N., & Williamson, D. M. (2013). Assessing writing special issue: Assessing writing with automated scoring systems. Assessing Writing, 18 (1), 1–6. https://doi.org/10.1016/j.asw.2012.11.002 .
Ellis, C. (2013). Broadening the scope and increasing usefulness of learning analytics: The case for assessment analytics. British Journal of Educational Technology, 44 (4), 662–664. https://doi.org/10.1111/bjet.12028 .
Flower, L., & Hayes, J. (1981). A cognitive process theory of writing. College Composition and Communication, 32 (4), 365–387.
Gašević, D., Dawson, S., & Siemens, G. (2015). Let’s not forget: Learning analytics are about learning. TechTrends, 59 (1), 64–71. https://doi.org/10.1007/s11528-014-0822-x .
Gierl, M. J., Latifi, S., Lai, H., Boulais, A.-P., & Champlain, A. (2014). Automated essay scoring and the future of educational assessment in medical education. Medical Education, 48 (10), 950–962. https://doi.org/10.1111/medu.12517 .
Glavas, G., Ganesh, A., & Somasundaran, S. (2021). Training and domain adaptation for supervised text segmentation. Paper presented at the Proceedings of the 16th Workshop on Innovative Use of NLP for Building Educational Applications, Virtual Conference.
Hartley, R. T., & Barnden, J. A. (1997). Semantic networks: Visualizations of knowledge. Trends in Cognitive Science, 1 (5), 169–175. https://doi.org/10.1016/S1364-6613(97)01057-7 .
Ifenthaler, D. (2014). AKOVIA: Automated knowledge visualization and assessment. Technology, Knowledge and Learning, 19 (1–2), 241–248. https://doi.org/10.1007/s10758-014-9224-6 .
Ifenthaler, D. (2015). Learning analytics. In J. M. Spector (Ed.), The SAGE encyclopedia of educational technology (Vol. 2, pp. 447–451). Thousand Oaks, CA: Sage.
Ifenthaler, D. (2016). Automated grading. In S. Danver (Ed.), The SAGE encyclopedia of online education (p. 130). Thousand Oaks, CA: Sage.
Ifenthaler, D., & Dikli, S. (2015). Automated scoring of essays. In J. M. Spector (Ed.), The SAGE encyclopedia of educational technology (Vol. 1, pp. 64–68). Thousand Oaks, CA: Sage.
Ifenthaler, D., & Greiff, S. (2021). Leveraging learning analytics for assessment and feedback. In J. Liebowitz (Ed.), Online learning analytics (pp. 1–18). Boca Raton, FL: Auerbach Publications.
Ifenthaler, D., Greiff, S., & Gibson, D. C. (2018). Making use of data for assessments: Harnessing analytics and data science. In J. Voogt, G. Knezek, R. Christensen, & K.-W. Lai (Eds.), International handbook of IT in primary and secondary education (2nd ed., pp. 649–663). New York, NY: Springer.
Johnson, W. L., & Lester, J. C. (2016). Face-to-face interaction with pedagogical agents, twenty years later. International Journal of Artificial Intelligence in Education, 26 (1), 25–36. https://doi.org/10.1007/s40593-015-0065-9 .
Kawate-Mierzejewska, M. (2003). E-rater software . Paper presented at the Japanese Association for Language Teaching, Tokyo, Japan. Paper presentation retrieved from
Kim, Y. J., & Ifenthaler, D. (2019). Game-based assessment: The past ten years and moving forward. In D. Ifenthaler & Y. J. Kim (Eds.), Game-based assessment revisted (pp. 3–12). Cham, Switzerland: Springer.
Chapter Google Scholar
Kumar, V. S., & Boulanger, D. (2020). Automated essay scoring and the deep learning black box: How are rubric scores determined? International Journal of Artificial Intelligence in Education . https://doi.org/10.1007/s40593-020-00211-5 .
Landauer, T. K., Laham, D., & Foltz, P. W. (2003). Automated scoring and annotation of essays with the intelligent essay assessor. In M. D. Shermis & J. Burstein (Eds.), Automated essay scoring: A cross-disciplinary perspective (pp. 87–112). Mahwah, NJ: Erlbaum.
Lehmann, T., Haehnlein, I., & Ifenthaler, D. (2014). Cognitive, metacognitive and motivational perspectives on preflection in self-regulated online learning. Computers in Human Behavior, 32 , 313–323. https://doi.org/10.1016/j.chb.2013.07.051 .
McLoughlin, C., & Lee, M. J. W. (2010). Personalized and self regulated learning in the Web 2.0 era: International exemplars of innovative pedagogy using social software. Australasian Journal of Educational Technology, 26 (1), 28–43.
Page, E. B. (1966). The imminence of grading essays by computer. Phi Delta Kappan, 47 (5), 238–243.
Page, E. B. (1968). The use of the computer in analyzing student essays. International Review of Education, 14 (2), 210–225. https://doi.org/10.1007/BF01419938 .
Pirnay-Dummer, P., & Ifenthaler, D. (2010). Automated knowledge visualization and assessment. In D. Ifenthaler, P. Pirnay-Dummer, & N. M. Seel (Eds.), Computer-based diagnostics and systematic analysis of knowledge (pp. 77–115). New York, NY: Springer.
Pirnay-Dummer, P., & Ifenthaler, D. (2011). Text-guided automated self assessment. A graph-based approach to help learners with ongoing writing. In D. Ifenthaler, K. P. Isaias, D. G. Sampson, & J. M. Spector (Eds.), Multiple perspectives on problem solving and learning in the digital age (pp. 217–225). New York, NY: Springer.
Pirnay-Dummer, P., Ifenthaler, D., & Seel, N. M. (2012). Semantic networks. In N. M. Seel (Ed.), Encyclopedia of the sciences of learning (Vol. 19, pp. 3025–3029). New York, NY: Springer.
Ramesh, D., & Sanampudi, S. K. (2021). An automated essay scoring systems: A systematic literature review. Artificial Intelligence Review . https://doi.org/10.1007/s10462-021-10068-2 .
Ramineni, C., & Williamson, D. M. (2013). Automated essay scoring: Psychometric guidelines and practices. Assessing Writing, 18 (1), 25–39. https://doi.org/10.1016/j.asw.2012.10.004 .
Rissanen, M. J., Kume, N., Kuroda, Y., Kuroda, T., Yoshimura, K., & Yoshihara, H. (2008). Asynchronous teaching of psychomotor skills through VR annotations: Evaluation in digital rectal examination. Studies in Health Technology and Informatics, 132 , 411–416.
Schumacher, C., & Ifenthaler, D. (2018). The importance of students’ motivational dispositions for designing learning analytics. Journal of Computing in Higher Education, 30 (3), 599–619. https://doi.org/10.1007/s12528-018-9188-y .
Schumacher, C., & Ifenthaler, D. (2021). Investigating prompts for supporting students’ self-regulation – A remaining challenge for learning analytics approaches? The Internet and Higher Education, 49 , 100791. https://doi.org/10.1016/j.iheduc.2020.100791 .
Shermis, M. D. (2010). Automated essay scoring in a high stakes testing environment. In V. J. Shute & B. J. Becker (Eds.), Innovative assessment for the 21st century (pp. 167–184). New York, NY: Springer.
Shermis, M. D., Burstein, J., Higgins, D., & Zechner, K. (2010). Automated essay scoring: Writing assessment and instruction. In P. Petersen, E. Baker, & B. McGaw (Eds.), International encyclopedia of education (pp. 75–80). Oxford, England: Elsevier.
Spector, J. M., Ifenthaler, D., Sampson, D. G., Yang, L., Mukama, E., Warusavitarana, A., … Gibson, D. C. (2016). Technology enhanced formative assessment for 21st century learning. Educational Technology & Society, 19 (3), 58–71.
Stephen, T. C., Gierl, M. C., & King, S. (2021). Automated essay scoring (AES) of constructed responses in nursing examinations: An evaluation. Nurse Education in Practice, 54 , 103085. https://doi.org/10.1016/j.nepr.2021.103085 .
Stödberg, U. (2012). A research review of e-assessment. Assessment & Evaluation in Higher Education, 37 (5), 591–604. https://doi.org/10.1080/02602938.2011.557496 .
Tversky, A. (1977). Features of similarity. Psychological Review, 84 , 327–352.
Valenti, S., Neri, F., & Cucchiarelli, A. (2003). An overview of current research on automated essay grading. Journal of Information Technology Education, 2 , 319–330.
Vantage Learning. (2001). A preliminary study of the efficacy of IntelliMetric ® for use in scoring Hebrew assessments . Retrieved from Newtown, PA:
Vantage Learning. (2002). A study of IntelliMetric ® scoring for responses written in Bahasa Malay (No. RB-735) . Retrieved from Newtown, PA:
Webb, M., Gibson, D. C., & Forkosh-Baruch, A. (2013). Challenges for information technology supporting educational assessment. Journal of Computer Assisted Learning, 29 (5), 451–462. https://doi.org/10.1111/jcal.12033 .
Webb, M., & Ifenthaler, D. (2018). Section introduction: Using information technology for assessment: Issues and opportunities. In J. Voogt, G. Knezek, R. Christensen, & K.-W. Lai (Eds.), International handbook of IT in primary and secondary education (2nd ed., pp. 577–580). Cham, Switzerland: Springer.
White, B. (2014). Is “MOOC-mania” over? In S. S. Cheung, J. Fong, J. Zhang, R. Kwan, & L. Kwok (Eds.), Hybrid learning. Theory and practice (Vol. 8595, pp. 11–15). Cham, Switzerland: Springer International Publishing.
Whitelock, D., & Bektik, D. (2018). Progress and challenges for automated scoring and feedback systems for large-scale assessments. In J. Voogt, G. Knezek, R. Christensen, & K.-W. Lai (Eds.), International handbook of IT in primary and secondary education (2nd ed., pp. 617–634). New York, NY: Springer.
Wild, F. (2016). Learning analytics in R with SNA, LSA, and MPIA . Heidelberg, Germany: Springer.
Book Google Scholar
Wilson, J., & Rodrigues, J. (2020). Classification accuracy and efficiency of writing screening using automated essay scoring. Journal of School Psychology, 82 , 123–140. https://doi.org/10.1016/j.jsp.2020.08.008 .
Zupanc, K., & Bosnic, Z. (2015). Advances in the field of automated essay evaluation. Informatica, 39 (4), 383–395.
Zupanc, K., & Bosnic, Z. (2017). Automated essay evaluation with semantic analysis. Knowledge-Based Systems, 120 , 118–132. https://doi.org/10.1016/j.knosys.2017.01.006 .
Download references
Author information
Authors and affiliations.
University of Mannheim, Mannheim, Germany
Dirk Ifenthaler
Curtin University, Perth, WA, Australia
You can also search for this author in PubMed Google Scholar
Corresponding author
Correspondence to Dirk Ifenthaler .
Editor information
Editors and affiliations.
Center of Open Education Research, Carl von Ossietzky University of Oldenburg, Oldenburg, Niedersachsen, Germany
Olaf Zawacki-Richter
Education Research Institute, Seoul National University, Seoul, Korea (Republic of)
Insung Jung
Section Editor information
Florida State University, Tallahassee, FL, USA
Vanessa Dennen
Rights and permissions
Open Access This chapter is licensed under the terms of the Creative Commons Attribution 4.0 International License ( http://creativecommons.org/licenses/by/4.0/ ), which permits use, sharing, adaptation, distribution and reproduction in any medium or format, as long as you give appropriate credit to the original author(s) and the source, provide a link to the Creative Commons license and indicate if changes were made.
The images or other third party material in this chapter are included in the chapter's Creative Commons license, unless indicated otherwise in a credit line to the material. If material is not included in the chapter's Creative Commons license and your intended use is not permitted by statutory regulation or exceeds the permitted use, you will need to obtain permission directly from the copyright holder.
Reprints and permissions
Copyright information
© 2023 The Author(s)
About this entry
Cite this entry.
Ifenthaler, D. (2023). Automated Essay Scoring Systems. In: Zawacki-Richter, O., Jung, I. (eds) Handbook of Open, Distance and Digital Education. Springer, Singapore. https://doi.org/10.1007/978-981-19-2080-6_59
Download citation
DOI : https://doi.org/10.1007/978-981-19-2080-6_59
Published : 01 January 2023
Publisher Name : Springer, Singapore
Print ISBN : 978-981-19-2079-0
Online ISBN : 978-981-19-2080-6
eBook Packages : Education Reference Module Humanities and Social Sciences Reference Module Education
Share this entry
Anyone you share the following link with will be able to read this content:
Sorry, a shareable link is not currently available for this article.
