

McKinsey technology trends outlook 2024
Despite challenging overall market conditions in 2023, continuing investments in frontier technologies promise substantial future growth in enterprise adoption. Generative AI (gen AI) has been a standout trend since 2022, with the extraordinary uptick in interest and investment in this technology unlocking innovative possibilities across interconnected trends such as robotics and immersive reality. While the macroeconomic environment with elevated interest rates has affected equity capital investment and hiring, underlying indicators—including optimism, innovation, and longer-term talent needs—reflect a positive long-term trajectory in the 15 technology trends we analyzed.
What’s new in this year’s analysis
This year, we reflected the shifts in the technology landscape with two changes on the list of trends: digital trust and cybersecurity (integrating what we had previously described as Web3 and trust architectures) and the future of robotics. Robotics technologies’ synergy with AI is paving the way for groundbreaking innovations and operational shifts across the economic and workforce landscapes. We also deployed a survey to measure adoption levels across trends.
These are among the findings in the latest McKinsey Technology Trends Outlook, in which the McKinsey Technology Council identified the most significant technology trends unfolding today. This research is intended to help executives plan ahead by developing an understanding of potential use cases, sources of value, adoption drivers, and the critical skills needed to bring these opportunities to fruition.
Our analysis examines quantitative measures of interest, innovation, investment, and talent to gauge the momentum of each trend. Recognizing the long-term nature and interdependence of these trends, we also delve into the underlying technologies, uncertainties, and questions surrounding each trend. (For more about new developments in our research, please see the sidebar “What’s new in this year’s analysis”; for more about the research itself, please see the sidebar “Research methodology.”)
New and notable
The two trends that stood out in 2023 were gen AI and electrification and renewables. Gen AI has seen a spike of almost 700 percent in Google searches from 2022 to 2023, along with a notable jump in job postings and investments. The pace of technology innovation has been remarkable. Over the course of 2023 and 2024, the size of the prompts that large language models (LLMs) can process, known as “context windows,” spiked from 100,000 to two million tokens. This is roughly the difference between adding one research paper to a model prompt and adding about 20 novels to it. And the modalities that gen AI can process have continued to increase, from text summarization and image generation to advanced capabilities in video, images, audio, and text. This has catalyzed a surge in investments and innovation aimed at advancing more powerful and efficient computing systems. The large foundation models that power generative AI, such as LLMs, are being integrated into various enterprise software tools and are also being employed for diverse purposes such as powering customer-facing chatbots, generating ad campaigns, accelerating drug discovery, and more. We expect this expansion to continue, pushing the boundaries of AI capabilities. Senior leaders’ awareness of gen AI innovation has increased interest, investment, and innovation in AI technologies, such as robotics, which is a new addition to our trends analysis this year. Advancements in AI are ushering in a new era of more capable robots, spurring greater innovation and a wider range of deployments.
Research methodology
To assess the development of each technology trend, our team collected data on five tangible measures of activity: search engine queries, news publications, patents, research publications, and investment. For each measure, we used a defined set of data sources to find occurrences of keywords associated with each of the 15 trends, screened those occurrences for valid mentions of activity, and indexed the resulting numbers of mentions on a 0–1 scoring scale that is relative to the trends studied. The innovation score combines the patents and research scores; the interest score combines the news and search scores. (While we recognize that an interest score can be inflated by deliberate efforts to stimulate news and search activity, we believe that each score fairly reflects the extent of discussion and debate about a given trend.) Investment measures the flows of funding from the capital markets into companies linked with the trend.
Data sources for the scores include the following:
- Patents. Data on patent filings are sourced from Google Patents, where the data highlight the number of granted patents.
- Research. Data on research publications are sourced from Lens.
- News. Data on news publications are sourced from Factiva.
- Searches. Data on search engine queries are sourced from Google Trends.
- Investment. Data on private-market and public-market capital raises (venture capital and corporate and strategic M&A, including joint ventures), private equity (including buyouts and private investment in public equity), and public investments (including IPOs) are sourced from PitchBook.
- Talent demand. Number of job postings is sourced from McKinsey’s proprietary Organizational Data Platform, which stores licensed, de-identified data on professional profiles and job postings. Data are drawn primarily from English-speaking countries.
In addition, we updated the selection and definition of trends from last year’s report to reflect the evolution of technology trends:
- The future of robotics trend was added since last year’s publication.
- Data sources and keywords were updated. For data on the future of space technologies investments, we used research from McKinsey’s Aerospace & Defense Practice.
Finally, we used survey data to calculate the enterprise-wide adoption scores for each trend:
- Survey scope. The survey included approximately 1,000 respondents from 50 countries.
- Geographical coverage. Survey representation was balanced across Africa, Asia, Europe, Latin America, the Middle East, and North America.
- Company size. Size categories, based on annual revenue, included small companies ($10 million to $50 million), medium-size companies ($50 million to $1 billion), and large companies (greater than $1 billion).
- Respondent profile. The survey was targeted to senior-level professionals knowledgeable in technology, who reported their perception of the extent to which their organizations were using the technologies.
- Survey method. The survey was conducted online to enhance reach and accessibility.
- Question types. The survey employed multiple-choice and open-ended questions for comprehensive insights.
- 1: Frontier innovation. This technology is still nascent, with few organizations investing in or applying it. It is largely untested and unproven in a business context.
- 2: Experimentation. Organizations are testing the functionality and viability of the technology with a small-scale prototype, typically done without a strong focus on a near-term ROI. Few companies are scaling or have fully scaled the technology.
- 3: Piloting. Organizations are implementing the technology for the first few business use cases. It may be used in pilot projects or limited deployments to test its feasibility and effectiveness.
- 4: Scaling. Organizations are in the process of scaling the deployment and adoption of the technology across the enterprise. The technology is being scaled by a significant number of companies.
- 5: Fully scaled. Organizations have fully deployed and integrated the technology across the enterprise. It has become the standard and is being used at a large scale as companies have recognized the value and benefits of the technology.
Electrification and renewables was the other trend that bucked the economic headwinds, posting the highest investment and interest scores among all the trends we evaluated. Job postings for this sector also showed a modest increase.
Although many trends faced declines in investment and hiring in 2023, the long-term outlook remains positive. This optimism is supported by the continued longer-term growth in job postings for the analyzed trends (up 8 percent from 2021 to 2023) and enterprises’ continued innovation and heightened interest in harnessing these technologies, particularly for future growth.
In 2023, technology equity investments fell by 30 to 40 percent to approximately $570 billion due to rising financing costs and a cautious near-term growth outlook, prompting investors to favor technologies with strong revenue and margin potential. This approach aligns with the strategic perspective leading companies are adopting, in which they recognize that fully adopting and scaling cutting-edge technologies is a long-term endeavor. This recognition is evident when companies diversify their investments across a portfolio of several technologies, selectively intensifying their focus on areas most likely to push technological boundaries forward. While many technologies have maintained cautious investment profiles over the past year, gen AI saw a sevenfold increase in investments, driven by substantial advancements in text, image, and video generation.
About QuantumBlack, AI by McKinsey
QuantumBlack, McKinsey’s AI arm, helps companies transform using the power of technology, technical expertise, and industry experts. With thousands of practitioners at QuantumBlack (data engineers, data scientists, product managers, designers, and software engineers) and McKinsey (industry and domain experts), we are working to solve the world’s most important AI challenges. QuantumBlack Labs is our center of technology development and client innovation, which has been driving cutting-edge advancements and developments in AI through locations across the globe.
Despite an overall downturn in private equity investment, the pace of innovation has not slowed. Innovation has accelerated in the three trends that are part of the “AI revolution” group: gen AI, applied AI, and industrializing machine learning. Gen AI creates new content from unstructured data (such as text and images), applied AI leverages machine learning models for analytical and predictive tasks, and industrializing machine learning accelerates and derisks the development of machine learning solutions. Applied AI and industrializing machine learning, boosted by the widening interest in gen AI, have seen the most significant uptick in innovation, reflected in the surge in publications and patents from 2022 to 2023. Meanwhile, electrification and renewable-energy technologies continue to capture high interest, reflected in news mentions and web searches. Their popularity is fueled by a surge in global renewable capacity, their crucial roles in global decarbonization efforts, and heightened energy security needs amid geopolitical tensions and energy crises.
The talent environment largely echoed the investment picture in tech trends in 2023. The technology sector faced significant layoffs, particularly among large technology companies, with job postings related to the tech trends we studied declining by 26 percent—a steeper drop than the 17 percent decrease in global job postings overall. The greater decline in demand for tech-trends-related talent may have been fueled by technology companies’ cost reduction efforts amid decreasing revenue growth projections. Despite this reduction, the trends with robust investment and innovation, such as gen AI, not only maintained but also increased their job postings, reflecting a strong demand for new and advanced skills. Electrification and renewables was the other trend that saw positive job growth, partially due to public sector support for infrastructure spending.
Even with the short-term vicissitudes in talent demand, our analysis of 4.3 million job postings across our 15 tech trends underscored a wide skills gap. Compared with the global average, fewer than half the number of potential candidates have the high-demand tech skills specified in job postings. Despite the year-on-year decreases for job postings in many trends from 2022 to 2023, the number of tech-related job postings in 2023 still represented an 8 percent increase from 2021, suggesting the potential for longer-term growth (Exhibit 1).
Enterprise technology adoption momentum
The trajectory of enterprise technology adoption is often described as an S-curve that traces the following pattern: technical innovation and exploration, experimenting with the technology, initial pilots in the business, scaling the impact throughout the business, and eventual fully scaled adoption (Exhibit 2). This pattern is evident in this year’s survey analysis of enterprise adoption conducted across our 15 technologies. Adoption levels vary across different industries and company sizes, as does the perceived progress toward adoption.
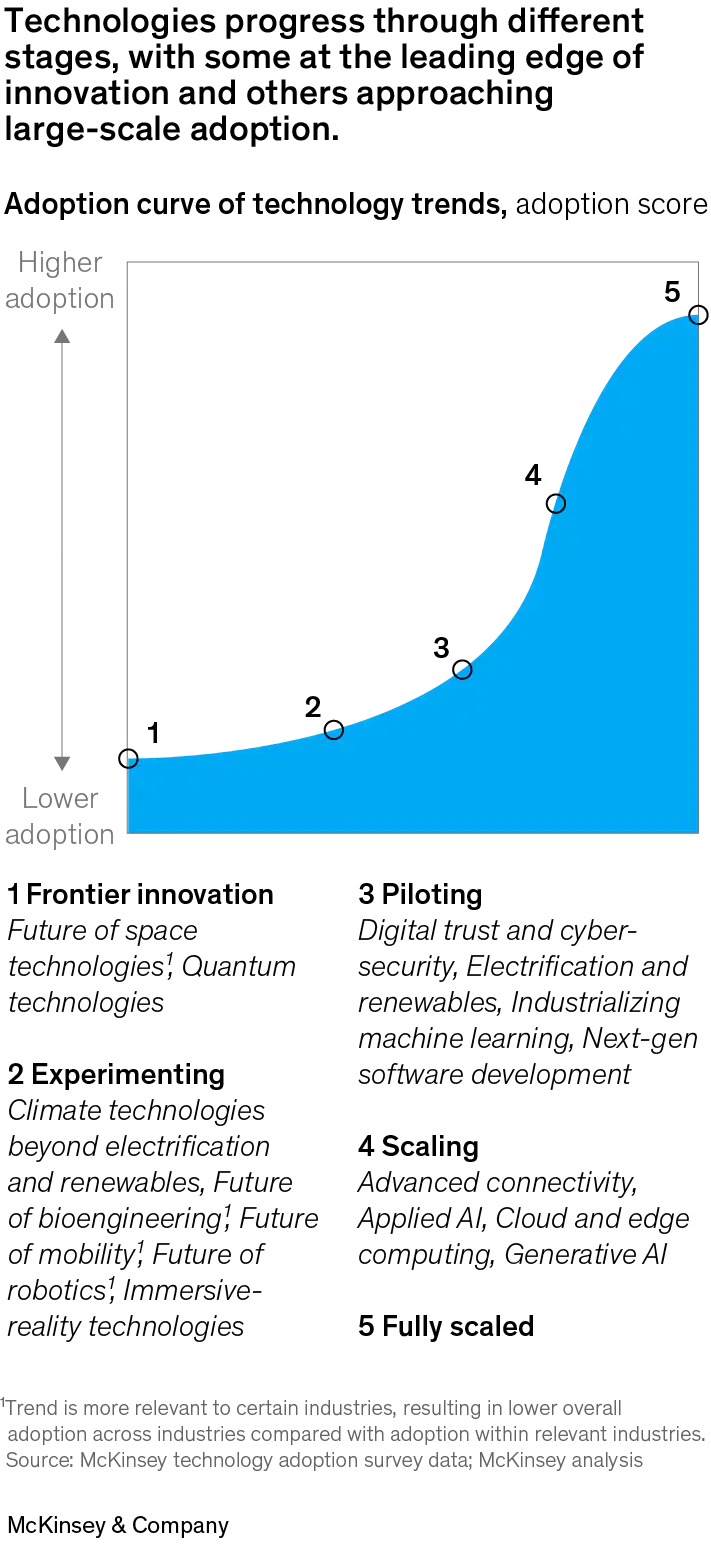
Image description:
A graph depicts the adoption curve of technology trends, scored from 1 to 5, where 1 represents frontier innovation, located at the bottom left corner of the curve; 2 is experimenting, located slightly above frontier innovation; 3 is piloting, which follows the upward trajectory of the curve; 4 is scaling, marked by a vertical ascent as adoption increases; and 5 is fully scaled, positioned at the top of the curve, indicating near-complete adoption.
In 2023, the trends are positioned along the adoption curve as follows: future of space technologies and quantum technologies are at the frontier innovation stage; climate technologies beyond electrification and renewables, future of bioengineering, future of mobility, future of robotics, and immersive-reality technologies are at the experimenting stage; digital trust and cybersecurity, electrification and renewables, industrializing machine learning, and next-gen software development are at the piloting stage; and advanced connectivity, applied AI, cloud and edge computing, and generative AI are at the scaling stage.
Footnote: Trend is more relevant to certain industries, resulting in lower overall adoption across industries compared with adoption within relevant industries.
Source: McKinsey technology adoption survey data
End of image description.
We see that the technologies in the S-curve’s early stages of innovation and experimenting are either on the leading edge of progress, such as quantum technologies and robotics, or are more relevant to a specific set of industries, such as bioengineering and space. Factors that could affect the adoption of these technologies include high costs, specialized applications, and balancing the breadth of technology investments against focusing on a select few that may offer substantial first-mover advantages.
As technologies gain traction and move beyond experimenting, adoption rates start accelerating, and companies invest more in piloting and scaling. We see this shift in a number of trends, such as next-generation software development and electrification. Gen AI’s rapid advancement leads among trends analyzed, about a quarter of respondents self-reporting that they are scaling its use. More mature technologies, like cloud and edge computing and advanced connectivity, continued their rapid pace of adoption, serving as enablers for the adoption of other emerging technologies as well (Exhibit 3).
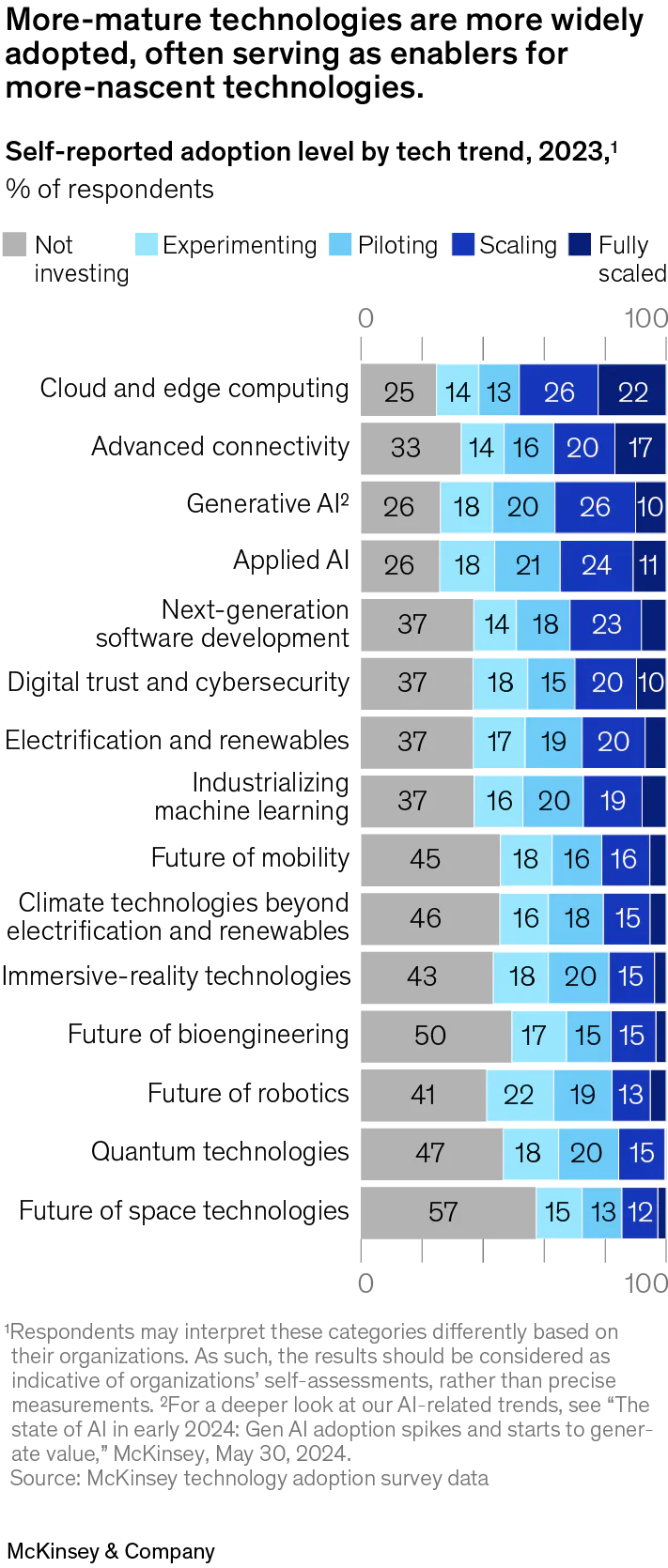
A segmented bar graph shows the adoption levels of tech trends in 2023 as a percentage of respondents. The trends are divided into 5 segments, comprising 100%: fully scaled, scaling, piloting, experimenting, and not investing. The trends are arranged based on the combined percentage sum of fully scaled and scaling shares. Listed from highest to lowest, these combined percentages are as follows:
- cloud and edge computing at 48%
- advanced connectivity at 37%
- generative AI at 36%
- applied AI at 35%
- next-generation software development at 31%
- digital trust and cybersecurity at 30%
- electrification and renewables at 28%
- industrializing machine learning at 27%
- future of mobility at 21%
- climate technologies beyond electrification and renewables at 20%
- immersive-reality technologies at 19%
- future of bioengineering at 18%
- future of robotics at 18%
- quantum technologies at 15%
- future of space technologies at 15%
The process of scaling technology adoption also requires a conducive external ecosystem where user trust and readiness, business model economics, regulatory environments, and talent availability play crucial roles. Since these ecosystem factors vary by geography and industry, we see different adoption scenarios playing out. For instance, while the leading banks in Latin America are on par with their North American counterparts in deploying gen AI use cases, the adoption of robotics in manufacturing sectors varies significantly due to differing labor costs affecting the business case for automation.
As executives navigate these complexities, they should align their long-term technology adoption strategies with both their internal capacities and the external ecosystem conditions to ensure the successful integration of new technologies into their business models. Executives should monitor ecosystem conditions that can affect their prioritized use cases to make decisions about the appropriate investment levels while navigating uncertainties and budgetary constraints on the way to full adoption (see the “Adoption developments across the globe” sections within each trend or particular use cases therein that executives should monitor). Across the board, leaders who take a long-term view—building up their talent, testing and learning where impact can be found, and reimagining the businesses for the future—can potentially break out ahead of the pack.
Lareina Yee is a senior partner in McKinsey’s Bay Area office, where Michael Chui is a McKinsey Global Institute partner, Roger Roberts is a partner, and Mena Issler is an associate partner.
The authors wish to thank the following McKinsey colleagues for their contributions to this research: Aakanksha Srinivasan, Ahsan Saeed, Alex Arutyunyants, Alex Singla, Alex Zhang, Alizee Acket-Goemaere, An Yan, Anass Bensrhir, Andrea Del Miglio, Andreas Breiter, Ani Kelkar, Anna Massey, Anna Orthofer, Arjit Mehta, Arjita Bhan, Asaf Somekh, Begum Ortaoglu, Benjamin Braverman, Bharat Bahl, Bharath Aiyer, Bhargs Srivathsan, Brian Constantine, Brooke Stokes, Bryan Richardson, Carlo Giovine, Celine Crenshaw, Daniel Herde, Daniel Wallance, David Harvey, Delphine Zurkiya, Diego Hernandez Diaz, Douglas Merrill, Elisa Becker-Foss, Emma Parry, Eric Hazan, Erika Stanzl, Everett Santana, Giacomo Gatto, Grace W Chen, Hamza Khan, Harshit Jain, Helen Wu, Henning Soller, Ian de Bode, Jackson Pentz, Jeffrey Caso, Jesse Klempner, Jim Boehm, Joshua Katz, Julia Perry, Julian Sevillano, Justin Greis, Kersten Heineke, Kitti Lakner, Kristen Jennings, Liz Grennan, Luke Thomas, Maria Pogosyan, Mark Patel, Martin Harrysson, Martin Wrulich, Martina Gschwendtner, Massimo Mazza, Matej Macak, Matt Higginson, Matt Linderman, Matteo Cutrera, Mellen Masea, Michiel Nivard, Mike Westover, Musa Bilal, Nicolas Bellemans, Noah Furlonge-Walker, Obi Ezekoye, Paolo Spranzi, Pepe Cafferata, Robin Riedel, Ryan Brukardt, Samuel Musmanno, Santiago Comella-Dorda, Sebastian Mayer, Shakeel Kalidas, Sharmila Bhide, Stephen Xu, Tanmay Bhatnagar, Thomas Hundertmark, Tinan Goli, Tom Brennan, Tom Levin-Reid, Tony Hansen, Vinayak HV, Yaron Haviv, Yvonne Ferrier, and Zina Cole.
They also wish to thank the external members of the McKinsey Technology Council for their insights and perspectives, including Ajay Agrawal, Azeem Azhar, Ben Lorica, Benedict Evans, John Martinis, and Jordan Jacobs.
Special thanks to McKinsey Global Publishing colleagues Barr Seitz, Diane Rice, Kanika Punwani, Katie Shearer, LaShon Malone, Mary Gayen, Nayomi Chibana, Richard Johnson, Stephen Landau, and Victor Cuevas for making this interactive come alive.
Explore a career with us
Related articles.
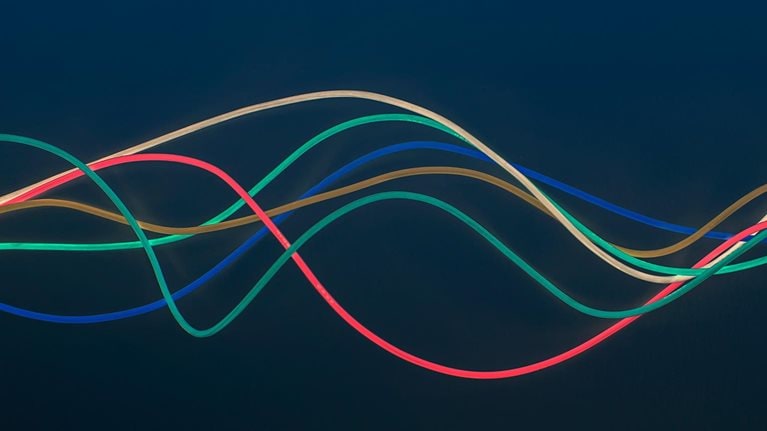
Rewired and running ahead: Digital and AI leaders are leaving the rest behind

False friends or good ends? The CIO’s four-point guide to navigating technology trends
Popular Searches
Your previous searches, recently visited pages.
Content added to Red Folder
Removed from Red Folder
Coming Soon: Technology Report 2024
Bain’s annual report examines the state of the technology sector as skyrocketing AI demand rearranges the competitive landscape, creates profit pools, and generates new opportunities.
Sign up for the report
Receive our technology insights delivered to your inbox, including our upcoming annual report when it is published.
Thank you for registering to receive Bain’s technology insights, including our upcoming Technology Report 2024. Once you confirm your email address, you will start receiving our latest research on the industries and business topics that matter most to you.
Technology Report 2023
Bain's annual report examines the technology sector's period of renewal and reinvention, propelled by strong global demand and investments in resilient value chains and generative AI.

Contact Bain
How can we help you?
- Business inquiry
- Career information
- Press relations
- Partnership request
- Speaker request

Browsing: Technology
Read the latest technology news on SciTechDaily, your comprehensive source for the latest breakthroughs, trends, and innovations shaping the world of technology. We bring you up-to-date insights on a wide array of topics, from cutting-edge advancements in artificial intelligence and robotics to the latest in green technologies, telecommunications, and more.
Our expertly curated content showcases the pioneering minds, revolutionary ideas, and transformative solutions that are driving the future of technology and its impact on our daily lives. Stay informed about the rapid evolution of the tech landscape, and join us as we explore the endless possibilities of the digital age.
Discover recent technology news articles on topics such as Nanotechnology , Artificial Intelligence , Biotechnology , Graphene , Green Tech , Battery Tech , Computer Tech , Engineering , and Fuel-cell Tech featuring research out of MIT , Cal Tech , Yale , Georgia Tech , Karlsruhe Tech , Vienna Tech , and Michigan Technological University . Discover the future of technology with SciTechDaily.
Scientists Develop New Molecular Strategies To Break the Barriers of Electronic Miniaturization
University of Illinois researchers have innovated in molecular electronics by creating stable, shape-persistent molecules with…
Nervous System’s Master Matchmaker Sparks Breakthrough in Computer Science
A researcher has enhanced the bipartite matching problem in computer science by drawing parallels with…
New Graphene Technology Could Revolutionize Battery Safety and Performance
Researchers have developed a scalable method for producing large graphene current collectors, significantly improving lithium-ion…
Memory Breakthrough: Helical Magnets Pave the Way for Next-Gen Storage
Researchers have developed a new magnet-based memory device using helical magnets, promising high-density, non-volatile storage…
Brighter Than Ever: Tiny Green Lasers Are Changing the Game
NIST scientists have made a breakthrough in laser technology by filling the “green gap” with…
Researchers Discover a Surprising Way To Jump-Start Battery Performance
A study conducted at the SLAC-Stanford Battery Center has found that charging lithium-ion batteries at…
Tiny Laser-Plasma Accelerator Breakthrough Could Revolutionize Scientific Research
Laser-plasma accelerators could revolutionize scientific research by providing compact, efficient particle acceleration. They are seen…
Finally Revealed: The Surprising Cause of Qubit Decay in Quantum Computers
Researchers at Aalto University have uncovered the thermal energy loss in qubits through a simple…
Revolutionary Eco-Friendly Cooling Device Shatters Performance World Record
HKUST’s new cooling device breaks world records with a 75 K temperature lift and a…
The Future of Fighting Disease: AI Detects Cancer and Viral Infections With Nanoscale Precision
Researchers have developed AINU, an AI that can differentiate between cancer and normal cells and…
Hydrogels Take On Pong: How They “Learn” To Win
Researchers have discovered that non-living hydrogels can play the video game Pong and improve over…
Turning Heat Into Custom Light With Advanced Nanotechnology
Researchers at the CUNY Graduate Center have made significant strides in manipulating the optical properties…
Quantum Breakthrough: Scientists Use Sound Waves To Enable the Future of the Internet
Researchers have developed new methods for coupling light to surface-gliding sound waves. Researchers at the…
Fusion’s New Hero: The Liquid Metal That Beats the Heat
Scientists at the Princeton Plasma Physics Laboratory are pioneering the use of liquid lithium in…
Perovskite Waveguides: Revolutionary Crystals for Next-Gen Photonics
Scientists have developed perovskite crystals that could revolutionize optical technologies by facilitating efficient room-temperature operations…
130-Million-Year-Old Navigation Trick Could Transform Space and Drone Tech
An AI sensor accurately measures the orientation of the Milky Way. A new research study…
Revolutionary Solar Cells Set To Slash Costs and Boost Energy Production
Rice University’s new method for synthesizing stable, high-quality perovskite solar cells promises to revolutionize solar…
Revolutionary Superconductor Set to Turbocharge Quantum Computers
Physicists have developed a groundbreaking superconductor material that could revolutionize the scalablity and reliability of…
Type above and press Enter to search. Press Esc to cancel.
RESEARCH REPORT
Technology Vision 2024
Human by design
15-minute read
January 9, 2024
- Technology is becoming human by design, and enterprises that prepare now will win in the future.
- How people access and interact with information is radically changing as human-like, AI-powered chatbots synthesize vast amounts of information and provide answers and advice.
- AI is starting to reason like us, and will soon comprise entire ecosystems of AI agents who will work with one another and act for people and organizations alike.
- A new spatial computing medium is emerging, letting the digital world reflect what it means to be human and in a physical space.
- The challenge of tech not understanding us and our intent is disappearing: machines are getting much better at interacting with humans on their level.
Human by design: How AI unleashes the next level of human potential
It’s time to make technology human by design..
This is a moment for reinvention. In the coming years, businesses will have an increasingly powerful array of technologies at their disposal that will open new pathways to unleash greater human potential, productivity, and creativity. Early adopters and leading businesses have kick-started a race toward a new era of value and capability. And their strategies are underpinned by one common thread – the technology is becoming more human.
It sounds counterintuitive: after all, wasn’t technology built by, and for, humans? Creating tools that expand our physical and cognitive abilities is so unique to humanity that some argue it defines us as a species.
Despite this, the tools we build are often distinctly unhuman, filling gaps by doing and being what we couldn’t, and in the process radically transforming our lives. Automobiles expanded our freedom of mobility. Cranes let us build skyscrapers and bridges. Machines helped us create, distribute, and listen to music.
Technology’s unhuman nature can also be its drawback. Extended use of hand tools can lead to arthritis. Years of looking at screens can accelerate vision problems. We have amazing navigational tools, but they still distract us from driving. Granted, there have been efforts to create tools that are more ergonomic or easier to use. But even so, time and again we see and make decisions about our lives based on what is best for a machine rather than optimizing human potential.
Now, for the first time in history, there’s strong evidence to indicate that we are reversing course—not by moving away from technology, but rather by embracing a generation of technology that is more human. Technology that is more intuitive, both in design and its very nature, demonstrates more human-like intelligence, and is easy to integrate across every aspect of our lives.
Generative AI has the potential to impact much more than just the task at hand. It’s also starting to profoundly reshape organizations and markets.
Consider the impact generative AI and transformer models are having on the world around us. What began as chatbots like ChatGPT and Bard has become a driving force in making technology more intuitive, intelligent and accessible to all. Where AI once focused on automation and routine tasks, it’s now shifting to augmentation, changing how people approach work, and is rapidly democratizing the technologies and specialized knowledge work that were once reserved for the highly trained or deep pocketed.
Of course, the advent of more human technology isn’t limited to AI: It’s starting to address many of the pain points that exist between us and technology, paving the way for greater human potential.
Technology that is human by design will reach new people and expand access to knowledge, which will enable ongoing innovation. Think of all the people historically alienated by technology who will be able to contribute to the digital revolution. As technology becomes more intuitive, we can tap into these people as new customers and new employees.
Make It Human—the 2024 Trends
A match made in AI
People are asking generative AI chatbots for information – transforming the business of search today, and the futures of software and data-driven enterprises tomorrow.
Meet my agent
AI is taking action, and soon whole ecosystems of AI agents could command major aspects of business. Appropriate human guidance and oversight is critical.
The space we need
The spatial computing technology landscape is rapidly growing, but to successfully capitalize on this new medium, enterprises will need to find its killer apps.
Our bodies electronic
A suite of technologies – from eye-tracking to machine learning to BCI – are starting to understand people more deeply, and in more human-centric ways.