Provided by the Springer Nature SharedIt content-sharing initiative
- Publish with us
Policies and ethics
- Find a journal
- Track your research
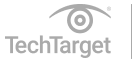
Data Science Central
- Author Portal
- 3D Printing
- AI Data Stores
- AI Hardware
- AI Linguistics
- AI User Interfaces and Experience
- AI Visualization
- Cloud and Edge
- Cognitive Computing
- Containers and Virtualization
- Data Science
- Data Security
- Digital Factoring
- Drones and Robot AI
- Internet of Things
- Knowledge Engineering
- Machine Learning
- Quantum Computing
- Robotic Process Automation
- The Mathematics of AI
- Tools and Techniques
- Virtual Reality and Gaming
- Blockchain & Identity
- Business Agility
- Business Analytics
- Data Lifecycle Management
- Data Privacy
- Data Strategist
- Data Trends
- Digital Communications
- Digital Disruption
- Digital Professional
- Digital Twins
- Digital Workplace
- Marketing Tech
- Sustainability
- Agriculture and Food AI
- AI and Science
- AI in Government
- Autonomous Vehicles
- Education AI
- Energy Tech
- Financial Services AI
- Healthcare AI
- Logistics and Supply Chain AI
- Manufacturing AI
- Mobile and Telecom AI
- News and Entertainment AI
- Smart Cities
- Social Media and AI
- Functional Languages
- Other Languages
- Query Languages
- Web Languages
- Education Spotlight
- Newsletters
- O’Reilly Media
Automated Grading Systems: How AI is Revolutionizing Exam Evaluation
- May 31, 2023 at 9:26 am September 14, 2024 at 7:33 am
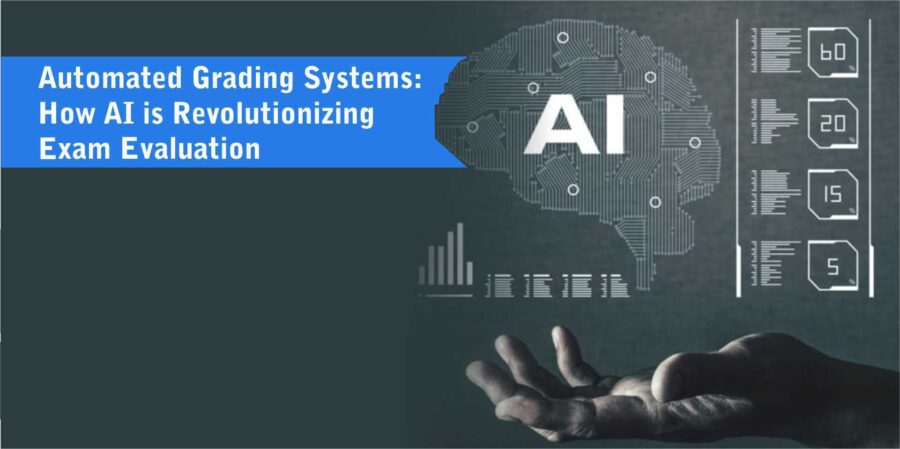
As technology continues to advance rapidly, the realm of education is not immune to its transformative effects. One area that has seen significant progress is exam evaluation. Traditionally, grading exams has been a time-consuming and subjective process, prone to human error and bias. However, with the emergence of automated grading systems powered by Artificial Intelligence (AI), the landscape of exam evaluation is undergoing a revolutionary change. In this blog post, we will explore the benefits of automated grading systems, discuss how AI is transforming exam evaluation, and highlight the role of AI courses offered by Great Learning in shaping this revolutionary field.
The Benefits of Automated Grading Systems
Automated grading systems bring a host of advantages to the process of exam evaluation. Here are a few key benefits:
Efficiency:
Manual grading exams can be labor-intensive, especially in large educational institutions. Automated grading systems significantly reduce the time and effort required to evaluate exams, enabling teachers and professors to focus on other critical aspects of education, such as lesson planning and personalized student feedback.
Consistency:
Human graders may introduce inconsistencies in their evaluation due to fatigue, personal biases, or varying interpretations of grading rubrics. Automated grading systems, on the other hand, follow a predefined set of rules and standards consistently, ensuring fair and objective evaluations for all students.
With AI-powered grading systems, exams can be evaluated and results generated in a fraction of the time it would take manually. This not only benefits students by providing timely feedback but also allows educational institutions to streamline their administrative processes.
Scalability:
As educational institutions continue to grow, the demand for efficient grading systems increases. Automated grading systems can easily handle many exams, making them highly scalable solutions that can adapt to changing educational needs.
How AI is Transforming Exam Evaluation?
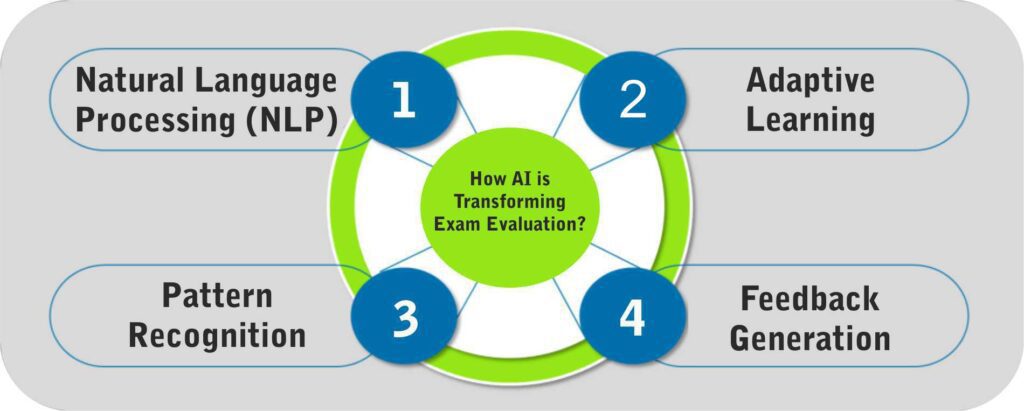
Artificial Intelligence plays a vital role in revolutionizing exam evaluation through automated grading systems. Here are some ways in which AI technology is transforming this field:
Natural Language Processing (NLP):
NLP algorithms enable automated grading systems to analyze and understand written responses. By employing machine learning techniques, AI can assess the quality, coherence, and relevance of the student’s answers, providing valuable insights into their understanding of the subject matter.
Pattern Recognition:
AI-powered systems can recognize patterns in student responses and identify common errors or misconceptions. This allows educators to pinpoint areas where students may be struggling and tailor their teaching strategies accordingly.
Adaptive Learning:
AI-based grading systems, empowered by the knowledge and techniques acquired through an artificial intelligence course , can adapt and improve over time. By analyzing vast amounts of student data, these systems can identify areas of weakness and develop personalized feedback and recommendations for individual learners. This personalized approach enhances the learning experience and helps students progress at their own pace.
Feedback Generation:
AI algorithms can generate detailed feedback for students, highlighting their strengths and improvement areas. This feedback not only guides students but also saves educators’ time by automating the process of generating individualized feedback.
Accuracy and Efficiency: How Automated Grading Systems Improve Exam Assessment
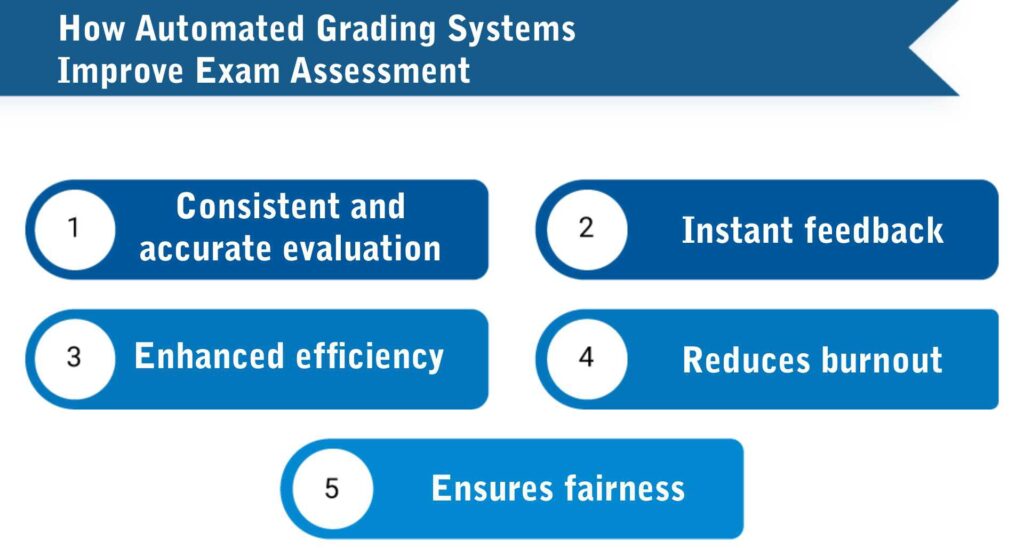
Automated grading systems powered by artificial intelligence (AI) have revolutionized the exam evaluation process, increasing accuracy and efficiency. Traditional manual grading methods are time-consuming and susceptible to human errors and subjectivity. In contrast, automated grading systems offer a reliable and objective approach to assessing exams.
- Consistent and accurate evaluation: AI algorithms can recognize patterns and evaluate responses based on predefined criteria, ensuring consistent and impartial grading.
- Instant feedback: Automated grading systems provide students with immediate feedback, allowing them to promptly understand their mistakes and areas for improvement.
- Enhanced efficiency: Automated systems can process exams at a faster pace than manual grading, reducing the workload on educators and freeing up their time for other important tasks.
- Reduces burnout: By automating the grading process, educators are relieved from the daunting task of evaluating a large number of exams within a limited timeframe, reducing the risk of burnout.
- Ensures fairness: Automated grading eliminates subjective biases that can arise from human grading, promoting fairness in the assessment process.
Overcoming Challenges: Implementing Automated Grading Systems in Educational Institutions
The implementation of automated grading systems in educational institutions presents various challenges that need to be addressed for successful integration. While these systems offer numerous benefits, their adoption requires careful planning, collaboration, and overcoming certain hurdles.
- Compatibility with existing assessment practices and infrastructure: Ensuring that automated grading systems align with established evaluation methods, grading scales, and software systems may require modifications or adaptations. Collaborative efforts between administrators, educators, and technology experts are crucial in addressing this challenge.
- Training and familiarization of teachers: Educators need to understand how automated grading systems work, their limitations, and how to interpret and utilize the generated results effectively. Professional development programs and training workshops can equip teachers with the necessary skills and knowledge for incorporating automated grading systems into their teaching practices.
- Privacy and data security: Protecting student data and complying with privacy regulations are essential considerations. Implementing robust security measures, obtaining consent from students and parents, and transparently communicating data handling practices are necessary steps to address privacy and data security concerns.
The Role of Teachers in an AI-Driven Grading Environment
As automated grading systems powered by artificial intelligence (AI) become more prevalent in educational institutions, the role of teachers in an AI-driven grading environment evolves and expands. While these systems handle the bulk of exam evaluation, the importance of teachers in providing guidance, support, and context remains crucial for holistic student development.
- Setting assessment criteria and standards: Teachers define the rubrics and guidelines used by automated grading systems, ensuring alignment with educational objectives and desired learning outcomes.
- Interpreting and analyzing results: Teachers bring a human touch by understanding the context of student responses and providing nuanced feedback. They can identify patterns, offer individualized support, and guide students based on their strengths, weaknesses, and learning styles.
- Personalized guidance and support: Teachers act as mentors and motivators, providing emotional support and encouragement to students. They foster a positive learning environment, inspire critical thinking, and cultivate a growth mindset. Teachers can also identify students who may require additional assistance and provide tailored support.
- Going beyond automated grading: Teachers offer support and interventions that extend beyond the scope of automated grading systems. They provide personalized attention, address individual student needs, and offer academic and personal development guidance.
Automated grading systems powered by Artificial Intelligence are transforming the way exams are evaluated. With their efficiency, consistency, speed, and scalability, these systems offer numerous benefits to both educators and students. AI technology, including natural language processing, pattern recognition, and adaptive learning, plays a pivotal role in revolutionizing exam evaluation. Artificial Intelligence courses provide learners with the necessary skills and expertise to thrive in this field and contribute to the advancement of automated grading systems. By leveraging the knowledge gained from MIT’s AI course , learners can become catalysts for change in education, applying cutting-edge AI techniques to enhance exam evaluation methods. Embracing the potential of AI in exam evaluation will undoubtedly lead to a more efficient, accurate, and personalized learning experience for students worldwide.
Related Content

- Corpus ID: 1399957
Benefits of Modularity in an Automated Essay Scoring System
- J. Burstein , D. Marcu
- Published in International Conference on… 5 August 2000
- Computer Science, Linguistics
15 Citations
Automated essay scoring..
- Highly Influenced
- 12 Excerpts
The Effect of Specific Language Features on the Complexity of Systems for Automated Essay Scoring.
Towards the use of semi-structured annotators for automated essay grading, an automated grader for chinese essay combining shallow and deep semantic attributes, embedding information retrieval and nearest-neighbour algorithm into automated essay grading system, evaluating computer automated scoring: issues, methods, and an empirical illustration, an overview of automated scoring of essays., invoking the cyber-muse: automatic essay assessment in the online learning environment, automatic essay grading with probabilistic latent semantic analysis, automatic essay scoring of swedish essays using neural networks, 13 references, automated scoring using a hybrid feature identification technique, toward using text summarization for essay-based feedback, discourse trees are good indicators of importance in text, the rhetorical parsing of unrestricted natural language texts, unsupervised learning of disambiguation rules for part of speech tagging, rhetorical structure theory: toward a functional theory of text organization, part-of-speech tagging and partial parsing, comlex syntax: building a computational lexicon, a __ comprehensive grammar of the english language, automatic text processing: the transformation, analysis, and retrieval of information by computer, related papers.
Showing 1 through 3 of 0 Related Papers
AI Grader App | Easily Grade Assignments
Unlock the power of AI grading with our AI Grader App – the ultimate solution for educators seeking to streamline their grading process. Effortlessly grade assignments, save valuable time, and provide instant, personalized feedback to students.
The Best AI Graders for Automated Essay Evaluation
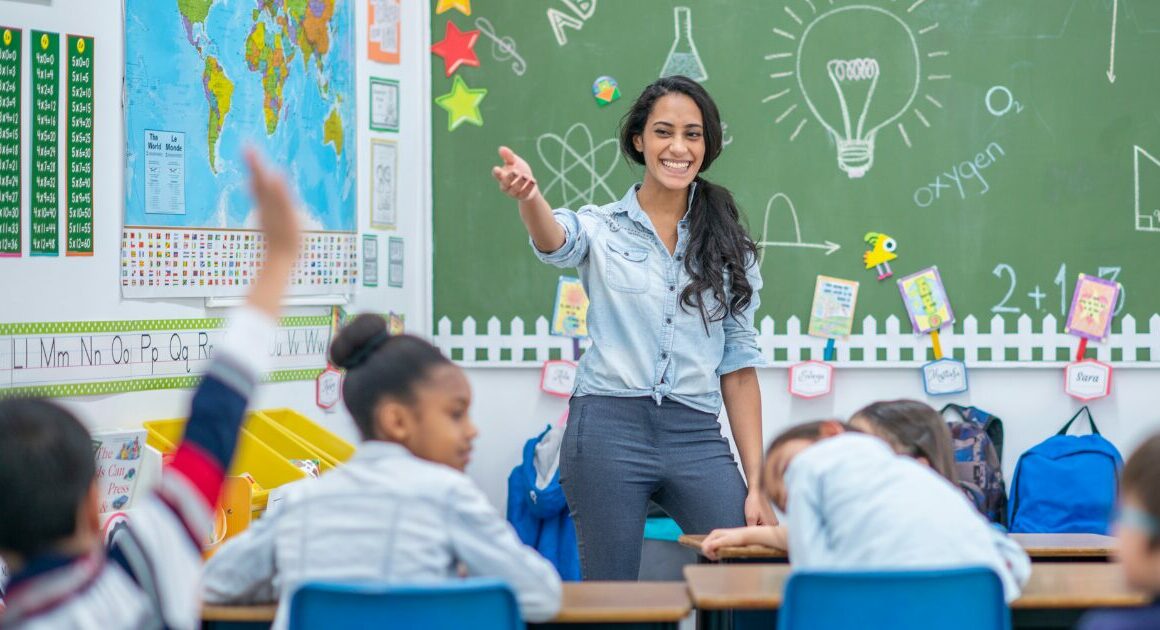
Introduction
In the dynamic landscape of education, teachers are increasingly turning to cutting-edge technologies to enhance their teaching methodologies. At the forefront of this revolution are AI graders, designed to automate and elevate the essay grading process. In this article, we will delve into the world of AI for grading, specifically focusing on the best AI graders and their role in automated essay evaluation.
Understanding AI Graders
AI graders, often referred to as automated grading systems, utilize advanced machine learning algorithms to assess and evaluate student essays. These systems bring efficiency to the grading process, providing instant feedback and insights for both teachers and students. This article explores the top contenders in the realm of AI essay grading.
Top Contenders in AI Essay Grading:
- Key Features : Can grade assignments in multiple languages, catering to a diverse student population.
- Benefits : Automates the grading process, significantly reducing the time teachers spend on marking assignments.
- Key Features: Easy-to-use AI essay grader with integrated AI tools and teaching assistant.
- Benefits: Reduces the need for multiple subscriptions with its multifunctional capabilities.
- Key Features: Recognized as one of the best AI graders, Gradescope offers a sophisticated platform for automated essay evaluation.
- Benefits: Quick and accurate grading, customizable rubrics, and insightful analytics to identify common misconceptions among students.
- Key Features: Renowned for its plagiarism detection capabilities, Turnitin extends its functionality to AI essay grading, ensuring academic integrity.
- Benefits: Enhances originality, provides in-depth feedback on writing quality, and supports automated grading for various writing formats.
- Key Features: Integrates AI to provide detailed feedback on essays, enhancing student engagement.
- Benefits: Automates feedback, reducing educator workload and fostering peer learning.
- Key Features: Uses AI for consistent, unbiased grading across various subjects on the PaperRater platform.
- Benefits: Offers immediate feedback, essential for large-scale online courses, and improves scalability.
- Key Features: Known as one of the best AI graders for ChatGPT, aiforteachers.ai offers 1000+ free AI graders and tools for teachers.
- Benefits: AI graders that are easy for teachers to use, personalized rubrics, and excellent customer support.
Key Considerations for Teachers:
As educators explore the potential of AI graders for essay evaluation, several key considerations should guide their choices:
- Accuracy: Assess the accuracy of the AI grader in evaluating diverse essay formats to ensure reliable and consistent results.
- User-Friendliness: Choose an AI grader that is user-friendly, minimizing the learning curve for both educators and students.
- Customization: Opt for platforms that allow customization of grading criteria and rubrics, aligning with specific teaching methodologies.
- Integration: Select AI grading tools that seamlessly integrate with existing Learning Management Systems, fostering efficiency in overall workflow.
In the realm of education technology, AI essay graders are emerging as essential tools for teachers seeking to streamline and enhance the essay grading process . By incorporating the best AI graders into their teaching arsenal, educators can not only save time but also provide valuable, constructive feedback to students. As the landscape of automated grading systems evolves, teachers should carefully assess the available options to find the AI grader that aligns seamlessly with their teaching style and classroom needs. Embrace the transformative power of AI for teachers and elevate the educational experience for both educators and students alike.
Subscribe to our blog to receive regular updates on the latest advancements in AI education, practical tips for integrating technology in the classroom, and in-depth discussions on the evolving role of artificial intelligence in teaching.
Subscribe for the Best AI Tips for Teachers:
Susan Schneider, AI Expert for Teachers
Related posts.
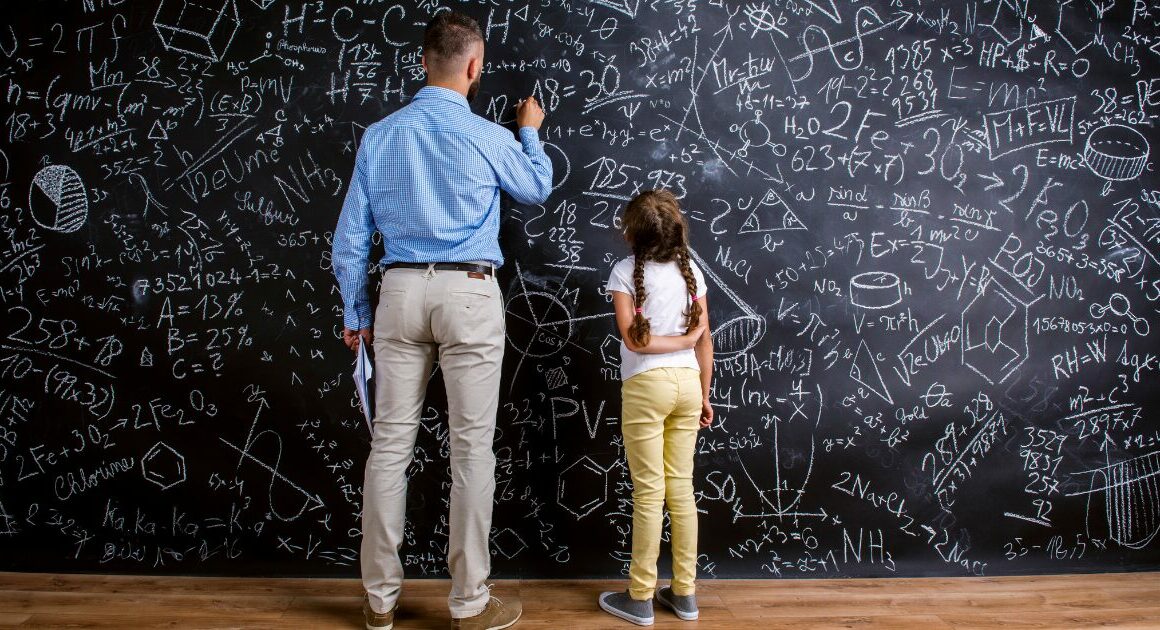
Easy Ways to Use ChatGPT to Effectively Grade Essays
Grading essays can be a time-consuming task for teachers. However, with the help of AI, specifically ChatGPT, paper grading can be streamlined.
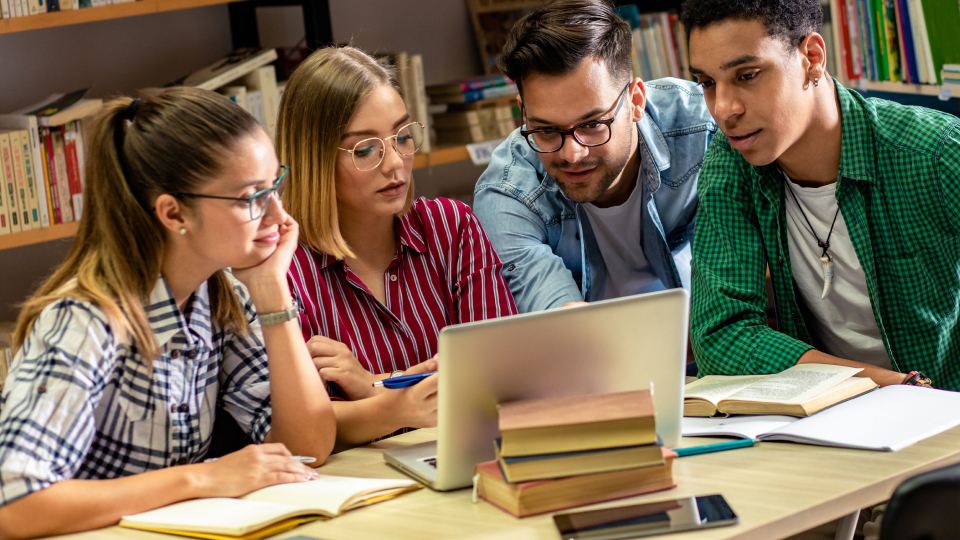
Can ChatGPT Grade Essays? An In-Depth Look
In the rapidly evolving landscape of education, AI has become a powerful ally for teachers. Among the various AI tools available, ChatGPT, particularly its latest iteration, ChatGPT-4o, has garnered significant attention for its potential to grade essays. But can ChatGPT truly take on the nuanced task of essay grading? Let’s explore this question in detail. […]
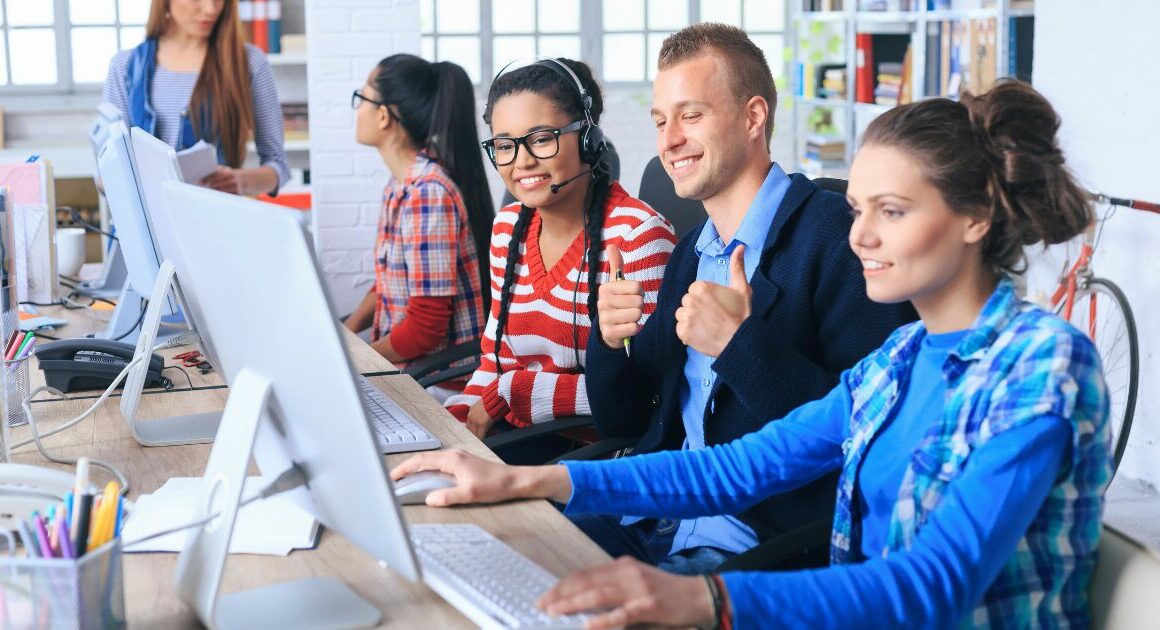
The Best Learning Center on AI Essay Graders (2024)
Kangaroos AI, a pioneer in educational technology, has launched a resourceful free online learning center dedicated to AI essay graders. This new resource is designed to empower educators by enhancing their understanding and utilization of AI-driven tools for grading essays. The Kangaroos AI Learning Center is not just a repository of information; it is a […]
Subscribe to the PwC Newsletter
Join the community, add a new evaluation result row, automated essay scoring.