of executives agree that making technology more human will massively expand the opportunities of every industry.
Leaders will face familiar questions: Which products and services are ripe for scaling? What new data is at your disposal? What transformative actions can you take? But they will also be at the center of answering questions they may have never expected: What kind of oversight does AI need? Who will be included in the digital transformation? What responsibilities do we have to the people in our ecosystem?
Human by design is not just a description of features, it’s a mandate for what comes next. As enterprises look to reinvent their digital core, human technology will become central to the success of their efforts. Every business is beginning to see the potential emerging technologies have to reinvent the pillars of their digital efforts. Digital experiences, data and analytics, products, all stand to change as technologies like generative AI, spatial computing, and others mature and scale.
In this moment of reinvention, enterprises have the chance to build a strategy that maximizes human potential, and erases the friction between people and technology. The future will be powered by artificial intelligence but must be designed for human intelligence. And as a new generation of technology gives enterprises the power to do more, every choice they make matters that much more too. The world is watching. Will you be a role model or a cautionary tale?
of executives agree that with rapid technological advancements, it is more important than ever for organizations to innovate with purpose.
Trend 1 - A match made in AI: Reshaping our relationship with knowledge
The big picture.
Our relationship with data is changing—and with it, how we think, work, and interact with technology. The entire basis of the digital enterprise is getting disrupted.
The search-based “librarian” model of human-data interaction is giving way to a new “advisor” model. Rather than running searches to curate results, people are now asking generative AI chatbots for answers . Case in point: OpenAI launched ChatGPT in November 2022, and it became the fastest-growing app of all time. Large language models (LLMs) had been around for years, but ChatGPT’s ability to answer questions in a direct and conversational manner made a huge difference.
Data is one of the most important factors shaping today’s digital businesses. And the new chatbots—that can synthesize vast amounts of information to provide answers and advice, use different data modalities, remember prior conversations, and even suggest what to ask next—are disrupting that undercurrent. Ultimately, these chatbots can operate as LLM-advisors, allowing companies to put one with the breadth of enterprise knowledge at every employee’s fingertips. This could unlock the latent value of data and finally let enterprises tap into the promise of data-driven business.
With generative AI, a digital butler is finally in the cards.
Companies possess valuable, unique information they want customers, employees, partners, and investors to find and use. But whether it’s because we don’t recall the right search terms, we can’t write the query, the data is siloed, or the documents are too dense, a lot of that information is hard to access or distill. For the data-driven business of today, that’s serious untapped value that generative AI could bring in.
However, the true disruption here isn’t just in how we access data; it’s in the potential to transform the entire software market. What if the interface to every app and digital platform became a generative AI chatbot? What if that became the way we read, write, and interact with data, as a core competency of all platforms?
To truly reap the benefits of generative AI and build the data-and-AI powered enterprise of the future, businesses need to radically rethink their core technology strategy. How they gather and structure data, their broader architectures, and how they deploy technology tools and the features they include need to be rethought. And new practices like training, debiasing, and AI-oversight must be built in from the start.
of executives believe generative AI will compel their organization to modernize its technology architecture.
The technology: Unlocking your data-driven enterprise
Shoring up your data foundation.
New technologies and techniques can help enterprises shore up their data foundation and prepare for the future of data-driven business. Wherever companies start from, LLM-advisors will demand a data foundation that’s more accessible and contextual than ever.
The knowledge graph is one of the most important technologies here. It’s a graph-structured data model including entities and the relationships between them, which encodes greater context and meaning. Not only can a knowledge graph aggregate information from more sources and support better personalization, but it can also enhance data access through semantic search.
In addition to knowledge graphs, data mesh and data fabric are two ways to help map and organize information that businesses should consider as they update their overall architecture.
Exploring LLMs as your new data interface
On their own, knowledge graphs, data mesh, and data fabric would be a huge step up for enterprise knowledge management systems. But there’s much value to be gained in taking the next step and shifting from the librarian to advisor model. Imagine if instead of using a search bar, employees could ask questions in natural language and get clear answers across every website and app in the enterprise. With an accessible and contextual data foundation, enterprises can start to build this—and there are a few options.
First, companies can train their own LLM from scratch, though this is rare given the significant resources required. A second option is to “fine-tune” an existing LLM. Essentially, this means taking a more general LLM and adapting it to a domain by further training it on a set of domain-specific documents. This option is best for domain-specific cases when real-time information is not necessary, like for creative outputs in design or marketing.
Enterprises are also beginning to fine-tune smaller language models (SLMs) for specialized use cases. These SLMs are more efficient, running at lower cost with smaller carbon footprints, and can be trained more quickly and used on smaller, edge devices.
Lastly, one of the most popular approaches to building an LLM-advisor has been to “ground” pre-trained LLMs by providing them with more relevant, use case-specific information, typically through retrieval augmented generation (RAG).
The field of generative AI and LLMs is moving fast, but whatever way you choose to explore, one thing will stay constant: your data foundation needs to be solid and contextual, or your LLM-advisor will never live up to its promise.
The implications: The future of enterprise knowledge
Understanding and mitigating risks.
First and most importantly, as businesses begin to explore the new possibilities LLM-advisors bring, they need to understand the associated risks.
Take “hallucinations,” an almost intrinsic characteristic of LLMs. Because they are trained to deliver probabilistic answers with a high degree of certainty, there are times when these advisors confidently relay incorrect information. And while hallucinations are perhaps LLMs’ most notorious risk, other issues must be considered. If using a public model, proprietary data must be carefully protected so that it cannot be leaked. And for private models too, data cannot be shared with employees who should not have access. The cost of computing is something that needs to be managed. And underlying everything, few people have the relevant expertise to implement these solutions well.
All that said, these challenges shouldn’t be taken as a deterrent, but rather as a call to implement the technology with appropriate controls.
The data going into the LLM—whether through training or the prompt—should be high quality data: fresh, well-labeled, and unbiased. Training data should be zero-party and proactively shared by customers, or first-party and collected directly by the company. And security standards should be implemented to protect any personal or proprietary data. Finally, data permissions must also be in place to ensure that the user is allowed to access any data retrieved for in-context learning.
Beyond accuracy, the outputs of the generative AI chatbot should also be explainable and align with the brand. Guardrails can be put in place so that the model does not respond with sensitive data or harmful words, and so that it declines questions outside its scope. Moreover, responses can convey uncertainty and provide sources for verification.
Finally, generative AI chatbots should be subject to continuous testing and human oversight. Companies should invest in ethical AI and develop minimum standards to adhere to. And they should gather regular feedback and provide training for employees as well.
Security implications
Among the many other security implications already discussed in this trend, companies should also think about how LLM-advisors may change user data dynamics.
We have an opportunity to reinvent the ethos of search and restore trust between businesses and their customers. Companies can now act as stewards of their own information—storing, securing, analyzing, and disseminating their data and institutional knowledge directly to customers through digital advisors. This is a big responsibility: your company must ensure that your data remains secure while yielding high-confidence responses in your advisory services. It’s an even bigger opportunity: without search providers mediating the exchange of information, companies can serve as a direct source of reliable insight and win back their customers’ trust.
Generative AI is the ultimate game-changer for data and software. LLMs are changing our relationship with information, and everything from how enterprises reach customers to how they empower employees and partners stands to transform. Leading companies are already diving in, imagining and building the next generation of data-driven business. And before long, it won’t just be leaders. It’ll be the new way digital business works.
Trend 2 - Meet my agent: Ecosystems for AI
AI is breaking out of its limited scope of assistance to engage more and more of the world through action . Over the next decade, we will see the rise of entire agent ecosystems—large networks of interconnected AI that will push enterprises to think about their intelligence and automation strategy in a fundamentally different way.
Today, most AI strategies are narrowly focused on assisting in task and function. To the extent that AI acts, it is as solitary actors, rather than an ecosystem of interdependent parts. But as AI evolves into agents, automated systems will make decisions and take actions on their own. Agents won’t just advise humans, they will act on humans’ behalf. AI will keep generating text, images, and insights, but agents will decide for themselves what to do with it.
As agents are promoted to become our colleagues and our proxies, we will need to reimagine the future of tech and talent together.
While this agent evolution is just getting underway, companies already need to start thinking about what’s next. Because if agents are starting to act, it won’t be long until they start interacting with each other . Tomorrow’s AI strategy will require the orchestration of an entire concert of actors: narrowly-trained AI, generalized agents, agents tuned for human collaboration, and agents designed for machine optimization.
But there’s a lot of work to do before AI agents can truly act on our behalf, or as our proxy. And still more before they can act in concert with each other. The fact is, agents are still getting stuck, misusing tools, and generating inaccurate responses—and these are errors that can compound quickly.
Humans and machines have been paired at the task-level, but leaders have never prepared for AI to operate our businesses—until today. As agents are promoted to become our colleagues and our proxies, we will need to reimagine the future of tech and talent together. It’s not just about new skills, it’s about ensuring that agents share our values and goals. Agents will help build our future world, and it’s our job to make sure it’s one we want to live in.
of executives agree leveraging AI agent ecosystems will be a significant opportunity for their organizations in the next 3 years.
The technology: From assistance to actions to ecosystems
As AI assistants mature into proxies that can act on behalf of humans, the resulting business opportunities will depend on three core capabilities: access to real time data and services; reasoning through complex chains of thought; and the creation of tools—not for human use, but for the use of the agents themselves.
Starting with access to real time data and services: When ChatGPT first launched, a common mistake people made was thinking the application was actively looking up information on the web. In reality, GPT-3.5 (the LLM upon which ChatGPT was initially launched) was trained on an extremely wide corpus of knowledge and drew on the relationships between that data to provide answers.
But new plugins to enable ChatGPT to access the internet were soon announced that could transform foundation models from powerful engines working in isolation to agents with the ability to navigate the current digital world. While plugins have powerful innovative potential on their own, they’ll also play a critical role in the emergence of agent ecosystems.
The second step in the agent evolution is the ability to reason and think logically—because even the simplest everyday actions for people require a series of complex instructions for machines. AI research is starting to break down barriers to machine reasoning. Chain-of-thought prompting is an approach developed to help LLMs better understand steps in a complex task.
Between chain-of-thought reasoning and plugins, AI has the potential to take on complex tasks by using both tighter logic and the abundance of digital tools available on the web. But what happens if the required solution isn’t yet available?
When humans face this challenge, we acquire or build the tools we need. AI used to rely on humans exclusively to grow its capabilities. But the third dimension of agency we are seeing emerge is the ability for AI to develop tools for itself.
The agent ecosystem may seem overwhelming. After all, beyond the three core capabilities of autonomous agents, we’re also talking about an incredibly complex orchestration challenge, and a massive reinvention of your human workforce to make it all possible. It’s enough to leave leaders wondering where to start. The good news is existing digital transformation efforts will go a long way to giving enterprises a leg up.
The implications: Aligning tech and talent in the workforce
What happens when the agent ecosystem gets to work? Whether as our assistants or as our proxies, the result will be explosive productivity, innovation and the revamping of the human workforce. As assistants or copilots, agents could dramatically multiply the output of individual employees. In other scenarios, we will increasingly trust agents to act on our behalf. As our proxies, they could tackle jobs currently performed by humans, but with a giant advantage—a single agent could wield all of your company’s knowledge and information.
Businesses will need to think about the human and technological approaches they need to support these agents. From a technology side, a major consideration will be how these entities identify themselves. And the impacts on human workers—their new responsibilities, roles, and functions—demand even deeper attention. To be clear, humans aren’t going anywhere. Humans will make and enforce the rules for agents.
Rethinking human talent
In the era of agent ecosystems, your most valuable employees will be those best equipped to set the guidelines for agents. A company’s level of trust in their autonomous agents will determine the value those agents can create, and your human talent is responsible for building that trust.
But agents also need to understand their limits. When does an agent have enough information to act alone, and when should it seek support before taking action? Humans will decide how much independence to afford their autonomous systems.
What companies can do now
What can you do now to set your human and agent workforce up for success? Give agents a chance to learn about your company, and give your company a chance to learn about agents.
Companies can start by weaving the connective fabric between agents’ predecessors, LLMs, and their support systems. By fine-tuning LLMs on your company’s information, you are giving foundation models a head-start at developing expertise.
It's also time to introduce humans to their future digital co-workers. Companies can lay the foundation for trust with future agents by teaching their workforce to reason with existing intelligent technologies. Challenge your employees to discover and transcend the limits of existing autonomous systems.
Finally, let there be no ambiguity about your company’s North Star. Every action your agents take will need to be traced back to your core values and a mission, so it is never too early to operationalize your values from the top to the bottom of your organization.
From a security standpoint, agent ecosystems will need to provide transparency into their processes and decisions. Consider the growing recognition of the need for a software bill of materials – a clear list of all the code components and dependencies that make up a software application – so as to let companies and agencies under the hood. Similarly, an agent bill of materials could help explain and track agent decision-making.
What logic did the agent follow to make a decision? Which agent made the call? What code was written? What data was used and with whom was that data shared? The better we can trace and understand agent decision-making processes, the more we can trust agents to act on our behalf.
Agent ecosystems have the potential to multiply enterprise productivity and innovation to a level that humans can hardly comprehend. But they will only be as valuable as the humans that guide them; human knowledge and reasoning will give one network of agents the edge over another. Today, artificial intelligence is a tool. In the future, AI agents will operate our companies. It is our job to make sure they don’t run amok. Given the pace of AI evolution, the time to start onboarding your agents is now.
Trend 3 - The space we need: Creating value in new realities
Spatial computing is about to change not just the course of technology innovation, but also the ways people work and live. Whereas desktop and mobile used screens as portals to the digital world, spatial will finally combine our disparate realities, fusing digital and physical together. Apps built for this medium will let people immerse themselves in digital worlds with a physical sense of space, or layer content on top of their physical surroundings.
So, why doesn’t it feel like we’re at the beginning of a new technology era? Why are we inundated instead with talk of a “metaverse slump”? The metaverse is one of the best-known applications of spatial computing. But just look at the price of digital real estate, booming in 2021 and 2022, and down 80-90% in 2023.
Spatial computing is about to change the course of technology innovation and the ways people work and live.
Some enterprises are holding off, content to say metaverse hype outpaced technology maturity. But others are racing ahead, building the technology capabilities. Meta has been rapidly developing VR and AR products, and introduced Codex Avatars, which use AI and smartphone cameras to create photorealistic avatars. Epic’s RealityScan App lets people scan 3D objects in the physical world with just their phone and turn them into 3D virtual assets.
Underlying it all, advancing technologies like generative AI continue to make it faster and cheaper to build spatial environments and experiences. And, perhaps quietly, these technologies are already being proven out in industrial applications. Digital twins for manufacturing, the growth of VR/AR in training and remote operation, and the establishment of collaborative design environments are all already having practical – and valuable – impacts on industry.
The truth is that new mediums don’t come very often, and when they do, the uptake is slow. But the payoff for diving in early is nearly immeasurable.
of executives agree their organization plans to create a competitive advantage leveraging spatial computing.
The technology: Today’s spatial technology landscape
Critically, new standards, tools and technologies are making it easier—and cheaper—to build spatial apps and experiences that feel familiar.
Think about the websites you frequent or your favorite apps on your phone. Even if their purposes are different, something feels undeniably universal across even the most disparate experiences. Why? They all used the same foundation.
For a long time, spatial never had such a foundation. Enter Universal Scene Description (USD), or what can best be described as a file format for 3D spaces. Developed by Pixar, USD is a framework that lets creators map out aspects of a scene, including specific assets and backgrounds, lighting, characters and more. Since USD is designed around bringing these assets together in a scene, different software can be used across each one, enabling collaborative content building and non-destructive editing. USD is quickly becoming central to the most impactful spatial applications, notably within industrial digital twins.
Enterprises need to understand they will not be operating spaces in isolation. Just as no webpage or app exists on the internet alone, the next iteration of the web promises to bring these parallel experiences even closer together.
Sense of place
One emerging capability that differentiates spatial computing from its digital counterparts is engaging our senses. New technologies are letting engineers design experiences that address all types of senses, like touch, smell and sound.
In past VR attempts, adding haptics, or touch, could be bulky or underwhelming. But University of Chicago researchers recently proposed using electrodes to better mimic touch.
Scents can make digital spaces lifelike, too, by evoking memories or triggering the all-important fight-or-flight response. Scentient, a company trying to bring olfactory senses to the metaverse, have been experimenting with the technology for training firefighters and emergency responders, where smells, like the presence of natural gas, can be critical for evaluating an emergency.
Of course, sound, or spatial audio, is also critical to realistic digital scene-building. Lastly, immersive spatial apps will need to respond to how we naturally move.
The implications: Spatial’s killer applications
Spatial computing is not coming to replace desktop or mobile computing, but it is becoming an important piece of the computing fabric that makes up enterprise IT strategy.
We’ve already seen the early stages. Digital twins make more sense when you walk through them. Training is more impactful when you can live the experience rather than watch a video. While these were often standalone pilots, a careful consideration of the unique advantages of spatial computing can help shape and guide enterprise strategy. The market is still maturing, but it is quickly becoming clear that spatial apps thrive when applied in three ways: conveying large volumes of complex information; giving users agency over their experience; and, perhaps counterintuitively, allowing us to augment physical spaces.
When it comes to conveying complex information, the advantage of the spatial medium over the alternatives is probably clearest. Since a space can let users move and act naturally, information can be conveyed in more dynamic, immersive ways. We’ve already seen it in action. Some of the earliest examples of successful spatial apps were industrial digital twins, virtual training scenarios, or real-time remote assistance.
The second advantage spatial has over older mediums is the ability to give users agency to shape their in-app experiences. Because spatial computing lets us build digital experiences that embody a physical sense of space, we can design experiences that give users more flexibility to move and explore.
Lastly, spatial applications bring advantages to physical spaces; they can augment, enhance, and extend physical places without materially changing them. Imagine a future office where physical monitors, projectors, and displays are replaced by spatial computers and apps. People will have the flexibility to design simpler spaces, lowering overhead costs, and to change their surroundings more easily.
As the working world goes spatial, businesses will also need to think about security. There will be more devices than ever – employees will use spatial devices for work, customers will use them to access experiences. And with this ever expanding device ecosystem there will be more entry points for attackers too. So how do you put borders on the borderless? Businesses’ spatial strategies will need to be designed with zero trust principles.
Additionally, businesses should recognize that spatial is unfamiliar territory, so both vendors and users should expect to have blind spots. One line of defense won’t be enough, but Defense in Depth strategies that leverage multiple layers of security (like administrative, technical, and physical) can be deployed to defend this new frontier.
Spatial computing is about to hit its stride, and the race is on for leaders to get ahead. To position themselves at the top of the next era of technology innovation, enterprise leaders will need to rethink their position on spatial and recognize the effect recent technology advances are about to have. New computing mediums are few and far between, and they can have immeasurable impact on businesses and people for decades. Are you ready to immerse yourself in the moment?
Trend 4 - Our bodies electronic: A new human interface
Failing to understand people is a limiting factor for many of technologies we use today. Just think about the robots and drones that humans can only control if we translate what we want into commands they recognize. The fact is, when tech struggles to connect with us, it’s often because people—what they want, expect, or intend—are an enigma.
Now, innovators are trying to change that. Across industries, they’re building technologies and systems that can understand people in new and deeper ways. They’re creating a “human interface,” and the ripple effect will go far beyond, say, improving smart homes.
Look at how neurotech is beginning to connect with people’s minds. Recently, two separate studies from researchers at the University of California San Francisco and Stanford University demonstrated using neural prostheses—like brain-computer interfaces (BCI)—to decode speech from neural data. This could help patients with verbal disabilities “talk” by translating attempted speech into text or generated voices.
When technologies can better understand us—our behavior and our intentions—they will more effectively adapt to us.
Or consider technologies that read body movement, like eye and hand tracking. In 2023, Apple’s Vision Pro introduced visionOS, which lets users navigate and click with just their gaze and a simple gesture, bypassing the need for a handheld controller.
Innovations like these are rewriting the rules and pushing the limits that have guided human-machine interaction for decades. So often today, we bend over backwards, adapting and changing what we do to make technologies work. But the “human interface” will turn that on its head; when technologies can better understand us—our behavior and our intentions—they will more effectively adapt to us.
To succeed, enterprises will also need to address growing issues around trust and technology misuse. Companies and individuals alike may balk at the idea of letting technology read and understand us in these new and more intimate ways. Biometric privacy standards will need to be updated. And new neuroethics safeguards will need to be defined, including how to appropriately handle brain and other biometric data that can be used to infer people’s intentions and cognitive states. Until formal regulations catch up, it’s on enterprises’ shoulders to earn people’s trust.
of consumers agree they are often frustrated that technology fails to understand them and their intentions accurately.
The technology: A more human-centric view of people
Attempting to understand people—as individuals, target groups or populations—is a centuries’ old business challenge. And in recent decades, using digital technology to do this has been the ultimate differentiator. Digital platforms and devices have let businesses track and quantify people’s behaviors with enormously valuable impact. Now, the “human interface” is changing the game again, making it possible to understand people in deeper, more human-centric ways.
Recent technologies used to understand people have been based on tracking and observing patterns that lack specificity. People may read or watch familiar content, but they may actually want something new. We’re very good at recognizing what people do, but we don’t always understand why they do it.
How the “human interface” measures intent
The “human interface” isn’t any one single technology. Rather, it encompasses a suite of technologies that are deepening how innovators see and make sense of people.
Some are using wearable devices to track biosignals that can help predict what people want or understand their cognitive state. Others are building more detailed ways to understand people’s intent in relation to their environments.
Another approach to human intent is through AI. Consider human-robot collaborations. People’s state of mind, like if they’re feeling ambitious or tired, can impact how they approach a task. But while humans tend to be good at understanding these states of mind, robots haven’t been. But efforts are underway to teach robots to identify these states.
Lastly, perhaps one of the most exciting “human interface” technologies is neurotech: neuro-sensing and BCI. Many new neurotech companies have appeared in the last decade, and the field holds clear potential to read and identify human intent.
Neurotech highlights the pace of “human interface” advances
Many may think neural-sensing and BCI are years away from widespread commercial use, but recent advances tell a different story.
Skeptics tend to assert that neurotech will stay limited to the healthcare industry. But new use cases are being identified by the day. Two key advances are driving this. The first is decoding brain signals. Advances in AI pattern detection, as well as greater availability of brain data, are making a big difference.
The second area to watch is neuro-hardware – specifically, the quality of external devices. Historically, EEG (electroencephalogram) and fMRI (functional magnetic resonance imaging) have been two of the most widely used external brain sensing techniques. However, until recently, capturing either type of brain signal required a lab setting. But that is starting to change.
The implications: Getting started—the right way
As more enterprises start to build “human interface” strategies, they should begin by scoping out the different business areas and challenges that can be transformed.
First, consider how “human interface” technologies are raising the bar when it comes to anticipating people’s actions. Some of the most promising use cases are in areas where people and machines operate in shared spaces. For instance, enterprises could create safer and more productive manufacturing systems if robots could anticipate what people were about to do.
Another area that can be transformed is direct human-machine collaboration: how we use and control technology. As an example, think about how neurotech is letting us tap into our minds and connect with technology in new, potentially more natural ways.
Lastly, the “human interface” could drive the invention of new products and services. Brain-sensing, for instance, could help people “get” themselves better. L'Oréal is working with EMOTIV to help people better understand their fragrance preferences.
Still others are thinking about the “human interface” as a safety measure. Meili Technologies is a startup working to improve vehicle safety. It uses deep learning, visual inputs, and in-cabin sensors to detect if a driver has been incapacitated by a heart attack, seizure, stroke, or other emergency.
Business competition is changing—and trust is more important than ever
Businesses need to start assessing the risks posed by these technologies, and what new policies and safeguards need to be put in place. Rather than wait for regulations to ramp up, responsible enterprises need to begin now, looking to existing biometric laws and to the medical industry for guidance.
If tin foil hats don’t prevent mind reading, what will? More than any other trend this year, security will make or break enterprise and consumer adoption of the “human interface."
Acceptance of more perceptive and connected tools hinges on humans’ ability to be the primary gatekeepers of what information gets shared, at a minimum. This practice needs to be integrated into the design of the next generation of human-computer interface tools, letting people either opt into sharing data or telemetry relevant to the task at hand, or opt out of sharing extraneous or sensitive information.
The human interface is a new approach to addressing one of the oldest business challenges: understanding people as humans . That’s a big responsibility and an even bigger opportunity. People will have questions, and concerns about privacy will be the first and most important hurdle enterprises face. But the chance to understand people in this deeper, more human-centric way, is worth it.
Positive engineering: Our technology crossroads
The world is arriving at what might be technology’s biggest inflection point in history, and enterprises—and the decisions their leaders make—are at the heart of shaping how we move forward.
As we experience more growth and innovation, it won’t all be for the better. There will be more (and new) opportunities for fraud, misinformation and breaches of security. If we engineer tools with human capabilities but without human intelligence—or even human conscience—we can create in a way that deteriorates both the bottom line and the greater good.
In the era of human tech, every product and every service that enterprises bring to market holds the potential to transform lives, empower communities, and ignite change, for better or for worse. And, invariably, enterprises will face the delicate balancing act of needing to act fast versus needing to act carefully, as well as the expectation that competitors or other countries may not share the same concerns or impose the same guardrails.
As we strive to make technology human by design, we need to think of security as an enabler, an essential way to build trust between people and technology, rather than as a limitation or requirement. And we need to build technology without overshadowing or upending what it means to be human. It’s a concept we call “positive engineering.” Over the last few years, ethical questions have entered the technology domain from a number of different directions. Inclusivity, accessibility, sustainability, job security, protection of creative intellectual property, and so much more. Each of them roots back to one single question: how do we balance what we can achieve with technology with what we want as people?
This is a transformative moment for technology and people alike, and the world is ready for you to help shape it.
Paul Daugherty
Chief Technology & Innovation Officer
Adam Burden
Global Innovation Lead
Suggestions or feedback?
MIT News | Massachusetts Institute of Technology
- Machine learning
- Sustainability
- Black holes
- Classes and programs
Departments
- Aeronautics and Astronautics
- Brain and Cognitive Sciences
- Architecture
- Political Science
- Mechanical Engineering
Centers, Labs, & Programs
- Abdul Latif Jameel Poverty Action Lab (J-PAL)
- Picower Institute for Learning and Memory
- Lincoln Laboratory
- School of Architecture + Planning
- School of Engineering
- School of Humanities, Arts, and Social Sciences
- Sloan School of Management
- School of Science
- MIT Schwarzman College of Computing
A comprehensive study of technological change
Press contact :.
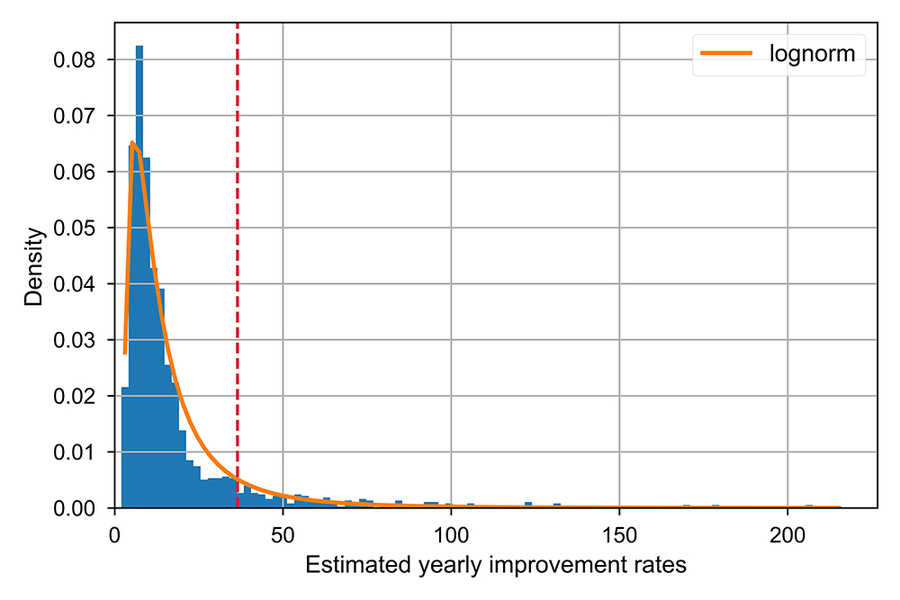
Previous image Next image
The societal impacts of technological change can be seen in many domains, from messenger RNA vaccines and automation to drones and climate change. The pace of that technological change can affect its impact, and how quickly a technology improves in performance can be an indicator of its future importance. For decision-makers like investors, entrepreneurs, and policymakers, predicting which technologies are fast improving (and which are overhyped) can mean the difference between success and failure.
New research from MIT aims to assist in the prediction of technology performance improvement using U.S. patents as a dataset. The study describes 97 percent of the U.S. patent system as a set of 1,757 discrete technology domains, and quantitatively assesses each domain for its improvement potential.
“The rate of improvement can only be empirically estimated when substantial performance measurements are made over long time periods,” says Anuraag Singh SM ’20, lead author of the paper. “In some large technological fields, including software and clinical medicine, such measures have rarely, if ever, been made.”
A previous MIT study provided empirical measures for 30 technological domains, but the patent sets identified for those technologies cover less than 15 percent of the patents in the U.S. patent system. The major purpose of this new study is to provide predictions of the performance improvement rates for the thousands of domains not accessed by empirical measurement. To accomplish this, the researchers developed a method using a new probability-based algorithm, machine learning, natural language processing, and patent network analytics.
Overlap and centrality
A technology domain, as the researchers define it, consists of sets of artifacts fulfilling a specific function using a specific branch of scientific knowledge. To find the patents that best represent a domain, the team built on previous research conducted by co-author Chris Magee, a professor of the practice of engineering systems within the Institute for Data, Systems, and Society (IDSS). Magee and his colleagues found that by looking for patent overlap between the U.S. and international patent-classification systems, they could quickly identify patents that best represent a technology. The researchers ultimately created a correspondence of all patents within the U.S. patent system to a set of 1,757 technology domains.
To estimate performance improvement, Singh employed a method refined by co-authors Magee and Giorgio Triulzi, a researcher with the Sociotechnical Systems Research Center (SSRC) within IDSS and an assistant professor at Universidad de los Andes in Colombia. Their method is based on the average “centrality” of patents in the patent citation network. Centrality refers to multiple criteria for determining the ranking or importance of nodes within a network.
“Our method provides predictions of performance improvement rates for nearly all definable technologies for the first time,” says Singh.
Those rates vary — from a low of 2 percent per year for the “Mechanical skin treatment — Hair removal and wrinkles” domain to a high of 216 percent per year for the “Dynamic information exchange and support systems integrating multiple channels” domain. The researchers found that most technologies improve slowly; more than 80 percent of technologies improve at less than 25 percent per year. Notably, the number of patents in a technological area was not a strong indicator of a higher improvement rate.
“Fast-improving domains are concentrated in a few technological areas,” says Magee. “The domains that show improvement rates greater than the predicted rate for integrated chips — 42 percent, from Moore’s law — are predominantly based upon software and algorithms.”
TechNext Inc.
The researchers built an online interactive system where domains corresponding to technology-related keywords can be found along with their improvement rates. Users can input a keyword describing a technology and the system returns a prediction of improvement for the technological domain, an automated measure of the quality of the match between the keyword and the domain, and patent sets so that the reader can judge the semantic quality of the match.
Moving forward, the researchers have founded a new MIT spinoff called TechNext Inc. to further refine this technology and use it to help leaders make better decisions, from budgets to investment priorities to technology policy. Like any inventors, Magee and his colleagues want to protect their intellectual property rights. To that end, they have applied for a patent for their novel system and its unique methodology.
“Technologies that improve faster win the market,” says Singh. “Our search system enables technology managers, investors, policymakers, and entrepreneurs to quickly look up predictions of improvement rates for specific technologies.”
Adds Magee: “Our goal is to bring greater accuracy, precision, and repeatability to the as-yet fuzzy art of technology forecasting.”
Share this news article on:
Related links.
- Sociotechnical Systems Research Center
- Institute for Data, Systems, and Society (IDSS)
Related Topics
- Technology and society
- Innovation and Entrepreneurship (I&E)
Related Articles
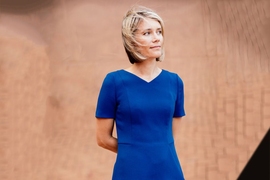
Shaping technology’s future
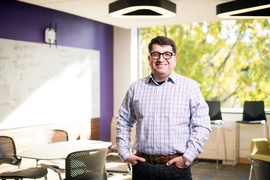
Tackling society’s big problems with systems theory
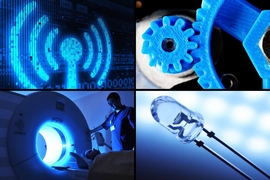
Patents forecast technological change
Previous item Next item
More MIT News
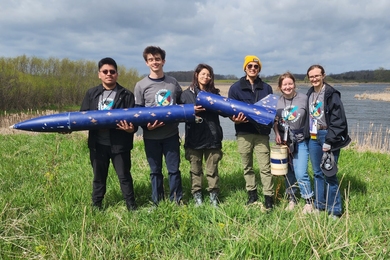
MIT team wins grand prize at NASA’s First Nations Launch High-Power Rocket Competition
Read full story →
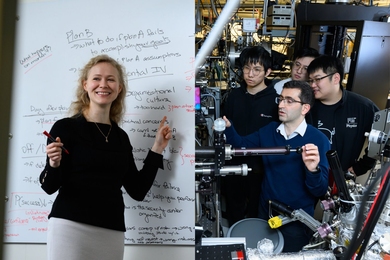
Nurturing success
For developing designers, there’s magic in 2.737 (Mechatronics)
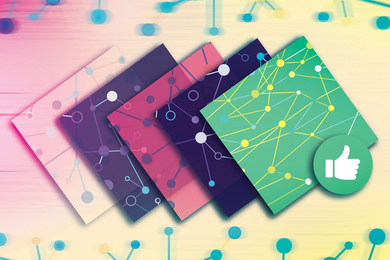
Study: Transparency is often lacking in datasets used to train large language models
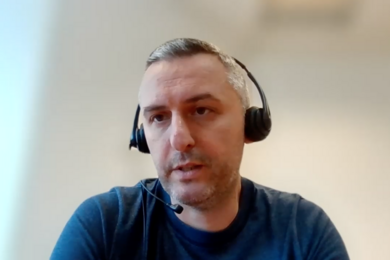
How MIT’s online resources provide a “highly motivating, even transformative experience”
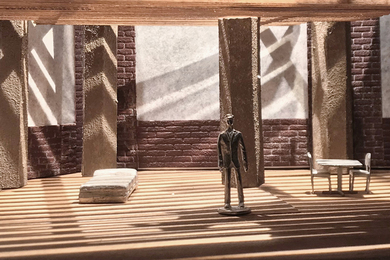
Students learn theater design through the power of play
- More news on MIT News homepage →
Massachusetts Institute of Technology 77 Massachusetts Avenue, Cambridge, MA, USA
- Map (opens in new window)
- Events (opens in new window)
- People (opens in new window)
- Careers (opens in new window)
- Accessibility
- Social Media Hub
- MIT on Facebook
- MIT on YouTube
- MIT on Instagram

Our Insights | Your Vision
With unmatched insights on countless markets, our industry research will help you take your business to new heights..
Actionable Insights
Strategic thinking
Ask Us Anything
No matter the hour, we’re here to help.
Cookie Policy
The Site uses cookies to record users' preferences in relation to the functionality of accessibility. We, our Affiliates, and our Vendors may store and access cookies on a device, and process personal data including unique identifiers sent by a device, to personalise content, tailor, and report on advertising and to analyse our traffic. By clicking “I’m fine with this”, you are allowing the use of these cookies. You may change your settings based on a legitimate interest at any time, by selecting “Manage Settings” on our site. Please refer to the help guide of your browser for further information on cookies, including how to disable them. Review our Privacy & Cookie Notice.
Manage Settings
When you visit any website, it may store or retrieve information on your browser, mostly in the form of cookies. This information might be about you, your preferences, or your device and is mostly used to make the site work as you expect it to.
The information does not usually directly identify you, but it can give you a more personalised web experience. Because we respect your right to privacy. Click on the different category headings to find out more. These cookies will be stored in your browser only with your consent. Please read our Privacy & Cookie Notice. before managing your preferences.
Necessary Always Enabled
These cookies are necessary for the website to function and cannot be switched off in our systems. They are usually only set in response to actions made by you which amount to a request for services, such as setting your privacy preferences, logging in or filling in forms. These cookies are essential in order to enable You to move around the site and use its features, such as accessing secure areas of the site. These cookies do not store any personally identifiable information.
These cookies enable the website to provide enhanced functionality and personalisation. They may be set by us or by third party providers like Freshdesk, Piwik whose services we have added to our pages. These cookies cannot track your browsing activity on other websites. All information these cookies collect is anonymous and is only used to improve how this website works. If you do not allow these cookies we will not know when you have visited our site and will not be able to monitor its performance.
This website uses Google Analytics, a web analytics service provided by Google, Inc. (Google). These cookies are used to calculate visitor, session, and campaign data, and to keep track of site usage for this site’s analytics report. These cookies store the behaviour of each user’s information such as your login information, what pages you visit, how long you stayed on the page, the device you were using, your time zone, what language you’re using, where you’re logging in from, and the operating system of the device you are on. Such information is necessary for a website’s digital marketing efforts, increasing the chances of campaign success by tailoring content to the target audience.
Numbers, Facts and Trends Shaping Your World
Read our research on:
Full Topic List
Regions & Countries
Publications
- Our Methods
- Short Reads
- Tools & Resources
Read Our Research On:
Internet & Technology
Why many parents and teens think it’s harder being a teen today.
Is it harder being a teen today? Or do they have it easier than those of past generations? We asked parents and teens who say being a teenager has gotten harder or easier to explain in their own words why they think so.
Many Israelis say social media content about the Israel-Hamas war should be censored
About half of tiktok users under 30 say they use it to keep up with politics, news, 72% of u.s. high school teachers say cellphone distraction is a major problem in the classroom, sign up for our internet, science, and tech newsletter.
New findings, delivered monthly
Most Israeli adults do not post or share about political and social issues online – including the war between Israel and Hamas.
TikTok users under 30 see its impact on democracy more positively than older users, with 45% of this group saying it’s mostly good for American democracy.
Some 72% of high school teachers say that students being distracted by cellphones is a major problem in their classroom.
How Americans Navigate Politics on TikTok, X, Facebook and Instagram
X stands out as a place people go to keep up with politics. Still, some users see political posts on Facebook, TikTok and Instagram, too.
How Americans Get News on TikTok, X, Facebook and Instagram
X is still more of a news destination than these other platforms, but the vast majority of users on all four see news-related content.
Electric Vehicle Charging Infrastructure in the U.S.
64% of Americans live within 2 miles of a public electric vehicle charging station, and those who live closest to chargers view EVs more positively.
When Online Content Disappears
A quarter of all webpages that existed at one point between 2013 and 2023 are no longer accessible.
A quarter of U.S. teachers say AI tools do more harm than good in K-12 education
High school teachers are more likely than elementary and middle school teachers to hold negative views about AI tools in education.
Teens and Video Games Today
85% of U.S. teens say they play video games. They see both positive and negative sides, from making friends to harassment and sleep loss.