26 papers with code • 1 benchmarks • 1 datasets
Essay scoring: Automated Essay Scoring is the task of assigning a score to an essay, usually in the context of assessing the language ability of a language learner. The quality of an essay is affected by the following four primary dimensions: topic relevance, organization and coherence, word usage and sentence complexity, and grammar and mechanics.
Source: A Joint Model for Multimodal Document Quality Assessment
Benchmarks Add a Result
Trend | Dataset | Best Model | -->Paper | Code | Compare |
---|---|---|---|---|---|
Tran-BERT-MS-ML-R | -->
Most implemented papers
Automated essay scoring based on two-stage learning.
Current state-of-art feature-engineered and end-to-end Automated Essay Score (AES) methods are proven to be unable to detect adversarial samples, e. g. the essays composed of permuted sentences and the prompt-irrelevant essays.
A Neural Approach to Automated Essay Scoring
nusnlp/nea • EMNLP 2016
SkipFlow: Incorporating Neural Coherence Features for End-to-End Automatic Text Scoring

Our new method proposes a new \textsc{SkipFlow} mechanism that models relationships between snapshots of the hidden representations of a long short-term memory (LSTM) network as it reads.
Neural Automated Essay Scoring and Coherence Modeling for Adversarially Crafted Input
Youmna-H/Coherence_AES • NAACL 2018
We demonstrate that current state-of-the-art approaches to Automated Essay Scoring (AES) are not well-suited to capturing adversarially crafted input of grammatical but incoherent sequences of sentences.
Co-Attention Based Neural Network for Source-Dependent Essay Scoring
This paper presents an investigation of using a co-attention based neural network for source-dependent essay scoring.
Language models and Automated Essay Scoring
In this paper, we present a new comparative study on automatic essay scoring (AES).
Evaluation Toolkit For Robustness Testing Of Automatic Essay Scoring Systems
midas-research/calling-out-bluff • 14 Jul 2020
This number is increasing further due to COVID-19 and the associated automation of education and testing.
Prompt Agnostic Essay Scorer: A Domain Generalization Approach to Cross-prompt Automated Essay Scoring
Cross-prompt automated essay scoring (AES) requires the system to use non target-prompt essays to award scores to a target-prompt essay.
Many Hands Make Light Work: Using Essay Traits to Automatically Score Essays
To find out which traits work best for different types of essays, we conduct ablation tests for each of the essay traits.
EXPATS: A Toolkit for Explainable Automated Text Scoring
octanove/expats • 7 Apr 2021
Automated text scoring (ATS) tasks, such as automated essay scoring and readability assessment, are important educational applications of natural language processing.
- AI Essay Grader
Premium Essay Grader
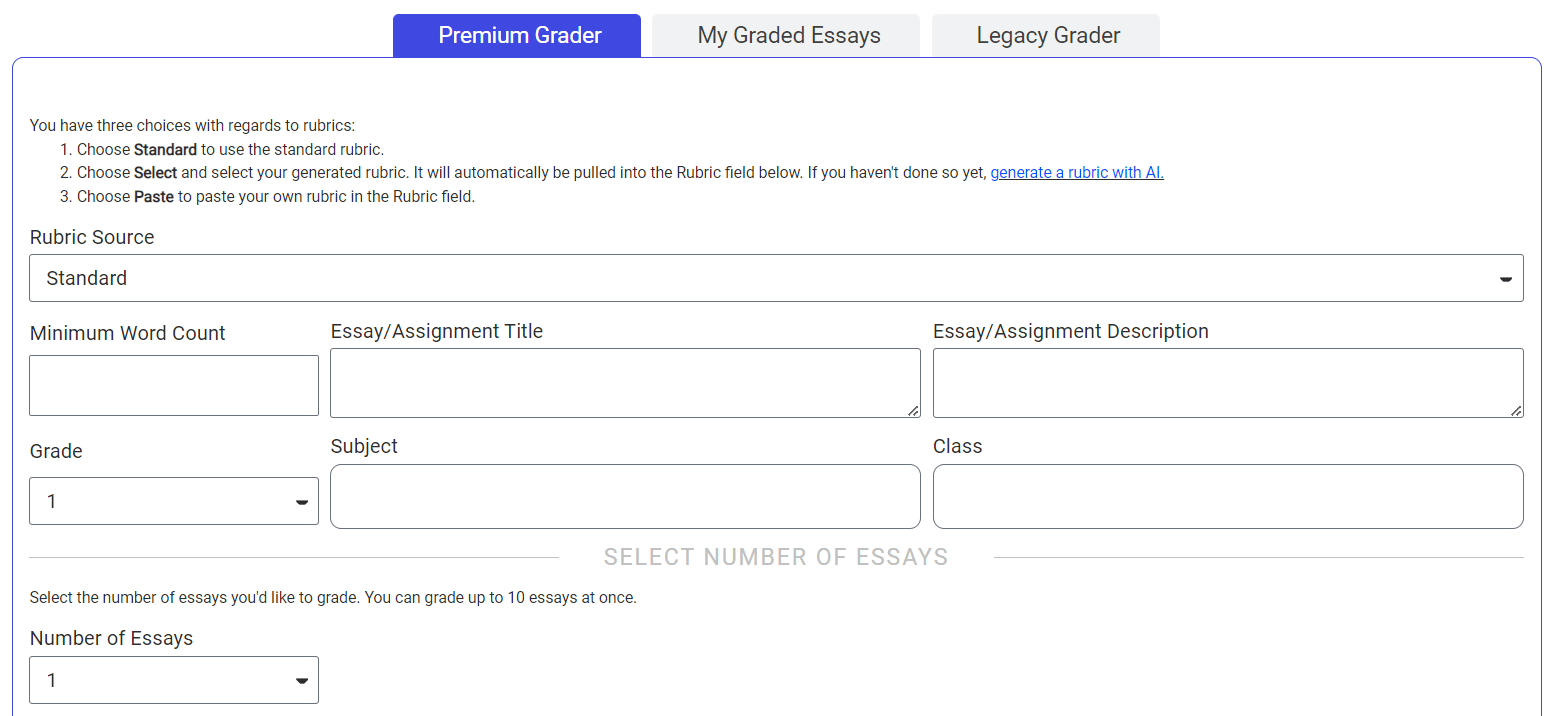
Sample AI Graded Essay
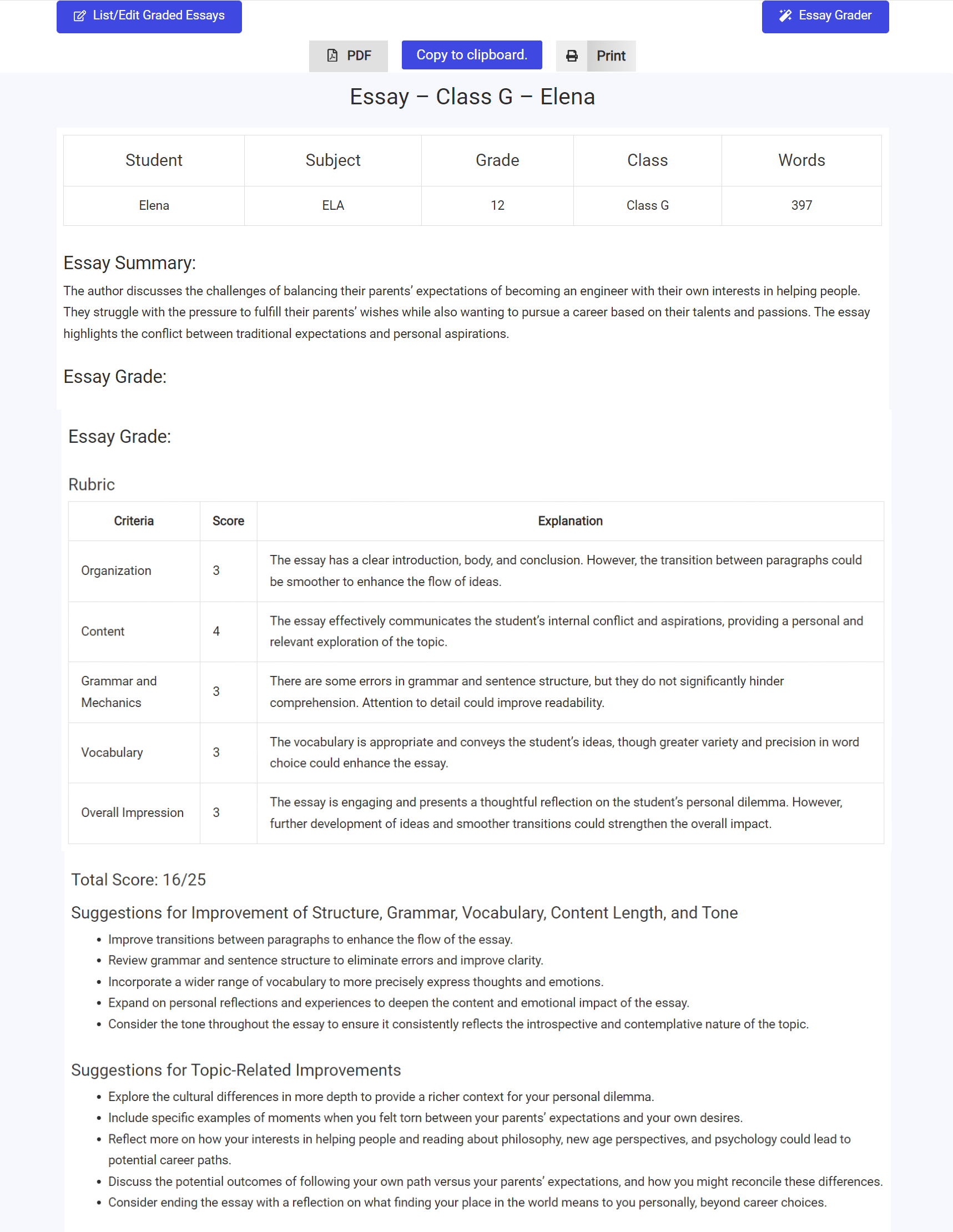
About Our AI Essay Grader
Welcome to the future of education assessment with ClassX’s AI Essay Grader! In an era defined by technological advancements, educators are constantly seeking innovative ways to streamline their tasks while maintaining the quality of education. ClassX’s AI Essay Grader is a revolutionary tool designed to significantly alleviate the burden on teachers, offering a seamless and efficient solution to evaluate students’ essays.
Traditionally, assessing essays has been a time-consuming process, requiring educators to meticulously read through each piece of writing, analyze its content, and apply complex rubrics to assign grades. With the advent of AI, however, the landscape of education evaluation is rapidly changing. ClassX’s AI Essay Grader empowers teachers by automating the grading process without compromising on accuracy or fairness.
The concept is elegantly simple: teachers input or copy the students’ essays into the provided text box, select the appropriate grade level and subject, and ClassX’s AI Essay Grader takes it from there. Leveraging the cutting-edge technology of ChatGPT, the AI system meticulously evaluates essays against a predefined rubric. The rubric encompasses various criteria, ranging from content depth and structure to grammar and style, ensuring a comprehensive assessment of the writing.
Criteria | Score 4 | Score 3 | Score 2 | Score 1 |
Organization | Writing has a clear introduction, body, and conclusion with appropriate use of paragraphs. | Writing has a clear introduction and conclusion but may have some inconsistencies in paragraphing. | Writing has some attempt at organization but lacks a clear introduction, body, or conclusion. | Writing is disorganized and lacks clear structure. |
Content | Writing includes relevant details, facts, or examples that support the main idea. | Writing includes some relevant details, facts, or examples, but may lack consistency or specificity. | Writing includes limited or unrelated details, facts, or examples. | Writing lacks relevant content or is off-topic. |
Grammar and Mechanics | Writing demonstrates correct use of punctuation, capitalization, and verb tense. | Writing has some errors in punctuation, capitalization, or verb tense, but does not significantly impact readability. | Writing has frequent errors in punctuation, capitalization, or verb tense that may impact readability. | Writing has pervasive errors in punctuation, capitalization, or verb tense that significantly impact readability. |
Vocabulary | Writing uses a variety of age-appropriate vocabulary with precise word choices. | Writing uses some age-appropriate vocabulary but may lack variety or precision. | Writing uses limited or basic vocabulary that may not be age-appropriate. | Writing lacks appropriate vocabulary or word choices. |
Overall Impression | Writing is engaging, well-crafted, and demonstrates strong effort and creativity. | Writing is generally engaging and shows effort, but may have some areas for improvement. | Writing is somewhat engaging but lacks polish or effort. | Writing is not engaging, poorly crafted, or lacks effort. |
Teachers can now allocate more time to personalized instruction, classroom engagement, and curriculum development, rather than being bogged down by the time-consuming task of manual essay evaluation. The AI’s rapid and consistent grading also means that students receive prompt feedback on their work, enabling them to learn from their mistakes and improve their writing skills at an accelerated pace.
Moreover, the AI Essay Grader enhances objectivity in grading. By removing potential biases and inconsistencies inherent in manual grading, educators can ensure that every student receives a fair and unbiased evaluation of their work. This contributes to a more equitable educational environment where all students have an equal chance to succeed.
In summary, ClassX’s AI Essay Grader represents a groundbreaking leap in the evolution of educational assessment. By seamlessly integrating advanced AI technology with the art of teaching, this tool unburdens educators from the arduous task of essay grading, while maintaining the highest standards of accuracy and fairness. As we embrace the potential of AI in education, ClassX is leading the way in revolutionizing the classroom experience for both teachers and students.
Share this tool
- Categories: Assessments and Grading , AI Tools for Teachers , AI Tools
- Keywords: essay , essay grade , grade essay , grading
Privacy Overview
Cookie | Duration | Description |
---|---|---|
cookielawinfo-checkbox-analytics | 11 months | This cookie is set by GDPR Cookie Consent plugin. The cookie is used to store the user consent for the cookies in the category "Analytics". |
cookielawinfo-checkbox-functional | 11 months | The cookie is set by GDPR cookie consent to record the user consent for the cookies in the category "Functional". |
cookielawinfo-checkbox-necessary | 11 months | This cookie is set by GDPR Cookie Consent plugin. The cookies is used to store the user consent for the cookies in the category "Necessary". |
cookielawinfo-checkbox-others | 11 months | This cookie is set by GDPR Cookie Consent plugin. The cookie is used to store the user consent for the cookies in the category "Other. |
cookielawinfo-checkbox-performance | 11 months | This cookie is set by GDPR Cookie Consent plugin. The cookie is used to store the user consent for the cookies in the category "Performance". |
viewed_cookie_policy | 11 months | The cookie is set by the GDPR Cookie Consent plugin and is used to store whether or not user has consented to the use of cookies. It does not store any personal data. |
- Learn the Alphabet
- Video Lessons
AI CONVERSATIONS
- AI Homework Helper
- Interview a Historical Figure
- AI Conversation Practice
- AI Book Chat
- AI Country Guide
AI TOOLS FOR STUDENTS
- AI Dictionary
- AI Thesaurus
- AI Sentence Generator
- AI Grammar Correction
- AI Paraphraser
- AI Summarizer
- AI Lyrics Generator
- AI Poem Generator
- AI Ancient Text Translator
- AI Children's Story Generator
- Role-Play Game: Fantasy Quest
- AI Figure of Speech Generators
AI TOOLS FOR TEACHERS
- AI Rubric Generator
- AI Prompt Optimizer
- AI Lesson Creator
- AI Lesson Plan Creator
- AI Multiple-Choice Quiz Creator
- AI True-False Quiz Creator
- AI Fill-in-the-Blanks Quiz Creator
- AI Book Quiz Creator
- AI Report Card Comments
- AI Comments for English Teachers
- IEP Generator
- Children's Music
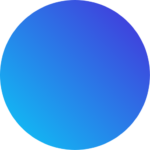
Suggestions
Suggest an ai tool or improvements to an existing one..
The ultimate AI assistant for grading assignments.
Grade assignments 10x faster and provide personalized writing feedback. GradeWrite streamlines grading process with bulk-grading, auto-checkers, AI re-grades, custom rubrics, and much more.
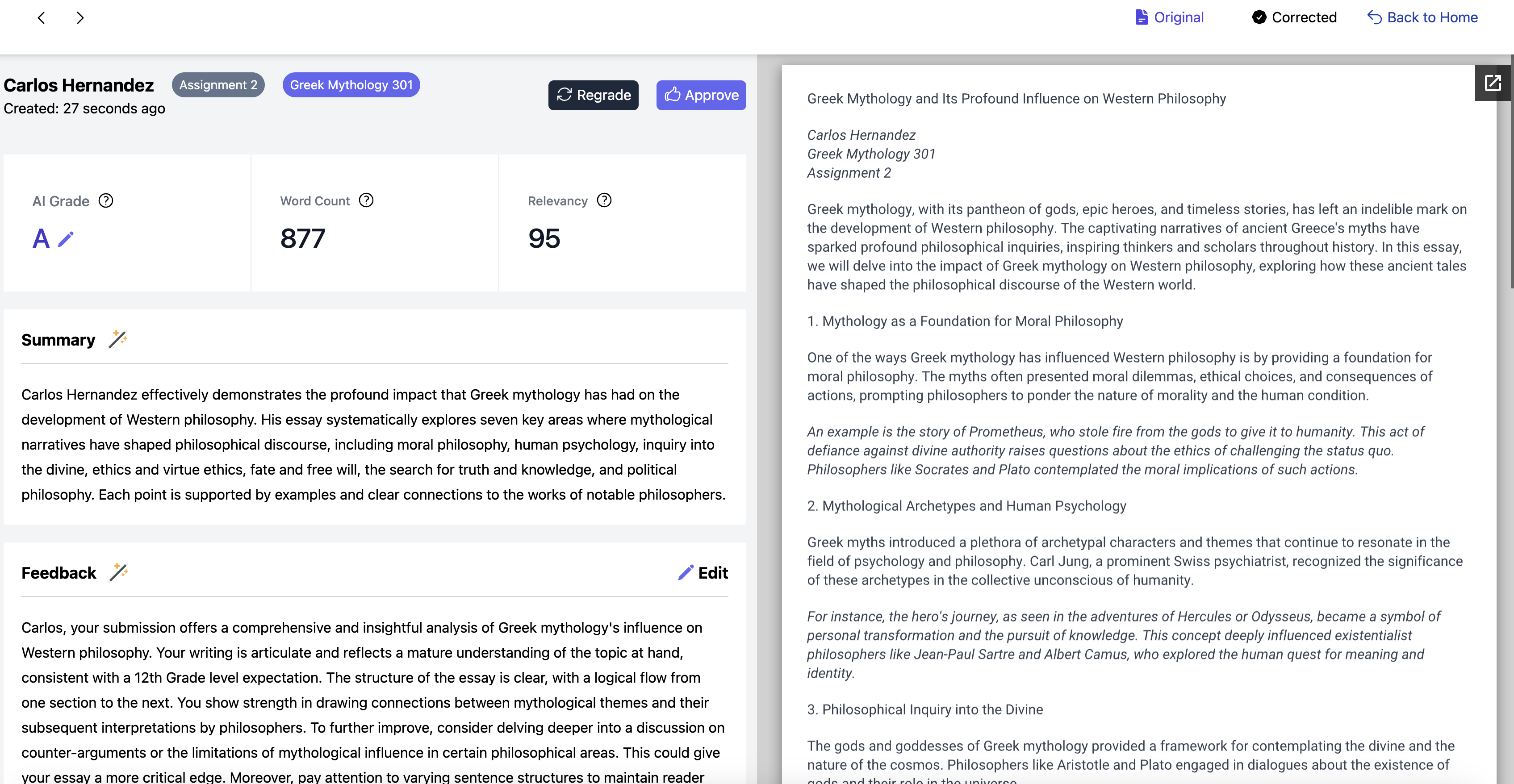
How It Works
Seamless, Interactive Grading with AI
Leverage the AI grading co-pilot to streamline your grading process with AI-powered precision and ease that helps you grade 10x faster.
Features and Benefits
Bulk uploads and 5000+ word limit, grade 10x faster with streamlined grading.
GradeWrite AI's bulk upload feature allows you to upload multiple files at once, saving you time and effort. Our system also supports files up to 3000 words, allowing you to grade longer assignments with ease. Additionally, our side-by-side grading feature allows you to view the original submission and the AI-generated feedback simultaneously, ensuring a seamless grading experience.
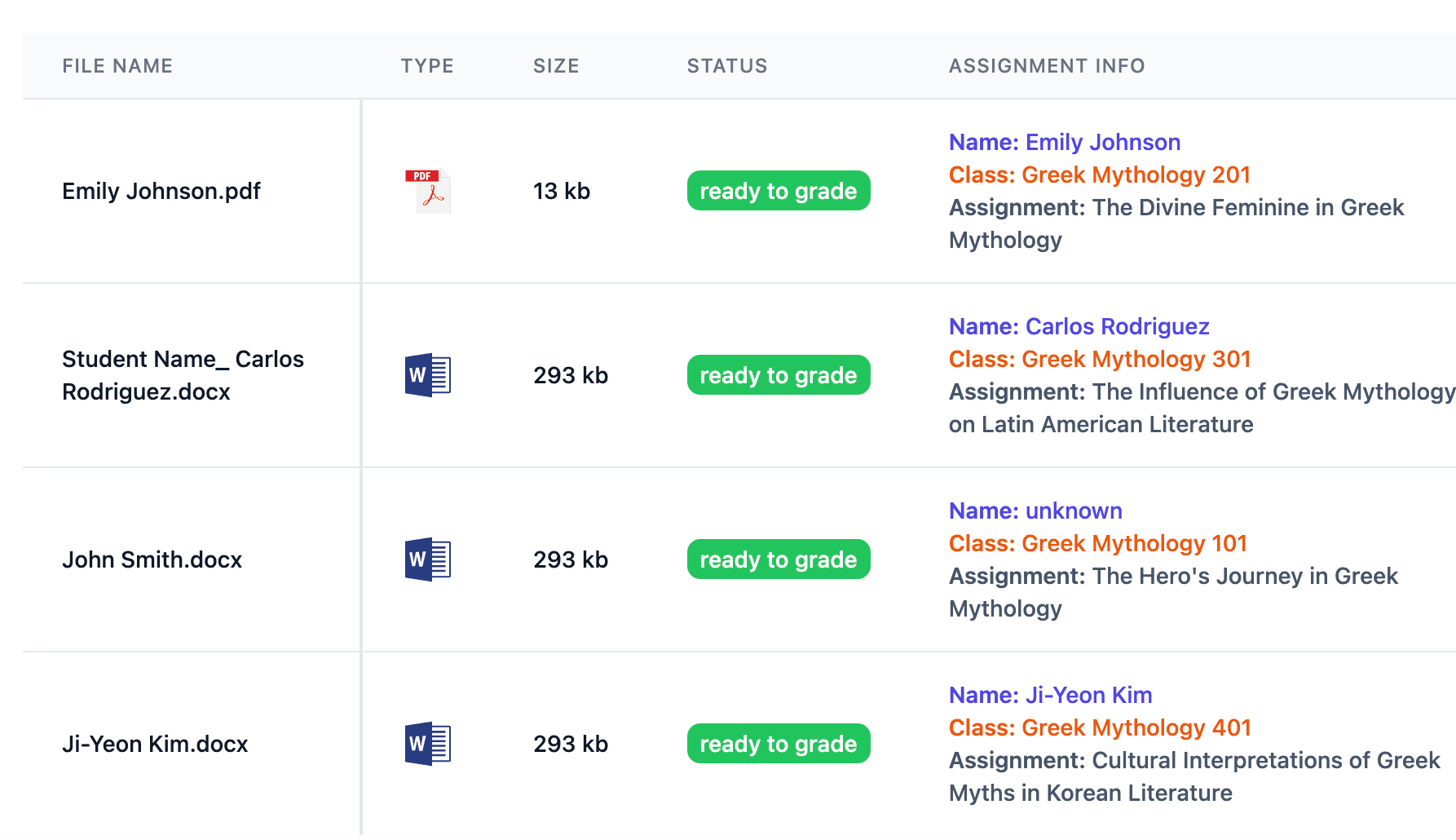
AI enhanced, custom writing feedback
Iterate feedback with ai-generated suggestions.