REFINE YOUR SELECTION
Research teams, signature reports.
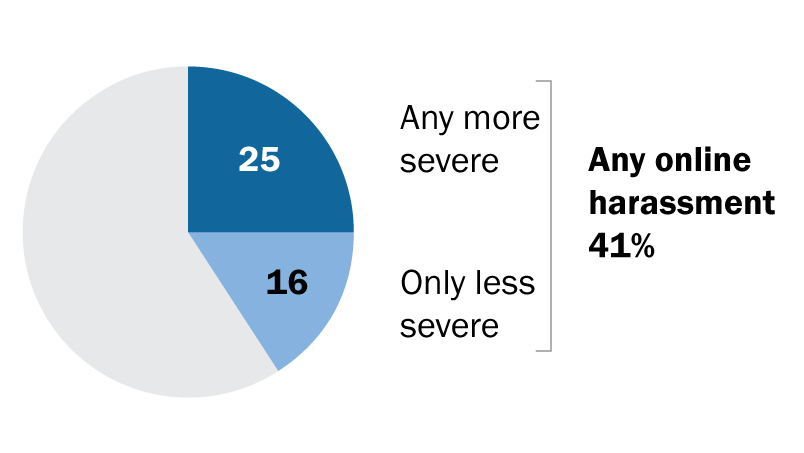
The State of Online Harassment
Roughly four-in-ten Americans have experienced online harassment, with half of this group citing politics as the reason they think they were targeted. Growing shares face more severe online abuse such as sexual harassment or stalking
Parenting Children in the Age of Screens
Two-thirds of parents in the U.S. say parenting is harder today than it was 20 years ago, with many citing technologies – like social media or smartphones – as a reason.
Dating and Relationships in the Digital Age
From distractions to jealousy, how Americans navigate cellphones and social media in their romantic relationships.
Americans and Privacy: Concerned, Confused and Feeling Lack of Control Over Their Personal Information
Majorities of U.S. adults believe their personal data is less secure now, that data collection poses more risks than benefits, and that it is not possible to go through daily life without being tracked.
Americans and ‘Cancel Culture’: Where Some See Calls for Accountability, Others See Censorship, Punishment
Social media fact sheet, digital knowledge quiz, video: how do americans define online harassment.
901 E St. NW, Suite 300 Washington, DC 20004 USA (+1) 202-419-4300 | Main (+1) 202-857-8562 | Fax (+1) 202-419-4372 | Media Inquiries
Research Topics
- Email Newsletters
ABOUT PEW RESEARCH CENTER Pew Research Center is a nonpartisan fact tank that informs the public about the issues, attitudes and trends shaping the world. It conducts public opinion polling, demographic research, media content analysis and other empirical social science research. Pew Research Center does not take policy positions. It is a subsidiary of The Pew Charitable Trusts .
© 2024 Pew Research Center
Technology Research Paper
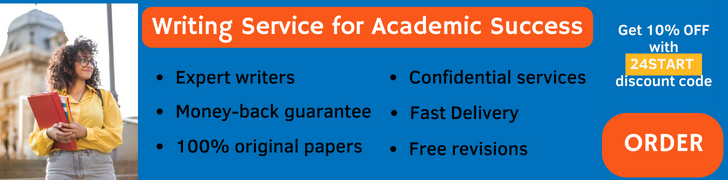
This sample technology research paper features: 8300 words (approx. 27 pages), an outline, and a bibliography with 48 sources. Browse other research paper examples for more inspiration. If you need a thorough research paper written according to all the academic standards, you can always turn to our experienced writers for help. This is how your paper can get an A! Feel free to contact our writing service for professional assistance. We offer high-quality assignments for reasonable rates.
Introduction
Man’s relation to technology: a brief history, technology and biological anthropology, the sts approach, classical philosophical anthropology, philosophy of technology, the continental approach to the philosophy of technology, the analytic approach to the philosophy of technology, recent developments: bridging the gap, conclusion and future directions.
- Bibliography
More Technology Research Papers:
- History of Technology Research Paper
- Internet Research Paper
- Nanotechnology Research Paper
- Compstat Research Paper
- Computer Forensics Research Paper
- Healthcare Technology Assessment Research Paper
- Ethics of Information Technology Research Paper
- Neurotechnology Research Paper
The term technology is derived from the Greek word techné. The Greek word refers to all forms of skillful, rule-based mastery in any field of human praxis, originally encompassing both arts (like painting, sculpture, writing, and the like) and craftsmanship (like carpentry, shipbuilding, architecture, and the like). The Roman culture uses the Latin word arts for these domains. Accordingly the medieval terminology distinguishes between the seven free arts (grammar, rhetoric, logic, geometry, arithmetic, music, astronomy) and the mechanical arts (e.g., agriculture, architecture, tailoring), thus prefiguring the later distinction between arts (as linked to the study of humans and the humanities) and technology (as linked to engineering and the study and science of nature).
Academic Writing, Editing, Proofreading, And Problem Solving Services
Get 10% off with 24start discount code.
The modern word technology finally refers either to procedures and skillful application of sciences for the production of industrial or manual products or to the products of these processes themselves. In this sense, technology nowadays encompasses only a part of the original Greek definition. The place of technology as being on the one hand a product of humans (being thus rooted in human anthropology and human tool usage), and being on the other hand based on a solid scientific understanding of the laws of nature (modern technology), can be seen as the two key features of contemporary and recent approaches to analyze and understand technology. Technology is then in one respect as old as humankind: Many approaches in anthropology thus refer to the general structure of technology in all of human history and relate it to the biological condition of humans. But recent anthropological thinking also reflects on the specific details of modern technology. It has often been argued that there is a structural difference between modern, science-based technology and older forms of craftsmanship of ancient or medieval types of technology. Therefore, a central question for modern anthropology is to analyze the consequences modern technology has for our picture of humankind: how to define man in the age of technology.
Reflection about the anthropological function of technology is probably as old as human self-reflection itself, since the ability to use tools and create cultural products has always been seen as a unique human feature, distinguishing humankind from most other animals (see also the next section on biological anthropology). But an analysis of technology was not at the center of political, social, anthropological, or philosophical thoughts before the development of the modern natural sciences and their counterpart, modern technology. Following Carl Mitcham (1994) one can roughly distinguish three approaches to technology before the 20th century, encompassing many topics that later became essential parts of contemporary discussions about technology (p. 275). The three approaches are as follows:
- In the ancient world, technology is looked at with certain skepticism. The use of tools is seen as necessary for survival, but also regarded as dangerous, since it might lead to human hubris and might raise the envy and anger of the gods. In this sense, mythological thinking envisions technology as, for example, stolen from the gods (the myth of Prometheus), and thus not properly belonging to humans. The extensive use of technology is often seen as leading to megalomaniac fantasies or unjustified overstepping of religious and ethical boundaries (e.g., myth of the Tower of Babel, myth of Icarus). Philosophical reflection, however, acknowledges the value of technology for an otherwise defenseless human being. Already Plato anticipates a central thought of modern anthropology: Human beings are poorly equipped for survival in nature. They need to compensate for this lack by developing skills of rational thinking and the usage of tools (this idea later becomes a central thesis of the famous anthropology of Arnold Gehlen [1988]). But the emphasis in ancient philosophical anthropology lies not so much on man’s capacities to invent technology, but on man’s moral character (exemplified by ancient wisdom or medieval religiosity). The usage of technical knowledge should thus be kept within strict ethical boundaries.
- In the hierarchy of knowledge, ethical wisdom is regarded in principle as higher than and superior to technological skills. Socrates points to the question that we should not only seek knowledge about how to do certain things (technical knowledge), but rather about whether we should perform certain actions (ethical knowledge); this idea can also be found in the medieval distinction between the (superior form of a) life in contemplation ( vita contemplativa ) and the (lower) life in active involvement ( vita activa ). Ancient and medieval technology is thus embedded in an anthropological vision, in which human virtues play an important role. Different forms of virtues are combined in the original crafts, as opposed to the later, modern differentiation of these virtues: In craftmanship one can find a union of economical virtues (e.g., efficient usage of limited resources), technical virtues (creating new entities that did not exist before), and often also aesthetic virtues (a sense of beauty that adds an aesthetic component to these newly created entities going beyond the modern idea that “form follows function”). In the Greek world, these three skills are combined in the realm of poiesis, while in modernity they are separated in the three domains of economy, technology, and art—each relatively independent of the others (Hösle, 2004, p. 366).
- A profound change in the evaluation of technology emerges with modernity, a position that Mitcham (1994) summarizes as Enlightenment optimism. Already in the writings of Francis Bacon (1620), the new science of nature and its application to experimental and technological research is highly welcomed. Progress in technology is seen as very beneficial to humankind, as it may lead to the cure of diseases, mastery over nature, and a constant progress toward a more human society. Many utopian writings mark the beginning of early modern thoughts in which technology is seen as essential in leading to a brighter future for humankind (e.g., Thomas More’s Utopia [1516], J. V. Andreae’s Christianopolis [1619], F. Bacon’s New Atlantis [1627]). In a similar line of thought, Enlightenment thinkers defend science and modern technology against attacks from religious conservatism, pointing at the beneficial consequences of technological and scientific progress.
- A countermovement to the Enlightenment is Romanticism, which accordingly has a different view on technology, referred to by Mitcham (1994) as Romantic uneasiness. Again, the central thought is an anthropological perspective in which man is seen as being good by nature, while it is civilization that poses the danger of alienating man from nature and from his fellow man, focusing only on his rational capacities and suppressing his emotional and social skills. Already Vico (1709) opposed Cartesian rationalism and feared that the new interest in science would lead to a neglect of traditional humanistic education. Rousseau’s critique of modern societies then became influential, seeing an advancement of knowledge and science, but a decay of virtues and immediacy ( Discourse on the Arts and Sciences; Rousseau, 1750). With the age of industrialism, the negative social consequences of modern labor work become the scope of interest of social theorists, leading up to Marx’s famous analysis of modern societies (see subsequent section on cultural and sociological anthropology). In opposition to the positive utopias centered on technology in early modernity, the 20th century then sees the literary success of pessimistic dystopias, in which often technological means of suppression or control play an important role (e.g., already in M. W. Schelley’s Frankenstein or the Modern Prometheus [1818] and later in H. G. Wells’s The Island of Doctor Moreau [1896], A. Huxley’s Brave New World [1932], George Orwell’s 1984 [1948], and Ray Bradbury’s Fahrenheit 451 [1953]).
The tension between approaches praising the benefits of technology (in the spirit of the Enlightenment) and approaches focusing on negative consequences (in the spirit of Romanticism) still forms the background of most of the contemporary philosophical and anthropological debate; this debate circles around an understanding of modern technology, often rooted in the different “cultures” of the humanities and the sciences. It can be regarded as being a particularly vivid opposition at the beginning of the 20th century, that only later gave room for more detailed and balanced accounts of technology (some classics of the debate being Snow, 1959; McDermott, 1969).
Recent contributions toward a deeper understanding of the usage and development of technology stem from such different disciplines as biology, sociology, philosophical anthropology, metaphysics, ethics, theory of science, and religious worldviews. This research paper aims at a brief overview of important topics in the debate over technology during the 20th century to the present time. Three anthropological perspectives will be distinguished, depending on the main focus of anthropological interest. This will start with a brief summary of the biological anthropological perspective on technology, move on to those theories which focus more on social or cultural aspects, and conclude with more general philosophical anthropologies. This research paper is thus not chronologically organized, but tries to identify common themes of the debate, even though sometimes the topics might overlap (e.g., the case of Gehlen, a philosophical anthropologist who starts from a biological perspective and then moves on toward a more social view on technology).
In contemporary anthropology, technology becomes a central issue for at least two different reasons:
- From a biological perspective the usage of tools is regarded (next to the development of language and a cognitive rational apparatus) as one of the key features of humanization. Biological anthropology thus initially focuses on the differences and similarities of tool usage in humans and animals, trying to understand the role technology plays in general for an understanding of humans’ biological and social nature. With the focus on human evolution, attention is often drawn to the question of which role technology played at the beginning of humankind.
- While in this way always being a part of human culture, technology becomes arguably one of the single most influential key features of society only in modernity. According to Max Weber, science, technology, and economy form the “superstructure” of modernity, while they all share a common “rationality” (mainly of means-ends reasoning in economy and technology). The experience of the powers and dangers of modern technology (as in industrialized labor work, medical progress, nuclear energy and weapon technology, environmental problems due to pollution, and extensive usage of resources, etc.) has triggered many social, political, and philosophical reflections that—in opposition to biological anthropology—aim primarily at understanding the specifics of modern
Let us look at these two tendencies in turn, starting with the biological perspective, before moving to the social or cultural anthropology of technology.
Biological anthropologists are interested in the role technology played during humanization, and they attempt to give evolutionary accounts of the development of tool usage and technology and compare tool usage in man with tool usage in other animals. The development of technology has often been regarded as an evolutionarily necessary form of adaption or compensation. Since most of man’s organs are less developed than those of other species, he needed to compensate for this disadvantage in the evolutionary struggle for life (see Gehlen, 1980). Initially the usage of tools was considered a unique human feature, distinguishing the genus Homo from other animals (Oakley, 1957), but research on tool usage in different animals, especially chimpanzees, led to a more or less complete revision of this thesis (Schaik, Deaner, & Merrill, 1999).
Nowadays, many examples of tool usage in the animal kingdom are known (Beck, 1980). For example, chimpanzees use sticks to fish for termites, and elephants have been described as having a remarkable capacity for tool usage. Even though tool usage must thus be regarded as more common among animals, attention still needs to be drawn to the specifics of man’s tool usage, which arguably in scope and quality goes beyond what is known from the animal kingdom. It has been pointed out that our biological anatomy offers us several advantages for an extended usage of tools: walking erectly frees the two hands, which can then be used for other purposes. Furthermore, the position of the human thumb and short straight finger are of great benefit, especially in making and using stone tools (Ambrose, 2001). Still debated, however, is whether social and technological developments go hand in hand or whether one of the two factors is prior.
Even though many anthropologists tended to see social behaviors and cultural revolutions mostly as a consequence of a change in tool usage or a development of new technologies, it has also occasionally been argued that the development of social skills precedes the development of technical skills (e.g., in joint group hunting). It has additionally been acknowledged that chimpanzees also pass over some of their technical knowledge through the mechanism of learning and establishing cultural “traditions” that resemble, to some extent, human traditions (Wrangham, 1994; Laland, 2009). But there seems to be a specific difference in human and primate learning, namely in the fact that human children learn tool usage mainly via imitation and by simply copying a shown behavior, even if it is not the most efficient solution to a given problem. Opposed to this, chimpanzees seem to learn through a process called emulation, which implies that they diverge from the paradigmatic solution that has been “taught” to them. It has been argued that learning through imitation has been selected in humans, even though it is a less flexible strategy, because it is a more social strategy of learning (Tomasello, 1999, p. 28). In this way, biological anthropology mirrors a debate in social anthropology about the role of technology; this can be seen either as a driving force born out of necessity that calls for social changes (technical determinism), or as highly mediated or even constructed by culture (social constructivism).
Technology and Social/Cultural Anthropology
As already mentioned, technology was identified early on as a key feature of modern society (Misa, Brey, & Feenberg, 2004). Many studies have been written about the impact of modern technology on society, focusing mainly on the industrial revolution (e.g., Haferkamp, 1992; Pressnell, 1960; Smelser, 1969) or on the more recent revolution of the information society (e.g., Castells, 1999; Nora, 1980), as well as on the impact of technological change on traditional societies.
The analyses of Karl Marx and the Frankfurt School are influential, not only in trying to grasp the role of modern technology in society, but also in hinting on potential anthropological roots of technology and their essential interrelation with social aspects of the human condition. Marx insisted that the study of technology holds the highest relevance for human sciences, since it reveals the way humans deal with nature and sustain life (Marx, 1938). An essential feature of man’s nature is that he has to work in order to sustain his life, that he is the “toolmaking animal” or—as he has later been called—the Homo faber. Marx analyzes the role of technology in Chapter 13 of his first volume of Das Kapital. He argues that the division of labor becomes fostered through machines, which at the same time replace more and more traditional manpower and can furthermore be operated by less skilled employees, thus leading to very bad labor conditions for the working class. Technology in general is, however, still greeted as an option to make humans’ lives easier; it is mainly the social distribution of the possession of the means of production that Marx regards as problematic. (Also later thinkers, inspired by Marxian thought, tend to see technology as an important means toward establishing a better future.) On the other hand, at the same time, technology is seen as rooted in man’s will to dominate nature.
Following this later insight in particular, Theodor Adorno argues that Western civilization has developed powerful tools to ensure its self-preservation against nature. Technical rationality is regarded as the exercise of strategic power to dominate (external) nature, but it is at the same time also leading to a suppression of the inner nature of man (Adorno, 1979). The main strategy of this rationality is quantification, which lies at the heart of the mathematical-scientific interpretation of nature and the development of modern technology. At the same time it brings forth a type of rationality, which leads to a selfmutilation. The will to exercise power becomes the main feature of modern rationality, thus leading to a dialectic that turns the noble aims of the Age of Enlightenment into a morality of humankind that is its very opposite: A new barbaric system of oppression and dictatorship arises, using technology for totalitarian purposes.
While Adorno seeks redemption mainly in the arts (Adorno, 1999), seeming to promise the possibility of a completely different kind of subjectivity, Jürgen Habermas (1971) tries to propose an antidote; this does not lie outside of modern-Enlightenment rationality, but rather returns to its original intention. Habermas argues with Marx and Adorno, asserting that technological knowledge has its anthropological roots in the will to dominate nature and therefore serves a strategic interest of man. With this, man is not only Homo faber but also a social animal. Besides the strategic means-end rationality he also possesses a communicative rationality, aimed at defining common moral values and engaging in discourse over ethically acceptable principles of actions. In thus distinguishing two types of rationality, Habermas tries to incorporate much of the German tradition of cognitivistic ethics into his approach. It is important for Habermas that technology be brought under the control of democratic decision-making processes; his discourse ethics has thus helped to inspire ideas of participatory technology assessment.
Outside the Frankfurt School, technology has not been at the center of social and cultural anthropology, as has been often complained (Pfaffenberger, 1988, 1992). Langdon Winner (1986) coined the term technological somnambulism to refer to those theories that neglect the social dimension of technology. According to this dominant tradition, the human-technology relation is “too obvious” to merit serious reflection. Technology is seen as an independent factor of the material and social world, one that forms a relatively autonomous realm of ethically neutral tools to acquire human ends. But already Winner argues that technology is essentially social and is shaped by cultural conditions and underlying value decisions. He claims in a famous article (Winner, 1980) that Long Island’s low bridges were intentionally built in a way that would keep buses away, making it more difficult for the poor, and mainly the black population, to reach the island. Even though this particular claim has been challenged, Winner seems to be correct in pointing out that value decisions play a role in creating technology, and that the social value system leaves its trace in technological artifacts.
In line with this renewed interest in social issues, a new field of studies related to technology emerged in the 1980s, focusing explicitly on this neglected relation between society and technology: the so-called STS approach. Having been labeled the “turn to technology” (Woolgar, 1991), science and technology studies (STS) analyzes society’s impact on science and technology, and science and technology’s impact on society. Several writers draw attention to the social shaping of technology. An influential author is Bruno Latour, who contributed to both the initial appeal to social constructivism (that he later gave up) and the development of the actor-network theory; both are at the center of the debate about the theoretical underpinnings of STS.
Social Constructivism
Woolgar and Latour employ a social-constructivist perspective in their early case study on the production of scientific results, in which they analyze scientists’ attempt to establish and accumulate recognition and credibility of their research through the “cycle of credibility” (Latour, 1979). The main idea of social constructivism is the attempt to interpret alleged objective “facts” in the social world as being socially constructed, so that knowledge of the world and its interpretation depends on social mechanisms and cannot be traced back to objective facts (Berger & Luckmann, 1966). In this sense technology is also not an objective, independent given, but shaped by social ideas and societal interpretations.
Actor-Network Theory
In the 1980s and 1990s, Latour became one of the main proponents of the actor-network theory (Latour, 2005); this is also attractive to scholars who reject social constructivism, since it can be combined with the idea that not all of technology is socially constructed. The social-constructive interpretation of this theory aims to develop a framework in which society and nature, or society and technology, are not separated. The idea of technology as a sociotechnical system implies that agent and tool form a unity, which cannot be explained completely by referring to one of the two elements in isolation. According to this idea, technological artifacts dispose over some form of agency and can be—to some extent—regarded as actants. This ascription of intentionality and agency to technical systems is, however, highly debated. The debate between realism and social constructivism has thus not been settled.
Philosophical Anthropology and the Philosophy of Technology
Research in philosophical anthropology peaked in early 20th-century Germany, discussed in the next section. But outside of anthropological discussions, the topic of technology became an important issue for philosophy, so in this brief overview, important contributions and themes of the continental and analytic tradition will be discussed next. Finally, more recent developments and topics in the philosophy of technology will be sketched that do not try to revitalize a philosophical anthropology, but that nevertheless do touch in one way or another on anthropological perspectives on technology.
Classical philosophical anthropology was mainly interested in understanding the essence of human nature and often draws specific attention to the role of technology. Important contributions came from Gehlen, Plessner, and Scheler during the first half of the 20th century. The attempt to link technology to a biological interpretation of man in Gehlen’s early works especially deserves attention. Given his biological constitution, man must be seen as deficient by nature ( Mängelwesen ), since he is not endowed with instinctive routines and is not adapted well to a specific natural environment, but rather is open to the world ( weltoffen ). He compensates for this deficiency with the help of his mental capacities and tool usage. Gehlen interprets human language and human institutions as relief mechanisms ( Entlastungen ) that help him to interpret and organize the plentitude of impressions (the sensory overload, Reizüberflutung ) that he is exposed to. Most technologies can thus be regarded to be either organ-amplification ( Organverstärkung ) or organ-replacement ( Organersatz ) (Gehlen, 1988). In Man in the Age of Technology (1980), Gehlen focuses more on sociological perspectives of technology. He identifies two essential cultural breaks marking principle changes in humans’ world interpretation and social organization, both of which are linked to technological developments: (1) the neolithic revolution of sedentism, marking the passage from a hunter’s culture to a society of agriculture and cattle breeding, and (2) the industrial revolution in modernity (Gehlen, 1980).
Scheler also analyzes man’s rational capacities from a biological perspective, but he concludes that a purely naturalistic approach does not render justice to our selfunderstanding. The human ways of sustaining life are from an often inefficient biological perspective. Therefore, it must be pointed out that the main function of human knowledge is not only to strategically ensure humans’ own survival, but also to be directed toward the discovery of moral values and toward the process of self-education ( Bildung ). Humans not only live in an environment, but also reflect on their place in the world—a capacity that marks a fundamental difference between humans and animals (Scheler, 1961).
This type of philosophical anthropology came to a certain end when the main interest of philosophers shifted from understanding “man” to understanding “society” during the 1960s. With the recent developments of sociobiology, philosophers have taken a renewed interest in the linkage between biological and cultural interpretations of man. Let us look at some tendencies of later research in the philosophy of technology.
If we look at a philosophical interpretation of technology, we find the first origins of a discipline of the philosophy of technology by the end of the 19th and the beginning of the 20th century (see Kapp, 1877, and Dessauer, 1933). During the first half of the 20th century, the philosophical analysis of technology can, roughly speaking, be divided into two main schools of thought: the continental, often skeptical approach, and the analytical, often optimistic approach . As with all such very generic typologies, this distinction likewise does not claim to be more than an approximation, while the general tendency of recent research seems precisely to be to overcome this gap and to aim for a convergence or crossfertilization of these two approaches. Therefore, what follows is an ideal-type distinction that tries to make some of the basic ideas of these two approaches more visible and aims at understanding their more general features.
The continental approach originally focused on a humanities-centered perspective on technology, its (mainly negative) consequences for society, and its rootedness in a problematic feature of human anthropology (the will to power), and finally tried to understand technology as such (its “essence”). The analytic approach, on the other hand, originally focused on a more science-based understanding of technology, its (mostly beneficial) potential for the progress of societies, and its rootedness in a rational (scientific) way to approach nature, and it finally tried to look not at technology as such but at specific problems or specific types of technologies.
In the continental philosophy of technology, technology is often interpreted as closely linked to a certain form of consciousness, a form of approaching nature (and also human interaction) from a perspective that is rooted in a scientific understanding of the world, which itself is rooted in the will to dominate nature. This approach is seen to replace or at least to endanger a value-based approach to reality. In this sense, Edmund Husserl’s phenomenology regards science and technology as a mere abstraction from the fullfledged real experience of the world we live in. In this way, the sphere of technical knowledge is limited and needs to be guided by value decisions, which do not have their basis in scientific or technical knowledge, but stem from our ethical knowledge of our life-world.
While technology is not at the center of Husserl’s interest, José Ortega y Gasset (1914/1961) was one of the first philosophers who aimed at a deeper understanding of the relation between human nature and technology. Rejecting Husserl’s later emphasis on the transcendental subject, he insists that human nature can only be understood by the formula “I am I plus my circumstances.” Philosophy can thus neither start from the isolated subject (as in idealism), nor can it interpret everything from the perspective of the material conditions (as in materialism). Rather, it must find a middle ground. The essence of humans is for Ortega not determined by nature; this distinguishes humans from plants or animals or from physical objects—all having a defined, specific given nature. Man must determine his own nature by himself by way of the creative imagination. Technology is interpreted as the material realization of this self-image; it is a projection of an inner invention into nature. According to Ortega, technology evolved in three phases: It started as a collection of accidental findings of means toward ends by pure chance. In a later state, these findings became traditions and skills that were passed on to the next generation. Modern technology marks a radical difference, since it is based on a systematic scientific approach, which forms the third phase. This approach, however, tends to become the dominant mode of thinking, so that man’s creative capacity for imagination (which is at the heart of man’s very essence) is in danger of being replaced or losing its importance (Ortega y Gasset, 1914/1961).
Martin Heidegger’s (1977) analysis of technology in his essay “The Question Concerning Technology” is also very influential. His philosophy aims at understanding the notion of being, which—so claims Heidegger—has been misinterpreted or neglected by traditional European philosophy. Since man is the only known being that can ask for the meaning of being, Heidegger’s analysis in Sein und Zeit starts from an interpretation of the existence of such a being ( Da-sein ). Even though his book is meant to be an exercise in philosophical (fundamental) ontology, it offers many anthropological insights about the specific human form of existence, in which the knowledge and the denial of one’s own mortality form essential human features.
In his later work, Heidegger (1977) understands technology as a specific form of disclosing reality. Asked for the essence of technology, people usually refer to it as a means to achieve an end (instrumental definition), or they define technology as an essential human activity (anthropological definition). Even though Heidegger admits that these definitions are “correct,” they do not disclose the essential truth about technology for two reasons. Essentially, (1) technology is not a tool for achieving an end, but rather the perspective under which everything that exists is seen only as a potential resource to achieve an (external) end. Furthermore, (2) this disclosure of reality is not a human-directed practice: Humans are driven objects rather than being themselves the active subjects. According to these conclusions, the instrumental and the anthropological definitions of technology do not capture the whole truth of technology. Let us look at these two points in turn, as follows:
- The essence of technology lies, according to Heidegger, in its capacity to disclose reality ( entbergen ) under a very specific, limited perspective. This perspective reduces everything to a potential object for manipulation, a resource ( Bestand ) for further activity. Technology is thus a way to disclose something hidden. Following his analysis of the Greek word for truth ( aletheia ) as referring to something undisclosed, he sees thus a “truth” at work, under which reality presents itself as a mere collection of resources for external purposes, rid of all inner logic and teleology that was so prominent in traditional understandings of nature. Heidegger points at the different ways in which a river is seen by a poet in an artwork ( Kunst werk), on the one hand, and, on the other hand, in which the same river is seen by an engineer as a potential resource for energy generation in a power plant ( Kraft werk).
- Heidegger then goes on to claim that opposed to the image of man being in control of technology and using it for his purposes, he should rather be seen as being provoked ( herausgefordert ) by this coming to pass. Heidegger clearly wants to reject the optimistic idea of “man being in control” through the help of modern technology and, rather, revert it to its opposite: man being driven by a force greater than himself. He calls this driving force the essence of technology, the en-framing ( Ge-stell ) that prompts humans to look at nature under the idea of its usability. In doing so, man is in highest danger, but not because of potential hazards or specific negative consequence of modern technology. The danger is, rather, that he loses sight of understanding nature in a different way and that he might finally end up understanding also himself and other humans only as potential “resources” or potential material for manipulation and instrumentalization. Heidegger suspects that art might be a potential antidote to this development: In Greek, techne originally encompassed also the production of beautiful objects in art. Thus, a deeper understanding of technology might reveal its relation to art and might point to the fact that art offers a potential answer to the challenge that modern technology poses to human self-understanding.
Certainly, Heidegger’s contribution to the modern philosophy of technology lies more in highlighting this essential dimension of technology as a threat, rather than in elaborating strategies to counter these inherent dangers. Heidegger’s article is arguably the single most influential essay written in the philosophy of technology, although his mannered, often dark language allows for different interpretations and often lacks the clarity of philosophical contributions from the analytical school. But the idea that “technology” and technological rationality is a limited form of looking at reality—one that is in strong need of a countervision, and that might further lead to a deformation of intersubjective human relations and that finally affects human self-understanding—has ever since been a prominent topic in different thinkers from Adorno and Marcuse to Jürgen Habermas, as illustrated earlier. This idea has often been linked with an ethical concern: Modern technology calls for new ethical guidelines, and despite some beneficial consequence, poses a potential threat to human existence. Much of this ethical debate about modern technology was triggered by its potential to radically destroy human life, be it through nuclear, biological, or chemical weapons or by consequences of environmental pollution and climate change.
Heidegger’s pupil Hans Jonas (1984) was one of the first philosophers to emphasize the need for a specific “ethics for the age of technology,” feeling that modern technology urges us to radically reconsider our ethical intuitions in order to meet the new challenges. Nevertheless, based on humans’ anthropological need to seek protection against nature, classical technology never fully reached this aim. Nature remained always more powerful than men, and the consequences of human actions were mostly not far-reaching. Traditional ethics could therefore focus on the “near and dear.” Modern technology, however, radically changes the picture: Its scope is unknown in premodern times; its consequences and potential dangers could be fatal, far-reaching, and irreversible. Focusing on the environmental problems of modern societies with, as the darkest perspective, the possible extinction of humankind, Jonas suggests broadening the scope of our ethical obligations: If our actions are more far-reaching than ever before in the history of humankind, we need to acquire a new ethical countervision. Jonas finds this remedy in the anthropological feature of our feelings of responsibility. Responsibility often expresses an asymmetrical relation, as in parents who feel responsible to care for their children. The old ethical intuition to derive obligations from the rights of free and conscious individuals, able to participate in argumentation and democratic decisions, seems to be too narrow to account for most environmental problems: Future generations are not yet born, animals and nature cannot in the same sense be regarded as having rights, as has been established in previous ethical approaches to the idea of universal human rights. But obligations may also stem from the idea of responsibility, from the idea that something has been given into our care.
Analytic philosophy is rooted in the quest for clear conceptualization, sound argumentation, and scientific precision. For early analytical philosophy in the Vienna Circle, the mathematical nature of scientific knowledge could serve as a role model for knowledge as such: hence, the need for and the extended usage of logical formalization within analytic philosophy. Skeptical of the quest to address the essence of things like “the technology” in general, analytic philosophers very often focus on concrete problems linked to very specific technologies. Even though many thinkers in the line of logical positivism thus greeted scientific knowledge as the highest form of knowledge, this did not always lead to an unbalanced embrace of technology. In Bertrand Russell (1951), we find a skeptical attitude toward the social benefits of technology, especially if it is linked with totalitarian ideology. Thus, he stresses the importance of democratic education; if placed in a democratic context and applied in well-defined careful steps, technology is, however, beneficial for progress in a way in which Karl Popper (1957) typically advertises as piecemeal social engineering. Important early contributions to an analytic philosophy of technology stem further from Mario Bunge (1979), whose ideas closely link to the program of logical empiricism and oppose the “romantic wailings about the alleged evils of technology” (p. 68).
Even though this distinction between humanities’ philosophy of technology and engineering’s philosophy of technology (Mitcham, 1994) marks the background of the philosophical discussion on technology in the early 20th century, the debate soon moved beyond this opposition. Three tendencies seem to be of importance.
First, continental philosophy was moving away from the attempt to come up with metaphysical, religious, or anthropological answers to the big questions. With the emergence of postmodernism, the alleged end of the “big stories” was proclaimed, thus making a metaphysical approach less fashionable. Appealing to ontology (as in Heidegger), to metaphysics, or to religious ideals (as in Jonas) seemed less promising. Even though early continental philosophy was very critical with regard to strategic rationality and technology, it has been criticized by postmodernism as not moving radically beyond the central modernistic Western ideal of a rational philosophical synthesis or universal world interpretation.
Second, the focus within the philosophy of technology moved toward a renewed interest in looking at concrete technologies and the challenges they pose for analytical and ethical reflection, a movement that has been called the empirical turn in the philosophy of technology (Kroes, 2001).
Third, different attempts were soon made to bridge the gap between the two camps. In post-world-war Germany, the Society of German Engineers (VDI) established a dialogue about the responsibilities of scientists and engineers, addressing topics and worries of the humanities. The experience of the massive and systematic use of technology for organized mass murder during the holocaust and the development of technology for modern warfare, including the development of the nuclear bomb, raised issues about the responsibilities of engineers. The debate of the VDI meetings resulted in a series of important publications on the philosophy of technology (Rapp, 1981); these must be recognized as an important attempt to synthesize different strands of philosophical thinking, even though it can be asked how far the VDI school was really successful in transcending its engineering-philosophical origins (Mitcham, 1994, p. 71).
Along a similar line, authors have tried to combine the phenomenological approach with American pragmatism, thus bridging insights of a more continental and a more analytical tradition. Common to phenomenology and pragmatism is the idea of the priority of praxis over theory and thus the tendency not to see technology as applied science but, rather, science as a purified or abstract form of (technological) praxis. Following the works of John Dewey, thinkers like Paul T. Durbin (1992), Larry Hickman (1990), and Don Ihde (1979) have tried to establish a pragmatist phenomenological approach to technology. The insights of Don Ihde that each technology either extends human bodily experience (e.g., the microscope) or calls for human interpretations (e.g., the thermometer) are of particular anthropological interest. If technology amplifies our experience, then it always does so at the cost of a reduction: In highlighting or amplifying certain aspects of reality, it makes invisible other aspects of this very same reality (as in an ultrasonic picture) (Ihde, 1979). The way technology thus “mediates” our interpretation of the world, and our actions within it, has been a further object of extended research (e.g., Verbeek, 2005).
A further attempt to bridge humanist and engineering tradition has been made by Carl Mitcham (1994), who nevertheless tries to defend the priority of the humanist perspective, but at the same time develops an analytic framework that should serve for further investigation within the philosophy of technology. He distinguishes among technology as object (tools), as type of knowledge, as activity, and as volition (expression of man’s intention or will). The 1980s and 1990s saw an increased interest, especially in the analyses of the first three aspects of this distinction.
With regard to the fourth aspect, ethical issues have been a central topic for many philosophers of technology, ranging from debates about the responsibility of scientists and engineers, medical and bioethics, business ethics, technology assessment, risk assessment and decision under uncertainty, to environmental ethics. Two of these fields are of particular interest from an anthropological perspective: In environmental ethics, those theories might shed light on anthropological questions seeking to interpret the environmental crisis as essentially rooted in human nature. It has been argued that it is a human tendency to value short-term (individual) interests more highly than long-term (collective) interests, thus putting a pessimistic neo-Hobbesian anthropology in the middle of the debate. According to Garrett Hardin (1968), it is this very human tendency (together with a mismatch in the growth of the human population that exceeds the growth of the supply of the food or other resources) that leads to the “tragedy of the commons.” Research in game theory and environmental sociobiology indicates the possibility of holding a more optimistic view of the development of cooperative strategies in humans (Axelrod, 1984), though the issue is still debated and there is room for a more pessimistic perspective, as has been defended early on by some sociobiologists (Dawkins, 1978) or recently by some philosophers (Gardiner, 2001).
In the ethical debate on transhumanism, finally, many links can be found to classical anthropological questions about the essence of man (e.g., Baillie, 2005; Fukuyama, 2004). The central debated question is whether it is morally allowed, forbidden, or even demanded from us to enhance our human capacities through new technologies, ranging from short-term nonevasive ways (like taking performanceenhancing drugs) to fundamental irreversible changes (like genetic engineering). While bioconservativists argue against an extended usage of enhancement technologies, transhumanists point to the potential benefits of these new options. It is reasonable to assume that these issues will be with us as technology advances and opens new possibilities to alter the human condition. This opens a radical new challenge to anthropology, which until recently dedicated itself to understanding the given human nature, while it now has to face the normative question of which we should choose as our future nature, once technology offers radical new options of changing human nature (e.g., as by slowing down or even stopping the process of aging). It seems that the anthropology of the future must take into consideration, more and more, normative claims and it must reach out to incorporate ethics to prepare itself for the challenges modern technology poses.
Looking at recent tendencies in research, it can be argued that the initial focus on linking technology with a universal, philosophical anthropological vision, also rooted in biological knowledge, was one of the key achievements of early philosophical anthropology in the works of Gehlen and others. What made these anthropologies remarkable was their attempt to bring together the different traditions of anthropological thought, ranging from philosophy to sociology and biology. A turn toward a more social perspective was established first by Gehlen himself, the Frankfurt school, and later STS studies, sometimes leading away from or even lacking both an underlying philosophical vision and an interest in our biological nature. Very recently, however, sociologists and philosophers have shown an increased interest in biology (as is visible in the ever-growing numbers of publications in sociobiology and the philosophy of biology). This increased attention has not yet led to a revival of an interest in the links between anthropology and technology. But in order to understand man—both in his evolutionary origins and (maybe even more) in his current historical situation—it seems to demand attention to man’s amazing capacity to develop technology.
It can reasonably be argued that what is thus needed is a new vision of how to synthesize the different fields of biological, social, and cultural anthropology. It seems that after the empirical turn to gather extended details over the biological and social aspects of technology, there is now a call for a new philosophical turn, seeking a new discourse synthesis. Many classical questions of anthropology will tend to remain unanswered, if academic research remains focused only on disciplinary perspectives, which always look at only a part of the whole picture. It is certainly true that man is a social animal, that he has biological roots and that he can ask ethical and philosophical questions about the good and about his place in this universe. The disciplinary separations in biology, sociology, and philosophy (to name just a few) tend, however, to distract from the fact that man in reality is a unity, meaning that a true answer to the most fundamental question of anthropology (What is man?) calls for a plausible combination of these approaches. To synthesize the different aspects of our knowledge about our own human nature is certainly far from being an easy task, but it seems more needed than ever.
But if this is not yet a big enough challenge, there is even a second aspect that makes the quest for a synthesis even more challenging. It seems that a new anthropological vision of humankind must answer a question that classical anthropology has not been dealing with: If technology soon allows us to alter our very nature, then we must know not only what the human condition is, but also what the human condition should be.
Ethics might again enter anthropological reflection, as has been hinted at already by early thinkers such as Scheler and Jonas. Recent attempts to place man in the middle of both a normative vision of ideals, on the one side, and against a profound overview of our descriptive knowledge about our essence, on the other side (as in the voluminous attempt at a synthesis in Hösle, 2004), deserve attention, as they might be the first steps toward a renewed synthetic anthropology that tries to bridge the gaps among the different disciplines. A deepened understanding of technology must be a central part of these efforts, since the way we use tools and produce artifacts is one of the remarkable features of humankind—a feature in much need of guidance by descriptive knowledge and ethical wisdom, especially in our age in which technology (of which humans have been the subject) is about to discover the condition humana as its potential object in a way more radical than ever before.
Bibliography:
- Adorno, T. (1979). Dialectic of enlightenment. London: Verso.
- Adorno, T. (1999). Aesthetic theory. London: Athlone.
- Ambrose, S. H. (2001). Paleolithic technology and human evolution. Science, 291 (5509), 1748–1753.
- Axelrod, R. (1984). The evolution of cooperation. New York: Basic Books.
- Baillie, H. (2005). Is human nature obsolete? Genetics, bioengineering, and the future of the human condition. Cambridge: MIT Press.
- Beck, B. (1980). Animal tool behaviour. New York: Garland.
- Berger, P. L., & Luckmann, T. (1966). The social construction of reality: A treatise in the sociology of knowledge. Garden City, NY: Anchor Books.
- Bunge, M. (1979). The five buds of technophilosophy. Technology in Society, 1 (1), 67–74.
- Castells, M. (1999). The information age: Economy, society and culture. Malden, MA: Blackwell.
- Dawkins, R. (1978). The selfish gene. Oxford, UK: Oxford University Press.
- Durbin, P. (1992). Social responsibility in science, technology, and medicine. Bethlehem, PA: Lehigh University Press.
- Fukuyama, F. (2004). Transhumanism. Foreign Policy, 144, 42–43.
- Gardiner, S. M. (2001). The real tragedy of the commons. Philosophy and Public Affairs, 30 (4), 387–416.
- Gehlen, A. (1980). Man in the age of technology. New York: Columbia University Press.
- Gehlen, A. (1988). Man, his nature and place in the world. New York: Columbia University Press.
- Habermas, J. (1971). Knowledge and human interests. Boston: Beacon Press.
- Haferkamp, H. (1992). Social change and modernity. Berkeley: University of California Press.
- Heidegger, M. (1977). The question concerning technology, and other essays. New York: Harper & Row.
- Hickman, L. (1990). John Dewey’s pragmatic technology. Bloomington: Indiana University Press.
- Hösle, V. (2004). Morals and politics (S. Randall, Trans.). Notre Dame, IN: University of Notre Dame Press.
- Ihde, D. (1979). Technics and praxis. Boston: D. Reidel.
- Jonas, H. (1984). The imperative of responsibility: In search of an ethics for the technological age. Chicago: University of Chicago Press.
- Kroes, P. (2001). The empirical turn in the philosophy of technology. New York: JAI.
- Laland, K. (2009). The question of animal culture. Cambridge, MA: Harvard University Press.
- Latour, B. (1979). The social construction of scientific facts. Beverly Hills, CA: Sage.
- Latour, B. (2005). Reassembling the social: An introduction to actor-network-theory. New York: Oxford University Press.
- Marx, K. (1938). London: Allen & Unwin.
- McDermott, J. (1969). Technology: The opiate of the intellectuals. The New York Review of Books, 13 (2), 25–35.
- Misa, T. J., Brey, P., & Feenberg, A. (2004). Modernity and technology. Cambridge: MIT Press.
- Mitcham, C. (1994). Thinking through technology: The path between engineering and philosophy. Chicago: University of Chicago Press.
- Nora, S. (1980). The computerization of society: A report to the president of France. Cambridge: MIT Press.
- Oakley, K. (1957). Man the tool-maker. Chicago: University of Chicago Press.
- Ortega y Gasset, J. (1961). Meditations on Quixote. New York: Norton. (Original work published 1914)
- Pfaffenberger, B. (1988). Fetishised objects and humanized nature: Towards an anthropology of technology. Man, 23 (2), 236–252.
- Pfaffenberger, B. (1992). Social anthropology of technology. Annual Revue of Anthropology, 21, 491–516.
- Pressnell, L. (1960). Studies in the industrial revolution, presented to T. S. Ashton. London: University of LondonAthlone Press.
- Rapp, F. (1981). Analytical philosophy of technology. Boston: D. Reidel.
- Russell, B. (1951). The impact of science on society. New York: Columbia University Press.
- Schaik, C. P., Deaner, R. O., & Merrill, M.Y. (1999).The conditions for tool use in primates: Implications for the evolution of material culture. Journal of Human Evolution, 36 (6), 719–741.
- Scheler, M. (1961). Man’s place in nature. New York: Farrar, Strauss & Giroux.
- Smelser, N. (1969). Social change in the industrial revolution: An application of theory to the British cotton industry. Chicago: University of Chicago Press.
- Snow, C. (1959). The two cultures and the scientific revolution (The Rede lecture, 1959). Cambridge, UK: Cambridge University Press.
- Tomasello, M. (1999). The cultural origins of human cognition. Cambridge, MA: Harvard University Press.
- Verbeek, P. P. (2005). What things do: Philosophical reflections on technology, agency, and design. University Park: Pennsylvania State University Press.
- Winner, L. (1980). Do artifacts have politics? Daedalus, 109, 121–123.
- Winner, L. (1986). Technology as forms of life. In The whale and the reactor: A search for limits in an age of high technology. Chicago: University of Chicago Press.
- Woolgar, S. (1991). The turn to technology. Science, Technology and Human Values, 16 (1), 20–50.
- Wrangham, R. (1994). Chimpanzee cultures. Cambridge, MA: Harvard University Press.
ORDER HIGH QUALITY CUSTOM PAPER

- Information Systems
- Information Systems (Business Informatics)
- Information Technology
Current Trends In Information Technology: Which Way For Modern It Experts
- 68(7):2231-2803

- Masinde Muliro University of Science and Technology

Abstract and Figures
![research report technology Mobile-Computing Devices Source: [3]](https://www.researchgate.net/profile/Victor-Kadima/publication/342988285/figure/fig1/AS:995326487969793@1614315735463/Mobile-Computing-Devices-Source-3_Q320.jpg)
Discover the world's research
- 25+ million members
- 160+ million publication pages
- 2.3+ billion citations