Use GradeWrite.AI’s AI-generated feedback to quickly and easily generate detailed, constructive feedback. You can easily iterate on this feedback by editing it, or refreshing AI feedback. This feature not only saves you time, but also helps students improve their writing skills. Our AI-generated feedback is designed to enhance your feedback, not replace it.
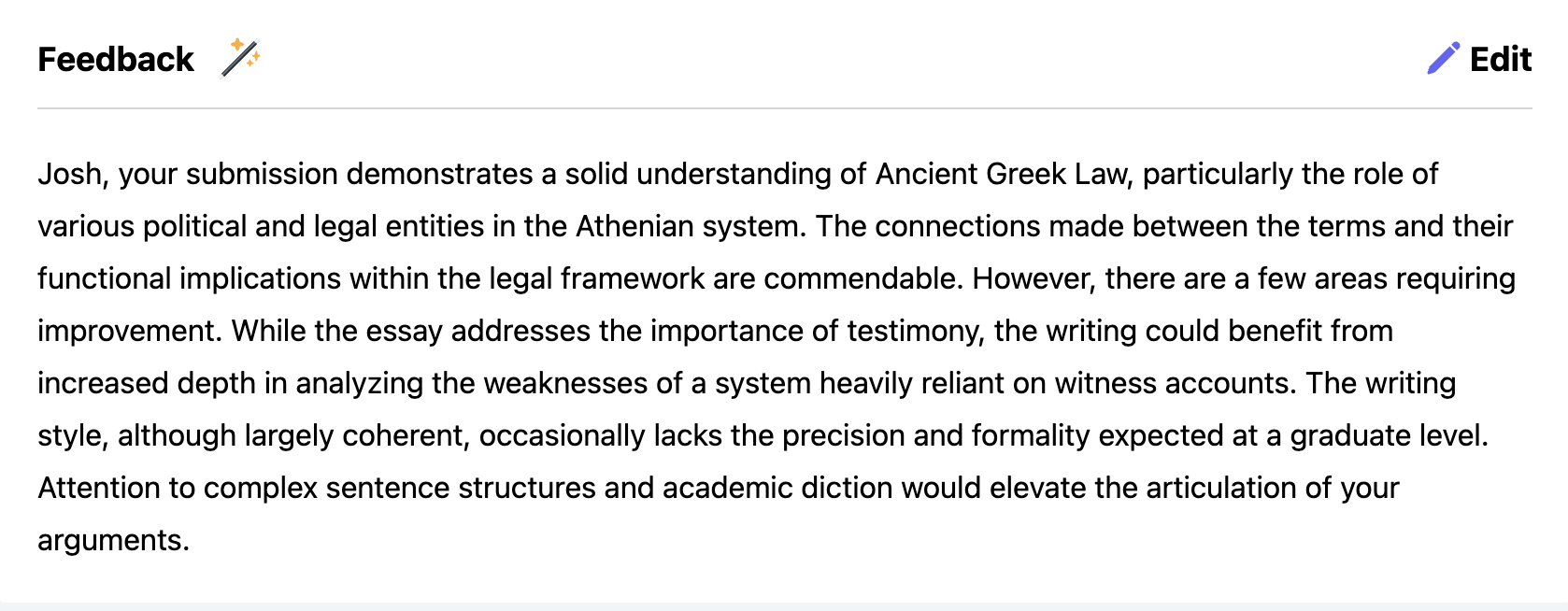
Side by side view of original submission and AI-generated feedback
Easily see original submission and ai-generated feedback.
GradeWrite.AI’s side-by-side view allows you to easily compare the original submission and the AI-generated feedback. This feature ensures that you don’t miss any important details, while saving you time and effort.
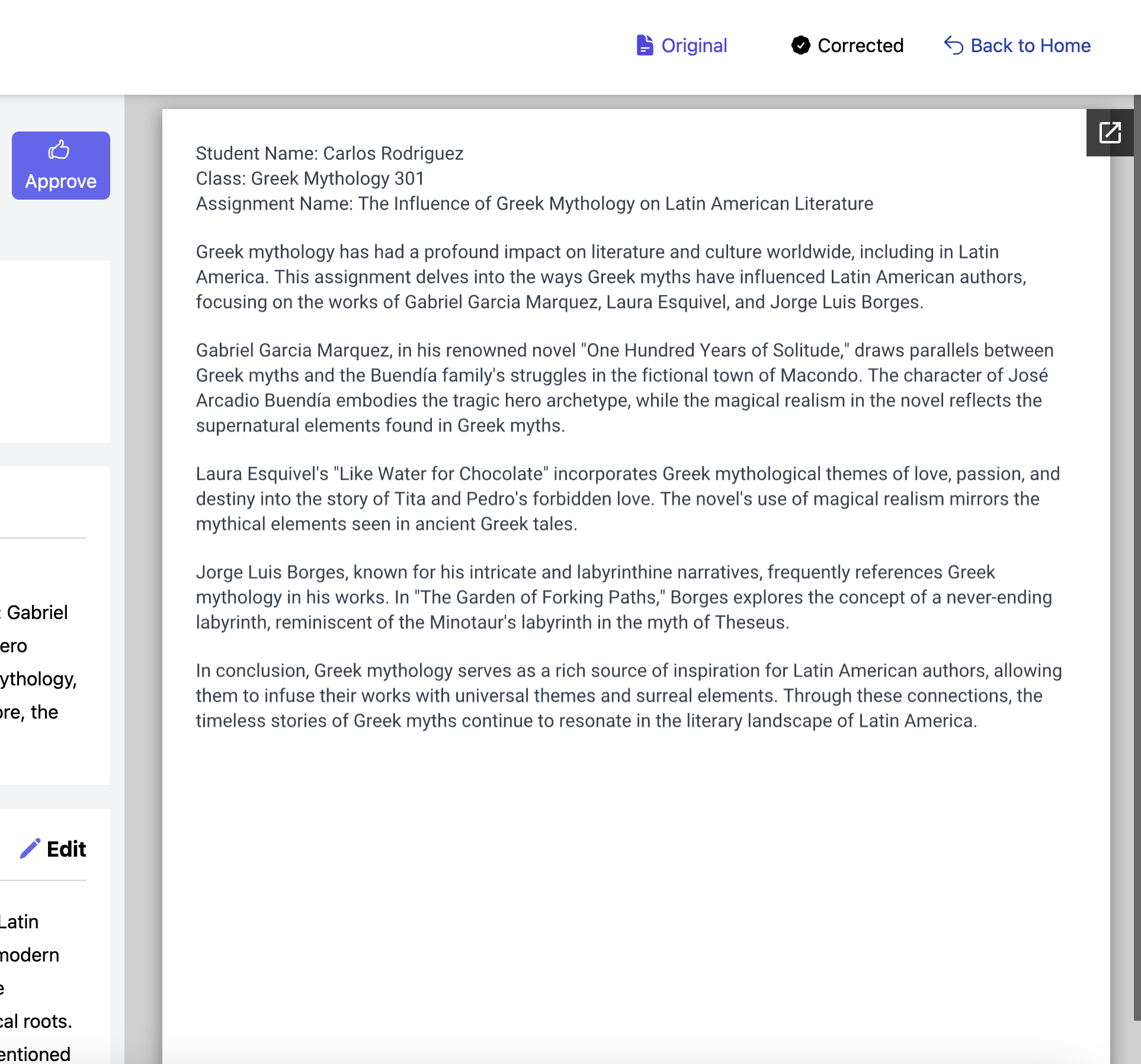
Auto-checkers for word count, AI detection, and rubric adherence
Flag issues early with auto-checkers.
GradeWrite.AI’s auto-checkers ensure that every submission meets your standards. Our system checks for word count, AI detection, and rubric adherence, flagging any issues early in the grading process. This allows you to focus on the most important aspects of grading, while ensuring that every submission meets your standards.

Auto-summaries of assignments for quick review
Get a quick overview of assignments.
GradeWrite.AI’s auto-summaries provide a quick overview of each assignment, allowing you to quickly review submissions. This feature saves you time and effort, while ensuring that you don’t miss any important details.
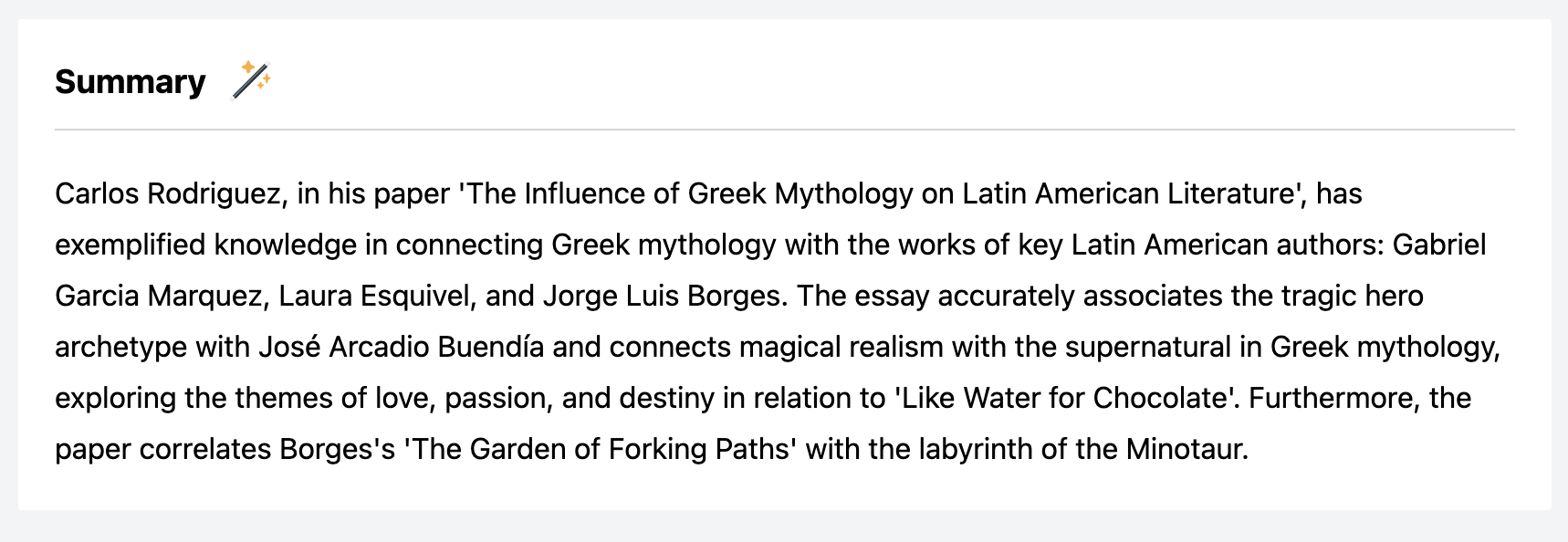
Automated grammar and spelling checks (beta)
Allow students to submit polished work.
Say goodbye to manual proofreading with our automated grammar and spelling check feature. This tool ensures every submission is polished and error-free, saving time and enhancing the quality of student work.
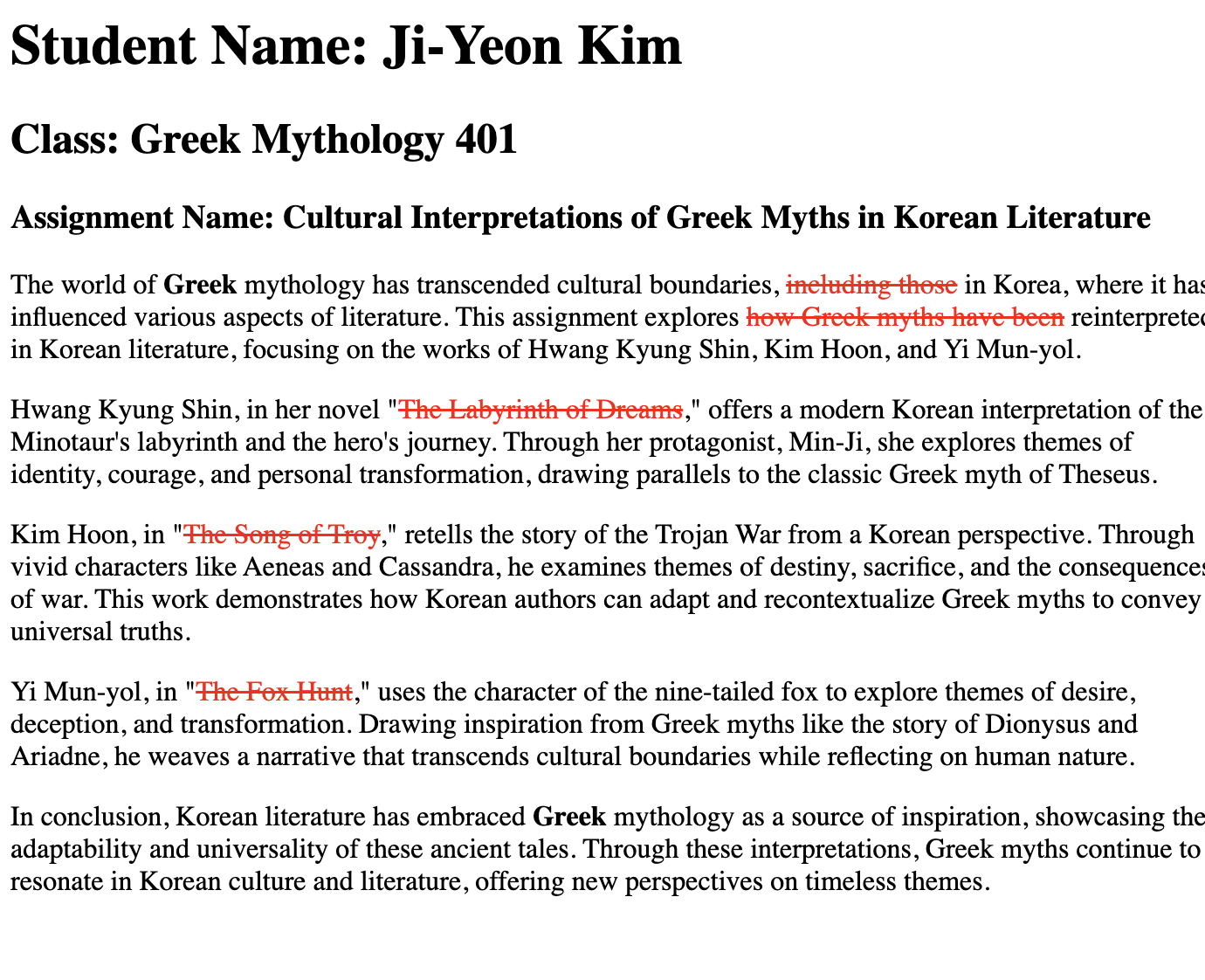
Custom rubrics to teach AI how to grade
Flexible custom rubrics.
Create custom rubrics that align with your specific grading criteria. This feature allows you to tailor the AI’s grading process to match your unique teaching style, ensuring consistent and personalized assessments.
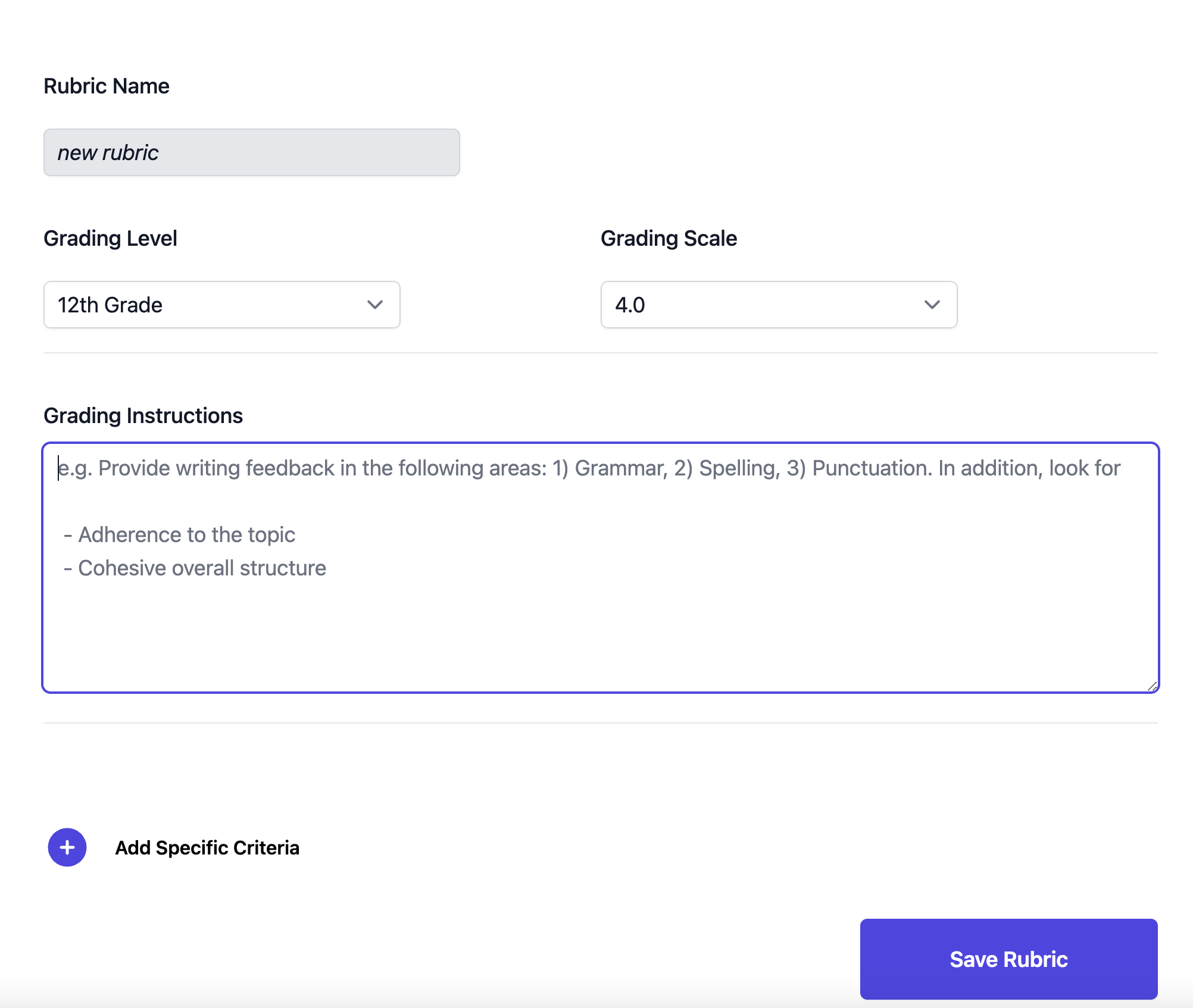
Streamline your grading process, today
Grade assignments 10x faster and provide personalized writing feedback with AI. GradeWrite streamlines grading process with bulk-grading, auto-checkers, AI re-grades, custom rubrics, and much more.
Frequently asked questions
- Newsletters
Automated Grade Control’s Steady Plow Toward Full Autonomy
The same construction equipment manufacturers who brought you automated grade control precision and efficiency anticipate full autonomy as the next leap forward.
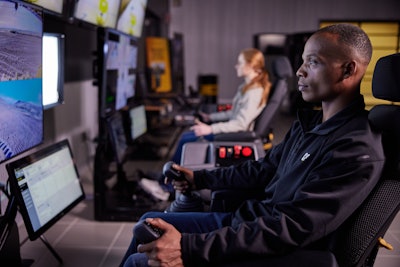
It’s a bit of a stretch to realize zero human interaction in the operation of heavy construction equipment. However, make no mistake. The move is on to make it happen.
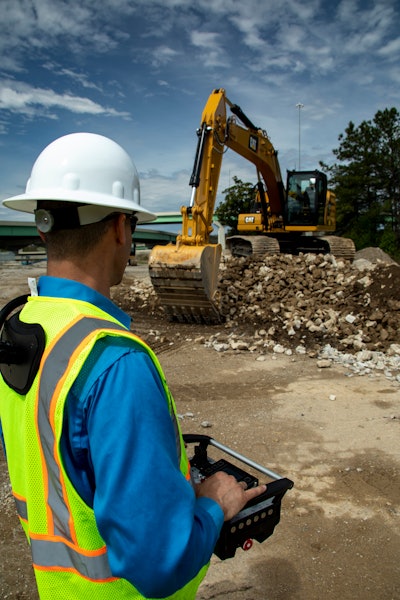
“Part of the problem involves the environmental context,” said Scott Hagemann, senior market professional for Caterpillar. “Autonomous trucks work in a mine, where the location is isolated; there’s no traffic or people in harm’s way during the operation. But with grade control, you’re usually operating in a densely populated area, where there are too many factors to trust safe operations to pure robotics.”
So, for now, automated grade control is the best way to improve precision, safety and efficiency on a construction site. That is...for now.
On the Road to Full Autonomy
The Society of Automotive Engineers identified six stages of automation, starting at Stage zero and progressing to Stage 5, the yet-to-be-realized holy grail of vehicular automation. These stages progress as follows:
- Stage 0 – No automation
- Stage 1 – Singular automated driver assist (for example, cruise control)
- Stage 2 – Dual automated driver assist (for example, cruise plus braking control)
- Stage 3 – Human monitoring and interdiction of autonomous operation
- Stage 4 – Full autonomy within set boundaries (for example, driverless taxis inside geofenced areas)
- Stage 5 – Fully self-driving vehicles without drivers or boundaries
Where haul trucks might operate at a Stage 4 level of autonomy in a mining environment, Stage 3 bulldozers and excavators are equipped to support automated grade control on construction sites. Common operator assists might include blade and bucket controls, monitored by an in-cab operator who reads grading progress on an output display and can override or refine the operation using onboard commands.
Remote control is also possible when performing grading operations under Stage 3 conditions. In this instance, the operator is not on board the vehicle but operating it from a separate location using a joystick or belly box.
“Technically, you could perform the operation from your living room, oceans away,” said Cameron Clark, earthmoving industry director for Trimble. “The idea would be to ensure safety in a diverse work environment, such as along a steep dam or embankment or provide convenience where travel or labor shortages might inhibit job progress.”
Advancements in Grade Control
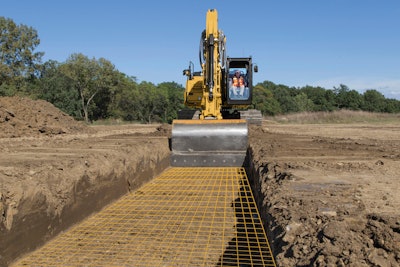
“The last mile in the journey to fully autonomous will come when we can consistently and accurately read and react to dynamic elements in the immediate environment around a fully equipped machine in motion,” Hagemann said. “When we can fully know the position of everything on the machine, not just the blade but every corner, elevation, from the top of the machine to the bottom of the tread, we’ll at last be into Stage 5 autonomy.”
In the meantime, automated grade control is continuing to advance. The ability to make data-driven design decisions and push a button to automate them has been enhanced by ever-more sophisticated software used on earthworks systems. As geo-sensors send data from the extremities of the machine to the operating system, onboard software steers and controls the blade to execute the grade accordingly.
“Data fusion is the enabler behind any automated grading system,” said Clark, who helps product managers and developers push the envelope toward improving grade control. “As more intelligence gets added to grade control systems, we get closer to autonomous grade control and the goal of getting the operator out of the cab.”
Get into the Game
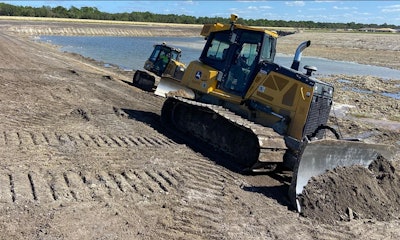
Operators can use control pads on smart phones to connect to a system wirelessly and update plan changes or troubleshooting information. Simple 2D technology that integrates onboard inertial measurement sensors with an in-cab monitor can produce highly accurate single or dual angle slopes efficiently, potentially paying off a technology investment on a single large project.
“Starting small, with a 2D system and basic laser technology, is usually the easiest way to see results and get familiar with the technology,” said Bob Flynn, construction precision sales manager for CASE CE. “For more experienced users, I’d recommend investing in off-board solutions that help with data management. These are typically under-utilized but can offer valuable insights into jobsite productivity.”
The key is to make sure the system matches the application and not to overspend. Most solutions are upgradable and evolve over time, so the best advice would be to visit a dealer, discuss your situation, and demo a few products.
“Just as you wouldn’t use a 20-pound sledgehammer to nail a picture into a wall, you never want to purchase technology simply for technology’s sake.” said Hagemann. “Go in with a few use cases and solve those first. The rest will fall into place.”
Noteworthy Concerns
Until automated grade control makes the leap to autonomous, contractors would do well to pay attention to a few basic best practices when using automated technology. Training may be at the top of the list.
Most manufacturers offer excellent training materials and support to get new operators up to speed quickly on the technology. Some even provide remote or onboard training by way of downloadable videos for instant playback in the cab. An operator can simply punch up a help topic and watch a how-to instructional video to correct on-the-job issues without contacting an expert or leaving the site. This is critical for the safe use of automated systems and for accelerating the contractor’s return on investment (ROI).
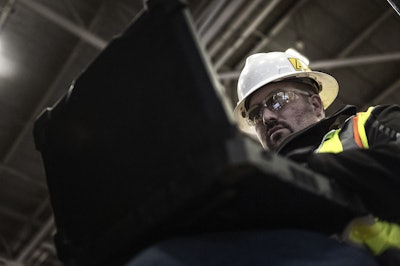
Also, be wary of how reliable your technology really is. Ask around and do your homework before you buy.