- Arif Muhamad Nurdin

- Saumitra Singh

- S H H Hashmi
- P M Hassan Umar
- Recruit researchers
- Join for free
- Login Email Tip: Most researchers use their institutional email address as their ResearchGate login Password Forgot password? Keep me logged in Log in or Continue with Google Welcome back! Please log in. Email · Hint Tip: Most researchers use their institutional email address as their ResearchGate login Password Forgot password? Keep me logged in Log in or Continue with Google No account? Sign up
Thank you for visiting nature.com. You are using a browser version with limited support for CSS. To obtain the best experience, we recommend you use a more up to date browser (or turn off compatibility mode in Internet Explorer). In the meantime, to ensure continued support, we are displaying the site without styles and JavaScript.
- View all journals
- Explore content
- About the journal
- Publish with us
- Sign up for alerts
- Open access
- Published: 31 August 2024
Knowledge mapping and evolution of research on older adults’ technology acceptance: a bibliometric study from 2013 to 2023
- Xianru Shang ORCID: orcid.org/0009-0000-8906-3216 1 ,
- Zijian Liu 1 ,
- Chen Gong 1 ,
- Zhigang Hu 1 ,
- Yuexuan Wu 1 &
- Chengliang Wang ORCID: orcid.org/0000-0003-2208-3508 2
Humanities and Social Sciences Communications volume 11 , Article number: 1115 ( 2024 ) Cite this article
Metrics details
- Science, technology and society
The rapid expansion of information technology and the intensification of population aging are two prominent features of contemporary societal development. Investigating older adults’ acceptance and use of technology is key to facilitating their integration into an information-driven society. Given this context, the technology acceptance of older adults has emerged as a prioritized research topic, attracting widespread attention in the academic community. However, existing research remains fragmented and lacks a systematic framework. To address this gap, we employed bibliometric methods, utilizing the Web of Science Core Collection to conduct a comprehensive review of literature on older adults’ technology acceptance from 2013 to 2023. Utilizing VOSviewer and CiteSpace for data assessment and visualization, we created knowledge mappings of research on older adults’ technology acceptance. Our study employed multidimensional methods such as co-occurrence analysis, clustering, and burst analysis to: (1) reveal research dynamics, key journals, and domains in this field; (2) identify leading countries, their collaborative networks, and core research institutions and authors; (3) recognize the foundational knowledge system centered on theoretical model deepening, emerging technology applications, and research methods and evaluation, uncovering seminal literature and observing a shift from early theoretical and influential factor analyses to empirical studies focusing on individual factors and emerging technologies; (4) moreover, current research hotspots are primarily in the areas of factors influencing technology adoption, human-robot interaction experiences, mobile health management, and aging-in-place technology, highlighting the evolutionary context and quality distribution of research themes. Finally, we recommend that future research should deeply explore improvements in theoretical models, long-term usage, and user experience evaluation. Overall, this study presents a clear framework of existing research in the field of older adults’ technology acceptance, providing an important reference for future theoretical exploration and innovative applications.
Similar content being viewed by others
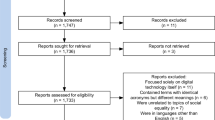
Research progress and intellectual structure of design for digital equity (DDE): A bibliometric analysis based on citespace
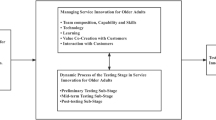
Exploring the role of interaction in older-adult service innovation: insights from the testing stage
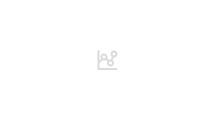
Smart device interest, perceived usefulness, and preferences in rural Alabama seniors
Introduction.
In contemporary society, the rapid development of information technology has been intricately intertwined with the intensifying trend of population aging. According to the latest United Nations forecast, by 2050, the global population aged 65 and above is expected to reach 1.6 billion, representing about 16% of the total global population (UN 2023 ). Given the significant challenges of global aging, there is increasing evidence that emerging technologies have significant potential to maintain health and independence for older adults in their home and healthcare environments (Barnard et al. 2013 ; Soar 2010 ; Vancea and Solé-Casals 2016 ). This includes, but is not limited to, enhancing residential safety with smart home technologies (Touqeer et al. 2021 ; Wang et al. 2022 ), improving living independence through wearable technologies (Perez et al. 2023 ), and increasing medical accessibility via telehealth services (Kruse et al. 2020 ). Technological innovations are redefining the lifestyles of older adults, encouraging a shift from passive to active participation (González et al. 2012 ; Mostaghel 2016 ). Nevertheless, the effective application and dissemination of technology still depends on user acceptance and usage intentions (Naseri et al. 2023 ; Wang et al. 2023a ; Xia et al. 2024 ; Yu et al. 2023 ). Particularly, older adults face numerous challenges in accepting and using new technologies. These challenges include not only physical and cognitive limitations but also a lack of technological experience, along with the influences of social and economic factors (Valk et al. 2018 ; Wilson et al. 2021 ).
User acceptance of technology is a significant focus within information systems (IS) research (Dai et al. 2024 ), with several models developed to explain and predict user behavior towards technology usage, including the Technology Acceptance Model (TAM) (Davis 1989 ), TAM2, TAM3, and the Unified Theory of Acceptance and Use of Technology (UTAUT) (Venkatesh et al. 2003 ). Older adults, as a group with unique needs, exhibit different behavioral patterns during technology acceptance than other user groups, and these uniquenesses include changes in cognitive abilities, as well as motivations, attitudes, and perceptions of the use of new technologies (Chen and Chan 2011 ). The continual expansion of technology introduces considerable challenges for older adults, rendering the understanding of their technology acceptance a research priority. Thus, conducting in-depth research into older adults’ acceptance of technology is critically important for enhancing their integration into the information society and improving their quality of life through technological advancements.
Reviewing relevant literature to identify research gaps helps further solidify the theoretical foundation of the research topic. However, many existing literature reviews primarily focus on the factors influencing older adults’ acceptance or intentions to use technology. For instance, Ma et al. ( 2021 ) conducted a comprehensive analysis of the determinants of older adults’ behavioral intentions to use technology; Liu et al. ( 2022 ) categorized key variables in studies of older adults’ technology acceptance, noting a shift in focus towards social and emotional factors; Yap et al. ( 2022 ) identified seven categories of antecedents affecting older adults’ use of technology from an analysis of 26 articles, including technological, psychological, social, personal, cost, behavioral, and environmental factors; Schroeder et al. ( 2023 ) extracted 119 influencing factors from 59 articles and further categorized these into six themes covering demographics, health status, and emotional awareness. Additionally, some studies focus on the application of specific technologies, such as Ferguson et al. ( 2021 ), who explored barriers and facilitators to older adults using wearable devices for heart monitoring, and He et al. ( 2022 ) and Baer et al. ( 2022 ), who each conducted in-depth investigations into the acceptance of social assistive robots and mobile nutrition and fitness apps, respectively. In summary, current literature reviews on older adults’ technology acceptance exhibit certain limitations. Due to the interdisciplinary nature and complex knowledge structure of this field, traditional literature reviews often rely on qualitative analysis, based on literature analysis and periodic summaries, which lack sufficient objectivity and comprehensiveness. Additionally, systematic research is relatively limited, lacking a macroscopic description of the research trajectory from a holistic perspective. Over the past decade, research on older adults’ technology acceptance has experienced rapid growth, with a significant increase in literature, necessitating the adoption of new methods to review and examine the developmental trends in this field (Chen 2006 ; Van Eck and Waltman 2010 ). Bibliometric analysis, as an effective quantitative research method, analyzes published literature through visualization, offering a viable approach to extracting patterns and insights from a large volume of papers, and has been widely applied in numerous scientific research fields (Achuthan et al. 2023 ; Liu and Duffy 2023 ). Therefore, this study will employ bibliometric methods to systematically analyze research articles related to older adults’ technology acceptance published in the Web of Science Core Collection from 2013 to 2023, aiming to understand the core issues and evolutionary trends in the field, and to provide valuable references for future related research. Specifically, this study aims to explore and answer the following questions:
RQ1: What are the research dynamics in the field of older adults’ technology acceptance over the past decade? What are the main academic journals and fields that publish studies related to older adults’ technology acceptance?
RQ2: How is the productivity in older adults’ technology acceptance research distributed among countries, institutions, and authors?
RQ3: What are the knowledge base and seminal literature in older adults’ technology acceptance research? How has the research theme progressed?
RQ4: What are the current hot topics and their evolutionary trajectories in older adults’ technology acceptance research? How is the quality of research distributed?
Methodology and materials
Research method.
In recent years, bibliometrics has become one of the crucial methods for analyzing literature reviews and is widely used in disciplinary and industrial intelligence analysis (Jing et al. 2023 ; Lin and Yu 2024a ; Wang et al. 2024a ; Xu et al. 2021 ). Bibliometric software facilitates the visualization analysis of extensive literature data, intuitively displaying the network relationships and evolutionary processes between knowledge units, and revealing the underlying knowledge structure and potential information (Chen et al. 2024 ; López-Robles et al. 2018 ; Wang et al. 2024c ). This method provides new insights into the current status and trends of specific research areas, along with quantitative evidence, thereby enhancing the objectivity and scientific validity of the research conclusions (Chen et al. 2023 ; Geng et al. 2024 ). VOSviewer and CiteSpace are two widely used bibliometric software tools in academia (Pan et al. 2018 ), recognized for their robust functionalities based on the JAVA platform. Although each has its unique features, combining these two software tools effectively constructs mapping relationships between literature knowledge units and clearly displays the macrostructure of the knowledge domains. Particularly, VOSviewer, with its excellent graphical representation capabilities, serves as an ideal tool for handling large datasets and precisely identifying the focal points and hotspots of research topics. Therefore, this study utilizes VOSviewer (version 1.6.19) and CiteSpace (version 6.1.R6), combined with in-depth literature analysis, to comprehensively examine and interpret the research theme of older adults’ technology acceptance through an integrated application of quantitative and qualitative methods.
Data source
Web of Science is a comprehensively recognized database in academia, featuring literature that has undergone rigorous peer review and editorial scrutiny (Lin and Yu 2024b ; Mongeon and Paul-Hus 2016 ; Pranckutė 2021 ). This study utilizes the Web of Science Core Collection as its data source, specifically including three major citation indices: Science Citation Index Expanded (SCIE), Social Sciences Citation Index (SSCI), and Arts & Humanities Citation Index (A&HCI). These indices encompass high-quality research literature in the fields of science, social sciences, and arts and humanities, ensuring the comprehensiveness and reliability of the data. We combined “older adults” with “technology acceptance” through thematic search, with the specific search strategy being: TS = (elder OR elderly OR aging OR ageing OR senile OR senior OR old people OR “older adult*”) AND TS = (“technology acceptance” OR “user acceptance” OR “consumer acceptance”). The time span of literature search is from 2013 to 2023, with the types limited to “Article” and “Review” and the language to “English”. Additionally, the search was completed by October 27, 2023, to avoid data discrepancies caused by database updates. The initial search yielded 764 journal articles. Given that searches often retrieve articles that are superficially relevant but actually non-compliant, manual screening post-search was essential to ensure the relevance of the literature (Chen et al. 2024 ). Through manual screening, articles significantly deviating from the research theme were eliminated and rigorously reviewed. Ultimately, this study obtained 500 valid sample articles from the Web of Science Core Collection. The complete PRISMA screening process is illustrated in Fig. 1 .
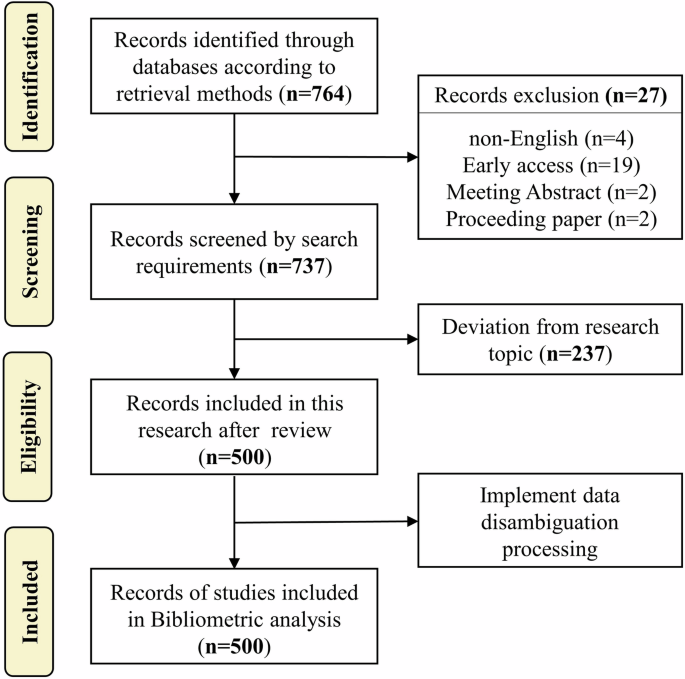
Presentation of the data culling process in detail.
Data standardization
Raw data exported from databases often contain multiple expressions of the same terminology (Nguyen and Hallinger 2020 ). To ensure the accuracy and consistency of data, it is necessary to standardize the raw data (Strotmann and Zhao 2012 ). This study follows the data standardization process proposed by Taskin and Al ( 2019 ), mainly executing the following operations:
(1) Standardization of author and institution names is conducted to address different name expressions for the same author. For instance, “Chan, Alan Hoi Shou” and “Chan, Alan H. S.” are considered the same author, and distinct authors with the same name are differentiated by adding identifiers. Diverse forms of institutional names are unified to address variations caused by name changes or abbreviations, such as standardizing “FRANKFURT UNIV APPL SCI” and “Frankfurt University of Applied Sciences,” as well as “Chinese University of Hong Kong” and “University of Hong Kong” to consistent names.
(2) Different expressions of journal names are unified. For example, “International Journal of Human-Computer Interaction” and “Int J Hum Comput Interact” are standardized to a single name. This ensures consistency in journal names and prevents misclassification of literature due to differing journal names. Additionally, it involves checking if the journals have undergone name changes in the past decade to prevent any impact on the analysis due to such changes.
(3) Keywords data are cleansed by removing words that do not directly pertain to specific research content (e.g., people, review), merging synonyms (e.g., “UX” and “User Experience,” “aging-in-place” and “aging in place”), and standardizing plural forms of keywords (e.g., “assistive technologies” and “assistive technology,” “social robots” and “social robot”). This reduces redundant information in knowledge mapping.
Bibliometric results and analysis
Distribution power (rq1), literature descriptive statistical analysis.
Table 1 presents a detailed descriptive statistical overview of the literature in the field of older adults’ technology acceptance. After deduplication using the CiteSpace software, this study confirmed a valid sample size of 500 articles. Authored by 1839 researchers, the documents encompass 792 research institutions across 54 countries and are published in 217 different academic journals. As of the search cutoff date, these articles have accumulated 13,829 citations, with an annual average of 1156 citations, and an average of 27.66 citations per article. The h-index, a composite metric of quantity and quality of scientific output (Kamrani et al. 2021 ), reached 60 in this study.
Trends in publications and disciplinary distribution
The number of publications and citations are significant indicators of the research field’s development, reflecting its continuity, attention, and impact (Ale Ebrahim et al. 2014 ). The ranking of annual publications and citations in the field of older adults’ technology acceptance studies is presented chronologically in Fig. 2A . The figure shows a clear upward trend in the amount of literature in this field. Between 2013 and 2017, the number of publications increased slowly and decreased in 2018. However, in 2019, the number of publications increased rapidly to 52 and reached a peak of 108 in 2022, which is 6.75 times higher than in 2013. In 2022, the frequency of document citations reached its highest point with 3466 citations, reflecting the widespread recognition and citation of research in this field. Moreover, the curve of the annual number of publications fits a quadratic function, with a goodness-of-fit R 2 of 0.9661, indicating that the number of future publications is expected to increase even more rapidly.
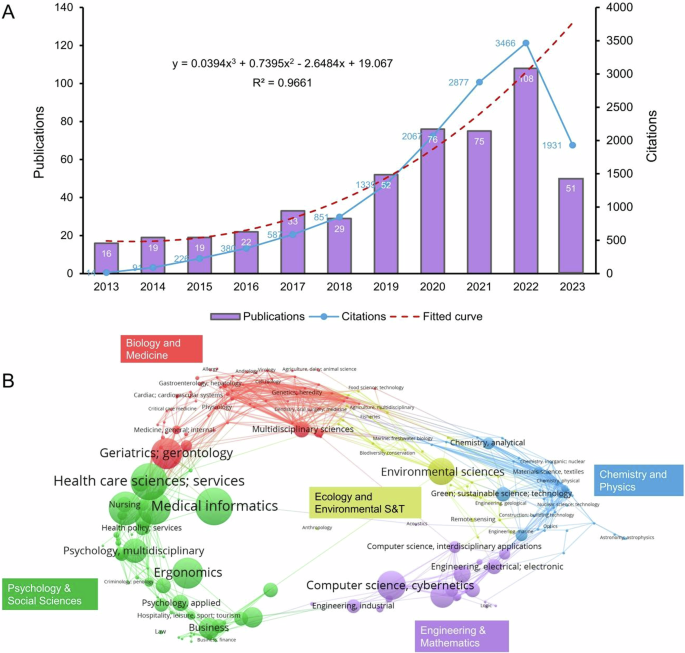
A Trends in trends in annual publications and citations (2013–2023). B Overlay analysis of the distribution of discipline fields.
Figure 2B shows that research on older adults’ technology acceptance involves the integration of multidisciplinary knowledge. According to Web of Science Categories, these 500 articles are distributed across 85 different disciplines. We have tabulated the top ten disciplines by publication volume (Table 2 ), which include Medical Informatics (75 articles, 15.00%), Health Care Sciences & Services (71 articles, 14.20%), Gerontology (61 articles, 12.20%), Public Environmental & Occupational Health (57 articles, 11.40%), and Geriatrics & Gerontology (52 articles, 10.40%), among others. The high output in these disciplines reflects the concentrated global academic interest in this comprehensive research topic. Additionally, interdisciplinary research approaches provide diverse perspectives and a solid theoretical foundation for studies on older adults’ technology acceptance, also paving the way for new research directions.
Knowledge flow analysis
A dual-map overlay is a CiteSpace map superimposed on top of a base map, which shows the interrelationships between journals in different domains, representing the publication and citation activities in each domain (Chen and Leydesdorff 2014 ). The overlay map reveals the link between the citing domain (on the left side) and the cited domain (on the right side), reflecting the knowledge flow of the discipline at the journal level (Leydesdorff and Rafols 2012 ). We utilize the in-built Z-score algorithm of the software to cluster the graph, as shown in Fig. 3 .
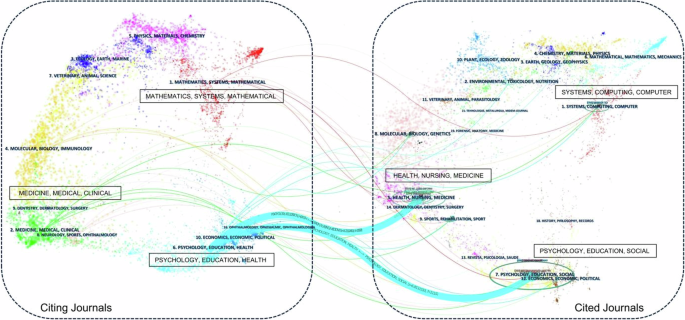
The left side shows the citing journal, and the right side shows the cited journal.
Figure 3 shows the distribution of citing journals clusters for older adults’ technology acceptance on the left side, while the right side refers to the main cited journals clusters. Two knowledge flow citation trajectories were obtained; they are presented by the color of the cited regions, and the thickness of these trajectories is proportional to the Z-score scaled frequency of citations (Chen et al. 2014 ). Within the cited regions, the most popular fields with the most records covered are “HEALTH, NURSING, MEDICINE” and “PSYCHOLOGY, EDUCATION, SOCIAL”, and the elliptical aspect ratio of these two fields stands out. Fields have prominent elliptical aspect ratios, highlighting their significant influence on older adults’ technology acceptance research. Additionally, the major citation trajectories originate in these two areas and progress to the frontier research area of “PSYCHOLOGY, EDUCATION, HEALTH”. It is worth noting that the citation trajectory from “PSYCHOLOGY, EDUCATION, SOCIAL” has a significant Z-value (z = 6.81), emphasizing the significance and impact of this development path. In the future, “MATHEMATICS, SYSTEMS, MATHEMATICAL”, “MOLECULAR, BIOLOGY, IMMUNOLOGY”, and “NEUROLOGY, SPORTS, OPHTHALMOLOGY” may become emerging fields. The fields of “MEDICINE, MEDICAL, CLINICAL” may be emerging areas of cutting-edge research.
Main research journals analysis
Table 3 provides statistics for the top ten journals by publication volume in the field of older adults’ technology acceptance. Together, these journals have published 137 articles, accounting for 27.40% of the total publications, indicating that there is no highly concentrated core group of journals in this field, with publications being relatively dispersed. Notably, Computers in Human Behavior , Journal of Medical Internet Research , and International Journal of Human-Computer Interaction each lead with 15 publications. In terms of citation metrics, International Journal of Medical Informatics and Computers in Human Behavior stand out significantly, with the former accumulating a total of 1,904 citations, averaging 211.56 citations per article, and the latter totaling 1,449 citations, with an average of 96.60 citations per article. These figures emphasize the academic authority and widespread impact of these journals within the research field.
Research power (RQ2)
Countries and collaborations analysis.
The analysis revealed the global research pattern for country distribution and collaboration (Chen et al. 2019 ). Figure 4A shows the network of national collaborations on older adults’ technology acceptance research. The size of the bubbles represents the amount of publications in each country, while the thickness of the connecting lines expresses the closeness of the collaboration among countries. Generally, this research subject has received extensive international attention, with China and the USA publishing far more than any other countries. China has established notable research collaborations with the USA, UK and Malaysia in this field, while other countries have collaborations, but the closeness is relatively low and scattered. Figure 4B shows the annual publication volume dynamics of the top ten countries in terms of total publications. Since 2017, China has consistently increased its annual publications, while the USA has remained relatively stable. In 2019, the volume of publications in each country increased significantly, this was largely due to the global outbreak of the COVID-19 pandemic, which has led to increased reliance on information technology among the elderly for medical consultations, online socialization, and health management (Sinha et al. 2021 ). This phenomenon has led to research advances in technology acceptance among older adults in various countries. Table 4 shows that the top ten countries account for 93.20% of the total cumulative number of publications, with each country having published more than 20 papers. Among these ten countries, all of them except China are developed countries, indicating that the research field of older adults’ technology acceptance has received general attention from developed countries. Currently, China and the USA were the leading countries in terms of publications with 111 and 104 respectively, accounting for 22.20% and 20.80%. The UK, Germany, Italy, and the Netherlands also made significant contributions. The USA and China ranked first and second in terms of the number of citations, while the Netherlands had the highest average citations, indicating the high impact and quality of its research. The UK has shown outstanding performance in international cooperation, while the USA highlights its significant academic influence in this field with the highest h-index value.
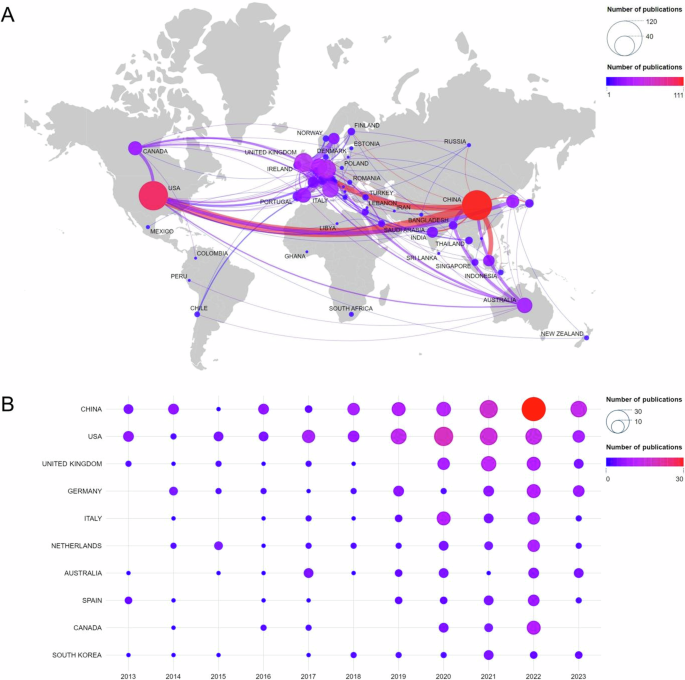
A National collaboration network. B Annual volume of publications in the top 10 countries.
Institutions and authors analysis
Analyzing the number of publications and citations can reveal an institution’s or author’s research strength and influence in a particular research area (Kwiek 2021 ). Tables 5 and 6 show the statistics of the institutions and authors whose publication counts are in the top ten, respectively. As shown in Table 5 , higher education institutions hold the main position in this research field. Among the top ten institutions, City University of Hong Kong and The University of Hong Kong from China lead with 14 and 9 publications, respectively. City University of Hong Kong has the highest h-index, highlighting its significant influence in the field. It is worth noting that Tilburg University in the Netherlands is not among the top five in terms of publications, but the high average citation count (130.14) of its literature demonstrates the high quality of its research.
After analyzing the authors’ output using Price’s Law (Redner 1998 ), the highest number of publications among the authors counted ( n = 10) defines a publication threshold of 3 for core authors in this research area. As a result of quantitative screening, a total of 63 core authors were identified. Table 6 shows that Chen from Zhejiang University, China, Ziefle from RWTH Aachen University, Germany, and Rogers from Macquarie University, Australia, were the top three authors in terms of the number of publications, with 10, 9, and 8 articles, respectively. In terms of average citation rate, Peek and Wouters, both scholars from the Netherlands, have significantly higher rates than other scholars, with 183.2 and 152.67 respectively. This suggests that their research is of high quality and widely recognized. Additionally, Chen and Rogers have high h-indices in this field.
Knowledge base and theme progress (RQ3)
Research knowledge base.
Co-citation relationships occur when two documents are cited together (Zhang and Zhu 2022 ). Co-citation mapping uses references as nodes to represent the knowledge base of a subject area (Min et al. 2021). Figure 5A illustrates co-occurrence mapping in older adults’ technology acceptance research, where larger nodes signify higher co-citation frequencies. Co-citation cluster analysis can be used to explore knowledge structure and research boundaries (Hota et al. 2020 ; Shiau et al. 2023 ). The co-citation clustering mapping of older adults’ technology acceptance research literature (Fig. 5B ) shows that the Q value of the clustering result is 0.8129 (>0.3), and the average value of the weight S is 0.9391 (>0.7), indicating that the clusters are uniformly distributed with a significant and credible structure. This further proves that the boundaries of the research field are clear and there is significant differentiation in the field. The figure features 18 cluster labels, each associated with thematic color blocks corresponding to different time slices. Highlighted emerging research themes include #2 Smart Home Technology, #7 Social Live, and #10 Customer Service. Furthermore, the clustering labels extracted are primarily classified into three categories: theoretical model deepening, emerging technology applications, research methods and evaluation, as detailed in Table 7 .
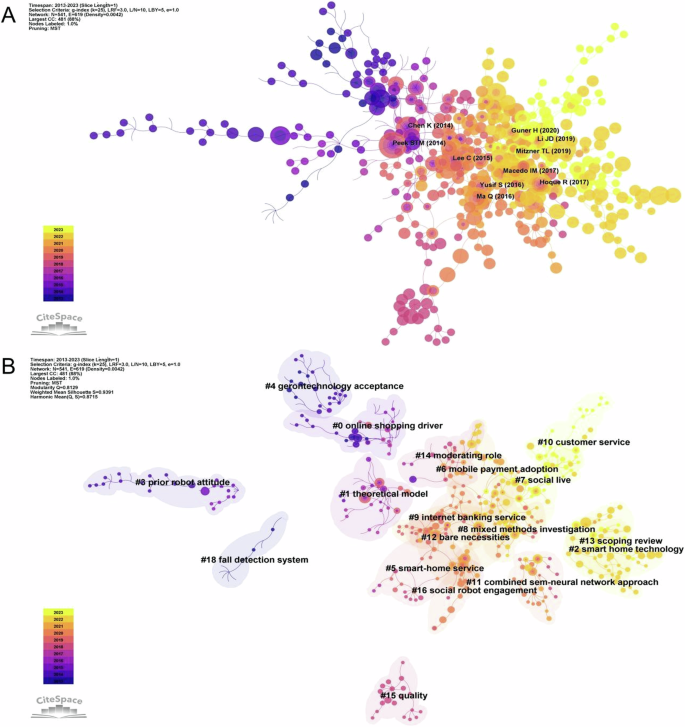
A Co-citation analysis of references. B Clustering network analysis of references.
Seminal literature analysis
The top ten nodes in terms of co-citation frequency were selected for further analysis. Table 8 displays the corresponding node information. Studies were categorized into four main groups based on content analysis. (1) Research focusing on specific technology usage by older adults includes studies by Peek et al. ( 2014 ), Ma et al. ( 2016 ), Hoque and Sorwar ( 2017 ), and Li et al. ( 2019 ), who investigated the factors influencing the use of e-technology, smartphones, mHealth, and smart wearables, respectively. (2) Concerning the development of theoretical models of technology acceptance, Chen and Chan ( 2014 ) introduced the Senior Technology Acceptance Model (STAM), and Macedo ( 2017 ) analyzed the predictive power of UTAUT2 in explaining older adults’ intentional behaviors and information technology usage. (3) In exploring older adults’ information technology adoption and behavior, Lee and Coughlin ( 2015 ) emphasized that the adoption of technology by older adults is a multifactorial process that includes performance, price, value, usability, affordability, accessibility, technical support, social support, emotion, independence, experience, and confidence. Yusif et al. ( 2016 ) conducted a literature review examining the key barriers affecting older adults’ adoption of assistive technology, including factors such as privacy, trust, functionality/added value, cost, and stigma. (4) From the perspective of research into older adults’ technology acceptance, Mitzner et al. ( 2019 ) assessed the long-term usage of computer systems designed for the elderly, whereas Guner and Acarturk ( 2020 ) compared information technology usage and acceptance between older and younger adults. The breadth and prevalence of this literature make it a vital reference for researchers in the field, also providing new perspectives and inspiration for future research directions.
Research thematic progress
Burst citation is a node of literature that guides the sudden change in dosage, which usually represents a prominent development or major change in a particular field, with innovative and forward-looking qualities. By analyzing the emergent literature, it is often easy to understand the dynamics of the subject area, mapping the emerging thematic change (Chen et al. 2022 ). Figure 6 shows the burst citation mapping in the field of older adults’ technology acceptance research, with burst citations represented by red nodes (Fig. 6A ). For the ten papers with the highest burst intensity (Fig. 6B ), this study will conduct further analysis in conjunction with literature review.
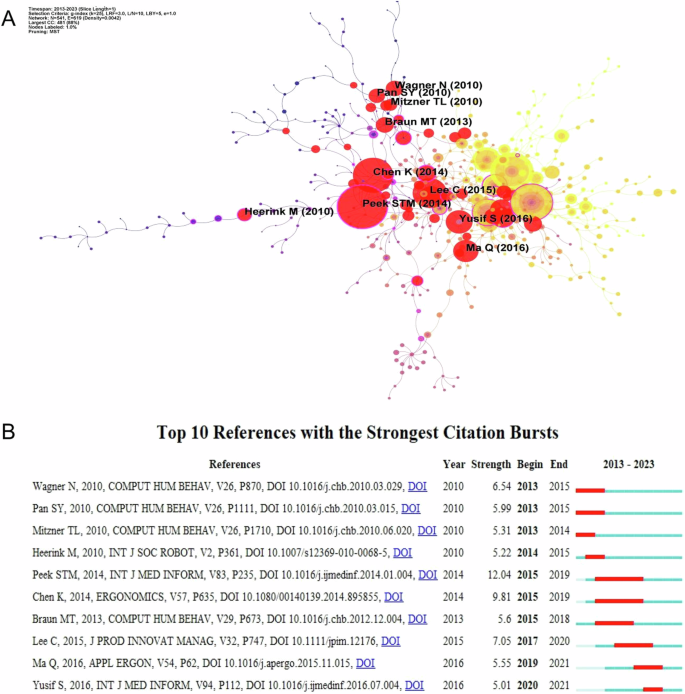
A Burst detection of co-citation. B The top 10 references with the strongest citation bursts.
As shown in Fig. 6 , Mitzner et al. ( 2010 ) broke the stereotype that older adults are fearful of technology, found that they actually have positive attitudes toward technology, and emphasized the centrality of ease of use and usefulness in the process of technology acceptance. This finding provides an important foundation for subsequent research. During the same period, Wagner et al. ( 2010 ) conducted theory-deepening and applied research on technology acceptance among older adults. The research focused on older adults’ interactions with computers from the perspective of Social Cognitive Theory (SCT). This expanded the understanding of technology acceptance, particularly regarding the relationship between behavior, environment, and other SCT elements. In addition, Pan and Jordan-Marsh ( 2010 ) extended the TAM to examine the interactions among predictors of perceived usefulness, perceived ease of use, subjective norm, and convenience conditions when older adults use the Internet, taking into account the moderating roles of gender and age. Heerink et al. ( 2010 ) adapted and extended the UTAUT, constructed a technology acceptance model specifically designed for older users’ acceptance of assistive social agents, and validated it using controlled experiments and longitudinal data, explaining intention to use by combining functional assessment and social interaction variables.
Then the research theme shifted to an in-depth analysis of the factors influencing technology acceptance among older adults. Two papers with high burst strengths emerged during this period: Peek et al. ( 2014 ) (Strength = 12.04), Chen and Chan ( 2014 ) (Strength = 9.81). Through a systematic literature review and empirical study, Peek STM and Chen K, among others, identified multidimensional factors that influence older adults’ technology acceptance. Peek et al. ( 2014 ) analyzed literature on the acceptance of in-home care technology among older adults and identified six factors that influence their acceptance: concerns about technology, expected benefits, technology needs, technology alternatives, social influences, and older adult characteristics, with a focus on differences between pre- and post-implementation factors. Chen and Chan ( 2014 ) constructed the STAM by administering a questionnaire to 1012 older adults and adding eight important factors, including technology anxiety, self-efficacy, cognitive ability, and physical function, based on the TAM. This enriches the theoretical foundation of the field. In addition, Braun ( 2013 ) highlighted the role of perceived usefulness, trust in social networks, and frequency of Internet use in older adults’ use of social networks, while ease of use and social pressure were not significant influences. These findings contribute to the study of older adults’ technology acceptance within specific technology application domains.
Recent research has focused on empirical studies of personal factors and emerging technologies. Ma et al. ( 2016 ) identified key personal factors affecting smartphone acceptance among older adults through structured questionnaires and face-to-face interviews with 120 participants. The study found that cost, self-satisfaction, and convenience were important factors influencing perceived usefulness and ease of use. This study offers empirical evidence to comprehend the main factors that drive smartphone acceptance among Chinese older adults. Additionally, Yusif et al. ( 2016 ) presented an overview of the obstacles that hinder older adults’ acceptance of assistive technologies, focusing on privacy, trust, and functionality.
In summary, research on older adults’ technology acceptance has shifted from early theoretical deepening and analysis of influencing factors to empirical studies in the areas of personal factors and emerging technologies, which have greatly enriched the theoretical basis of older adults’ technology acceptance and provided practical guidance for the design of emerging technology products.
Research hotspots, evolutionary trends, and quality distribution (RQ4)
Core keywords analysis.
Keywords concise the main idea and core of the literature, and are a refined summary of the research content (Huang et al. 2021 ). In CiteSpace, nodes with a centrality value greater than 0.1 are considered to be critical nodes. Analyzing keywords with high frequency and centrality helps to visualize the hot topics in the research field (Park et al. 2018 ). The merged keywords were imported into CiteSpace, and the top 10 keywords were counted and sorted by frequency and centrality respectively, as shown in Table 9 . The results show that the keyword “TAM” has the highest frequency (92), followed by “UTAUT” (24), which reflects that the in-depth study of the existing technology acceptance model and its theoretical expansion occupy a central position in research related to older adults’ technology acceptance. Furthermore, the terms ‘assistive technology’ and ‘virtual reality’ are both high-frequency and high-centrality terms (frequency = 17, centrality = 0.10), indicating that the research on assistive technology and virtual reality for older adults is the focus of current academic attention.
Research hotspots analysis
Using VOSviewer for keyword co-occurrence analysis organizes keywords into groups or clusters based on their intrinsic connections and frequencies, clearly highlighting the research field’s hot topics. The connectivity among keywords reveals correlations between different topics. To ensure accuracy, the analysis only considered the authors’ keywords. Subsequently, the keywords were filtered by setting the keyword frequency to 5 to obtain the keyword clustering map of the research on older adults’ technology acceptance research keyword clustering mapping (Fig. 7 ), combined with the keyword co-occurrence clustering network (Fig. 7A ) and the corresponding density situation (Fig. 7B ) to make a detailed analysis of the following four groups of clustered themes.
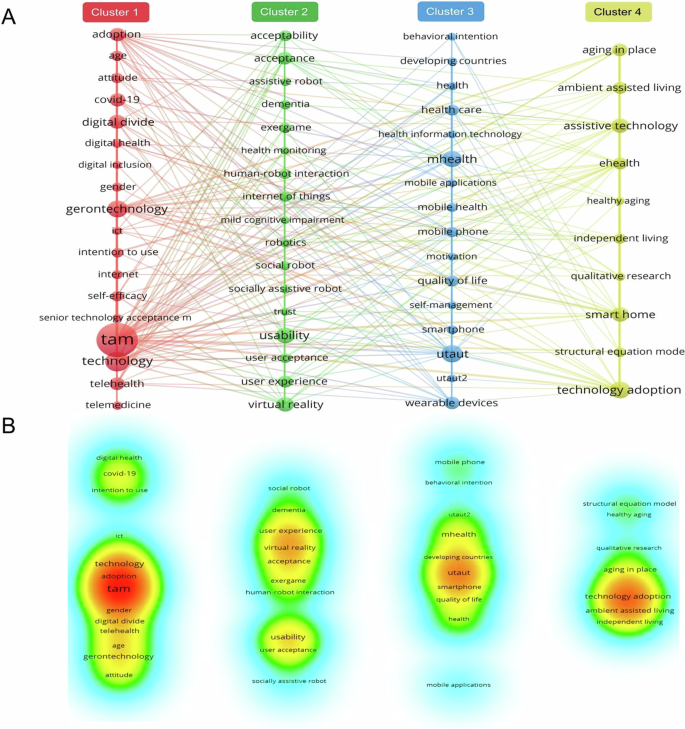
A Co-occurrence clustering network. B Keyword density.
Cluster #1—Research on the factors influencing technology adoption among older adults is a prominent topic, covering age, gender, self-efficacy, attitude, and and intention to use (Berkowsky et al. 2017 ; Wang et al. 2017 ). It also examined older adults’ attitudes towards and acceptance of digital health technologies (Ahmad and Mozelius, 2022 ). Moreover, the COVID-19 pandemic, significantly impacting older adults’ technology attitudes and usage, has underscored the study’s importance and urgency. Therefore, it is crucial to conduct in-depth studies on how older adults accept, adopt, and effectively use new technologies, to address their needs and help them overcome the digital divide within digital inclusion. This will improve their quality of life and healthcare experiences.
Cluster #2—Research focuses on how older adults interact with assistive technologies, especially assistive robots and health monitoring devices, emphasizing trust, usability, and user experience as crucial factors (Halim et al. 2022 ). Moreover, health monitoring technologies effectively track and manage health issues common in older adults, like dementia and mild cognitive impairment (Lussier et al. 2018 ; Piau et al. 2019 ). Interactive exercise games and virtual reality have been deployed to encourage more physical and cognitive engagement among older adults (Campo-Prieto et al. 2021 ). Personalized and innovative technology significantly enhances older adults’ participation, improving their health and well-being.
Cluster #3—Optimizing health management for older adults using mobile technology. With the development of mobile health (mHealth) and health information technology, mobile applications, smartphones, and smart wearable devices have become effective tools to help older users better manage chronic conditions, conduct real-time health monitoring, and even receive telehealth services (Dupuis and Tsotsos 2018 ; Olmedo-Aguirre et al. 2022 ; Kim et al. 2014 ). Additionally, these technologies can mitigate the problem of healthcare resource inequality, especially in developing countries. Older adults’ acceptance and use of these technologies are significantly influenced by their behavioral intentions, motivational factors, and self-management skills. These internal motivational factors, along with external factors, jointly affect older adults’ performance in health management and quality of life.
Cluster #4—Research on technology-assisted home care for older adults is gaining popularity. Environmentally assisted living enhances older adults’ independence and comfort at home, offering essential support and security. This has a crucial impact on promoting healthy aging (Friesen et al. 2016 ; Wahlroos et al. 2023 ). The smart home is a core application in this field, providing a range of solutions that facilitate independent living for the elderly in a highly integrated and user-friendly manner. This fulfills different dimensions of living and health needs (Majumder et al. 2017 ). Moreover, eHealth offers accurate and personalized health management and healthcare services for older adults (Delmastro et al. 2018 ), ensuring their needs are met at home. Research in this field often employs qualitative methods and structural equation modeling to fully understand older adults’ needs and experiences at home and analyze factors influencing technology adoption.
Evolutionary trends analysis
To gain a deeper understanding of the evolutionary trends in research hotspots within the field of older adults’ technology acceptance, we conducted a statistical analysis of the average appearance times of keywords, using CiteSpace to generate the time-zone evolution mapping (Fig. 8 ) and burst keywords. The time-zone mapping visually displays the evolution of keywords over time, intuitively reflecting the frequency and initial appearance of keywords in research, commonly used to identify trends in research topics (Jing et al. 2024a ; Kumar et al. 2021 ). Table 10 lists the top 15 keywords by burst strength, with the red sections indicating high-frequency citations and their burst strength in specific years. These burst keywords reveal the focus and trends of research themes over different periods (Kleinberg 2002 ). Combining insights from the time-zone mapping and burst keywords provides more objective and accurate research insights (Wang et al. 2023b ).
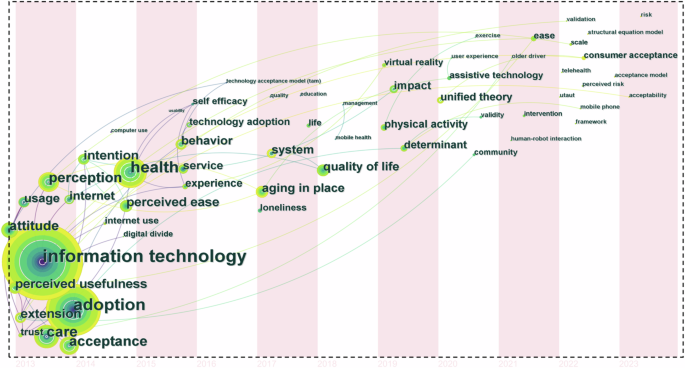
Reflecting the frequency and time of first appearance of keywords in the study.
An integrated analysis of Fig. 8 and Table 10 shows that early research on older adults’ technology acceptance primarily focused on factors such as perceived usefulness, ease of use, and attitudes towards information technology, including their use of computers and the internet (Pan and Jordan-Marsh 2010 ), as well as differences in technology use between older adults and other age groups (Guner and Acarturk 2020 ). Subsequently, the research focus expanded to improving the quality of life for older adults, exploring how technology can optimize health management and enhance the possibility of independent living, emphasizing the significant role of technology in improving the quality of life for the elderly. With ongoing technological advancements, recent research has shifted towards areas such as “virtual reality,” “telehealth,” and “human-robot interaction,” with a focus on the user experience of older adults (Halim et al. 2022 ). The appearance of keywords such as “physical activity” and “exercise” highlights the value of technology in promoting physical activity and health among older adults. This phase of research tends to make cutting-edge technology genuinely serve the practical needs of older adults, achieving its widespread application in daily life. Additionally, research has focused on expanding and quantifying theoretical models of older adults’ technology acceptance, involving keywords such as “perceived risk”, “validation” and “UTAUT”.
In summary, from 2013 to 2023, the field of older adults’ technology acceptance has evolved from initial explorations of influencing factors, to comprehensive enhancements in quality of life and health management, and further to the application and deepening of theoretical models and cutting-edge technologies. This research not only reflects the diversity and complexity of the field but also demonstrates a comprehensive and in-depth understanding of older adults’ interactions with technology across various life scenarios and needs.
Research quality distribution
To reveal the distribution of research quality in the field of older adults’ technology acceptance, a strategic diagram analysis is employed to calculate and illustrate the internal development and interrelationships among various research themes (Xie et al. 2020 ). The strategic diagram uses Centrality as the X-axis and Density as the Y-axis to divide into four quadrants, where the X-axis represents the strength of the connection between thematic clusters and other themes, with higher values indicating a central position in the research field; the Y-axis indicates the level of development within the thematic clusters, with higher values denoting a more mature and widely recognized field (Li and Zhou 2020 ).
Through cluster analysis and manual verification, this study categorized 61 core keywords (Frequency ≥5) into 11 thematic clusters. Subsequently, based on the keywords covered by each thematic cluster, the research themes and their directions for each cluster were summarized (Table 11 ), and the centrality and density coordinates for each cluster were precisely calculated (Table 12 ). Finally, a strategic diagram of the older adults’ technology acceptance research field was constructed (Fig. 9 ). Based on the distribution of thematic clusters across the quadrants in the strategic diagram, the structure and developmental trends of the field were interpreted.
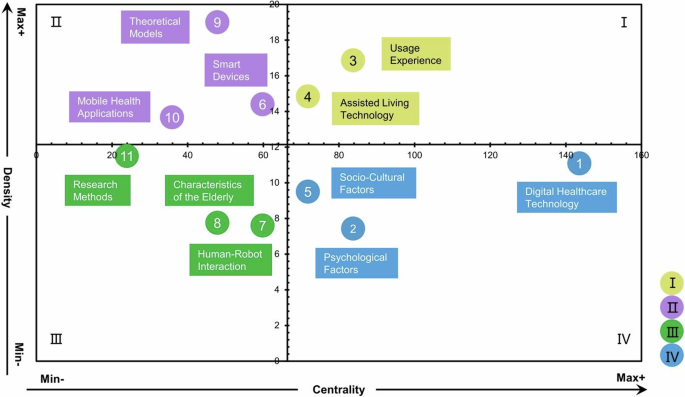
Classification and visualization of theme clusters based on density and centrality.
As illustrated in Fig. 9 , (1) the theme clusters of #3 Usage Experience and #4 Assisted Living Technology are in the first quadrant, characterized by high centrality and density. Their internal cohesion and close links with other themes indicate their mature development, systematic research content or directions have been formed, and they have a significant influence on other themes. These themes play a central role in the field of older adults’ technology acceptance and have promising prospects. (2) The theme clusters of #6 Smart Devices, #9 Theoretical Models, and #10 Mobile Health Applications are in the second quadrant, with higher density but lower centrality. These themes have strong internal connections but weaker external links, indicating that these three themes have received widespread attention from researchers and have been the subject of related research, but more as self-contained systems and exhibit independence. Therefore, future research should further explore in-depth cooperation and cross-application with other themes. (3) The theme clusters of #7 Human-Robot Interaction, #8 Characteristics of the Elderly, and #11 Research Methods are in the third quadrant, with lower centrality and density. These themes are loosely connected internally and have weak links with others, indicating their developmental immaturity. Compared to other topics, they belong to the lower attention edge and niche themes, and there is a need for further investigation. (4) The theme clusters of #1 Digital Healthcare Technology, #2 Psychological Factors, and #5 Socio-Cultural Factors are located in the fourth quadrant, with high centrality but low density. Although closely associated with other research themes, the internal cohesion within these clusters is relatively weak. This suggests that while these themes are closely linked to other research areas, their own development remains underdeveloped, indicating a core immaturity. Nevertheless, these themes are crucial within the research domain of elderly technology acceptance and possess significant potential for future exploration.
Discussion on distribution power (RQ1)
Over the past decade, academic interest and influence in the area of older adults’ technology acceptance have significantly increased. This trend is evidenced by a quantitative analysis of publication and citation volumes, particularly noticeable in 2019 and 2022, where there was a substantial rise in both metrics. The rise is closely linked to the widespread adoption of emerging technologies such as smart homes, wearable devices, and telemedicine among older adults. While these technologies have enhanced their quality of life, they also pose numerous challenges, sparking extensive research into their acceptance, usage behaviors, and influencing factors among the older adults (Pirzada et al. 2022 ; Garcia Reyes et al. 2023 ). Furthermore, the COVID-19 pandemic led to a surge in technology demand among older adults, especially in areas like medical consultation, online socialization, and health management, further highlighting the importance and challenges of technology. Health risks and social isolation have compelled older adults to rely on technology for daily activities, accelerating its adoption and application within this demographic. This phenomenon has made technology acceptance a critical issue, driving societal and academic focus on the study of technology acceptance among older adults.
The flow of knowledge at the level of high-output disciplines and journals, along with the primary publishing outlets, indicates the highly interdisciplinary nature of research into older adults’ technology acceptance. This reflects the complexity and breadth of issues related to older adults’ technology acceptance, necessitating the integration of multidisciplinary knowledge and approaches. Currently, research is primarily focused on medical health and human-computer interaction, demonstrating academic interest in improving health and quality of life for older adults and addressing the urgent needs related to their interactions with technology. In the field of medical health, research aims to provide advanced and innovative healthcare technologies and services to meet the challenges of an aging population while improving the quality of life for older adults (Abdi et al. 2020 ; Wilson et al. 2021 ). In the field of human-computer interaction, research is focused on developing smarter and more user-friendly interaction models to meet the needs of older adults in the digital age, enabling them to actively participate in social activities and enjoy a higher quality of life (Sayago, 2019 ). These studies are crucial for addressing the challenges faced by aging societies, providing increased support and opportunities for the health, welfare, and social participation of older adults.
Discussion on research power (RQ2)
This study analyzes leading countries and collaboration networks, core institutions and authors, revealing the global research landscape and distribution of research strength in the field of older adults’ technology acceptance, and presents quantitative data on global research trends. From the analysis of country distribution and collaborations, China and the USA hold dominant positions in this field, with developed countries like the UK, Germany, Italy, and the Netherlands also excelling in international cooperation and research influence. The significant investment in technological research and the focus on the technological needs of older adults by many developed countries reflect their rapidly aging societies, policy support, and resource allocation.
China is the only developing country that has become a major contributor in this field, indicating its growing research capabilities and high priority given to aging societies and technological innovation. Additionally, China has close collaborations with countries such as USA, the UK, and Malaysia, driven not only by technological research needs but also by shared challenges and complementarities in aging issues among these nations. For instance, the UK has extensive experience in social welfare and aging research, providing valuable theoretical guidance and practical experience. International collaborations, aimed at addressing the challenges of aging, integrate the strengths of various countries, advancing in-depth and widespread development in the research of technology acceptance among older adults.