“It can be easy to fall in love with technology just because it’s technology,” said Trimble’s Clark. “If a cable breaks or a sensor gets damaged on site, suddenly your machine can’t be used. So, from entry level to premier offerings, work with reputable dealers and make sure to keep quality and reliability high on your list of solution priorities.”
Enjoy the Benefits Along the Way
While the journey to full autonomy may not be complete yet, the benefits provided by positioning yourself for the breakthrough are many. Within the confines of automated grade control, consider these benefits:
- The safety afforded by not having to position surveyors on the site
- Reduced rework, material usage, fuel cost, environmental impact, and need for skilled labor
- Increased job completion
- Enhanced productivity
- Competitive stance that accompanies lower costs and improved margins
You don’t need full autonomy to enjoy these benefits. By adopting existing technology as it moves toward the holy grail, you set yourself up as a front runner in the quest. When full autonomy at last becomes available, you will have a jump on the competition. Your people will already be familiar with autonomous technology by virtue of their understanding of automated grade control, and they will be eager to claim the benefits that come with it.
Looking Ahead
In the march toward Stage 5 autonomy, remember, everyone is still learning. Haul trucks, compactors and other heavy equipment in mining and farming merely point the way to greater economies in construction and related industries. Where mundane and repetitive tasks are replaced by machines today, finesse work, such as grading, will likewise be managed using advanced technology.
“The ability to coordinate whole fleets from a remote site is coming soon,” said Flynn. “No one wants to work onsite in 100°F weather.”
Clark reports that Trimble is already adding features and functions that will minimize operator involvement in the grade control process to a point where the operator may be the only safety mechanism onboard.
“Once we figure out how to get the operator out of the machine altogether, it will be game over,” he said. “Jobsite safety and productivity will make a quantum leap, making construction in even the most hazardous locations practical.”
Trials are underway at research and development labs across the globe, in pilot projects designed to take semi-autonomous, human-directed operations to full autonomy with artificial intelligence and machine learning. As these pilots prove out, new options will become available, leading to cost reductions on the very technology designed to lower overall job costs for improve safety and profitability.
For early movers, renting autonomous equipment may be the economical way to sample its effectiveness without incurring steep, upfront costs. As well, retrofit kits for many existing machines will become available that preserve existing investments, allowing full autonomous operation without replacing manual operations altogether.
Keep Your Eyes on the Prize
While autonomy looms on the horizon today, automation is available, here and now. Automated grade control can produce huge returns on investment by way of improved grading accuracy, lower cost and enhanced productivity and safety.
“Autonomous control is already making inroads in unsafe conditions where a task is reliably repeatable,” said Hagemann. “Keep your eyes on the ‘secret squirrel’ stuff that’s happening now behind the curtains. You’ll be seeing more of it online, in showrooms and at tradeshows very soon.”
How 2D Technology Assists New Operators
Precision grade technology systems allow operators of all skill levels to complete their jobs faster and more accurately. Sensors on the boom, arm, bucket, and frame enable operators to view the bucket position relative to the target grade on their in-cab monitor as they work. 2D machine guidance allows an operator to know the location of their bucket tip in context with their target grade. It involves using slope sensors and lasers to provide the operator with height and/or slope references.
The Differences Between Machine Guidance & Machine Control
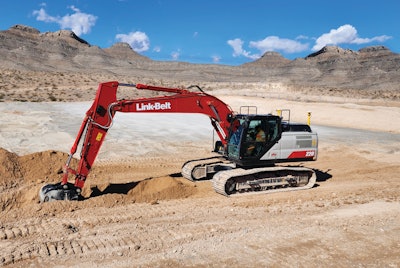
2D machine control systems add semiautonomous operation to the process, with the operator only controlling the arm when desired. The system controls the boom and bucket movements. The operator remains in complete control and can override the semiautomatic function at times. 2D machine control is ideal for applications that require the last pass to perfectly match the desired target grade, whether it’s a flat or sloping surface.
The Benefits of 2D Machine Guidance
Imagine having an extra set of eyes on your next excavation project. Precision 2D machine guidance offers this innovative system, which takes the guesswork out of trenching, site prep and mass excavation by providing real-time guidance for bucket depth and slope.
Tell the system your target depth and desired incline, and it will do the rest. Using a combination of advanced sensors and a user-friendly in-cab display, 2D machine guidance acts like your on-the-job assistant. Visual cues and clear audio signals inform you how much to adjust your bucket to achieve the perfect grade.
The Benefits of 2D Machine Control
Operators using these systems experience faster completion times thanks to fewer passes needed to achieve the desired grade. Moving the right amount of material from the start translates to better fuel efficiency and cost savings. Less time is wasted on setting up grade stakes, checking progress and fixing mistakes with rework.
Machine control takes this further, acting like an extra set of hands or even an autopilot for your machine. It uses the same sensors and data as grade control but goes beyond just giving instructions. Connecting to the machine's controls can automate specific movements based on the design. Imagine your excavator bucket automatically adjusting to hit the perfect depth every time. You're still in control of the overall operation, but the machine manages the fine-tuning, making your work faster, more accurate and less physically demanding.
– Contributed by Link-Belt Excavators
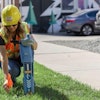
How RTK Creates Accurate Positioning Estimates to Protect Underground Utility Lines
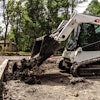
Experts Weigh in on Rental Industry Compact Equipment Advancements
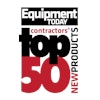
Equipment Today Recognizes the 2024 Contractors’ Top 50 New Products
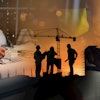
Why Gen Z Should Work Construction – From a Gen Zer
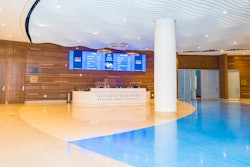
BCMason Group Founder & President Focuses on Community Projects
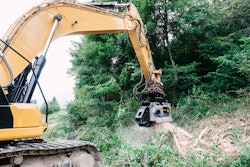
Today's Construction Heavy Equipment Attachments Bring Big Power, Versatility
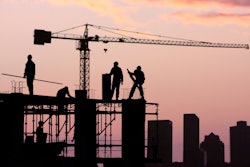
How to Build a Construction Execution Team
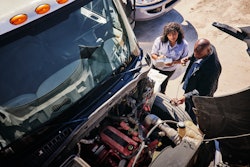
The Move to Synthetics & Synthetic Blends
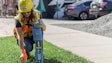
SL Laser Offers Laser Projection Systems for Concrete Projects
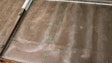
New Holland Releases Precision Technology Products and Updates
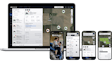
5 Lessons to Follow for Efficiency During Changing Demand
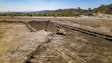
Back to the Basics: How 2D, Laser Systems Get Overlooked on Jobsites
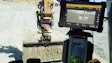
Cattron Unveils Tyro 2S Remote Control Systems
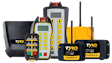
Can Machine Control Systems Really Improve Profits?
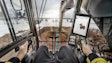
Digital Tools Transform Access Management
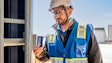
4 Construction Tech Pros Share Insights on the Connected Jobsite
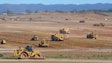

IMAGES
VIDEO
COMMENTS
Automated essay scoring (AES) is a compelling topic in Learning Analytics for the primary reason that recent advances in AI find it as a good testbed to explore artificial supplementation of human creativity. ... One of the key anticipated benefits of AES is the elimination of human bias such as rater fatigue, rater's expertise, severity ...
The tool is versatile and can be used in various learning contexts. 6. IntelliMetric. IntelliMetric is an AI-based essay-grading tool that can evaluate written prompts irrespective of the writing degree. It drastically reduces the time needed to evaluate writing without compromising accuracy.
Learn how AI can revolutionize assessment by automating grading and feedback processes, enhancing efficiency, objectivity, and personalized learning. Explore the benefits, challenges, and future trends of AI-based assessment platforms and tools.
A blog by Manjinder Kainth, PhD. CEO/CO-founder Graid In today's digital age, Artificial Intelligence (AI) is revolutionising various aspects of our lives, and education is no exception. With the rise of AI technologies, essay and report grading have undergone a significant transformation, making the process more efficient and accurate than ever before.
e-rater is a service that uses artificial intelligence and natural language processing to score and provide feedback on student essays. It is used in various applications, such as Criterion, TOEFL and GRE, and has been shown to demonstrate reliability and measurement benefits.
In this paper, we investigate the potential of using an artificial intelligence (AI) language model, specifically the GPT-3 text-davinci-003 model, for automated essay scoring (AES), particularly in terms of its accuracy and reliability. AES involves the use of computer algorithms to evaluate and provide feedback on student essays.
The foundational concept of automated scoring is that good writing can be predicted. PEG and other systems require training essays that have human scores, and these systems use such essays to create scoring (or prediction) models. The models typically include 30-40 features, or variables, within a set of essays that predict human ratings.
This paper surveys the Artificial Intelligence and Machine Learning techniques used to evaluate automatic essay scoring and analyzes the limitations of the current studies and research trends. It covers the history, features, challenges, and applications of AES systems in online education.
Benefits. Automated Grading for Handwritten Essays. Leverage advanced AI algorithms to accurately grade handwritten English essays, eliminating the tedious manual grading process. Consistent & Objective Feedback. Ensure every student receives unbiased and consistent feedback, thanks to our AI-driven grading system. ...
It is intended to provide a comprehensive overview of the evolution and state-of-the-art of automated essay scoring and evaluation technology across several disciplines, including education, testing and measurement, cognitive science, computer science, and computational linguistics. The development of this technology has led to many questions ...
This article investigates the feasibility of using automated scoring methods to evaluate the quality of student-written essays. In 2012, Kaggle hosted an Automated Student Assessment Prize contest to find effective solutions to automated testing and grading. This article: a) analyzes the datasets from the contest - which contained hand-graded essays - to measure their suitability for ...
coring of EssaysSemire DikliIntroductionAutomated Essay Scoring (AES) is defined as the computer technology that evaluates and scores the written prose (Shermis & Barrera, 2002; Shermis & Burstei. , 2003; Shermis, Raymat, & Barrera, 2003). AES sys-tems are developed to assist teachers in low-stakes classroom assessment as well as testing ...
Automated essay scoring (AES) is a computer-based assessment system that automatically scores or grades the student responses by considering appropriate features. The AES research started in 1966 with the Project Essay Grader (PEG) by Ajay et al. . PEG evaluates the writing characteristics such as grammar, diction, construction, etc., to grade ...
Automated essay scoring (AES) and automated writing evaluation (AWE) systems have potential benefits. AES refers to the use of computer programs to assign a score to a piece of writing; AWE systems provide feedback on one or more aspects of writing and may or may not also provide scores.
Its automated grading systems can handle multiple-choice questions and other tasks in the blink of an eye. ... 8 Benefits of Online Gradebooks. 1. Enhanced Accessibility and Convenience ... Imagine cutting down essay grading time from 10 minutes to just 30 seconds.
As a result, automated essay scoring systems generate a single score or detailed evaluation of predefined assessment features. This chapter describes the evolution and features of automated scoring systems, discusses their limitations, and concludes with future directions for research and practice.
Learn how AI-powered automated grading systems can improve exam evaluation by reducing time, error, and bias. Explore the benefits, challenges, and role of teachers in an AI-driven grading environment.
Benefits of Modularity in an Automated Essay Scoring System. How additional features from rhetorical parse trees were integrated into e-rater and how the salience of automatically generated discourse-based essay summaries was evaluated for use as instructional feedback through the re-use of e- rater's topical analysis module are discussed.
Smodin AI Grader: Key Features: Can grade assignments in multiple languages, catering to a diverse student population. Benefits: Automates the grading process, significantly reducing the time teachers spend on marking assignments. Kangaroos AI: Key Features: Easy-to-use AI essay grader with integrated AI tools and teaching assistant.
Find 26 papers, 1 benchmarks and 1 datasets on Automated Essay Scoring, the task of assigning a score to an essay based on four dimensions: topic relevance, organization, word usage and grammar. Compare different methods, models and tools for AES and explore the latest research and trends.
In automated essay scoring (AES), essays are automatically graded without human raters. Many AES models based on various manually designed features or various architectures of deep neural networks ...
ClassX's AI Essay Grader is a tool that uses ChatGPT to grade essays automatically against a predefined rubric. Teachers can input or copy students' essays, select the grade level and subject, and get instant feedback on writing, content, grammar, vocabulary, and overall impression.
GradeWrite.AI is a platform that uses AI to grade assignments 10x faster and provide personalized writing feedback. It offers features such as bulk uploads, auto-checkers, interactive feedback, custom rubrics, and more.
"Data fusion is the enabler behind any automated grading system," said Clark, who helps product managers and developers push the envelope toward improving grade control.