At the institutional and author level, City University of Hong Kong leads in publication volume, with research teams led by Chan and Chen demonstrating significant academic activity and contributions. Their research primarily focuses on older adults’ acceptance and usage behaviors of various technologies, including smartphones, smart wearables, and social robots (Chen et al. 2015 ; Li et al. 2019 ; Ma et al. 2016 ). These studies, targeting specific needs and product characteristics of older adults, have developed new models of technology acceptance based on existing frameworks, enhancing the integration of these technologies into their daily lives and laying a foundation for further advancements in the field. Although Tilburg University has a smaller publication output, it holds significant influence in the field of older adults’ technology acceptance. Particularly, the high citation rate of Peek’s studies highlights their excellence in research. Peek extensively explored older adults’ acceptance and usage of home care technologies, revealing the complexity and dynamics of their technology use behaviors. His research spans from identifying systemic influencing factors (Peek et al. 2014 ; Peek et al. 2016 ), emphasizing familial impacts (Luijkx et al. 2015 ), to constructing comprehensive models (Peek et al. 2017 ), and examining the dynamics of long-term usage (Peek et al. 2019 ), fully reflecting the evolving technology landscape and the changing needs of older adults. Additionally, the ongoing contributions of researchers like Ziefle, Rogers, and Wouters in the field of older adults’ technology acceptance demonstrate their research influence and leadership. These researchers have significantly enriched the knowledge base in this area with their diverse perspectives. For instance, Ziefle has uncovered the complex attitudes of older adults towards technology usage, especially the trade-offs between privacy and security, and how different types of activities affect their privacy needs (Maidhof et al. 2023 ; Mujirishvili et al. 2023 ; Schomakers and Ziefle 2023 ; Wilkowska et al. 2022 ), reflecting a deep exploration and ongoing innovation in the field of older adults’ technology acceptance.
Discussion on knowledge base and thematic progress (RQ3)
Through co-citation analysis and systematic review of seminal literature, this study reveals the knowledge foundation and thematic progress in the field of older adults’ technology acceptance. Co-citation networks and cluster analyses illustrate the structural themes of the research, delineating the differentiation and boundaries within this field. Additionally, burst detection analysis offers a valuable perspective for understanding the thematic evolution in the field of technology acceptance among older adults. The development and innovation of theoretical models are foundational to this research. Researchers enhance the explanatory power of constructed models by deepening and expanding existing technology acceptance theories to address theoretical limitations. For instance, Heerink et al. ( 2010 ) modified and expanded the UTAUT model by integrating functional assessment and social interaction variables to create the almere model. This model significantly enhances the ability to explain the intentions of older users in utilizing assistive social agents and improves the explanation of actual usage behaviors. Additionally, Chen and Chan ( 2014 ) extended the TAM to include age-related health and capability features of older adults, creating the STAM, which substantially improves predictions of older adults’ technology usage behaviors. Personal attributes, health and capability features, and facilitating conditions have a direct impact on technology acceptance. These factors more effectively predict older adults’ technology usage behaviors than traditional attitudinal factors.
With the advancement of technology and the application of emerging technologies, new research topics have emerged, increasingly focusing on older adults’ acceptance and use of these technologies. Prior to this, the study by Mitzner et al. ( 2010 ) challenged the stereotype of older adults’ conservative attitudes towards technology, highlighting the central roles of usability and usefulness in the technology acceptance process. This discovery laid an important foundation for subsequent research. Research fields such as “smart home technology,” “social life,” and “customer service” are emerging, indicating a shift in focus towards the practical and social applications of technology in older adults’ lives. Research not only focuses on the technology itself but also on how these technologies integrate into older adults’ daily lives and how they can improve the quality of life through technology. For instance, studies such as those by Ma et al. ( 2016 ), Hoque and Sorwar ( 2017 ), and Li et al. ( 2019 ) have explored factors influencing older adults’ use of smartphones, mHealth, and smart wearable devices.
Furthermore, the diversification of research methodologies and innovation in evaluation techniques, such as the use of mixed methods, structural equation modeling (SEM), and neural network (NN) approaches, have enhanced the rigor and reliability of the findings, enabling more precise identification of the factors and mechanisms influencing technology acceptance. Talukder et al. ( 2020 ) employed an effective multimethodological strategy by integrating SEM and NN to leverage the complementary strengths of both approaches, thus overcoming their individual limitations and more accurately analyzing and predicting older adults’ acceptance of wearable health technologies (WHT). SEM is utilized to assess the determinants’ impact on the adoption of WHT, while neural network models validate SEM outcomes and predict the significance of key determinants. This combined approach not only boosts the models’ reliability and explanatory power but also provides a nuanced understanding of the motivations and barriers behind older adults’ acceptance of WHT, offering deep research insights.
Overall, co-citation analysis of the literature in the field of older adults’ technology acceptance has uncovered deeper theoretical modeling and empirical studies on emerging technologies, while emphasizing the importance of research methodological and evaluation innovations in understanding complex social science issues. These findings are crucial for guiding the design and marketing strategies of future technology products, especially in the rapidly growing market of older adults.
Discussion on research hotspots and evolutionary trends (RQ4)
By analyzing core keywords, we can gain deep insights into the hot topics, evolutionary trends, and quality distribution of research in the field of older adults’ technology acceptance. The frequent occurrence of the keywords “TAM” and “UTAUT” indicates that the applicability and theoretical extension of existing technology acceptance models among older adults remain a focal point in academia. This phenomenon underscores the enduring influence of the studies by Davis ( 1989 ) and Venkatesh et al. ( 2003 ), whose models provide a robust theoretical framework for explaining and predicting older adults’ acceptance and usage of emerging technologies. With the widespread application of artificial intelligence (AI) and big data technologies, these theoretical models have incorporated new variables such as perceived risk, trust, and privacy issues (Amin et al. 2024 ; Chen et al. 2024 ; Jing et al. 2024b ; Seibert et al. 2021 ; Wang et al. 2024b ), advancing the theoretical depth and empirical research in this field.
Keyword co-occurrence cluster analysis has revealed multiple research hotspots in the field, including factors influencing technology adoption, interactive experiences between older adults and assistive technologies, the application of mobile health technology in health management, and technology-assisted home care. These studies primarily focus on enhancing the quality of life and health management of older adults through emerging technologies, particularly in the areas of ambient assisted living, smart health monitoring, and intelligent medical care. In these domains, the role of AI technology is increasingly significant (Qian et al. 2021 ; Ho 2020 ). With the evolution of next-generation information technologies, AI is increasingly integrated into elder care systems, offering intelligent, efficient, and personalized service solutions by analyzing the lifestyles and health conditions of older adults. This integration aims to enhance older adults’ quality of life in aspects such as health monitoring and alerts, rehabilitation assistance, daily health management, and emotional support (Lee et al. 2023 ). A survey indicates that 83% of older adults prefer AI-driven solutions when selecting smart products, demonstrating the increasing acceptance of AI in elder care (Zhao and Li 2024 ). Integrating AI into elder care presents both opportunities and challenges, particularly in terms of user acceptance, trust, and long-term usage effects, which warrant further exploration (Mhlanga 2023 ). These studies will help better understand the profound impact of AI technology on the lifestyles of older adults and provide critical references for optimizing AI-driven elder care services.
The Time-zone evolution mapping and burst keyword analysis further reveal the evolutionary trends of research hotspots. Early studies focused on basic technology acceptance models and user perceptions, later expanding to include quality of life and health management. In recent years, research has increasingly focused on cutting-edge technologies such as virtual reality, telehealth, and human-robot interaction, with a concurrent emphasis on the user experience of older adults. This evolutionary process demonstrates a deepening shift from theoretical models to practical applications, underscoring the significant role of technology in enhancing the quality of life for older adults. Furthermore, the strategic coordinate mapping analysis clearly demonstrates the development and mutual influence of different research themes. High centrality and density in the themes of Usage Experience and Assisted Living Technology indicate their mature research status and significant impact on other themes. The themes of Smart Devices, Theoretical Models, and Mobile Health Applications demonstrate self-contained research trends. The themes of Human-Robot Interaction, Characteristics of the Elderly, and Research Methods are not yet mature, but they hold potential for development. Themes of Digital Healthcare Technology, Psychological Factors, and Socio-Cultural Factors are closely related to other themes, displaying core immaturity but significant potential.
In summary, the research hotspots in the field of older adults’ technology acceptance are diverse and dynamic, demonstrating the academic community’s profound understanding of how older adults interact with technology across various life contexts and needs. Under the influence of AI and big data, research should continue to focus on the application of emerging technologies among older adults, exploring in depth how they adapt to and effectively use these technologies. This not only enhances the quality of life and healthcare experiences for older adults but also drives ongoing innovation and development in this field.
Research agenda
Based on the above research findings, to further understand and promote technology acceptance and usage among older adults, we recommend future studies focus on refining theoretical models, exploring long-term usage, and assessing user experience in the following detailed aspects:
Refinement and validation of specific technology acceptance models for older adults: Future research should focus on developing and validating technology acceptance models based on individual characteristics, particularly considering variations in technology acceptance among older adults across different educational levels and cultural backgrounds. This includes factors such as age, gender, educational background, and cultural differences. Additionally, research should examine how well specific technologies, such as wearable devices and mobile health applications, meet the needs of older adults. Building on existing theoretical models, this research should integrate insights from multiple disciplines such as psychology, sociology, design, and engineering through interdisciplinary collaboration to create more accurate and comprehensive models, which should then be validated in relevant contexts.
Deepening the exploration of the relationship between long-term technology use and quality of life among older adults: The acceptance and use of technology by users is a complex and dynamic process (Seuwou et al. 2016 ). Existing research predominantly focuses on older adults’ initial acceptance or short-term use of new technologies; however, the impact of long-term use on their quality of life and health is more significant. Future research should focus on the evolution of older adults’ experiences and needs during long-term technology usage, and the enduring effects of technology on their social interactions, mental health, and life satisfaction. Through longitudinal studies and qualitative analysis, this research reveals the specific needs and challenges of older adults in long-term technology use, providing a basis for developing technologies and strategies that better meet their requirements. This understanding aids in comprehensively assessing the impact of technology on older adults’ quality of life and guiding the optimization and improvement of technological products.
Evaluating the Importance of User Experience in Research on Older Adults’ Technology Acceptance: Understanding the mechanisms of information technology acceptance and use is central to human-computer interaction research. Although technology acceptance models and user experience models differ in objectives, they share many potential intersections. Technology acceptance research focuses on structured prediction and assessment, while user experience research concentrates on interpreting design impacts and new frameworks. Integrating user experience to assess older adults’ acceptance of technology products and systems is crucial (Codfrey et al. 2022 ; Wang et al. 2019 ), particularly for older users, where specific product designs should emphasize practicality and usability (Fisk et al. 2020 ). Researchers need to explore innovative age-appropriate design methods to enhance older adults’ usage experience. This includes studying older users’ actual usage preferences and behaviors, optimizing user interfaces, and interaction designs. Integrating feedback from older adults to tailor products to their needs can further promote their acceptance and continued use of technology products.
Conclusions
This study conducted a systematic review of the literature on older adults’ technology acceptance over the past decade through bibliometric analysis, focusing on the distribution power, research power, knowledge base and theme progress, research hotspots, evolutionary trends, and quality distribution. Using a combination of quantitative and qualitative methods, this study has reached the following conclusions:
Technology acceptance among older adults has become a hot topic in the international academic community, involving the integration of knowledge across multiple disciplines, including Medical Informatics, Health Care Sciences Services, and Ergonomics. In terms of journals, “PSYCHOLOGY, EDUCATION, HEALTH” represents a leading field, with key publications including Computers in Human Behavior , Journal of Medical Internet Research , and International Journal of Human-Computer Interaction . These journals possess significant academic authority and extensive influence in the field.
Research on technology acceptance among older adults is particularly active in developed countries, with China and USA publishing significantly more than other nations. The Netherlands leads in high average citation rates, indicating the depth and impact of its research. Meanwhile, the UK stands out in terms of international collaboration. At the institutional level, City University of Hong Kong and The University of Hong Kong in China are in leading positions. Tilburg University in the Netherlands demonstrates exceptional research quality through its high average citation count. At the author level, Chen from China has the highest number of publications, while Peek from the Netherlands has the highest average citation count.
Co-citation analysis of references indicates that the knowledge base in this field is divided into three main categories: theoretical model deepening, emerging technology applications, and research methods and evaluation. Seminal literature focuses on four areas: specific technology use by older adults, expansion of theoretical models of technology acceptance, information technology adoption behavior, and research perspectives. Research themes have evolved from initial theoretical deepening and analysis of influencing factors to empirical studies on individual factors and emerging technologies.
Keyword analysis indicates that TAM and UTAUT are the most frequently occurring terms, while “assistive technology” and “virtual reality” are focal points with high frequency and centrality. Keyword clustering analysis reveals that research hotspots are concentrated on the influencing factors of technology adoption, human-robot interaction experiences, mobile health management, and technology for aging in place. Time-zone evolution mapping and burst keyword analysis have revealed the research evolution from preliminary exploration of influencing factors, to enhancements in quality of life and health management, and onto advanced technology applications and deepening of theoretical models. Furthermore, analysis of research quality distribution indicates that Usage Experience and Assisted Living Technology have become core topics, while Smart Devices, Theoretical Models, and Mobile Health Applications point towards future research directions.
Through this study, we have systematically reviewed the dynamics, core issues, and evolutionary trends in the field of older adults’ technology acceptance, constructing a comprehensive Knowledge Mapping of the domain and presenting a clear framework of existing research. This not only lays the foundation for subsequent theoretical discussions and innovative applications in the field but also provides an important reference for relevant scholars.
Limitations
To our knowledge, this is the first bibliometric analysis concerning technology acceptance among older adults, and we adhered strictly to bibliometric standards throughout our research. However, this study relies on the Web of Science Core Collection, and while its authority and breadth are widely recognized, this choice may have missed relevant literature published in other significant databases such as PubMed, Scopus, and Google Scholar, potentially overlooking some critical academic contributions. Moreover, given that our analysis was confined to literature in English, it may not reflect studies published in other languages, somewhat limiting the global representativeness of our data sample.
It is noteworthy that with the rapid development of AI technology, its increasingly widespread application in elderly care services is significantly transforming traditional care models. AI is profoundly altering the lifestyles of the elderly, from health monitoring and smart diagnostics to intelligent home systems and personalized care, significantly enhancing their quality of life and health care standards. The potential for AI technology within the elderly population is immense, and research in this area is rapidly expanding. However, due to the restrictive nature of the search terms used in this study, it did not fully cover research in this critical area, particularly in addressing key issues such as trust, privacy, and ethics.
Consequently, future research should not only expand data sources, incorporating multilingual and multidatabase literature, but also particularly focus on exploring older adults’ acceptance of AI technology and its applications, in order to construct a more comprehensive academic landscape of older adults’ technology acceptance, thereby enriching and extending the knowledge system and academic trends in this field.
Data availability
The datasets analyzed during the current study are available in the Dataverse repository: https://doi.org/10.7910/DVN/6K0GJH .
Abdi S, de Witte L, Hawley M (2020) Emerging technologies with potential care and support applications for older people: review of gray literature. JMIR Aging 3(2):e17286. https://doi.org/10.2196/17286
Article PubMed PubMed Central Google Scholar
Achuthan K, Nair VK, Kowalski R, Ramanathan S, Raman R (2023) Cyberbullying research—Alignment to sustainable development and impact of COVID-19: Bibliometrics and science mapping analysis. Comput Human Behav 140:107566. https://doi.org/10.1016/j.chb.2022.107566
Article Google Scholar
Ahmad A, Mozelius P (2022) Human-Computer Interaction for Older Adults: a Literature Review on Technology Acceptance of eHealth Systems. J Eng Res Sci 1(4):119–126. https://doi.org/10.55708/js0104014
Ale Ebrahim N, Salehi H, Embi MA, Habibi F, Gholizadeh H, Motahar SM (2014) Visibility and citation impact. Int Educ Stud 7(4):120–125. https://doi.org/10.5539/ies.v7n4p120
Amin MS, Johnson VL, Prybutok V, Koh CE (2024) An investigation into factors affecting the willingness to disclose personal health information when using AI-enabled caregiver robots. Ind Manag Data Syst 124(4):1677–1699. https://doi.org/10.1108/IMDS-09-2023-0608
Baer NR, Vietzke J, Schenk L (2022) Middle-aged and older adults’ acceptance of mobile nutrition and fitness apps: a systematic mixed studies review. PLoS One 17(12):e0278879. https://doi.org/10.1371/journal.pone.0278879
Barnard Y, Bradley MD, Hodgson F, Lloyd AD (2013) Learning to use new technologies by older adults: Perceived difficulties, experimentation behaviour and usability. Comput Human Behav 29(4):1715–1724. https://doi.org/10.1016/j.chb.2013.02.006
Berkowsky RW, Sharit J, Czaja SJ (2017) Factors predicting decisions about technology adoption among older adults. Innov Aging 3(1):igy002. https://doi.org/10.1093/geroni/igy002
Braun MT (2013) Obstacles to social networking website use among older adults. Comput Human Behav 29(3):673–680. https://doi.org/10.1016/j.chb.2012.12.004
Article MathSciNet Google Scholar
Campo-Prieto P, Rodríguez-Fuentes G, Cancela-Carral JM (2021) Immersive virtual reality exergame promotes the practice of physical activity in older people: An opportunity during COVID-19. Multimodal Technol Interact 5(9):52. https://doi.org/10.3390/mti5090052
Chen C (2006) CiteSpace II: Detecting and visualizing emerging trends and transient patterns in scientific literature. J Am Soc Inf Sci Technol 57(3):359–377. https://doi.org/10.1002/asi.20317
Chen C, Dubin R, Kim MC (2014) Emerging trends and new developments in regenerative medicine: a scientometric update (2000–2014). Expert Opin Biol Ther 14(9):1295–1317. https://doi.org/10.1517/14712598.2014.920813
Article PubMed Google Scholar
Chen C, Leydesdorff L (2014) Patterns of connections and movements in dual‐map overlays: A new method of publication portfolio analysis. J Assoc Inf Sci Technol 65(2):334–351. https://doi.org/10.1002/asi.22968
Chen J, Wang C, Tang Y (2022) Knowledge mapping of volunteer motivation: A bibliometric analysis and cross-cultural comparative study. Front Psychol 13:883150. https://doi.org/10.3389/fpsyg.2022.883150
Chen JY, Liu YD, Dai J, Wang CL (2023) Development and status of moral education research: Visual analysis based on knowledge graph. Front Psychol 13:1079955. https://doi.org/10.3389/fpsyg.2022.1079955
Chen K, Chan AH (2011) A review of technology acceptance by older adults. Gerontechnology 10(1):1–12. https://doi.org/10.4017/gt.2011.10.01.006.00
Chen K, Chan AH (2014) Gerontechnology acceptance by elderly Hong Kong Chinese: a senior technology acceptance model (STAM). Ergonomics 57(5):635–652. https://doi.org/10.1080/00140139.2014.895855
Chen K, Zhang Y, Fu X (2019) International research collaboration: An emerging domain of innovation studies? Res Policy 48(1):149–168. https://doi.org/10.1016/j.respol.2018.08.005
Chen X, Hu Z, Wang C (2024) Empowering education development through AIGC: A systematic literature review. Educ Inf Technol 1–53. https://doi.org/10.1007/s10639-024-12549-7
Chen Y, Chen CM, Liu ZY, Hu ZG, Wang XW (2015) The methodology function of CiteSpace mapping knowledge domains. Stud Sci Sci 33(2):242–253. https://doi.org/10.16192/j.cnki.1003-2053.2015.02.009
Codfrey GS, Baharum A, Zain NHM, Omar M, Deris FD (2022) User Experience in Product Design and Development: Perspectives and Strategies. Math Stat Eng Appl 71(2):257–262. https://doi.org/10.17762/msea.v71i2.83
Dai J, Zhang X, Wang CL (2024) A meta-analysis of learners’ continuance intention toward online education platforms. Educ Inf Technol 1–36. https://doi.org/10.1007/s10639-024-12654-7
Davis FD (1989) Perceived usefulness, perceived ease of use, and user acceptance of information technology. MIS Q 13(3):319–340. https://doi.org/10.2307/249008
Delmastro F, Dolciotti C, Palumbo F, Magrini M, Di Martino F, La Rosa D, Barcaro U (2018) Long-term care: how to improve the quality of life with mobile and e-health services. In 2018 14th International Conference on Wireless and Mobile Computing, Networking and Communications (WiMob), pp. 12–19. IEEE. https://doi.org/10.1109/WiMOB.2018.8589157
Dupuis K, Tsotsos LE (2018) Technology for remote health monitoring in an older population: a role for mobile devices. Multimodal Technol Interact 2(3):43. https://doi.org/10.3390/mti2030043
Ferguson C, Hickman LD, Turkmani S, Breen P, Gargiulo G, Inglis SC (2021) Wearables only work on patients that wear them”: Barriers and facilitators to the adoption of wearable cardiac monitoring technologies. Cardiovasc Digit Health J 2(2):137–147. https://doi.org/10.1016/j.cvdhj.2021.02.001
Fisk AD, Czaja SJ, Rogers WA, Charness N, Sharit J (2020) Designing for older adults: Principles and creative human factors approaches. CRC Press. https://doi.org/10.1201/9781420080681
Friesen S, Brémault-Phillips S, Rudrum L, Rogers LG (2016) Environmental design that supports healthy aging: Evaluating a new supportive living facility. J Hous Elderly 30(1):18–34. https://doi.org/10.1080/02763893.2015.1129380
Garcia Reyes EP, Kelly R, Buchanan G, Waycott J (2023) Understanding Older Adults’ Experiences With Technologies for Health Self-management: Interview Study. JMIR Aging 6:e43197. https://doi.org/10.2196/43197
Geng Z, Wang J, Liu J, Miao J (2024) Bibliometric analysis of the development, current status, and trends in adult degenerative scoliosis research: A systematic review from 1998 to 2023. J Pain Res 17:153–169. https://doi.org/10.2147/JPR.S437575
González A, Ramírez MP, Viadel V (2012) Attitudes of the elderly toward information and communications technologies. Educ Gerontol 38(9):585–594. https://doi.org/10.1080/03601277.2011.595314
Guner H, Acarturk C (2020) The use and acceptance of ICT by senior citizens: a comparison of technology acceptance model (TAM) for elderly and young adults. Univ Access Inf Soc 19(2):311–330. https://doi.org/10.1007/s10209-018-0642-4
Halim I, Saptari A, Perumal PA, Abdullah Z, Abdullah S, Muhammad MN (2022) A Review on Usability and User Experience of Assistive Social Robots for Older Persons. Int J Integr Eng 14(6):102–124. https://penerbit.uthm.edu.my/ojs/index.php/ijie/article/view/8566
He Y, He Q, Liu Q (2022) Technology acceptance in socially assistive robots: Scoping review of models, measurement, and influencing factors. J Healthc Eng 2022(1):6334732. https://doi.org/10.1155/2022/6334732
Heerink M, Kröse B, Evers V, Wielinga B (2010) Assessing acceptance of assistive social agent technology by older adults: the almere model. Int J Soc Robot 2:361–375. https://doi.org/10.1007/s12369-010-0068-5
Ho A (2020) Are we ready for artificial intelligence health monitoring in elder care? BMC Geriatr 20(1):358. https://doi.org/10.1186/s12877-020-01764-9
Hoque R, Sorwar G (2017) Understanding factors influencing the adoption of mHealth by the elderly: An extension of the UTAUT model. Int J Med Inform 101:75–84. https://doi.org/10.1016/j.ijmedinf.2017.02.002
Hota PK, Subramanian B, Narayanamurthy G (2020) Mapping the intellectual structure of social entrepreneurship research: A citation/co-citation analysis. J Bus Ethics 166(1):89–114. https://doi.org/10.1007/s10551-019-04129-4
Huang R, Yan P, Yang X (2021) Knowledge map visualization of technology hotspots and development trends in China’s textile manufacturing industry. IET Collab Intell Manuf 3(3):243–251. https://doi.org/10.1049/cim2.12024
Article ADS Google Scholar
Jing Y, Wang C, Chen Y, Wang H, Yu T, Shadiev R (2023) Bibliometric mapping techniques in educational technology research: A systematic literature review. Educ Inf Technol 1–29. https://doi.org/10.1007/s10639-023-12178-6
Jing YH, Wang CL, Chen ZY, Shen SS, Shadiev R (2024a) A Bibliometric Analysis of Studies on Technology-Supported Learning Environments: Hotopics and Frontier Evolution. J Comput Assist Learn 1–16. https://doi.org/10.1111/jcal.12934
Jing YH, Wang HM, Chen XJ, Wang CL (2024b) What factors will affect the effectiveness of using ChatGPT to solve programming problems? A quasi-experimental study. Humanit Soc Sci Commun 11:319. https://doi.org/10.1057/s41599-024-02751-w
Kamrani P, Dorsch I, Stock WG (2021) Do researchers know what the h-index is? And how do they estimate its importance? Scientometrics 126(7):5489–5508. https://doi.org/10.1007/s11192-021-03968-1
Kim HS, Lee KH, Kim H, Kim JH (2014) Using mobile phones in healthcare management for the elderly. Maturitas 79(4):381–388. https://doi.org/10.1016/j.maturitas.2014.08.013
Article MathSciNet PubMed Google Scholar
Kleinberg J (2002) Bursty and hierarchical structure in streams. In Proceedings of the eighth ACM SIGKDD international conference on Knowledge discovery and data mining, pp. 91–101. https://doi.org/10.1145/775047.775061
Kruse C, Fohn J, Wilson N, Patlan EN, Zipp S, Mileski M (2020) Utilization barriers and medical outcomes commensurate with the use of telehealth among older adults: systematic review. JMIR Med Inform 8(8):e20359. https://doi.org/10.2196/20359
Kumar S, Lim WM, Pandey N, Christopher Westland J (2021) 20 years of electronic commerce research. Electron Commer Res 21:1–40. https://doi.org/10.1007/s10660-021-09464-1
Kwiek M (2021) What large-scale publication and citation data tell us about international research collaboration in Europe: Changing national patterns in global contexts. Stud High Educ 46(12):2629–2649. https://doi.org/10.1080/03075079.2020.1749254
Lee C, Coughlin JF (2015) PERSPECTIVE: Older adults’ adoption of technology: an integrated approach to identifying determinants and barriers. J Prod Innov Manag 32(5):747–759. https://doi.org/10.1111/jpim.12176
Lee CH, Wang C, Fan X, Li F, Chen CH (2023) Artificial intelligence-enabled digital transformation in elderly healthcare field: scoping review. Adv Eng Inform 55:101874. https://doi.org/10.1016/j.aei.2023.101874
Leydesdorff L, Rafols I (2012) Interactive overlays: A new method for generating global journal maps from Web-of-Science data. J Informetr 6(2):318–332. https://doi.org/10.1016/j.joi.2011.11.003
Li J, Ma Q, Chan AH, Man S (2019) Health monitoring through wearable technologies for older adults: Smart wearables acceptance model. Appl Ergon 75:162–169. https://doi.org/10.1016/j.apergo.2018.10.006
Article ADS PubMed Google Scholar
Li X, Zhou D (2020) Product design requirement information visualization approach for intelligent manufacturing services. China Mech Eng 31(07):871, http://www.cmemo.org.cn/EN/Y2020/V31/I07/871
Google Scholar
Lin Y, Yu Z (2024a) An integrated bibliometric analysis and systematic review modelling students’ technostress in higher education. Behav Inf Technol 1–25. https://doi.org/10.1080/0144929X.2024.2332458
Lin Y, Yu Z (2024b) A bibliometric analysis of artificial intelligence chatbots in educational contexts. Interact Technol Smart Educ 21(2):189–213. https://doi.org/10.1108/ITSE-12-2022-0165
Liu L, Duffy VG (2023) Exploring the future development of Artificial Intelligence (AI) applications in chatbots: a bibliometric analysis. Int J Soc Robot 15(5):703–716. https://doi.org/10.1007/s12369-022-00956-0
Liu R, Li X, Chu J (2022) Evolution of applied variables in the research on technology acceptance of the elderly. In: International Conference on Human-Computer Interaction, Cham: Springer International Publishing, pp 500–520. https://doi.org/10.1007/978-3-031-05581-23_5
Luijkx K, Peek S, Wouters E (2015) “Grandma, you should do it—It’s cool” Older Adults and the Role of Family Members in Their Acceptance of Technology. Int J Environ Res Public Health 12(12):15470–15485. https://doi.org/10.3390/ijerph121214999
Lussier M, Lavoie M, Giroux S, Consel C, Guay M, Macoir J, Bier N (2018) Early detection of mild cognitive impairment with in-home monitoring sensor technologies using functional measures: a systematic review. IEEE J Biomed Health Inform 23(2):838–847. https://doi.org/10.1109/JBHI.2018.2834317
López-Robles JR, Otegi-Olaso JR, Porto Gomez I, Gamboa-Rosales NK, Gamboa-Rosales H, Robles-Berumen H (2018) Bibliometric network analysis to identify the intellectual structure and evolution of the big data research field. In: International Conference on Intelligent Data Engineering and Automated Learning, Cham: Springer International Publishing, pp 113–120. https://doi.org/10.1007/978-3-030-03496-2_13
Ma Q, Chan AH, Chen K (2016) Personal and other factors affecting acceptance of smartphone technology by older Chinese adults. Appl Ergon 54:62–71. https://doi.org/10.1016/j.apergo.2015.11.015
Ma Q, Chan AHS, Teh PL (2021) Insights into Older Adults’ Technology Acceptance through Meta-Analysis. Int J Hum-Comput Interact 37(11):1049–1062. https://doi.org/10.1080/10447318.2020.1865005
Macedo IM (2017) Predicting the acceptance and use of information and communication technology by older adults: An empirical examination of the revised UTAUT2. Comput Human Behav 75:935–948. https://doi.org/10.1016/j.chb.2017.06.013
Maidhof C, Offermann J, Ziefle M (2023) Eyes on privacy: acceptance of video-based AAL impacted by activities being filmed. Front Public Health 11:1186944. https://doi.org/10.3389/fpubh.2023.1186944
Majumder S, Aghayi E, Noferesti M, Memarzadeh-Tehran H, Mondal T, Pang Z, Deen MJ (2017) Smart homes for elderly healthcare—Recent advances and research challenges. Sensors 17(11):2496. https://doi.org/10.3390/s17112496
Article ADS PubMed PubMed Central Google Scholar
Mhlanga D (2023) Artificial Intelligence in elderly care: Navigating ethical and responsible AI adoption for seniors. Available at SSRN 4675564. 4675564 min) Identifying citation patterns of scientific breakthroughs: A perspective of dynamic citation process. Inf Process Manag 58(1):102428. https://doi.org/10.1016/j.ipm.2020.102428
Mitzner TL, Boron JB, Fausset CB, Adams AE, Charness N, Czaja SJ, Sharit J (2010) Older adults talk technology: Technology usage and attitudes. Comput Human Behav 26(6):1710–1721. https://doi.org/10.1016/j.chb.2010.06.020
Mitzner TL, Savla J, Boot WR, Sharit J, Charness N, Czaja SJ, Rogers WA (2019) Technology adoption by older adults: Findings from the PRISM trial. Gerontologist 59(1):34–44. https://doi.org/10.1093/geront/gny113
Mongeon P, Paul-Hus A (2016) The journal coverage of Web of Science and Scopus: a comparative analysis. Scientometrics 106:213–228. https://doi.org/10.1007/s11192-015-1765-5
Mostaghel R (2016) Innovation and technology for the elderly: Systematic literature review. J Bus Res 69(11):4896–4900. https://doi.org/10.1016/j.jbusres.2016.04.049
Mujirishvili T, Maidhof C, Florez-Revuelta F, Ziefle M, Richart-Martinez M, Cabrero-García J (2023) Acceptance and privacy perceptions toward video-based active and assisted living technologies: Scoping review. J Med Internet Res 25:e45297. https://doi.org/10.2196/45297
Naseri RNN, Azis SN, Abas N (2023) A Review of Technology Acceptance and Adoption Models in Consumer Study. FIRM J Manage Stud 8(2):188–199. https://doi.org/10.33021/firm.v8i2.4536
Nguyen UP, Hallinger P (2020) Assessing the distinctive contributions of Simulation & Gaming to the literature, 1970–2019: A bibliometric review. Simul Gaming 51(6):744–769. https://doi.org/10.1177/1046878120941569
Olmedo-Aguirre JO, Reyes-Campos J, Alor-Hernández G, Machorro-Cano I, Rodríguez-Mazahua L, Sánchez-Cervantes JL (2022) Remote healthcare for elderly people using wearables: A review. Biosensors 12(2):73. https://doi.org/10.3390/bios12020073
Pan S, Jordan-Marsh M (2010) Internet use intention and adoption among Chinese older adults: From the expanded technology acceptance model perspective. Comput Human Behav 26(5):1111–1119. https://doi.org/10.1016/j.chb.2010.03.015
Pan X, Yan E, Cui M, Hua W (2018) Examining the usage, citation, and diffusion patterns of bibliometric map software: A comparative study of three tools. J Informetr 12(2):481–493. https://doi.org/10.1016/j.joi.2018.03.005
Park JS, Kim NR, Han EJ (2018) Analysis of trends in science and technology using keyword network analysis. J Korea Ind Inf Syst Res 23(2):63–73. https://doi.org/10.9723/jksiis.2018.23.2.063
Peek ST, Luijkx KG, Rijnaard MD, Nieboer ME, Van Der Voort CS, Aarts S, Wouters EJ (2016) Older adults’ reasons for using technology while aging in place. Gerontology 62(2):226–237. https://doi.org/10.1159/000430949
Peek ST, Luijkx KG, Vrijhoef HJ, Nieboer ME, Aarts S, van der Voort CS, Wouters EJ (2017) Origins and consequences of technology acquirement by independent-living seniors: Towards an integrative model. BMC Geriatr 17:1–18. https://doi.org/10.1186/s12877-017-0582-5
Peek ST, Wouters EJ, Van Hoof J, Luijkx KG, Boeije HR, Vrijhoef HJ (2014) Factors influencing acceptance of technology for aging in place: a systematic review. Int J Med Inform 83(4):235–248. https://doi.org/10.1016/j.ijmedinf.2014.01.004
Peek STM, Luijkx KG, Vrijhoef HJM, Nieboer ME, Aarts S, Van Der Voort CS, Wouters EJM (2019) Understanding changes and stability in the long-term use of technologies by seniors who are aging in place: a dynamical framework. BMC Geriatr 19:1–13. https://doi.org/10.1186/s12877-019-1241-9
Perez AJ, Siddiqui F, Zeadally S, Lane D (2023) A review of IoT systems to enable independence for the elderly and disabled individuals. Internet Things 21:100653. https://doi.org/10.1016/j.iot.2022.100653
Piau A, Wild K, Mattek N, Kaye J (2019) Current state of digital biomarker technologies for real-life, home-based monitoring of cognitive function for mild cognitive impairment to mild Alzheimer disease and implications for clinical care: systematic review. J Med Internet Res 21(8):e12785. https://doi.org/10.2196/12785
Pirzada P, Wilde A, Doherty GH, Harris-Birtill D (2022) Ethics and acceptance of smart homes for older adults. Inform Health Soc Care 47(1):10–37. https://doi.org/10.1080/17538157.2021.1923500
Pranckutė R (2021) Web of Science (WoS) and Scopus: The titans of bibliographic information in today’s academic world. Publications 9(1):12. https://doi.org/10.3390/publications9010012
Qian K, Zhang Z, Yamamoto Y, Schuller BW (2021) Artificial intelligence internet of things for the elderly: From assisted living to health-care monitoring. IEEE Signal Process Mag 38(4):78–88. https://doi.org/10.1109/MSP.2021.3057298
Redner S (1998) How popular is your paper? An empirical study of the citation distribution. Eur Phys J B-Condens Matter Complex Syst 4(2):131–134. https://doi.org/10.1007/s100510050359
Sayago S (ed.) (2019) Perspectives on human-computer interaction research with older people. Switzerland: Springer International Publishing. https://doi.org/10.1007/978-3-030-06076-3
Schomakers EM, Ziefle M (2023) Privacy vs. security: trade-offs in the acceptance of smart technologies for aging-in-place. Int J Hum Comput Interact 39(5):1043–1058. https://doi.org/10.1080/10447318.2022.2078463
Schroeder T, Dodds L, Georgiou A, Gewald H, Siette J (2023) Older adults and new technology: Mapping review of the factors associated with older adults’ intention to adopt digital technologies. JMIR Aging 6(1):e44564. https://doi.org/10.2196/44564
Seibert K, Domhoff D, Bruch D, Schulte-Althoff M, Fürstenau D, Biessmann F, Wolf-Ostermann K (2021) Application scenarios for artificial intelligence in nursing care: rapid review. J Med Internet Res 23(11):e26522. https://doi.org/10.2196/26522
Seuwou P, Banissi E, Ubakanma G (2016) User acceptance of information technology: A critical review of technology acceptance models and the decision to invest in Information Security. In: Global Security, Safety and Sustainability-The Security Challenges of the Connected World: 11th International Conference, ICGS3 2017, London, UK, January 18-20, 2017, Proceedings 11:230-251. Springer International Publishing. https://doi.org/10.1007/978-3-319-51064-4_19
Shiau WL, Wang X, Zheng F (2023) What are the trend and core knowledge of information security? A citation and co-citation analysis. Inf Manag 60(3):103774. https://doi.org/10.1016/j.im.2023.103774
Sinha S, Verma A, Tiwari P (2021) Technology: Saving and enriching life during COVID-19. Front Psychol 12:647681. https://doi.org/10.3389/fpsyg.2021.647681
Soar J (2010) The potential of information and communication technologies to support ageing and independent living. Ann Telecommun 65:479–483. https://doi.org/10.1007/s12243-010-0167-1
Strotmann A, Zhao D (2012) Author name disambiguation: What difference does it make in author‐based citation analysis? J Am Soc Inf Sci Technol 63(9):1820–1833. https://doi.org/10.1002/asi.22695
Talukder MS, Sorwar G, Bao Y, Ahmed JU, Palash MAS (2020) Predicting antecedents of wearable healthcare technology acceptance by elderly: A combined SEM-Neural Network approach. Technol Forecast Soc Change 150:119793. https://doi.org/10.1016/j.techfore.2019.119793
Taskin Z, Al U (2019) Natural language processing applications in library and information science. Online Inf Rev 43(4):676–690. https://doi.org/10.1108/oir-07-2018-0217
Touqeer H, Zaman S, Amin R, Hussain M, Al-Turjman F, Bilal M (2021) Smart home security: challenges, issues and solutions at different IoT layers. J Supercomput 77(12):14053–14089. https://doi.org/10.1007/s11227-021-03825-1
United Nations Department of Economic and Social Affairs (2023) World population ageing 2023: Highlights. https://www.un.org/zh/193220
Valk CAL, Lu Y, Randriambelonoro M, Jessen J (2018) Designing for technology acceptance of wearable and mobile technologies for senior citizen users. In: 21st DMI: Academic Design Management Conference (ADMC 2018), Design Management Institute, pp 1361–1373. https://www.dmi.org/page/ADMC2018
Van Eck N, Waltman L (2010) Software survey: VOSviewer, a computer program for bibliometric mapping. Scientometrics 84(2):523–538. https://doi.org/10.1007/s11192-009-0146-3
Vancea M, Solé-Casals J (2016) Population aging in the European Information Societies: towards a comprehensive research agenda in eHealth innovations for elderly. Aging Dis 7(4):526. https://doi.org/10.14336/AD.2015.1214
Venkatesh V, Morris MG, Davis GB, Davis FD (2003) User acceptance of information technology: Toward a unified view. MIS Q 27(3):425–478. https://doi.org/10.2307/30036540
Wagner N, Hassanein K, Head M (2010) Computer use by older adults: A multi-disciplinary review. Comput Human Behav 26(5):870–882. https://doi.org/10.1016/j.chb.2010.03.029
Wahlroos N, Narsakka N, Stolt M, Suhonen R (2023) Physical environment maintaining independence and self-management of older people in long-term care settings—An integrative literature review. J Aging Environ 37(3):295–313. https://doi.org/10.1080/26892618.2022.2092927
Wang CL, Chen XJ, Yu T, Liu YD, Jing YH (2024a) Education reform and change driven by digital technology: a bibliometric study from a global perspective. Humanit Soc Sci Commun 11(1):1–17. https://doi.org/10.1057/s41599-024-02717-y
Wang CL, Dai J, Zhu KK, Yu T, Gu XQ (2023a) Understanding the Continuance Intention of College Students Toward New E-learning Spaces Based on an Integrated Model of the TAM and TTF. Int J Hum-comput Int 1–14. https://doi.org/10.1080/10447318.2023.2291609
Wang CL, Wang HM, Li YY, Dai J, Gu XQ, Yu T (2024b) Factors Influencing University Students’ Behavioral Intention to Use Generative Artificial Intelligence: Integrating the Theory of Planned Behavior and AI Literacy. Int J Hum-comput Int 1–23. https://doi.org/10.1080/10447318.2024.2383033
Wang J, Zhao W, Zhang Z, Liu X, Xie T, Wang L, Zhang Y (2024c) A journey of challenges and victories: a bibliometric worldview of nanomedicine since the 21st century. Adv Mater 36(15):2308915. https://doi.org/10.1002/adma.202308915
Wang J, Chen Y, Huo S, Mai L, Jia F (2023b) Research hotspots and trends of social robot interaction design: A bibliometric analysis. Sensors 23(23):9369. https://doi.org/10.3390/s23239369
Wang KH, Chen G, Chen HG (2017) A model of technology adoption by older adults. Soc Behav Personal 45(4):563–572. https://doi.org/10.2224/sbp.5778
Wang S, Bolling K, Mao W, Reichstadt J, Jeste D, Kim HC, Nebeker C (2019) Technology to Support Aging in Place: Older Adults’ Perspectives. Healthcare 7(2):60. https://doi.org/10.3390/healthcare7020060
Wang Z, Liu D, Sun Y, Pang X, Sun P, Lin F, Ren K (2022) A survey on IoT-enabled home automation systems: Attacks and defenses. IEEE Commun Surv Tutor 24(4):2292–2328. https://doi.org/10.1109/COMST.2022.3201557
Wilkowska W, Offermann J, Spinsante S, Poli A, Ziefle M (2022) Analyzing technology acceptance and perception of privacy in ambient assisted living for using sensor-based technologies. PloS One 17(7):e0269642. https://doi.org/10.1371/journal.pone.0269642
Wilson J, Heinsch M, Betts D, Booth D, Kay-Lambkin F (2021) Barriers and facilitators to the use of e-health by older adults: a scoping review. BMC Public Health 21:1–12. https://doi.org/10.1186/s12889-021-11623-w
Xia YQ, Deng YL, Tao XY, Zhang SN, Wang CL (2024) Digital art exhibitions and psychological well-being in Chinese Generation Z: An analysis based on the S-O-R framework. Humanit Soc Sci Commun 11:266. https://doi.org/10.1057/s41599-024-02718-x
Xie H, Zhang Y, Duan K (2020) Evolutionary overview of urban expansion based on bibliometric analysis in Web of Science from 1990 to 2019. Habitat Int 95:102100. https://doi.org/10.1016/j.habitatint.2019.10210
Xu Z, Ge Z, Wang X, Skare M (2021) Bibliometric analysis of technology adoption literature published from 1997 to 2020. Technol Forecast Soc Change 170:120896. https://doi.org/10.1016/j.techfore.2021.120896
Yap YY, Tan SH, Choon SW (2022) Elderly’s intention to use technologies: a systematic literature review. Heliyon 8(1). https://doi.org/10.1016/j.heliyon.2022.e08765
Yu T, Dai J, Wang CL (2023) Adoption of blended learning: Chinese university students’ perspectives. Humanit Soc Sci Commun 10:390. https://doi.org/10.1057/s41599-023-01904-7
Yusif S, Soar J, Hafeez-Baig A (2016) Older people, assistive technologies, and the barriers to adoption: A systematic review. Int J Med Inform 94:112–116. https://doi.org/10.1016/j.ijmedinf.2016.07.004
Zhang J, Zhu L (2022) Citation recommendation using semantic representation of cited papers’ relations and content. Expert Syst Appl 187:115826. https://doi.org/10.1016/j.eswa.2021.115826
Zhao Y, Li J (2024) Opportunities and challenges of integrating artificial intelligence in China’s elderly care services. Sci Rep 14(1):9254. https://doi.org/10.1038/s41598-024-60067-w
Article ADS MathSciNet PubMed PubMed Central Google Scholar
Download references
Acknowledgements
This research was supported by the Social Science Foundation of Shaanxi Province in China (Grant No. 2023J014).
Author information
Authors and affiliations.
School of Art and Design, Shaanxi University of Science and Technology, Xi’an, China
Xianru Shang, Zijian Liu, Chen Gong, Zhigang Hu & Yuexuan Wu
Department of Education Information Technology, Faculty of Education, East China Normal University, Shanghai, China
Chengliang Wang
You can also search for this author in PubMed Google Scholar
Contributions
Conceptualization, XS, YW, CW; methodology, XS, ZL, CG, CW; software, XS, CG, YW; writing-original draft preparation, XS, CW; writing-review and editing, XS, CG, ZH, CW; supervision, ZL, ZH, CW; project administration, ZL, ZH, CW; funding acquisition, XS, CG. All authors read and approved the final manuscript. All authors have read and approved the re-submission of the manuscript.
Corresponding author
Correspondence to Chengliang Wang .
Ethics declarations
Competing interests.
The authors declare no competing interests.
Ethical approval
Ethical approval was not required as the study did not involve human participants.
Informed consent
Informed consent was not required as the study did not involve human participants.
Additional information
Publisher’s note Springer Nature remains neutral with regard to jurisdictional claims in published maps and institutional affiliations.
Rights and permissions
Open Access This article is licensed under a Creative Commons Attribution-NonCommercial-NoDerivatives 4.0 International License, which permits any non-commercial use, sharing, distribution and reproduction in any medium or format, as long as you give appropriate credit to the original author(s) and the source, provide a link to the Creative Commons licence, and indicate if you modified the licensed material. You do not have permission under this licence to share adapted material derived from this article or parts of it. The images or other third party material in this article are included in the article’s Creative Commons licence, unless indicated otherwise in a credit line to the material. If material is not included in the article’s Creative Commons licence and your intended use is not permitted by statutory regulation or exceeds the permitted use, you will need to obtain permission directly from the copyright holder. To view a copy of this licence, visit http://creativecommons.org/licenses/by-nc-nd/4.0/ .
Reprints and permissions
About this article
Cite this article.
Shang, X., Liu, Z., Gong, C. et al. Knowledge mapping and evolution of research on older adults’ technology acceptance: a bibliometric study from 2013 to 2023. Humanit Soc Sci Commun 11 , 1115 (2024). https://doi.org/10.1057/s41599-024-03658-2
Download citation
Received : 20 June 2024
Accepted : 21 August 2024
Published : 31 August 2024
DOI : https://doi.org/10.1057/s41599-024-03658-2
Share this article
Anyone you share the following link with will be able to read this content:
Sorry, a shareable link is not currently available for this article.
Provided by the Springer Nature SharedIt content-sharing initiative
Quick links
- Explore articles by subject
- Guide to authors
- Editorial policies

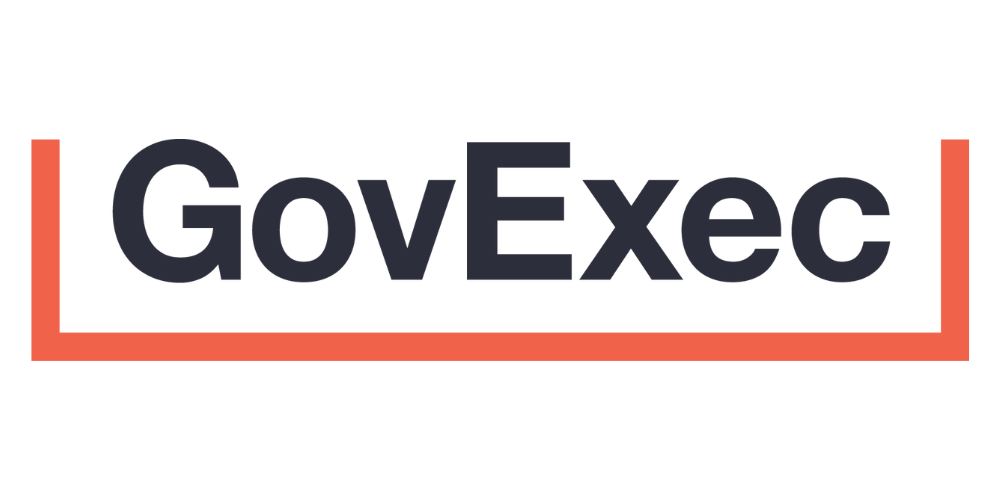
Unlocking GenAI’s potential to transform government operations
Artificial intelligence represents a significant step for federal agencies, promising enhancements to data processes, improved cybersecurity and streamlined citizen experiences. but where do agencies currently stand with the technology this infographic highlights key insights shared by public and private sector experts during a recent webinar, as well as findings from a recent govexec insights and research group (irg) report. the survey polled 100 decision-makers and influencers in federal civilian, defense, and intelligence sectors about ongoing genai initiatives in government..
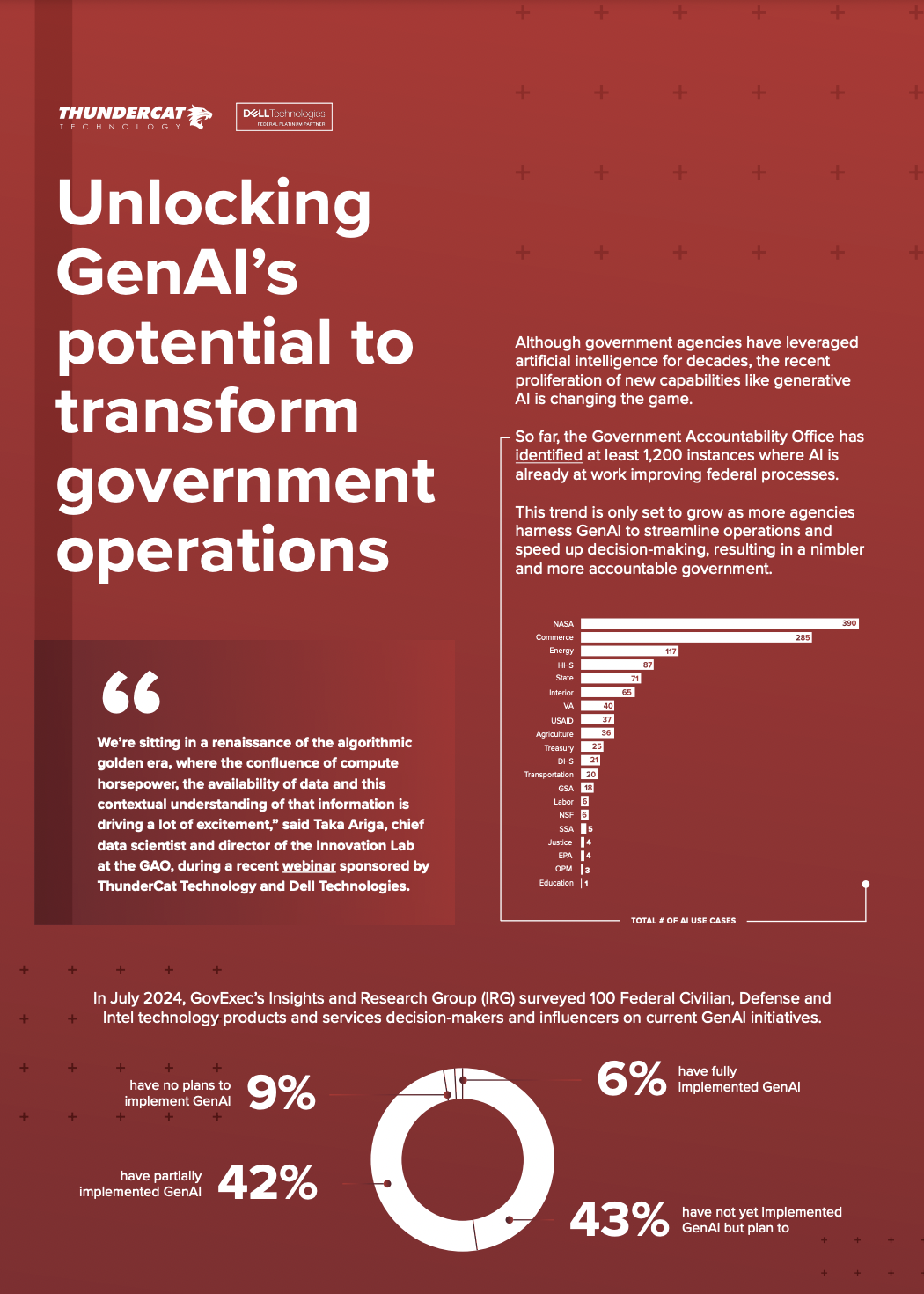
This site is protected by reCAPTCHA and the Google Privacy Policy and Terms and Conditions apply.
- Notice at Collection
- Your Privacy Choices
- Exercise Your Privacy Rights

An official website of the United States government
Here’s how you know
Official websites use .gov A .gov website belongs to an official government organization in the United States.
Secure .gov websites use HTTPS A lock ( Lock A locked padlock ) or https:// means you’ve safely connected to the .gov website. Share sensitive information only on official, secure websites.
https://www.nist.gov/news-events/news/2024/08/us-ai-safety-institute-signs-agreements-regarding-ai-safety-research
U.S. AI Safety Institute Signs Agreements Regarding AI Safety Research, Testing and Evaluation With Anthropic and OpenAI
These first-of-their-kind agreements between the u.s. government and industry will help advance safe and trustworthy ai innovation for all..
GAITHERSBURG, Md. — Today, the U.S. Artificial Intelligence Safety Institute at the U.S. Department of Commerce’s National Institute of Standards and Technology (NIST) announced agreements that enable formal collaboration on AI safety research, testing and evaluation with both Anthropic and OpenAI.
Each company’s Memorandum of Understanding establishes the framework for the U.S. AI Safety Institute to receive access to major new models from each company prior to and following their public release. The agreements will enable collaborative research on how to evaluate capabilities and safety risks, as well as methods to mitigate those risks.
“Safety is essential to fueling breakthrough technological innovation. With these agreements in place, we look forward to beginning our technical collaborations with Anthropic and OpenAI to advance the science of AI safety,” said Elizabeth Kelly, director of the U.S. AI Safety Institute. “These agreements are just the start, but they are an important milestone as we work to help responsibly steward the future of AI.”
Additionally, the U.S. AI Safety Institute plans to provide feedback to Anthropic and OpenAI on potential safety improvements to their models, in close collaboration with its partners at the U.K. AI Safety Institute.
The U.S. AI Safety Institute builds on NIST’s more than 120-year legacy of advancing measurement science, technology, standards and related tools. Evaluations under these agreements will further NIST’s work on AI by facilitating deep collaboration and exploratory research on advanced AI systems across a range of risk areas.
Evaluations conducted pursuant to these agreements will help advance the safe, secure and trustworthy development and use of AI by building on the Biden-Harris administration’s Executive Order on AI and the voluntary commitments made to the administration by leading AI model developers.
About the U.S. AI Safety Institute
The U.S. AI Safety Institute , located within the Department of Commerce at the National Institute of Standards and Technology (NIST), was established following the Biden-Harris administration’s 2023 Executive Order on the Safe, Secure, and Trustworthy Development and Use of Artificial Intelligence to advance the science of AI safety and address the risks posed by advanced AI systems. It is tasked with developing the testing, evaluations and guidelines that will help accelerate safe AI innovation here in the United States and around the world.
Information
- Author Services
Initiatives
You are accessing a machine-readable page. In order to be human-readable, please install an RSS reader.
All articles published by MDPI are made immediately available worldwide under an open access license. No special permission is required to reuse all or part of the article published by MDPI, including figures and tables. For articles published under an open access Creative Common CC BY license, any part of the article may be reused without permission provided that the original article is clearly cited. For more information, please refer to https://www.mdpi.com/openaccess .
Feature papers represent the most advanced research with significant potential for high impact in the field. A Feature Paper should be a substantial original Article that involves several techniques or approaches, provides an outlook for future research directions and describes possible research applications.
Feature papers are submitted upon individual invitation or recommendation by the scientific editors and must receive positive feedback from the reviewers.
Editor’s Choice articles are based on recommendations by the scientific editors of MDPI journals from around the world. Editors select a small number of articles recently published in the journal that they believe will be particularly interesting to readers, or important in the respective research area. The aim is to provide a snapshot of some of the most exciting work published in the various research areas of the journal.
Original Submission Date Received: .
- Active Journals
- Find a Journal
- Proceedings Series
- For Authors
- For Reviewers
- For Editors
- For Librarians
- For Publishers
- For Societies
- For Conference Organizers
- Open Access Policy
- Institutional Open Access Program
- Special Issues Guidelines
- Editorial Process
- Research and Publication Ethics
- Article Processing Charges
- Testimonials
- Preprints.org
- SciProfiles
- Encyclopedia
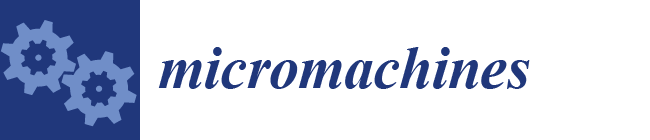
Article Menu
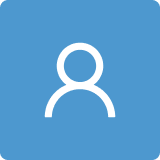
- Subscribe SciFeed
- Recommended Articles
- Google Scholar
- on Google Scholar
- Table of Contents
Find support for a specific problem in the support section of our website.
Please let us know what you think of our products and services.
Visit our dedicated information section to learn more about MDPI.
JSmol Viewer
Current research status and future trends of vibration energy harvesters.
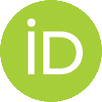
1. Introduction
2. electromagnetic vibration energy harvester, 2.1. working principle and characteristics of the electromagnetic vibration energy harvester, 2.2. advances in electromagnetic vibration energy harvesters, 3. piezoelectric vibration energy harvester, 3.1. operating principle and characteristics of piezoelectric vibration energy harvester, 3.2. progress of research on piezoelectric vibration energy harvesters, 4. friction electric vibration energy harvester, 4.1. mechanism of operation and characteristics of the friction electric vibration energy harvesters, 4.1.1. friction nanogenerator, 4.1.2. principle of friction electric vibration energy harvester, 4.2. advances in friction electric vibration energy harvesters, 5. electrostatic vibration energy harvester, 5.1. the working principle of the electrostatic vibration energy harvester and its characteristics, 5.2. current research status of electrostatic vibration energy harvesters, 6. magnetostrictive vibration energy harvester, 6.1. operating principle and characteristics of magnetostrictive vibration energy harvesters, 6.2. current status of research on magnetostrictive vibration energy harvesters, 7. conclusions, author contributions, institutional review board statement, informed consent statement, data availability statement, conflicts of interest.
- Bouhedma, S.; Bin Taufik, J.; Lange, F.; Ouali, M.; Seitz, H.; Hohlfeld, D. Different Scenarios of Autonomous Operation of an Environmental Sensor Node Using a Piezoelectric-Vibration-Based Energy Harvester. Sensors 2024 , 24 , 1338. [ Google Scholar ] [ CrossRef ] [ PubMed ]
- Wang, X.; Yin, G.; Sun, T.; Xu, X.; Rasool, G.; Abbas, K. Mechanical Vibration Energy Harvesting and Vibration Monitoring Based on Triboelectric Nanogenerators. Energy Technol. 2024 , 12 , 2300931. [ Google Scholar ] [ CrossRef ]
- Yue, O.; Wang, X.; Xie, L.; Bai, Z.; Zou, X.; Liu, X. Biomimetic Exogenous “Tissue Batteries” as Artificial Power Sources for Implantable Bioelectronic Devices Manufacturing. Adv. Sci. 2024 , 11 , e2307369. [ Google Scholar ] [ CrossRef ]
- Zhang, Y.; Wang, Z.J.; Jiang, S.L.; Wang, Q. Retrospectives and Perspectives of Vibration Energy Harvest Technologies. Mech. Sci. Technol. 2019 , 38 , 985–1018. [ Google Scholar ] [ CrossRef ]
- Ke, Z.; Deng, Z.; Ren, T.; Liu, X.; Yi, H.; Liu, Z.; Li, H.; Sun, Y. Simultaneous vibration suppression and energy harvesting system design via electromagnetic shunt damper for high temperature superconducting pinning maglev. Mech. Syst. Signal Process 2024 , 214 , 111374. [ Google Scholar ] [ CrossRef ]
- Zhao, L.; Zhang, H.; Liu, D.; Zou, Y.; Li, Z.; Liu, B. Application of nanogenerators in self-powered microfluidic systems. Nano Energy 2024 , 123 , 109432. [ Google Scholar ] [ CrossRef ]
- Christenson, G.N.; Yu, Z.; Frontiera, R.R. Wavelength Dependence of Plasmon-Induced Vibrational Energy Transfer in Fluorophore–Plasmonic Systems. J. Phys. Chem. 2024 , 128 , 10784–10789. [ Google Scholar ] [ CrossRef ]
- Qi, Y.; Zhao, J.; Zhang, C. Review and Prospect of Micro-nano Vibration Energy Harvesters. J. Mech. Eng. 2020 , 56 , 1–15. [ Google Scholar ]
- Huo, S.; Wang, P.; Long, H.; Ren, Z.; Yi, Q.; Dai, J.; An, B.; Wang, P.; Wang, Y.; Gao, M.; et al. Dual-mode electromagnetic energy harvester by Halbach arrays. Energy Convers. Manag. 2023 , 286 , 117038. [ Google Scholar ] [ CrossRef ]
- Liu, C.; Meng, A.; Chen, W.; Li, H.; Song, H. Research status and development trend of vibration energy harvesting technology. Equip. Manuf. Technol. 2013 , 12 , 43–47. [ Google Scholar ]
- Qi, L.; Song, J.; Wang, Y.; Yi, M.; Zhang, Z.; Yan, J. Mechanical motion rectification-based electromagnetic vibration energy harvesting technology: A review. Energy 2024 , 289 , 130030. [ Google Scholar ] [ CrossRef ]
- Hadas, Z.; Vetiska, V.; Vetiska, J.; Krejsa, J. Analysis and efficiency measurement of electromagnetic vibration energy harvesting system. Microsyst. Technol. 2016 , 22 , 1767–1779. [ Google Scholar ] [ CrossRef ]
- Choi, W.K.; Kim, H.J.; Park, C.W.; Lee, B. Study on Electromagnetic Energy Transducer in Ambient Vibration. IET Power Electron. 2018 , 11 , 1983–1990. [ Google Scholar ] [ CrossRef ]
- Wang, Y.; Gao, Y.; Chen, Z.; Zong, R.; Li, Y.; Guo, R.; Azam, A.; Qi, L.; Zhang, Z. Performance analysis of electromagnetic vibration energy harvester under square excitation. Int. J. Mech. Sci. 2024 , 271 , 109127. [ Google Scholar ] [ CrossRef ]
- Peng, Y.; Zhang, L.; Li, Z.; Zhong, S.; Liu, Y.; Xie, S.; Luo, J. Influences of Wire Diameters on Output Power in Electromagnetic Energy Harvester. Int. J. Precis. Eng. Manuf. Green Technol. 2022 , 10 , 205–216. [ Google Scholar ] [ CrossRef ]
- Lo Monaco, M.; Russo, C.; Somà, A. Identification Procedure for Design Optimization of Gravitational Electromagnetic Energy Harvesters. Appl. Sci. 2023 , 13 , 2736. [ Google Scholar ] [ CrossRef ]
- Sun, H.; Peng, H.; Xiao, H.; Liu, X.; Chen, Y.; Gao, K.; Wang, S.; Xu, P. An Optimized Design of Compact Self-Powered Module Based on Electromagnetic Vibration Energy Harvester Considering Engineering Feasibility. IEEE Trans. Ind. Appl. 2023 , 59 , 767–778. [ Google Scholar ] [ CrossRef ]
- Ghouli, Z.; Hamdi, M.; Belhaq, M. Energy harvesting from quasi-periodic vibrations using electromagnetic coupling with delay. Nonlinear Dyn. 2017 , 89 , 1625–1636. [ Google Scholar ] [ CrossRef ]
- Nicolini, L.; Castagnetti, D.; Sorrentino, A. A tunable multi-arm electromagnetic pendulum for ultra-low frequency vibration energy harvesting. Smart Mater. Struct. 2022 , 31 , 115009. [ Google Scholar ] [ CrossRef ]
- Xiong, L.; Gao, S.; Jin, L.; Sun, Y.; Du, X.; Liu, F. Study on the Influence of Coil Arrangement on the Output Characteristics of Electromagnetic Galloping Energy Harvester. Micromachines 2023 , 14 , 2158. [ Google Scholar ] [ CrossRef ]
- Sun, R.; Zhou, S.; Li, Z.; Cheng, L. Dual electromagnetic mechanisms with internal resonance for ultra-low frequency vibration energy harvesting. Appl. Energy 2024 , 369 , 123528. [ Google Scholar ] [ CrossRef ]
- Kumar, A.; Kiran, R.; Chauhan, V.S.; Kumar, R.; Vaish, R. Piezoelectric energy harvester for pacemaker application: A comparative study. Mater. Res. Express 2018 , 5 , 075701. [ Google Scholar ] [ CrossRef ]
- Zhang, Y. Sandwich Piezoelectric Transducer and Its Applications ; Science Press: Beijing, China, 2006. [ Google Scholar ]
- Zhang, Y. Key Technology Study of Mass-Sharing Cantilever Array Based Micro Piezoelectric Vibration Energy Harvester. Master’s Thesis, Chongqing University, Chongqing, China, 2016. [ Google Scholar ]
- Galassi, C.; Roncari, E.; Capiani, C.; Craciun, F. Processing and characterization of high Qm ferroelectric ceramics. J. Eur. Ceram. Soc. 1999 , 19 , 1237–1241. [ Google Scholar ] [ CrossRef ]
- Fang, H.-B.; Liu, J.Q.; Xu, Z.Y.; Dong, L.; Wang, L.; Chen, D.; Cai, B.C.; Liu, Y. Fabrication and performance of MEMS-based piezoelectric power generator for vibration energy harvesting. Microelectron. J. 2006 , 37 , 1280–1284. [ Google Scholar ] [ CrossRef ]
- Shen, D.; Park, J.H.; Ajitsaria, J.; Choe, S.Y.; Wikle, H.C.; Kim, D.J. The design, fabrication and evaluation of a MEMS PZT cantilever with an integrated Si proof mass for vibration energy harvesting. J. Micromech. Microeng. 2008 , 18 , 055017. [ Google Scholar ] [ CrossRef ]
- Ye, J.; Ding, G.; Wu, X.; Zhou, M.; Wang, J.; Chen, Y.; Yu, Y. Development and performance research of PSN-PZT piezoelectric ceramics based on road vibration energy harvesting technology. Mater. Today Commun. 2023 , 34 , 105135. [ Google Scholar ] [ CrossRef ]
- Xiang, Z.Y.; Zhang, J.K.; Li, S.J.; Xie, S.L.; Liu, F.P.; Zhu, R.D.; He, D.K. Friction-induced vibration energy harvesting via a piezoelectric cantilever vibration energy collector. Tribol. Int. 2023 , 189 , 108933. [ Google Scholar ] [ CrossRef ]
- Zhang, Y.; Zhang, G.; Wang, W. A piezoelectric cantilever-beam-spring-pendulum oscillator for multi-directional vibration energy harvesting. Commun. Nonlinear Sci. Numer. Simul. 2024 , 138 , 108199. [ Google Scholar ] [ CrossRef ]
- Wang, J.; Han, C.; Jo, S.-H.; Xu, W.; Tian, H. Enhanced flow induced vibration piezoelectric energy harvesting performance by optimizing tapered beam. Ocean Eng. 2024 , 300 , 117459. [ Google Scholar ] [ CrossRef ]
- Man, D.; Jiang, B.; Xu, Q.; Tang, L.; Zhang, Y.; Xu, G.; Han, T. Enhancing low-orbit vibration energy harvesting by a tri-stable piezoelectric energy harvester with an innovative dynamic amplifier. AIP Adv. 2024 , 14 , 045210. [ Google Scholar ] [ CrossRef ]
- Cho, K.-H.; Park, H.-Y.; Heo, J.S.; Priya, S. Structure—Performance relationships for cantilever-type piezoelectric energy harvesters. J. Appl. Phys. 2014 , 115 , 204108. [ Google Scholar ] [ CrossRef ]
- Lee, B.S.; Lin, S.C.; Wu, W.J.; Wang, X.Y.; Chang, P.Z.; Lee, C.K. Piezoelectric MEMS generators fabricated with an aerosol deposition PZT thin film. J. Micromech. Microeng. 2009 , 19 , 065014. [ Google Scholar ] [ CrossRef ]
- Nair, R.; Tripathi, B.; Jain, A.; Shehata, N. Vibrational energy harvesting and tactile sensing applications based on PVDF-TPU piezoelectric nanofibers. J. Mater. Sci. Mater. Electron. 2024 , 35 , 857. [ Google Scholar ] [ CrossRef ]
- Ramírez, J.M.; Gatti, C.D.; Machado, S.P.; Febbo, M. A multi-modal energy harvesting device for low-frequency vibrations. Extrem. Mech. Lett. 2018 , 22 , 1–7. [ Google Scholar ] [ CrossRef ]
- Su, Y. The Study of Design Preparation and Applications of Triboelectric Nanogenerators. Ph.D. Thesis, University of Electronic Science and Technology, Chengdu, China, 2016. [ Google Scholar ]
- Du, X.; Zhang, K. Recent progress in fibrous high-entropy energy harvesting devices for wearable applications. Nano Energy 2022 , 101 , 107600. [ Google Scholar ] [ CrossRef ]
- Haroun, A.; Tarek, M.; Mosleh, M.; Ismail, F. Recent Progress on Triboelectric Nanogenerators for Vibration Energy Harvesting and Vibration Sensing. Nanomaterials 2022 , 12 , 2960. [ Google Scholar ] [ CrossRef ] [ PubMed ]
- Wang, Z.L.; Wang, A.C. On the origin of contact-electrification. Mater. Today 2019 , 30 , 34–51. [ Google Scholar ] [ CrossRef ]
- Yang, W.; Chen, J.; Jing, Q.; Yang, J.; Wen, X.; Su, Y.; Zhu, G.; Bai, P.; Wang, Z.L. 3D Stack Integrated Triboelectric Nanogenerator for Harvesting Vibration Energy. Adv. Funct. Mater. 2014 , 24 , 4090–4096. [ Google Scholar ] [ CrossRef ]
- Qiu, W.; Feng, Y.; Luo, N.; Chen, S.; Wang, D. Sandwich-like sound-driven triboelectric nanogenerator for energy harvesting and electrochromic based on Cu foam. Nano Energy 2020 , 70 , 104543. [ Google Scholar ] [ CrossRef ]
- Shi, Q.; Yang, J.; Gui, H.; Gui, Y.; Tang, C.; Yu, Y.; Zhao, Z. Self-powered circular-honeycomb triboelectric nanogenerator for vibration energy harvesting and resonance detection of synchronous machine. Sens. Actuators Phys. 2023 , 354 , 114291. [ Google Scholar ] [ CrossRef ]
- Liu, Z.; Zhao, C.; Hu, G.; Yang, Y. A multi-degree-of-freedom triboelectric energy harvester for dual-frequency vibration energy harvesting. Mech. Syst. Signal Process. 2023 , 188 , 109951. [ Google Scholar ] [ CrossRef ]
- Yang, X.; Zheng, H.; Ren, H.; An, G.; Zhang, Y.; Yang, W. A tuned triboelectric nanogenerator using a magnetic liquid for low-frequency vibration energy harvesting. Nanoscale 2024 , 16 , 1915–1923. [ Google Scholar ] [ CrossRef ]
- Gao, S.; Wei, H.; Wang, J.; Luo, X.; Wang, R.; Chen, Y.; Xiang, M.; Chen, X.; Xie, H.; Feng, S. Self-powered system by a suspension structure-based triboelectric-electromagnetic-piezoelectric hybrid generator for unifying wind energy and vibration harvesting with vibration attenuation function. Nano Energy 2024 , 122 , 109323. [ Google Scholar ] [ CrossRef ]
- Zhao, H.; Ouyang, H. Theoretical investigation and experiment of a disc-shaped triboelectric energy harvester with a magnetic bistable mechanism. Smart Mater. Struct. 2021 , 30 , 095026. [ Google Scholar ] [ CrossRef ]
- Naruse, Y.; Matsubara, N.; Mabuchi, K.; Izumi, M.; Suzuki, S. Electrostatic micro power generation from low-frequency vibration such as human motion. J. Micromech. Microeng. 2009 , 19 , 094002. [ Google Scholar ] [ CrossRef ]
- Bu, L.; Wu, X.; Wang, X.; Liu, L. A Novel Vibration Energy Harvester of Polytetrafluoroethylene Bulk Electrets and Double Side Charging Structure. Nanotechnol. Precis. Eng. 2013 , 11 , 497–502. [ Google Scholar ]
- Kloub, H.; Hoffmann, D.; Folkmer, B.; Manoli, Y. A micro capacitive vibration energy harvester for low power electronics. Work 2009 , 11 , 1. [ Google Scholar ]
- Tao, K.; Wu, J.; Tang, L.; Hu, L.; Lye, S.W.; Miao, J. Enhanced electrostatic vibrational energy harvesting using integrated opposite-charged electrets. J. Micromech. Microeng. 2017 , 27 , 044002. [ Google Scholar ] [ CrossRef ]
- Aliasghary, M.; Azizi, S.; Madinei, H.; Haddad Khodaparast, H. On the Efficiency Enhancement of an Actively Tunable MEMS Energy Harvesting Device. Vibration 2022 , 5 , 603–612. [ Google Scholar ] [ CrossRef ]
- Erturun, U.; Eisape, A.; West, J.E. Design and analysis of a vibration energy harvester using push-pull electrostatic conversion. Smart Mater. Struct. 2020 , 29 , 105018. [ Google Scholar ] [ CrossRef ]
- Yamane, D.; Tamura, K.; Nota, K.; Iwakawa, R.; Lo, C.Y.; Miwa, K.; Ono, S. Contactless Electrostatic Vibration Energy Harvesting Using Electric Double Layer Electrets. Sens. Mater. 2022 , 34 , 1869–1877. [ Google Scholar ] [ CrossRef ]
- Deng, Z.; Dapino, M.J. Review of magnetostrictive vibration energy harvesters. Smart Mater. Struct. 2017 , 26 , 103001. [ Google Scholar ] [ CrossRef ]
- Dai, X.-Z.; Wen, Y.-M.; Li, P.; Yang, J.; Jiang, X.-F. Vibration energy harvester based on magnetoelectric transducer. Acta Phys. Sin. 2010 , 59 , 2137–2146. [ Google Scholar ] [ CrossRef ]
- Cao, S.; Sun, S.; Zheng, J.; Wang, X.; Han, J.; Liu, L. Research Progress of Magnetostrictive Vibration Energy Harvesters. Micro Nano Electron. 2017 , 54 , 612–620. [ Google Scholar ] [ CrossRef ]
- Kita, S.; Ueno, T.; Yamada, S. Improvement of force factor of magnetostrictive vibration power generator for high efficiency. J. Appl. Phys. 2015 , 117 , 17B508. [ Google Scholar ] [ CrossRef ]
- Yan, B.; Zhang, C.; Li, L. Design and Fabrication of a High-Efficiency Magnetostrictive Energy Harvester for High-Impact Vibration Systems. IEEE Trans. Magn. 2015 , 51 , 1–4. [ Google Scholar ] [ CrossRef ]
- Dong, W.; Liang, Q.; Liu, H.; Mei, X.; Shu, L.; Liu, Z.; Chang, Y. Characterization of magnetostrictive bi-stable rotational vibration energy harvester with integrated centrifugal effect. Smart Mater. Struct. 2024 , 33 , 025034. [ Google Scholar ] [ CrossRef ]
- Liu, H.; Tong, X.; Sun, X.; Wang, W.; Su, L.; Chang, Y.; Liu, Z. Design and analysis of magnetostrictive two-dimensional kinetic energy harvester. Smart Mater. Struct. 2024 , 33 , 025032. [ Google Scholar ] [ CrossRef ]
- Liu, Z.; Huang, W.; Guo, P.; Yang, H.; Weng, L.; Wang, B. Nonlinear Model for Magnetostrictive Vibration Energy Harvester Considering Dynamic Forces. IEEE Sens. J. 2024 , 24 , 16270–16278. [ Google Scholar ] [ CrossRef ]
- Liu, H.; Liu, H.; Zhao, X.; Li, A.; Yu, X. Design and Characteristic Analysis of Magnetostrictive Vibration Harvester with Double-Stage Rhombus Amplification Mechanism. Machines 2022 , 10 , 848. [ Google Scholar ] [ CrossRef ]
- Ueno, T. Performance of improved magnetostrictive vibrational power generator, simple and high power output for practical applications. J. Appl. Phys. 2015 , 117 , 17A740. [ Google Scholar ] [ CrossRef ]
- Clemente, C.S.; Davino, D.; Visone, C. Experimental Characterization of a Three-Rod Magnetostrictive Device for Energy Harvesting. IEEE Trans. Magn. 2017 , 53 , 1–4. [ Google Scholar ] [ CrossRef ]
Click here to enlarge figure
Authors | Fabric | Frequency (Hz) | Output Voltage/V | Output Power/mW | Power Density/ ) |
---|---|---|---|---|---|
Cui et al. [ ] | Permanent magnets—coils | 31 | - | 3.83 | - |
Wang et al. [ ] | Rack and pinion, bevel gears | - | 18.5 | 2700 | - |
Peng et al. [ ] | Magnets—coils | 20 | - | 37.45 | - |
Monaco et al. [ ] | magnetic levitation solution | 4 | - | 32 | - |
Sun et al. [ ] | Magnets—coils | 116 | - | 27.2 | 3.6 |
Lorenzo et al. [ ] | Dobby electromagnetic pendulum | 9 | - | 14.4 | - |
Sun et al. [ ] | Spring pendulum | 0.85 | - | 750 | - |
Piezoelectricity | Electromechanical Coupling Coefficient | Piezoelectric Constant (pC/N) |
---|---|---|
AlN | 0.23 | −2.00 |
CdS | 0.26 | −5.18 |
ZnO | 0.48 | −5.00 |
BaTiO | 0.49 | −58.0 |
PZT-4 | 0.70 | −123 |
PZT-5H | 0.75 | −274 |
LiNbO (lithium niobate) | 0.23 | −1.00 |
PVDF | 0.19 | 21.0 |
Authors | Fabric | Frequency (Hz) | Output Voltage/V | Output Power/μW | Power Density/ ) | |
---|---|---|---|---|---|---|
Fang et al. [ ] | Cantilever beam type | 609 | 0.898 | 21.4 | 2.16 | - |
Shen et al. [ ] | Cantilever beam type | 461.15 | 0.16 | 6 | 2.15 | 3.272 |
Ye et al. [ ] | PSN-PZT piezoelectric ceramics | 10 | 52.89 | - | 35,010 | - |
Wang et al. [ ] | tapered beam | 10.06 | 19.82 | - | - | - |
Cho et al. [ ] | Cantilever beam type | 30 | - | - | 52,500 | 28.48 |
Lee et al. [ ] | Cantilever beam type | 255.9 | 1.792 | 150 | 2.765 | - |
Remya et al. [ ] | Spring-mass block | 30 | 38 | 2700 | - | - |
Ramírez et al. [ ] | Cantilever beam type | 7.91 | 9.8 | 1000 | 96.04 | - |
Authors | Fabric | Frequency (Hz) | Output Voltage/V | Short-Circuit Current/ | Output Power/mW | Power Density/ ) | Current Density/ (mA-m ) | |
---|---|---|---|---|---|---|---|---|
Yang et al. [ ] | Three-dimensional (3D) integrated multilayer TENGs | - | 303 | - | - | 0.6 | 104.6 | - |
Qiu et al. [ ] | Sandwich-shaped acoustic drive TENG | 125 | 546.3 | - | 60.9 | - | - | 25.01 |
Shi et al. [ ] | circular honeycomb | 37 | 50 | - | 3.3 | - | - | - |
Liu et al. [ ] | L-shaped beam | - | 11.56 | 85 | - | 0.3 | - | - |
Yang et al. [ ] | Magnetic fluids | 7 | 0.6 | 90 | 0.00457 | 0.0054 | - | - |
Gao et al. [ ] | Suspension Structure | 13.6 | 30.5 | 1026.6 | 8.2 | - | - | |
Zhao et al. [ ] | Rejection magnet | 2 | 9.7 | - | - | 0.0086 | - | - |
Authors | Fabric | Frequency (Hz) | Acceleration/(m/s ) | Load/MΩ | Starting Voltage/V | Output Voltage/V | Output Power/ |
---|---|---|---|---|---|---|---|
Naruse et al. [ ] | Stripe mask electret | 2 | - | - | - | - | 40 |
Bu et al. [ ] | Block electrodes | 10 | - | - | −700 | - | 5.5 |
Kloub et al. [ ] | Area overlap | - | 1 g | - | 25 | 5.7 | - |
Tao et al. [ ] | Sandwich construction | 122.1 | 5 | - | - | - | 0.22 |
Ugur et al. [ ] | Electret—variable area | - | - | - | - | 300 | 15 |
Daisuke et al. [ ] | Double electret electret | 155 | 1 g | 1 | - | - | - |
Authors | Fabric | Frequency (Hz) | Output Voltage/mV | Output Power/ | Power Density/ (mW-cm ) |
---|---|---|---|---|---|
Shota et al. [ ] | Parallel beam construction | 202 | - | 0.73 | - |
Dong et al. [ ] | Cantilever | - | 780 | 4.35 | - |
Liu et al. [ ] | Galfenol rods—excitation coils | - | 2.64 | 170 | - |
Liu et al. [ ] | Double-stage lozenge | 30 | 250 | 1.056 | - |
Ueno et al. [ ] | Cantilever | 212 | 3000 | 1.2 | 3 |
Carmine et al. [ ] | Three Galfenol rods—permanent magnets | 100 | 6 | 7 |
The statements, opinions and data contained in all publications are solely those of the individual author(s) and contributor(s) and not of MDPI and/or the editor(s). MDPI and/or the editor(s) disclaim responsibility for any injury to people or property resulting from any ideas, methods, instructions or products referred to in the content. |
Share and Cite
Qu, G.; Xia, H.; Liang, Q.; Liu, Y.; Ming, S.; Zhao, J.; Xia, Y.; Wu, J. Current Research Status and Future Trends of Vibration Energy Harvesters. Micromachines 2024 , 15 , 1109. https://doi.org/10.3390/mi15091109
Qu G, Xia H, Liang Q, Liu Y, Ming S, Zhao J, Xia Y, Wu J. Current Research Status and Future Trends of Vibration Energy Harvesters. Micromachines . 2024; 15(9):1109. https://doi.org/10.3390/mi15091109
Qu, Guohao, Hui Xia, Quanwei Liang, Yunping Liu, Shilin Ming, Junke Zhao, Yushu Xia, and Jianbo Wu. 2024. "Current Research Status and Future Trends of Vibration Energy Harvesters" Micromachines 15, no. 9: 1109. https://doi.org/10.3390/mi15091109
Article Metrics
Article access statistics, further information, mdpi initiatives, follow mdpi.
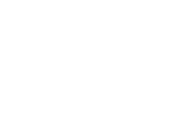
Subscribe to receive issue release notifications and newsletters from MDPI journals
This paper is in the following e-collection/theme issue:
Published on 3.9.2024 in Vol 26 (2024)
This is a member publication of University of Oxford (Jisc)
Value of Engagement in Digital Health Technology Research: Evidence Across 6 Unique Cohort Studies
Authors of this article:

Original Paper
- Sarah M Goodday 1, 2 , MSc, PhD ;
- Emma Karlin 1 , MSc ;
- Alexa Brooks 1 , MS, RD ;
- Carol Chapman 3 , MPH ;
- Christiana Harry 1 , MPH ;
- Nelly Lugo 1 , BS ;
- Shannon Peabody 1 , BA ;
- Shazia Rangwala 4 , MPH ;
- Ella Swanson 1 , BS ;
- Jonell Tempero 1 , BS, MS ;
- Robin Yang 1 , MS ;
- Daniel R Karlin 1, 5, 6 , MA, MD ;
- Ron Rabinowicz 7, 8 , MD ;
- David Malkin 7, 9 , MD ;
- Simon Travis 10 , Prof Dr ;
- Alissa Walsh 10 , MD ;
- Robert P Hirten 11 , MD ;
- Bruce E Sands 11 , MS, MD ;
- Chetan Bettegowda 12 , MD, PhD ;
- Matthias Holdhoff 13 , MD, PhD ;
- Jessica Wollett 12 , MS ;
- Kelly Szajna 12 , BSc, RN ;
- Kallan Dirmeyer 12 , BS ;
- Anna Dodd 14 , MS ;
- Shawn Hutchinson 14 , MS ;
- Stephanie Ramotar 14 , BSc ;
- Robert C Grant 14 , MD, PhD ;
- Adrien Boch 15 , MA ;
- Mackenzie Wildman 16 , PhD ;
- Stephen H Friend 2, 4 , MD, PhD
1 4YouandMe, Seattle, WA, United States
2 Department of Psychiatry, University of Oxford, Oxford, United Kingdom
3 Crohn's & Colitis Foundation, New York, NY, United States
4 Section of Urology and Renal Transplantation, Virginia Mason Francisan Health, Seattle, WA, United States
5 MindMed Inc, New York, NY, United States
6 Tufts University School of Medicine, Boston, MA, United States
7 Department of Paediatrics, University of Toronto, Toronto, ON, Canada
8 Department of Pediatric Hematology/Oncology, Schneider Children's Medical Center of Israel, Petach-Tikva, Israel
9 Department of Pediatrics, University of Toronto, Toronto, ON, Canada
10 Gasteroentology Unit, Oxford University Hospitals NHS Foundation Trust and Biomedical Research Centre, Oxford, United Kingdom
11 The Dr. Henry D. Janowitz Division of Gastroenterology, Icahn School of Medicine at Mount Sinai, New York, NY, United States
12 Department of Neurosurgery, Johns Hopkins University School of Medicine, Baltimore, MD, United States
13 The Sidney Kimmel Comprehensive Cancer Center at Johns Hopkins, Baltimore, MD, United States
14 Princess Margaret Cancer Center, University Health Network, Toronto, ON, Canada
15 Evidation Health Inc, Santa Mateo, CA, United States
16 Sage Bionetworks, Seattle, WA, United States
Corresponding Author:
Sarah M Goodday, MSc, PhD
2901 3rd Ave
Seattle, WA, 98121
United States
Phone: 1 (206) 928 8243
Email: [email protected]
Background: Wearable digital health technologies and mobile apps (personal digital health technologies [DHTs]) hold great promise for transforming health research and care. However, engagement in personal DHT research is poor.
Objective: The objective of this paper is to describe how participant engagement techniques and different study designs affect participant adherence, retention, and overall engagement in research involving personal DHTs.
Methods: Quantitative and qualitative analysis of engagement factors are reported across 6 unique personal DHT research studies that adopted aspects of a participant-centric design. Study populations included (1) frontline health care workers; (2) a conception, pregnant, and postpartum population; (3) individuals with Crohn disease; (4) individuals with pancreatic cancer; (5) individuals with central nervous system tumors; and (6) families with a Li-Fraumeni syndrome affected member. All included studies involved the use of a study smartphone app that collected both daily and intermittent passive and active tasks, as well as using multiple wearable devices including smartwatches, smart rings, and smart scales. All studies included a variety of participant-centric engagement strategies centered on working with participants as co-designers and regular check-in phone calls to provide support over study participation. Overall retention, probability of staying in the study, and median adherence to study activities are reported.
Results: The median proportion of participants retained in the study across the 6 studies was 77.2% (IQR 72.6%-88%). The probability of staying in the study stayed above 80% for all studies during the first month of study participation and stayed above 50% for the entire active study period across all studies. Median adherence to study activities varied by study population. Severely ill cancer populations and postpartum mothers showed the lowest adherence to personal DHT research tasks, largely the result of physical, mental, and situational barriers. Except for the cancer and postpartum populations, median adherences for the Oura smart ring, Garmin, and Apple smartwatches were over 80% and 90%, respectively. Median adherence to the scheduled check-in calls was high across all but one cohort (50%, IQR 20%-75%: low-engagement cohort). Median adherence to study-related activities in this low-engagement cohort was lower than in all other included studies.
Conclusions: Participant-centric engagement strategies aid in participant retention and maintain good adherence in some populations. Primary barriers to engagement were participant burden (task fatigue and inconvenience), physical, mental, and situational barriers (unable to complete tasks), and low perceived benefit (lack of understanding of the value of personal DHTs). More population-specific tailoring of personal DHT designs is needed so that these new tools can be perceived as personally valuable to the end user.
Introduction
Wearable digital health technologies (DHTs) [ 1 , 2 ] and mobile apps facilitate the remote, real-world assessment of health including objective signs of disease that are typically confined to health care visits and health care provider interpretation. These specific categories of DHTs, herein referred to as “personal DHTs,” hold promise for transforming health research through the new ability to capture high-resolution, high-frequency, in-the-moment health-related multimodal information in decentralized ways. Through the provision of personal DHTs in clinical care, individuals could be better empowered to navigate their health outside the health care system with greater accessibility, agency, and accuracy than currently possible [ 1 , 2 ]. One of the largest challenges in the future of digital health that involves the use of personal DHTs is end-user engagement. While direct comparisons of engagement in personal DHT research are challenging due to the heterogeneous reporting of retention and adherence factors, and a lack of consensus on a definition of “engagement” [ 3 - 6 ], accumulating evidence supports that so far engagement in the use of personal DHTs has been poor. Specifically, retention in personal DHT research studies and the use of health-related apps is low across diverse populations and applications [ 7 - 9 ]. Further, there is evidence of attrition biases in personal DHT research resulting in insufficient representation of minority populations [ 7 ]. In addition to poor retention, personal DHT research studies have low adherence to completing active app-based tasks resulting in large amounts of missing data. This missing data problem results in challenges in artificial intelligence models from insufficient volumes of data to follow individual patterns, and limits app-based context “label” data. This “label” data is crucial for validating passively collected information from personal DHTs, particularly given the early state of the field and as the utility of certain approaches such as knowledge graphs and large language models emerge.
Several personal DHTs health research studies have started to surface [ 7 - 12 ], resulting in the identification of barriers to engagement. These barriers include technical problems with the technology and in collecting the data, usability, privacy concerns, and digital literacy. Many of these barriers point to a need to retain a human element in the research process, and to include an aspect of co-designing with end users. Emerging personal DHT research studies that show better engagement retain some form of “human-in-the-loop” (regular contact with research staff) and co-design or end-user approach [ 11 - 15 ]. Among these studies, retention rates of 80% and higher have been observed, while average adherence to wearable device use and daily app surveys have been shown to be >90% and 70%, respectively [ 11 - 15 ].
The promise of digital health rests on the assumption that end users can be engaged in the long-term use of personal DHTs for health monitoring, yet this remains to be seen among most existing research applications. There have been increasing international calls for the inclusion of patients in the design and conduct of health research [ 16 - 18 ], and this seems particularly relevant for digital health research where the patient is the end user of these new remote tools. In this paper, we report on engagement across 6 unique personal DHT health research studies that adopted different aspects of a participant-centric design, but each with distinct population and design features. The objective is to describe how participant engagement techniques and different personal DHT designs affect participant adherence, retention, and overall engagement in personal DHT health research.
Study Design
In total, 6 personal DHT research studies are included in this quantitative and qualitative analysis of engagement that span diverse populations including a frontline health care population (the stress and recovery in frontline health care workers study) [ 11 ]; a conception, pregnancy, and postpartum population (Better Understanding the Metamorphosis of Pregnancy [BUMP] study) [ 19 ]; and populations with different diseases including Crohn disease (stress in Crohn: forecasting symptom transitions study), Li-Fraumeni syndrome (stress and LFS: a feasibility study of wearable technologies to detect stress in families with LFS), and patients with pancreatic and central nervous system (CNS) tumors (help enable real-time observations [HERO] in pancreatic [PANC] and CNS tumors studies) [ 20 ].
All of these studies were conducted by 4YouandMe—a US-based nonprofit (charitable) organization. 4YouandMe specializes in open-source research into the application of personal DHTs for health and wellness [ 20 ]. 4YouandMe has a particular focus on leveraging personal DHTs to empower the patient in navigating their unique disease or life transitional period. These 6 studies were included in this analysis as they reflect all of the completed studies by 4YouandMe at the time of this analysis. Characteristics of these studies can be found in Table 1 and additional methodological detail can be found in Multimedia Appendix 1 . All studies involved the use of a bespoke study smartphone app built by 4YouandMe and the use of the Oura smart ring, the Garmin smartwatch, the Apple smartwatch, an Empatica smartwatch, and the Bodyport Cardiac Scale. Details of these devices can be found in Multimedia Appendix 2 ).
Study and population | Sample size | Age (years), median (IQR) | Active study time (months) | Recruitment | Devices | Average (SD) app daily burden | Compensation | Engagement strategy | |
Frontline health care workers | 365 | 33.0 (28.0-42.0) | 4-6 | Remote: Social media and health care organization newsletters | 5 (1.8) minutes | None (participants completing the study kept the wearable devices) | |||
Patients with Crohn disease | 195 (MSSM , N=139; Oxford, N=56) | MSSM (median 29, IQR 24-37), Oxford (median 39, IQR 32-50) | 6-9 | In-clinic: through inflammatory bowel disease clinics | 7.7 (1.0) minutes | Yes, participants could keep the ring or receive compensation based on points accumulated | |||
Patients with CNS tumors | 12 | 52 (43-56) | 7 | In-clinic: through cancer specialty clinics | 5.3 (2.1) minutes | None (participants completing the study kept the wearable devices) | |||
Patients with pancreatic cancer | 26 | 57 (53-65) | 1 to 14 months | In-clinic: through cancer specialty clinics | 3.1 (1.9) minutes | None (participants completing the study kept the wearable devices) | |||
Affected and unaffected family members of a proband with LFS | 49 | 39.0 (7.9-68.0) | 6 | In-clinic: through cancer specialty clinics | 2.3 (0.9) minutes | None | |||
Pregnant individuals (up to 15 weeks) | 524 | 33.0 (30-36) | Up to 12 months | Remote: through patient-provider portals, social media, and community health clinics | 5.0 (2.3) minutes | Yes, participants received compensation based on study points accumulated | |||
Individuals actively attempting to get pregnant | 273 | 34.0 (31-36) | Up to 6 months | Remote: through patient-provider portals, social media, and community health clinics | 3.8 (2.0) minutes | Yes, participants could keep the ring or receive compensation |
a MSSM: Mount Sinai School of Medicine.
b HERO-CNS: help enable real-time observations—central nervous system.
c CNS: central nervous system.
d HERO-PANC: help enable real-time observations—pancreatic cancer.
e n=24, 2 unknown.
f Until withdrawal, progression, death, or study completion (October 31, 2022).
g LFS: Li-Fraumeni syndrome.
h BUMP: Better Understanding the Metamorphosis of Pregnancy.
i BUMP-C: Better Understanding the Metamorphosis of Pregnancy—Conception.
Ethical Considerations
All included studies were approved by the local institutional research ethics boards (REB) at their local sites ( Multimedia Appendix 1 ): stress and recovery in frontline health care workers study (institutional review board [IRB], Advarra [4UCOVID1901, Pro00043205]), BUMP study (IRB Advarra Pro00047893), stress in Crohn (Oxford site: Hampshire-A IRAS ID: 269286, Mount Sinai School of Medicine [MSSM] site: IRB of MSSM: GCO 19-1543 | IRB-19-02298), stress and LFS (Sick Kids: REB: 1000072240), HERO-CNS (John Hopkins Medicine IRB IRB00253818), and HERO-PANC (University Hospital Network REB: 20-5211).
Statistical Analysis
Definitions of adherence in digital health research studies are heterogeneous [ 3 - 6 ]. Consistent criteria for adherence across all included studies were attempted. While many different wearable features could be used as the basis for the use of the device, features that were most reliably monitored were selected. For studies using the Oura smart ring, daily adherence was defined as at least one sleep data event present for the prior night. The Oura ring was only expected to be worn at night for many of the included studies, which is why sleep data were used as the indicator for adherence. For studies using the Garmin smartwatch, daily adherence was defined as step data present for that day. For the Empatica smartwatch, daily adherence was defined as at least one data event (worn properly in a day). Adherence to the Bodyport Cardiac Scale was defined as the proportion of days where a weight event was present divided by the total number of expected follow-up days. Adherence to in-app task completion was defined as the proportion of tasks completed when prompted in the app divided by the total number of tasks that should have been completed over study follow-up. For example, all included studies had a daily survey. In a study with a minimum of 4 months of follow-up expected from participants, the total number of expected daily surveys is approximately 120. For a weekly app survey, the total number of expected surveys for a 4-month study follow-up would be 16. Adherence to biweekly check-in calls was defined as the proportion of calls completed divided by the total number of expected calls over study follow-up. Medians and ranges are described since the adherence distributions were nonnormally distributed. All adherence estimations were performed only among retained participants.
Differences in adherence and retention by sociodemographic characteristics were estimated using χ 2 , Fisher exact, Mann-Whitney U , and ANOVA tests where appropriate among studies that have sufficient sample sizes (stress and recovery, BUMP, and stress in Crohn). Survival probabilities using the Kaplan-Meier approach were calculated to display the probability of retention over the course of each included study. Retention (total proportion of participants completing the study among all enrolled) is also reported. Additional information on how retention was calculated for each unique study can be found in Multimedia Appendix 3 .
Description of Included Studies
Study design characteristics of all studies are described in Table 1 . All studies included the use of at least one wearable device plus a study app that involved daily, as well as intermittent surveys (daily question prompts, validated questionnaires) and active tasks (cognitive active or physical function tasks [eg, walk tests], video diaries). In all included studies, participants were required to use their own Android or iPhone smartphone for study activities. Recruitment mechanisms differed across studies with some including remote recruitment through digital advertisements on social media, professional organizations and newsletters, and patient portals (stress and recovery, and BUMP), while others recruited patients in-person through specialty clinics (stress in Crohn, HERO studies, and stress and LFS). The daily burden of app active tasks across studies ranged from 2 to 7 minutes. Study follow-up periods across studies ranged from 4 to 18 months. Across all studies except the stress and LFS study, participants were offered to keep some of the study wearable devices (most often the ring and the watch). Further, 2 studies included the option for modest financial compensation (BUMP and stress in Crohn).
All studies included an engagement strategy that centered around a biweekly phone check-in with a consistent engagement specialist that served the purpose of supporting participants, helping them with onboarding, resolving potential technological problems, and discussing and collecting study experience feedback. Additionally, all included studies implemented different strategies that focused on working with participants as co-designers. These strategies included making app changes that were driven by direct participant feedback during active follow-up, offering a “your data” section in the app that allowed participants to track key symptoms over time, hosting optional investigator-participant Zoom calls where participants could meet the study team, receive study updates, preliminary results, and could offer more feedback, and inviting participants to contribute to and be listed as coauthors on published work.
Adherence by Study Population
Median adherence in engagement phone check-in calls, wearable device use, daily app survey completion, and in-app active tasks can be found in Table 2 . Median adherence varied across study populations. The stress in Crohn–MSSM site had a lower adherence on the engagement check-in calls (50%) compared to other studies, many of which had 100% adherence on these calls ( Table 2 ). This study site is herein referred to as the low-engagement cohort. In this low-engagement cohort, median adherence to completing daily app surveys, to wearing the Empatica smartwatch, and to using the Bodyport Cardiac Scale were lower than all other study cohorts that included these studies’ activities (except the BUMP-postpartum cohort). Further, median adherence to using the Oura smart ring was lower in the low-engagement cohort compared to other cohorts except for the postpartum and severely ill cancer populations.
The HERO studies included the most severely ill participants including patients with active diagnoses of CNS and pancreatic tumors. Some HERO participants were undergoing chemotherapy, some had therapy-related complications, some had infections, and some had progressive, life-threatening tumor growth. While the total number of participants in these studies was low, these studies showed low adherence on the daily survey (<55%) and wearable device use (<65% HERO-CNS only). Interestingly, HERO-PANC participants exhibited high wearable device use median adherence (83.3%, IQR 51%-93.2%, Oura and 95.5%, IQR 75.2%-99.2%, Garmin), despite the health status of this population. Further, median adherence to in-app cognitive active tasks was higher among the HERO studies compared to most other studies. Engagement check-in call adherence was also high in the HERO studies. Among the BUMP postpartum cohort, there was consistently lower adherence on all study tasks except for the engagement check-in calls compared to other studies, particularly in comparison to the BUMP prenatal cohort. Specifically, median adherence to the Oura ring, Garmin smartwatch use, and the Bodyport Cardiac Scale in the BUMP-prenatal cohort compared to the BUMP postpartum cohort dropped from 87.2% (IQR 68.7%-96.7%) to 55% (IQR 5.5%-83.7%), 96.7% (IQR 82.9%-100%) to 62.5% (IQR 12.3%-96.4%), and 74.7% (IQR 52%-87.3%) to 33.1% (IQR 8.9%-67.7%), respectively ( Table 2 ).
Stress and recovery | BUMP-C | BUMP | BUMP-POST | SINC -MSSM | SINC-Oxford | HERO-CNS | HERO-PANC | Stress in LFS | |
Participants, n | 297 | 98 | 379 | 379 | 117 | 54 | 7 | 19 | 45 |
ES check-ins, median (IQR) | 75.0 (57.1-87.5) | 100.0 (87.9-100.0) | 100.0 (88.4-100.0) | 100.0 (100.0-100.0) | 50.0 (20.0-75.0) | 100.0 (90.9-100.0) | 85.7 (78.1-88.2) | 100.0 (100.0-100.0) | 60.0 (40.0-80.0) |
Oura ring, median (IQR) | 97.0 (86.0-100.0) | 90.6 (76.3-97.7) | 87.2 (68.7-96.7) | 55.0 (5.5-83.7) | 80.5 (37.1-92.4) | 98.9 (94.0-99.6) | 42.3 (32.0-58.2) | 83.3 (51.0-93.2) | — |
Garmin watch, median (IQR) | — | — | 96.7 (82.9-100.0) | 62.4 (12.3-96.4) | — | — | 63.3 (54.7-64.3) | 95.5 (75.2-99.2) | — |
Apple watch, median (IQR) | — | — | 98.1 (87.7-100.0) | 79.8 (32.4-96.3) | — | — | — | — | — |
Empatica watch, median (IQR) | — | — | — | — | 26.0 (6.2-64.1) | 72.5 (37.1-96.8) | — | — | 86.8 (66.7-95.6) |
Bodyport scale, median (IQR) | — | — | 74.7 (52.0-87.3) | 33.1 (8.9-67.7) | 38.5 (17.1-64.7) | — | — | 79.5 (52.7-88.4) | — |
Daily survey, median (IQR) | 75.4 (57.2-88.2) | 42.4 (24.6-69.7) | 60.1 (34.4-81.7) | 18.4 (1.0-47.6) | 27.9 (10.4-51.9) | 70.3 (41.9-84.0) | 53.3 (47.8-71.5) | 49.1 (20.2-83.4) | 62.5 (40.96-82.59) |
Reaction rime, median (IQR) | 88.9 (75.0-100.0) | 43.4 (24.3-72.8) | — | — | 30.4 (9.7-50.6) | 69.5 (46.6-89.3) | 59.0 (50.0-66.7) | 62.5 (20.9-86.6) | — |
Trail making, median (IQR) | 88.9 (71.1-100.0) | 46.5 (24.0-73.7) | — | — | 28.7 (9.4-50.0) | 71.6 (45.0-87.3) | 61.5 (52.1-76.5) | 38.1 (4.2-76.2) | 57.7 (36.8-72.0) |
EBT , median (IQR) | — | 30.1 (16.2-54.1) | 44.6 (22.6-73.9) | 6.5 (0.0-33.3) | 23.1 (9.1-44.4) | 32.1 (0.0-58.6) | — | — | — |
N-Back, median (IQR) | — | — | 51.4 (24.9-76.4) | 8.3 (0.0-44.4) | — | — | — | — | — |
Gait task, median (IQR) | — | — | 25.0 (0.0-60.0) | 0.0 (0.0-0.0) | — | — | 24.5 (18.8-62.8) | 36.0 (2.2-74.0) | — |
Walk test, median (IQR) | — | — | 14.3 (0.0-40.0) | 0.0 (0.0-0.0) | — | — | 23.1 (13.9-60.4) | 25.0 (7.8-49.5) | — |
Video diary, median (IQR) | — | 4.3 (0.0-27.7) | 8.3 (0.0-50.0) | 0.0 (0.0-0.0) | 5.6 (0.0-22.2) | 9.4 (0.0-35.1) | 25.0 (8.7-77.1) | 0.0 (0.0-37.5) | — |
a BUMP-C: Better Understanding the Metamorphosis of Pregnancy—Conception.
b BUMP: Better Understanding the Metamorphosis of Pregnancy.
c BUMP-POST: Better Understanding the Metamorphosis of Pregnancy—Postpartum.
d SINC: stress in Crohn.
e MSSM: Mount Sinai School of Medicine.
f HERO-CNS: help enable real-time observations—central nervous system.
g HERO-PANC: help enable real-time observations—pancreatic cancer.
h LFS: Li-Fraumeni syndrome.
i ES: engagement specialist.
j Not available.
k EBT: emotional bias test.
Adherence by Study Activity
There were differences in adherence rates across different study activities. Adherence to wearable device use was consistently higher across studies compared to in-app activities, which is not surprising given the passive nature of these devices. Excluding the postpartum and HERO-CNS study, median adherence to Oura ring use was >80% across all studies, and as high as 99% (IQR 94.9%-99.6%; stress in Crohn-Oxford site; Table 2 ). There were also differences in adherence across specific wearable devices. Garmin and Apple smartwatch adherence was >95% in BUMP pregnant individuals and HERO-PANC participants, while median adherence for the Empatica Watch was lower among the studies that used this device (stress in Crohn-Oxford, 72.5%, IQR 37.1%-96.8%; stress in Crohn-MSSM, low-engagement cohort, 26%, IQR 6.2%-64.1%; and stress in LFS, 86.8%, IQR 0.7%-0.9%). Median adherence to the Bodyport Cardiac Scale was 74.7% (IQR 52%-87.3%) among BUMP pregnant individuals and 79.5% (IQR 52.7%-88.4%) in HERO-PANC participants ( Table 2 ). Excluding the postpartum and HERO study populations and the low-engagement cohort, in-app daily survey adherence was >60% for all studies ( Table 2 ). Finally, adherence to in-app active tasks was lower in general compared to other activities such as wearable device use or in-app surveys. Tasks that involved walking (gait and walk task) or speaking (video diaries) showed lower adherence compared to other active tasks (eg, cognitive and emotional bias tasks; Table 2 ).
Adherence by Study Recruitment and Engagement Strategy
There did not appear to be any meaningful difference in median adherence rates across study activities by study recruitment methods (in-clinic vs remote) or follow-up time. Further, 2 studies that included modest financial compensation in addition to engagement strategies showed higher adherence rates compared to some of the other studies (ie, BUMP and stress in Crohn), but the impact of compensation is difficult to disentangle from other study characteristics such as population differences, and these studies did not show superior adherence rates compared to the stress and recovery study that did not offer financial compensation.
The median proportion of participants retained in the study across the 6 studies was 77.2% (IQR 72.6%-88%; Table 3 ). The probability of staying in the study stayed above 80% for all studies during the first month of study participation and stayed above 50% for the entire active study period across all studies ( Multimedia Appendix 4 ).
Study | Proportion retained at study completion, retained/enrolled (%) |
Stress and recovery | 297/365 (81.4) |
BUMP-C | 134/187 (72.7) |
BUMP | 379/524 (72.3) |
Stress in Crohn-MSSM | 117/139 (84.2) |
Stress in Crohn-Oxford | 54/56 (96.4) |
HERO-CNS | 7/12 (58.3) |
HERO-PANC | 19/26 (73.1) |
Stress and LFS | 45/49 (91.8) |
b Only includes participants who were enrolled in the Better Understanding the Metamorphosis of Pregnancy—Conception-specific app.
c BUMP: Better Understanding the Metamorphosis of Pregnancy.
d MSSM: Mount Sinai School of Medicine.
e HERO-CNS: help enable real-time observations—central nervous system.
f HERO-PANC: help enable real-time observations—pancreatic cancer.
g Help enable real-time observations—pancreatic cancer has unique factors to consider when interpreting the proportion retained until study completion, since the study aimed to monitor patients until they developed progressive disease or died, or the study end date (October 31, 2022; see Multimedia Appendix 3 ).
Adherence and Retention by Participant Sociodemographic Characteristics
Median adherence for the Oura smart ring, a smartwatch (Garmin, Apple, and Empatica), and the Bodyport Cardiac Scale was lower among younger participants compared to older participants across most studies ( Multimedia Appendix 5 ). Specifically, Oura smart ring adherence was significantly lower in those aged 18-25 years compared to those aged ≥26 years in the BUMP study ( P =.03) and stress in Crohn-MSSM studies ( P =.02), and was lower in the BUMP-C and stress and recover studies, but this difference was not statistically significant at P =.59 and P =.08, respectively. Median adherence for Apple smartwatch use was significantly lower in those aged 18-25 years compared to those aged ≥26 years in the BUMP study ( P =.02), while median adherence for Garmin smartwatch use was lower but not statistically significant ( P =.06). Median adherence for the Bodyport Cardiac Scale was significantly lower in those aged 18-25 years compared to those aged ≥26 years in BUMP ( P <.005) and stress in Crohn-MSSM ( P <.006).
In the BUMP study, Black or African American ethnicity had significantly higher median adherence to completing the in-app daily survey compared to other race or ethnicity groups ( P =.01). This trend was observed in the stress and recovery study ( P =.07) and the stress in Crohn-MSSM study ( P =.24), although the difference was not statistically significant. In contrast, median adherence to Oura smart ring, smartwatch, and Bodyport Cardiac Scale use was lower among Black or African American individuals compared to other race or ethnicity groups, although these differences were not statistically significant ( Multimedia Appendix 5 ).
Retention did not significantly differ by age group or gender ( Multimedia Appendix 6 ).
Retention likelihood was significantly different by race or ethnicity groups in BUMP-C ( P <.001) and BUMP ( P= .001). Specifically, participants of White ethnicity were more likely to stay in the study in both BUMP-C and BUMP, while participants reporting their race or ethnicity as either unknown or not reporting this item were less likely to be retained ( Multimedia Appendix 6 ).
Barriers to Engagement (Qualitative Synthesis of Participant Feedback)
Figure 1 describes key themes that impacted participant retention, adherence, and overall engagement that cut across all included studies. These themes include participant burden and forgetfulness, digital literacy, physical and mental barriers, personal and altruistic benefits, and privacy and confidentiality. Qualitative feedback from participants, research staff, and investigators across these 5 themes is summarized in Multimedia Appendix 7 . The top three barriers to engagement in active study tasks were (1) participant burden and in particular fatigue with the repetitiveness of tasks; (2) physical or mental and situational barriers that prevented the ability to complete tasks; and (3) personal and altruistic benefit, namely the perception that the use of the personal DHTs was not personally useful for a health benefit or a lack of understanding as to why and how certain features (eg, heart rate variability) could be useful to track for health benefit. Qualitative feedback from participants in the 2 cohorts demonstrating lower adherence (HERO-PANC and BUMP post partum) suggested that while participants were highly engaged, they were either too ill, distracted, or tired to complete many of the study activities while navigating a serious illness or the early postpartum period.

Principal Findings
Evidence across 6 unique and diverse studies involving the longitudinal use of personal DHTs supports that participant-centric engagement strategies aid in participant retention and maintaining good adherence in some populations. These strategies centered around (1) human contact with an engagement specialist as often as every 2 weeks, (2) investigator-participant meetings during active study follow-up, (3) offering returned symptom data in the app, (4) inviting participants to contribute as coauthors in published work, and (5) real-time modifications to the study app based on participant feedback.
In the majority of included studies, the probability of staying in the study stayed above 90% for the first month and stayed above 50% for active study periods for all studies. Lower retention or adherence was observed among studies that included a severely ill cancer population and a postpartum population. Barriers to participation in these cohorts were largely the result of physical and situational roadblocks. Excluding studies of a severely ill and postpartum population and the low-engagement cohort in the stress in Crohn study, adherence to Oura smart ring and Garmin smartwatch use was 80% and as high as 99% in some cohorts, while adherence to the Bodyport Cardiac Scale was 75% in a pregnant population. This supports that different populations can successfully be engaged in the use of active app assessments and wearable devices in the long term with adequate support.
Retention and adherence rates observed in these studies are higher than typically reported by other personal DHT research studies [ 7 - 9 , 12 , 13 , 21 ]. For example, a review of 8 large app-based DHT research studies in the United States reported that the probability of staying in the study dropped to or below 50% after the first 4 weeks of participation for all included studies [ 7 ]. Further, across the 8 included studies in this review, >50% of participants did not engage with the app for at least 7 days. Another large app-based study in the United States, the Warfighter Analytics Using Smartphones for Health study that collected daily active and passive app data reported a median retention of 45.2% (38/84 days), while the probability of staying in the study hit 50% at approximately 5.5 weeks [ 10 ]. A large app-based study in the United Kingdom (cloudy with a chance of pain study) involving daily active app assessments reported that 64% of participants fell into the low engagement or no engagement categories after baseline [ 12 ]. The RADAR study [ 14 ], a multinational study involving active and passive assessments from an app, and a Fitbit reported comparable retention results among participants with major depression to those reported here. This study reported a retention rate of 54.6% for 43 weeks of study participation; however, the probability of staying in the study stayed above 75% for the first several months of participation (~6 months). While the active app assessments in this study only included assessments every 2 weeks as opposed to daily assessments, this study additionally included aspects of a participant-centric design, which may have contributed to the higher reported retention [ 15 ].
Taken together, in comparison to other published personal DHT research studies, the 6 studies included in this paper reflect higher levels of engagement. Importantly, the included studies in this analysis involved high burden designs in comparison to other studies that request, for example, weekly or biweekly active tasks of participants [ 14 ] or only involve the use of a smartwatch. Specifically, across the included studies here, participants were expected to complete on average 4.6 (SD 1.62) minutes a day of app activities in addition to continuously using multiple wearable devices.
While different variations of participant-centric strategies were used across the 6 included studies, a key common feature was a biweekly check-in call with an engagement specialist. These calls served the purpose of providing support and building rapport with participants, working through onboarding and technological issues with study devices, tracking adherence, and receiving study-related feedback from participants. Numerous challenges arise in the conduct of remote, personal DHT research, and without frequent check-in and semiregular data monitoring by research staff, knowledge of these issues is a black box. The most significant drop in retention in personal DHT research studies tends to be during the first few weeks of participation [ 7 ]. These early onboarding weeks are crucial in working with participants to ensure they can get into a rhythm of participation. The passive sensing nature of personal DHTs has much potential to inform new objective measures of health, however, are not always intuitively understood as personally important for unique diseases (eg, heart rate variability or phone screen time). Personal DHT studies allow for “light touch” research approaches that enable data collection without traditional research coordinator contact, but this may come with a cost that inadvertently creates a less engaging study environment for participants and limits the opportunity to help participants understand the value in their participation. Of the included 6 studies, 1 cohort had much lower engagement on the check-in calls (50% adherence) compared to other included studies and, in turn, consistently demonstrated lower adherence to study-related activities. Still, even with extensive engagement designs, populations that had physical, mental, and situational barriers to study task completion (ie, severely ill, postpartum mothers) showed lower adherence to wearable device use and active smartphone tasks compared to other study populations. Top reported barriers to engagement included participant burden, physical, mental, and situational barriers, and low perceived value of personal DHTs for health care. These engagement barriers have been reported in previous literature [ 8 , 9 ] relating to DHT research and in the use of DHT interventions. However, the conveyed importance of the perceived value of the approach among participants in the current analysis is noteworthy. Given the foreign nature of personal DHTs for many individuals, particularly older populations, further work is needed to co-design and educate end users on the potential value of self-monitoring unique health-related data.
Irrespective of the engagement approach, adherence to in-app surveys and tasks was lower than wearable device use, which is not surprising given the higher burden related to in-app activities. The self-reported information captured from frequent or momentary in-app assessments is extremely valuable as context information. This context information or “label” data is useful for validating objectively captured information, yet remains the most difficult to capture in sufficient detail. Further, certain in-app activity adherences were consistently lower than others. Namely, activities that required the user to be active (walk in a straight line or complete a video diary) were low across studies. Still, adherence to daily in-app surveys was >60% for all studies excluding the postpartum and HERO study populations.
Limitations
This quantitative and qualitative analysis compared observational data across different digital health studies. However, no true comparison cohort that did not include engagement strategies was included. Therefore, the inferred casualty of participant check-ins with engagement specialists on retention and adherence rates cannot be not concluded. We are formally testing whether the biweekly check-in significantly increases adherence and retention in an ongoing study with an appropriate comparison arm without check-in support (NCT05753605). One of the included studies (stress and recovery) was conducted during the early 2020 COVID-19 pandemic. There is some evidence that engagement in research was higher during the early pandemic time periods [ 22 ]. It cannot be ruled out that the higher observed retention and adherence in this study compared to others was not due to this potential time period bias. The stress in the Crohn-Oxford site included a population of patients some of whom were already engaged in the use of web-based monitoring of symptoms. In turn, this could have contributed to the high retention and higher adherence observed at this site compared to the other stress in the Crohn-MSSM site. The results presented on barriers to engagement were primarily qualitative and collected from conversations with participants, research staff, and investigators across studies.
Conclusions
Globally, mobile apps are used for a variety of purposes in everyday life, while the use of smartwatches for activity monitoring is gaining increasing popularity. However, the use of these tools for health remains a challenge. These findings support that human support via phone and other participant-centric engagement strategies centered on giving back to participants and working with them as co-designers can support sufficient retention and adherence in personal DHT research across diverse populations. This has implications for the utility and potential necessity of a digital support worker in digital health care, as highlighted by others [ 23 ]. A power of personal DHTs is enabling the patient to be in control of their health through self-monitoring, but this new role comes with a responsibility. This important shift in role from doctor to patient outlines how crucial it is to include patients in the early design phase of personal DHT health research. Further work is needed to inform app designs that support habitual forming activities around task completion so that app-related activities become a part of participants’ daily routine and are perceived as personally valuable.
Acknowledgments
The stress and recovery study was supported in part by the Bill & Melinda Gates Foundation (INV-016651). The stress in Crohn study was funded by the Leona M. and Harry B. Helmsley Charitable Trust (1911-03376). The help enable real-time observation (HERO)–central nervus system study was funded by the Mark Foundation for Cancer Research through an ASPIRE award (19-024-ASP). The HERO–pancreatic cancer study was funded by the Mark Foundation for Cancer Research through an ASPIRE award (19-024-ASP), Pancreatic Cancer Canada, the Princess Margaret Cancer Foundation, and 4YouandMe. The Better Understanding the Metamorphosis of Pregnancy (BUMP) study was funded by 4YouandMe and Sema4 along with supplemental in-kind contributions from coalition partners (Evidation Health, Vector Institute, Cambridge Cognition, and Bodyport). The stress and LFS study was funded by in-kind contributions from 4YouandMe, SickKids Hospital, and the Vector Institute.
Conflicts of Interest
CB is a consultant for Depuy Synthes, Bionaut Labs, Galectin Therapeutics, Haystack Oncology, and Privo Technologies. CB is a cofounder of Belay Diagnostics and OrisDx. DRK is an officer, employee, and shareholder of MindMed; a consultant at Tempus, Nightware, and Limitless; and board member of Sonara. RPH is an advisory board member at Bristol Meyers Squibb. MH is an advisory board member for Servier, AnHeart, and Bayer; steering committee member for Novartis; honoraria from Novartis; data safety monitoring committee member for Advarra and Parexel. RG received a graduate scholarship from Pfizer and provided consulting or advisory roles for Astrazeneca, Tempus, Eisai, Incyte, Knight Therapeutics, Guardant Health, and Ipsen. The others declare no conflicts of interest.
Study descriptions.
Study wearable devices.
Retention calculations.
Probability of retaining in the study across studies.
Median adherence to study activities stratified by sociodemographic characteristics.
Sociodemographic differences in participants who were retained versus not retained.
Qualitative feedback from participants, research staff, and investigators surrounding barriers to engagement in digital health research, summarized across 6 unique studies.
- Friend SH, Ginsburg GS, Picard RW. Wearable digital health technology. N Engl J Med. 2023;389(22):2100-2101. [ CrossRef ] [ Medline ]
- Goodday SM, Geddes JR, Friend SH. Disrupting the power balance between doctors and patients in the digital era. Lancet Digit Health. 2021;3(3):e142-e143. [ FREE Full text ] [ CrossRef ] [ Medline ]
- Ng MM, Firth J, Minen M, Torous J. User engagement in mental health apps: a review of measurement, reporting, and validity. Psychiatr Serv. 2019;70(7):538-544. [ FREE Full text ] [ CrossRef ] [ Medline ]
- White KM, Williamson C, Bergou N, Oetzmann C, de Angel V, Matcham F, et al. A systematic review of engagement reporting in remote measurement studies for health symptom tracking. NPJ Digit Med. 2022;5(1):82. [ FREE Full text ] [ CrossRef ] [ Medline ]
- Daniore P, Nittas V, von Wyl V. Enrollment and retention of participants in remote digital health studies: scoping review and framework proposal. J Med Internet Res. 2022;24(9):e39910. [ FREE Full text ] [ CrossRef ] [ Medline ]
- Chan A, Chan D, Lee H, Ng CC, Yeo AHL. Reporting adherence, validity and physical activity measures of wearable activity trackers in medical research: a systematic review. Int J Med Inform. 2022;160:104696. [ FREE Full text ] [ CrossRef ] [ Medline ]
- Pratap A, Neto EC, Snyder P, Stepnowsky C, Elhadad N, Grant D, et al. Indicators of retention in remote digital health studies: a cross-study evaluation of 100,000 participants. NPJ Digit Med. 2020;3:21. [ FREE Full text ] [ CrossRef ] [ Medline ]
- Simblett S, Matcham F, Siddi S, Bulgari V, Barattieri di San Pietro C, Hortas López J, et al. RADAR-CNS Consortium. Barriers to and facilitators of engagement with mHealth technology for remote measurement and management of depression: qualitative analysis. JMIR mHealth uHealth. 2019;7(1):e11325. [ FREE Full text ] [ CrossRef ] [ Medline ]
- O'Connor S, Hanlon P, O'Donnell CA, Garcia S, Glanville J, Mair F. Understanding factors affecting patient and public engagement and recruitment to digital health interventions: a systematic review of qualitative studies. BMC Med Inform Decis Mak. 2016;16(1):120. [ FREE Full text ] [ CrossRef ] [ Medline ]
- Li SX, Halabi R, Selvarajan R, Woerner M, Fillipo IG, Banerjee S, et al. Recruitment and retention in remote research: learnings from a large, decentralized real-world study. JMIR Form Res. 2022;6(11):e40765. [ FREE Full text ] [ CrossRef ] [ Medline ]
- Goodday SM, Karlin E, Alfarano A, Brooks A, Chapman C, Desille R, Stress And Recovery Participants, et al. An alternative to the light touch digital health remote study: the stress and recovery in frontline COVID-19 health care workers study. JMIR Form Res. 2021;5(12):e32165. [ FREE Full text ] [ CrossRef ] [ Medline ]
- Druce KL, McBeth J, van der Veer SN, Selby DA, Vidgen B, Georgatzis K, et al. Recruitment and ongoing engagement in a UK smartphone study examining the association between weather and pain: cohort study. JMIR mHealth uHealth. 2017;5(11):e168. [ FREE Full text ] [ CrossRef ] [ Medline ]
- Reade S, Spencer K, Sergeant JC, Sperrin M, Schultz DM, Ainsworth J, et al. Cloudy with a chance of pain: engagement and subsequent attrition of daily data entry in a smartphone pilot study tracking weather, disease severity, and physical activity in patients with rheumatoid arthritis. JMIR mHealth uHealth. 2017;5(3):e37. [ FREE Full text ] [ CrossRef ] [ Medline ]
- Matcham F, Leightley D, Siddi S, Lamers F, White KM, Annas P, et al. RADAR-CNS consortium. Remote Assessment of Disease and Relapse in Major Depressive Disorder (RADAR-MDD): recruitment, retention, and data availability in a longitudinal remote measurement study. BMC Psychiatry. 2022;22(1):136. [ FREE Full text ] [ CrossRef ] [ Medline ]
- Zhang Y, Pratap A, Folarin AA, Sun S, Cummins N, Matcham F, et al. Long-term participant retention and engagement patterns in an app and wearable-based multinational remote digital depression study. NPJ Digit Med. Feb 17, 2023;6(1):25. [ CrossRef ] [ Medline ]
- How we engage the public. Wellcome. URL: https://wellcome.org/what-we-do/public-engagement [accessed 2024-02-02]
- Canada's strategy for patient-oriented research. Canadian Institutes of Health Research. URL: https://cihr-irsc.gc.ca/e/44000.html [accessed 2024-02-02]
- NIHR launches new centre for engagement and dissemination. National Institute of Health and Care Research. URL: https://www.nihr.ac.uk/news/nihr-launches-new-centre-for-engagement-and-dissemination/24576 [accessed 2024-02-02]
- Goodday SM, Karlin E, Brooks A, Chapman C, Karlin DR, Foschini L, et al. Better Understanding of the Metamorphosis of Pregnancy (BUMP): protocol for a digital feasibility study in women from preconception to postpartum. NPJ Digit Med. Mar 30, 2022;5(1):40. [ CrossRef ] [ Medline ]
- 4YouandMe. URL: http://4youandme.org [accessed 2023-06-07]
- Nowell WB, Curtis JR, Zhao H, Xie F, Stradford L, Curtis D, et al. Participant engagement and adherence to providing smartwatch and patient-reported outcome data: digital tracking of rheumatoid arthritis longitudinally (DIGITAL) real-world study. JMIR Hum Factors. 2023;10:e44034. [ FREE Full text ] [ CrossRef ] [ Medline ]
- Vargo D, Zhu L, Benwell B, Yan Z. Digital technology use during COVID-19 pandemic: a rapid review. Human Behav and Emerg Tech. Dec 28, 2020;3(1):13-24. [ CrossRef ]
- Perret S, Alon N, Carpenter-Song E, Myrick K, Thompson K, Li S, et al. Standardising the role of a digital navigator in behavioural health: a systematic review. Lancet Digit Health. 2023;5(12):e925-e932. [ FREE Full text ] [ CrossRef ] [ Medline ]
Abbreviations
Better Understanding the Metamorphosis of Pregnancy |
central nervous system |
digital health technology |
help enable real-time observation |
institutional review board |
Li-Fraumeni syndrome |
Mount Sinai School of Medicine |
pancreatic cancer |
research ethics board |
Edited by G Eysenbach, T de Azevedo Cardoso; submitted 06.03.24; peer-reviewed by C Godoy Jr; comments to author 05.04.24; revised version received 12.04.24; accepted 29.05.24; published 03.09.24.
©Sarah M Goodday, Emma Karlin, Alexa Brooks, Carol Chapman, Christiana Harry, Nelly Lugo, Shannon Peabody, Shazia Rangwala, Ella Swanson, Jonell Tempero, Robin Yang, Daniel R Karlin, Ron Rabinowicz, David Malkin, Simon Travis, Alissa Walsh, Robert P Hirten, Bruce E Sands, Chetan Bettegowda, Matthias Holdhoff, Jessica Wollett, Kelly Szajna, Kallan Dirmeyer, Anna Dodd, Shawn Hutchinson, Stephanie Ramotar, Robert C Grant, Adrien Boch, Mackenzie Wildman, Stephen H Friend. Originally published in the Journal of Medical Internet Research (https://www.jmir.org), 03.09.2024.
This is an open-access article distributed under the terms of the Creative Commons Attribution License (https://creativecommons.org/licenses/by/4.0/), which permits unrestricted use, distribution, and reproduction in any medium, provided the original work, first published in the Journal of Medical Internet Research (ISSN 1438-8871), is properly cited. The complete bibliographic information, a link to the original publication on https://www.jmir.org/, as well as this copyright and license information must be included.
AI server maker Super Micro denies short-seller Hindenburg's claims
- Medium Text
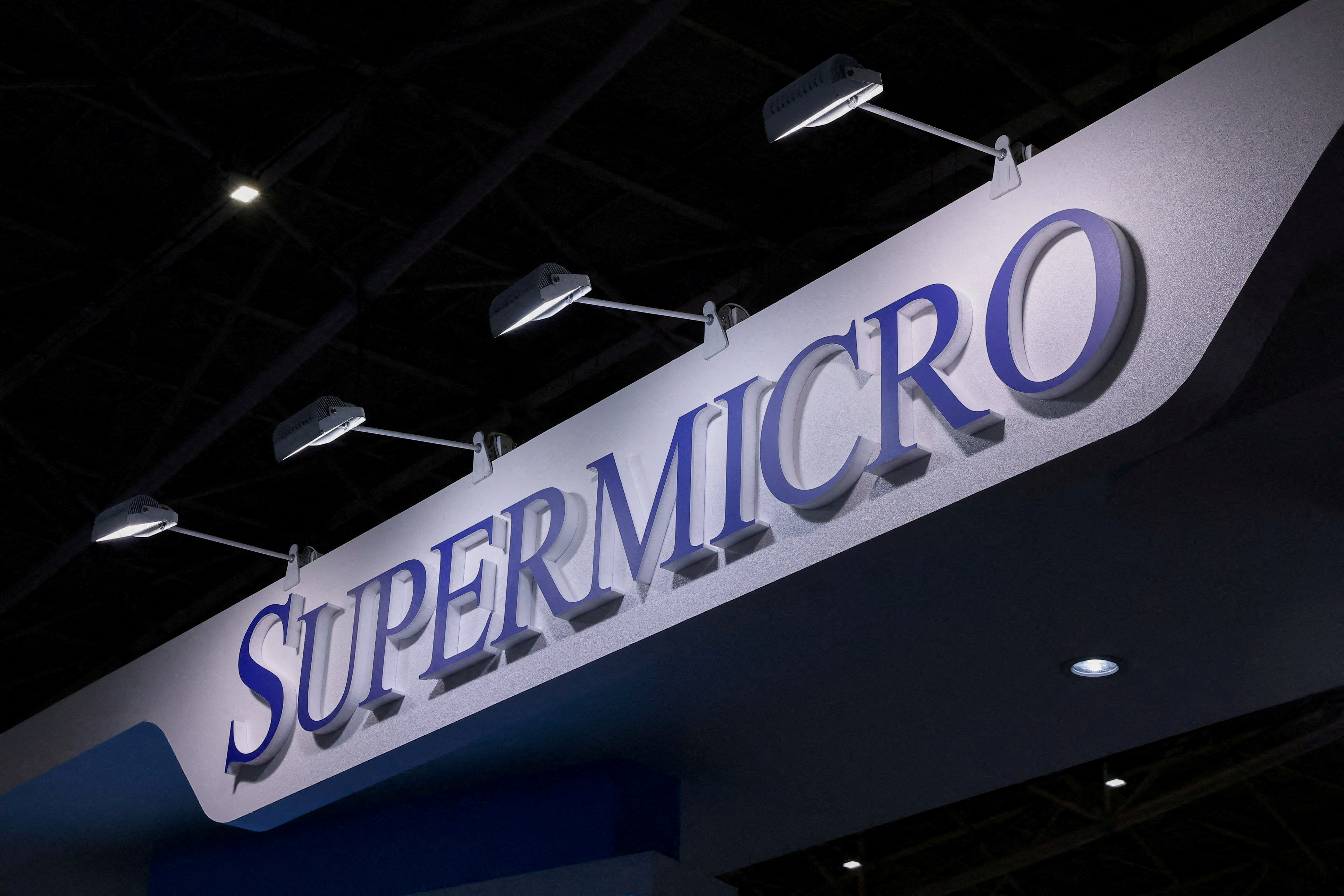
- Hindenburg Research LLC Follow
- Super Micro Computer Inc Follow
Sign up here.
Reporting by Deborah Sophia in Bengaluru; Editing by Shilpi Majumdar
Our Standards: The Thomson Reuters Trust Principles. , opens new tab
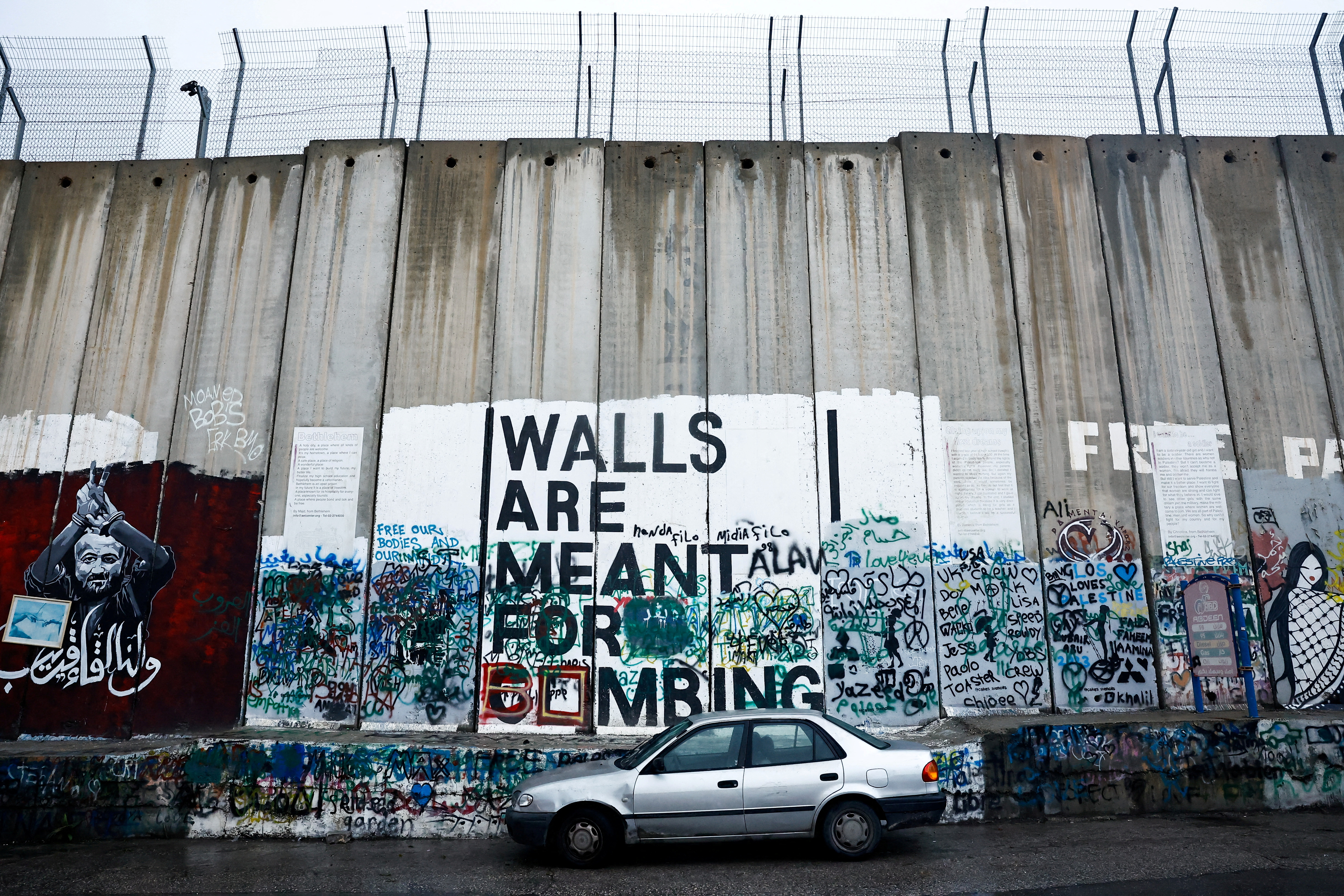
U.S. stocks tumble as September starts, investors cool on chips
Wall Street's main indexes slid on Tuesday, with the S&P 500 down more than 2% and the Nasdaq Composite down over 3% as investors softened their optimism about AI in a broad market sell-off that accelerated after tepid economic data. The benchmark S&P 500 index, Nasdaq and Dow registered their biggest daily drop since early August.
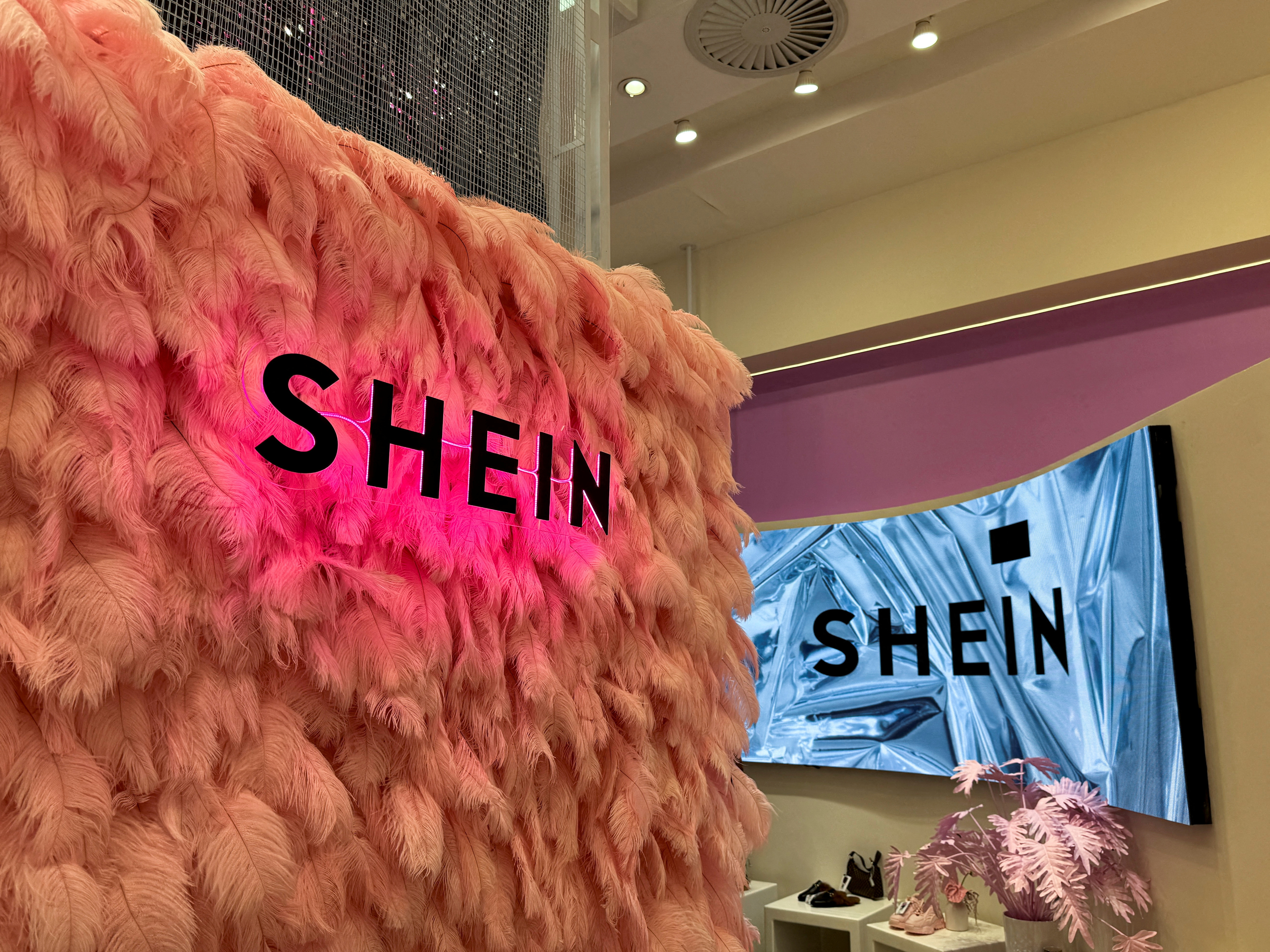

COMMENTS
The pace of technology innovation has been remarkable. Over the course of 2023 and 2024, the size of the prompts that large language models (LLMs) can process, known as "context windows," spiked from 100,000 to two million tokens. This is roughly the difference between adding one research paper to a model prompt and adding about 20 novels ...
Research Open Access 02 Sept 2024 Nature Communications. Volume: 15, P: 7629. ... streamlining cross-state licensing and billing, and adopting technology-enabled tools. ...
Deloitte's 2023 Global Technology Leadership Study explores the evolution of technology leaders, focusing on four key themes: leadership, talent, growth, and value. Insights from the futurists on Deloitte's NExT team help organizations sense—and make sense of—emerging technologies that have the potential for widespread business impact.
Once you confirm your email address, you will start receiving our latest research on the industries and business topics that matter most to you. Technology Report 2023. Bain's annual report examines the technology sector's period of renewal and reinvention, propelled by strong global demand and investments in resilient value chains and ...
Technology. Read the latest technology news on SciTechDaily, your comprehensive source for the latest breakthroughs, trends, and innovations shaping the world of technology. We bring you up-to-date insights on a wide array of topics, from cutting-edge advancements in artificial intelligence and robotics to the latest in green technologies ...
RESEARCH REPORT. Technology Vision 2024. Human by design. 15-minute read. January 9, 2024. In brief. Technology is becoming human by design, and enterprises that prepare now will win in the future. How people access and interact with information is radically changing as human-like, AI-powered chatbots synthesize vast amounts of information and ...
Gartner IT research index encompasses trusted research insights from different topics across distinctive industries to always stay ahead of the competition. ... Get the trusted insights into the major business and technology trends with free research, ebooks and special reports from Gartner. Show Filters ... Clients receive 24/7 access to ...
Information technology is the design and implementation of computer networks for data processing and communication. ... Research Open Access 30 Aug 2024 Scientific Reports. Volume: 14, P: 20220 ...
New research from MIT aims to assist in the prediction of technology performance improvement using U.S. patents as a dataset. The study describes 97 percent of the U.S. patent system as a set of 1,757 discrete technology domains, and quantitatively assesses each domain for its improvement potential. "The rate of improvement can only be ...
Read the latest Research articles in Information technology from Scientific Reports. ... Scientific Reports (Sci Rep) ISSN 2045-2322 (online) nature.com sitemap. About Nature Portfolio ...
CompTIA's IT Industry Outlook 2024 explores various aspects of the strategic and tactical sides of the technology industry, workplace and society today. Companies and individuals in the tech sector will have to decide for themselves which focus areas make most sense for the goals they are trying to accomplish, whether that's revenue growth ...
Technologies are becoming increasingly complicated and increasingly interconnected. Cars, airplanes, medical devices, financial transactions, and electricity systems all rely on more computer software than they ever have before, making them seem both harder to understand and, in some cases, harder to control. Government and corporate surveillance of individuals and information processing ...
Technavio offers over 17000 Market research reports, covering 800 technologies, spanning across 50 countries. Provides detailed insights on emerging technologies and untapped markets.
AI was implemented and examined in a wide variety of subject areas, such as science, medicine, arts, sports, engineering, mathematics, technologies, foreign language, business, history and more (See Table 3).The largest number of AIEd research studies (n = 14) were in engineering, computer science, information technology (IT), or informatics, followed by mathematics (n = 8), foreign language ...
Gartner's special report will help you stay ahead and make smarter organization decisions. Download Research! ... Clients receive 24/7 access to proven management and technology research, expert advice, benchmarks, diagnostics and more. Fill out the form to connect with a representative and learn more. Or give us a call .
New research on technological innovation from Harvard Business School faculty on issues including using data mining to improve productivity, why business IT innovation is so difficult, and the business implications of the technology revolution.
The Technology and Innovation Report 2021 urges all developing nations to prepare for a period of deep and rapid technological change that will profoundly affect markets and societies. All countries will need to ... Research of Ghana), Irmgard Nübler (ILO), Jacob Rubæk Holm (Aalborg University), Jean-Eric Aubert ...
Research Reports. Research reports present research findings and objective analysis that address the challenges facing the public and private sectors. All RAND research reports undergo rigorous peer review to ensure high standards for research quality and objectivity.
Full article: The rise of technology and impact on skills. International Journal of Training Research Volume 17, 2019 - Issue sup1: Special Open Access Supplement Issue: Emerging Labor Markets of the Future - Re-imagining Skills Development and Training, Joint Editors: Sungsup Ra, Shanti Jagannathan and Rupert Maclean.
About half of TikTok users under 30 say they use it to keep up with politics, news. TikTok users under 30 see its impact on democracy more positively than older users, with 45% of this group saying it's mostly good for American democracy. short readsJun 12, 2024.
Importance of technolog y in education. The role of technology in the field of education is four-. fold: it is included as a part of the curriculum, as an. instructional delivery system, as a ...
This sample technology research paper features: 8300 words (approx. 27 pages), an outline, and a bibliography with 48 sources. Browse other research paper examples for more inspiration. If you need a thorough research paper written according to all the academic standards, you can always turn to our experienced writers for help.
Information Technology is currently the enabler of most services. Advancements in technology has affected the society's way of living both positively and negatively. Today, most of the field of ...
User acceptance of technology is a significant focus within information systems (IS) research (Dai et al. 2024), with several models developed to explain and predict user behavior towards ...
This infographic highlights key insights shared by public and private sector experts during a recent webinar, as well as findings from a recent GovExec Insights and Research Group (IRG) report ...
The U.S. AI Safety Institute builds on NIST's more than 120-year legacy of advancing measurement science, technology, standards and related tools. Evaluations under these agreements will further NIST's work on AI by facilitating deep collaboration and exploratory research on advanced AI systems across a range of risk areas.
This report forecasts revenue growth at global, country, and regional levels and provides an analysis of the latest trends in each of the sub-segments from 2018 to 2030. For this study, Grand View Research has segmented the global portable power station market report on the basis of technology, application, and region:
The continuous worsening of the natural surroundings requires accelerating the exploration of green energy technology. Utilising ambient vibration to power electronic equipment constitutes an important measure to address the power crisis. Vibration power is widely dispersed in the surroundings, such as mechanical vibration, acoustic vibration, wind vibration, and water wave vibration ...
Background: Wearable digital health technologies and mobile apps (personal digital health technologies [DHTs]) hold great promise for transforming health research and care. However, engagement in personal DHT research is poor. Objective: The objective of this paper is to describe how participant engagement techniques and different study designs affect participant adherence, retention, and ...
Super Micro Computer on Tuesday denied claims made by short-seller Hindenburg Research in its report last week and said it contained "false or inaccurate statements" about the AI server maker.